ShuffleNet_V2_pytorch_caffe
=======================================
ShuffleNet-V2 for both PyTorch and Caffe.
This project supports both Pytorch and Caffe.
Multiple kinds of model width are supported.
Model with should be 0.25, 0.33, 0.5, 1.0, 1.5 or 2.0, other model width are not supported.
Usage
=======================================
PyTorch
---------------------------------------
Just use shufflenet_v2.py as following.
```
import shufflenet_v2
num_classes = 1000
model_width = 0.5
shufflenet_v2.Network(num_classes, model_width)
params = torch.load('shufflenet_v2_x0.5.pth', map_location=lambda storage, loc: storage)
net.load_state_dict(params)
```
Caffe
---------------------------------------
Prototxt files can be generated by shufflenet_v2.py
```
python shufflenet_v2.py --save_caffe net --num_classes 1000 --model_width 1.0
```
Converting Model from PyTorch to Caffe
---------------------------------------
```
python shufflenet_v2.py --load_pytorch net.pth --save_caffe net --num_classes 1000 --model_width 1.0
```
Pretrained ImageNet Models for PyTorch and Caffe
=======================================
Pretrained models can be downloaded from: https://github.com/miaow1988/ShuffleNet_V2_pytorch_caffe/releases
* shufflenet_v2_x0.25, Top-1 Acc = 46.04%. Unofficial.
* shufflenet_v2_x0.33, Top-1 Acc = 51.40%. Unofficial.
* shufflenet_v2_x0.50, Top-1 Acc = 58.93%. This accuracy is 1.37% lower compared with the result in the official paper.
Training Details
---------------------------------------
1. All ImageNet images are resized by a short edge size of 256 (bicubic interpolation by PIL). And then each of them are pickled by Python and stored in a LMDB dataset.
2. Training is done by PyTorch 0.4.0
3. data augmentation: 224x224 random crop and random horizontal flip. No image mean extraction is used here, which is done automatically by data/bn layers in the network.
4. As in my codes, networks are initialized by nn.init.kaiming_normal_(m.weight, mode='fan_out').
5. A SGD with nesterov momentum (0.9) is used for optimizing. The batch size is 1024. Models are trained by 300000 iterations, while the learning rate decayed linearly from 0.5 to 0.
Something you might have noticed
---------------------------------------
1. Models are trained by PyTorch and converted to Caffe. Thus, you should use scale parameter in Caffe's data layer to make sure all input images are rescaled from [0, 255] to [0, 1].
2. The RGB~BGR problem is not very crucial, you may just ignore the difference if you are use these models as pretrained models for other tasks.
Others
---------------------------------------
All these years, I barely achieved same or higher results of different kinds of complex ImageNet models reported in papers. If you got a better accuracy, please tell me.
没有合适的资源?快使用搜索试试~ 我知道了~
Python-ShuffleNetV2的PyTorch和Caffe实现
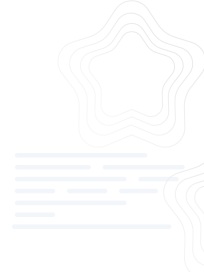
共10个文件
prototxt:4个
py:2个
license:1个

