<div align="center">
<a href="http://aima.cs.berkeley.edu/"><img src="https://raw.githubusercontent.com/aimacode/aima-python/master/images/aima_logo.png"></a><br><br>
</div>
# `aima-python` [](https://travis-ci.org/aimacode/aima-python) [](http://mybinder.org/repo/aimacode/aima-python)
Python code for the book *[Artificial Intelligence: A Modern Approach](http://aima.cs.berkeley.edu).* You can use this in conjunction with a course on AI, or for study on your own. We're looking for [solid contributors](https://github.com/aimacode/aima-python/blob/master/CONTRIBUTING.md) to help.
## Structure of the Project
When complete, this project will have Python implementations for all the pseudocode algorithms in the book, as well as tests and examples of use. For each major topic, such as `nlp` (natural language processing), we provide the following files:
- `nlp.py`: Implementations of all the pseudocode algorithms, and necessary support functions/classes/data.
- `tests/test_nlp.py`: A lightweight test suite, using `assert` statements, designed for use with [`py.test`](http://pytest.org/latest/), but also usable on their own.
- `nlp.ipynb`: A Jupyter (IPython) notebook that explains and gives examples of how to use the code.
- `nlp_apps.ipynb`: A Jupyter notebook that gives example applications of the code.
## Python 3.4 and up
This code requires Python 3.4 or later, and does not run in Python 2. You can [install Python](https://www.python.org/downloads) or use a browser-based Python interpreter such as [repl.it](https://repl.it/languages/python3).
You can run the code in an IDE, or from the command line with `python -i filename.py` where the `-i` option puts you in an interactive loop where you can run Python functions. All notebooks are available in a [binder environment](http://mybinder.org/repo/aimacode/aima-python). Alternatively, visit [jupyter.org](http://jupyter.org/) for instructions on setting up your own Jupyter notebook environment.
## Installation Guide
To download the repository:
`git clone https://github.com/aimacode/aima-python.git`
Then you need to install the basic dependencies to run the project on your system:
`pip install -r requirements.txt`
You also need to fetch the datasets from the [`aima-data`](https://github.com/aimacode/aima-data) repository:
```
cd aima-python
git submodule init
git submodule update
```
Wait for the datasets to download, it may take a while. Once they are downloaded, you need to install `pytest`, so that you can run the test suite:
`pip install pytest`
Then to run the tests:
`py.test`
And you are good to go!
# Index of Algorithms
Here is a table of algorithms, the figure, name of the algorithm in the book and in the repository, and the file where they are implemented in the repository. This chart was made for the third edition of the book and is being updated for the upcoming fourth edition. Empty implementations are a good place for contributors to look for an issue. The [aima-pseudocode](https://github.com/aimacode/aima-pseudocode) project describes all the algorithms from the book. An asterisk next to the file name denotes the algorithm is not fully implemented. Another great place for contributors to start is by adding tests and writing on the notebooks. You can see which algorithms have tests and notebook sections below. If the algorithm you want to work on is covered, don't worry! You can still add more tests and provide some examples of use in the notebook!
| **Figure** | **Name (in 3<sup>rd</sup> edition)** | **Name (in repository)** | **File** | **Tests** | **Notebook**
|:-------|:----------------------------------|:------------------------------|:--------------------------------|:-----|:---------|
| 2 | Random-Vacuum-Agent | `RandomVacuumAgent` | [`agents.py`][agents] | Done | Included |