需积分: 47 14 下载量 133 浏览量
2019-08-11
05:49:42
上传
评论 1
收藏 22KB ZIP 举报
温馨提示
近日,旷视科技提出针对移动端深度学习的第二代卷积神经网络 ShuffleNet V2。研究者指出过去在网络架构设计上仅注重间接指标 FLOPs 的不足,并提出两个基本原则和四项准则来指导网络架构设计,最终得到了无论在速度还是精度上都超越先前最佳网络(例如 ShuffleNet V1、MobileNet 等)的 ShuffleNet V2。
资源推荐
资源详情
资源评论
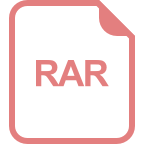
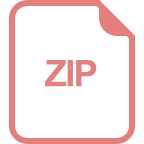
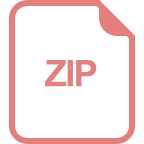
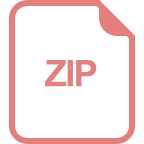
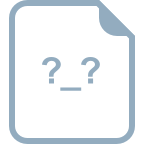
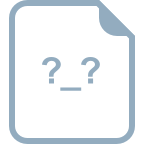
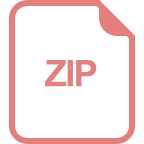
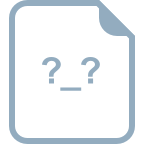
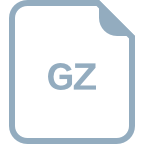
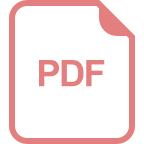
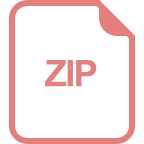
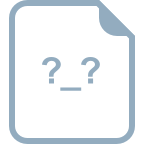
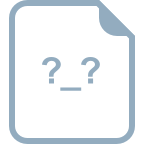
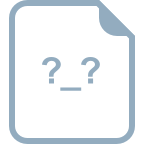
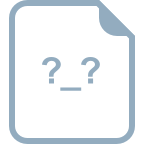
收起资源包目录


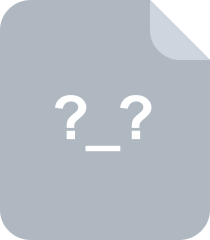
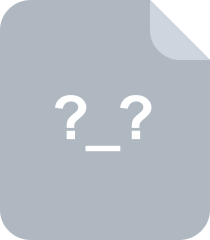
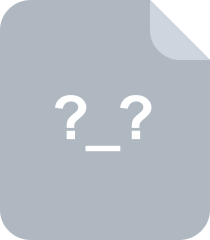
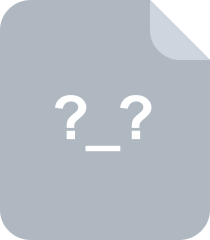
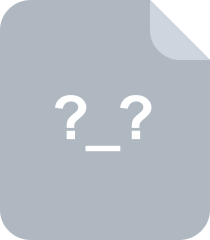
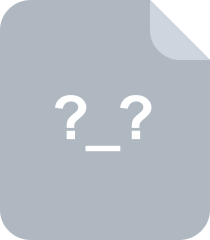
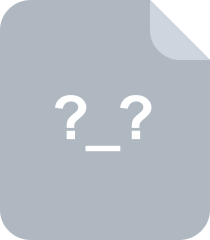
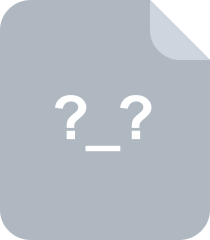
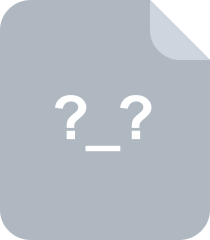
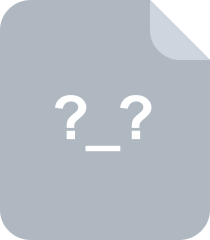
共 10 条
- 1
资源评论
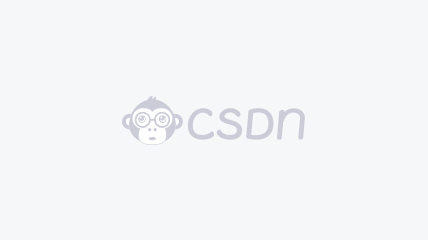

weixin_39840914
- 粉丝: 436
- 资源: 1万+
上传资源 快速赚钱
我的内容管理 展开
我的资源 快来上传第一个资源
我的收益
登录查看自己的收益我的积分 登录查看自己的积分
我的C币 登录后查看C币余额
我的收藏
我的下载
下载帮助

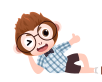
最新资源
资源上传下载、课程学习等过程中有任何疑问或建议,欢迎提出宝贵意见哦~我们会及时处理!
点击此处反馈


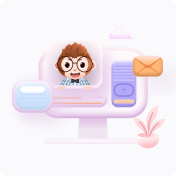
安全验证
文档复制为VIP权益,开通VIP直接复制