| 2 | Model-Based-Vacuum-Agent | `ModelBasedVacuumAgent` | [`agents.py`][agents] | Done | Included |
| 2.1 | Environment | `Environment` | [`agents.py`][agents] | Done | Included |
| 2.1 | Agent | `Agent` | [`agents.py`][agents] | Done | Included |
| 2.3 | Table-Driven-Vacuum-Agent | `TableDrivenVacuumAgent` | [`agents.py`][agents] | Done | Included |
| 2.7 | Table-Driven-Agent | `TableDrivenAgent` | [`agents.py`][agents] | Done | Included |
| 2.8 | Reflex-Vacuum-Agent | `ReflexVacuumAgent` | [`agents.py`][agents] | Done | Included |
| 2.10 | Simple-Reflex-Agent | `SimpleReflexAgent` | [`agents.py`][agents] | Done | Included |
| 2.12 | Model-Based-Reflex-Agent | `ReflexAgentWithState` | [`agents.py`][agents] | Done | Included |
| 3 | Problem | `Problem` | [`search.py`][search] | Done | Included |
| 3 | Node | `Node` | [`search.py`][search] | Done | Included |
| 3 | Queue | `Queue` | [`utils.py`][utils] | Done | No Need |
| 3.1 | Simple-Problem-Solving-Agent | `SimpleProblemSolvingAgent` | [`search.py`][search] | Done | Included |
| 3.2 | Romania | `romania` | [`search.py`][search] | Done | Included |
| 3.7 | Tree-Search | `depth/breadth_first_tree_search` | [`search.py`][search] | Done | Included |
| 3.7 | Graph-Search | `depth/breadth_first_graph_search` | [`search.py`][search] | Done | Included |
| 3.11 | Breadth-First-Search | `breadth_first_graph_search` | [`search.py`][search] | Done | Included |
| 3.14 | Uniform-Cost-Search | `uniform_cost_search` | [`search.py`][search] | Done | Included |
| 3.17 | Depth-Limited-Search | `depth_limited_search` | [`search.py`][search] | Done | Included |
| 3.18 | Iterative-Deepening-Search | `iterative_deepening_search` | [`search.py`][search] | Done | Included |
| 3.22 | Best-First-Search | `best_first_graph_search` | [`search.py`][search] | Done | Included |
| 3.24 | A\*-Search | `astar_search` | [`search.py`][search] | Done | Included |
| 3.26 | Recursive-Best-First-Search | `recursive_best_first_search` | [`search.py`][search] | Done | Included |
| 4.2 | Hill-Climbing | `hill_climbing` | [`search.py`][search] | Done | Included |
| 4.5 | Simulated-Annealing | `simulated_annealing` | [`search.py`][search] | Done | Included |
| 4.8 | Genetic-Algorithm | `genetic_algorithm` | [`search.py`][search] | Done | Included |
| 4.11 | And-Or-Graph-Search | `and_or_graph_search` | [`search.py`][search] | Done | Included |
| 4.21 | Online-DFS-Agent | `online_dfs_agent` | [`search.py`][search] | Done | Included |
| 4.24 | LRTA\*-Agent | `LRTAStarAgent` | [`search.py`][search] | Done | Included |
| 5.3 | Minimax-Decision | `minimax_decision` | [`games.py`][games] | Done | Included |
| 5.7 | Alpha-Beta-Search | `alphabeta_search` | [`games.py`][games] | Done | Included |
| 6 | CSP

weixin_39841856
- 粉丝: 491
- 资源: 1万+
最新资源
- 中科岩创隧道自动化监测解决方案
- Manatee 1.09电磁噪声振动计算软件:引领电机NVH领域革新,带教程易上手,超越同类软件的强大后处理功能,电机电磁振动噪声NVH解决利器:Manatee 1.09软件教程全面,强大后处理参数化
- C2000 MCU同步降压升压转换器的数字控制系统应用与优化
- .NET 9 彻底改变了 API 的文档:从 Swashbuckle 到 Scalar
- datax-mysql8驱动
- 气动影响下叶片裂纹应力集中现象的Fluent分析,基于气动影响的叶片裂纹应力集中现象的Fluent分析与研究,Fluent,考虑气动影响情况下的叶片裂纹应力集中 ,Fluent; 考虑气动影响; 叶片
- MATLAB驱动的高尔夫模拟仿真系统:深度定制球杆与挥杆参数的互动体验,基于MATLAB的全方位高尔夫模拟仿真系统:精确设定球杆与天气因素,让用户享受个性化的挥杆力量与角度掌控体验,基于MATLAB的
- 单向光伏并网逆变器:结构解析与性能追踪图集,包括整体结构图、并网电流电压曲线图、最大功率追踪MPPT控制图及直流母线电压曲线图,单向光伏并网逆变器:结构解析与性能追踪图集,含最大功率追踪图及电流电压曲
- COMSOL裂缝地层THM耦合离散模型与地热能开采过程研究:探究随机复杂裂缝对增强地热系统的影响,COMSOL裂缝地层THM耦合与离散随机复杂裂缝模型在地热能开采中的应用研究,COMSOL裂缝地层的T
- 基于C2000 MCU峰值电流控制模式的升压电路实现在电源领域的应用与优化
- site-packages.rar
- BUCK控制策略对比及主电路图详解:开环与闭环控制的波形与调节过程分析,BUCK控制策略对比及主电路图详解:开环与闭环控制的波形与调节过程分析,BUCK多种控制策略对比 图一BUCK主电路图与控制策略
- 永磁同步电机MATLAB仿真:直接转矩控制下的转速外环与磁链内环优化,转矩脉动显著减小,永磁同步电机MATLAB仿真:直接转矩控制下的转速外环与磁链内环优化,转矩脉动显著减小,永磁同步电机(PMSM)
- 清华大学最新学习教程《DeepSeek与AI幻觉》
- 销售数据集,数据集包含模拟不同产品、地区和客户的销售交易信息,可以用于机器学习
- 可以快速使用的一个日志模块,可以打印不同颜色,绑定到RichTextBox
资源上传下载、课程学习等过程中有任何疑问或建议,欢迎提出宝贵意见哦~我们会及时处理!
点击此处反馈


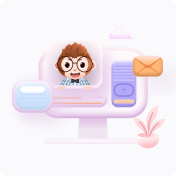