没有合适的资源?快使用搜索试试~ 我知道了~
信息共享对于多代理团队在动态且部分可观察的环境中进行合作决策至关重要。 除了建立完整的信息覆盖范围之外,如果团队中的座席可以自我指导以评估潜在的接收者以及在何处进行通信,则协调效率将大大提高。 尽管已经在本体图匹配和自然语言处理方面开发了对信息评估方法的深入研究,但是这些模型无法对大型分散代理的动态协调执行快速推理。 在本文中,我们提出了一种基于复杂网络图模型的快速信息评估方法,该方法有助于表明信息的重要性。 类似于人为的模糊信息评估,关键在于重要信息始终会随着合并而显着改变其复杂信息图。 因此,我们在图模型中计算该新信息的基于语义的值,并构建局部图评估算法来估计信息图的演化,而不是执行昂贵的完整图搜索。 优点是可以轻松地将本地评估算法转换为座席信息库中的有效查询,以便他们可以快速做出决策。 尽管该决定可能并不精确,但类似于人与人之间的交流,信息共享性能足以在多主体团队中传播有价值的信息。 我们在多主体合作案例研究中证明了提出的信息评估方法的可行性。
资源推荐
资源详情
资源评论
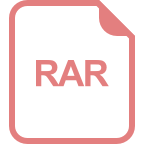
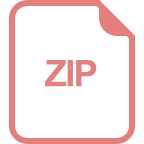
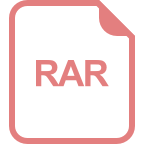
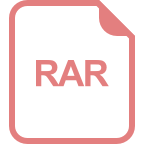
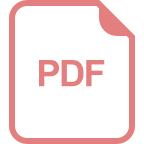
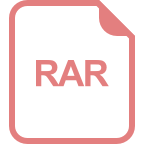
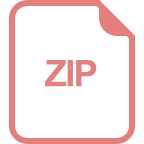
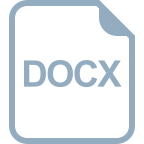
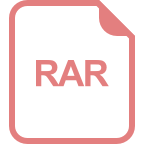
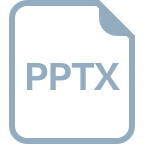
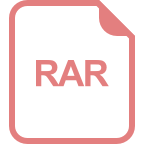
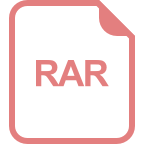
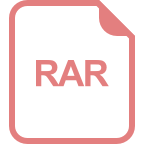
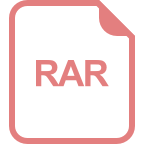
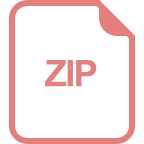
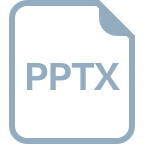
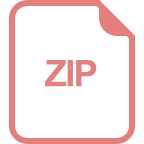
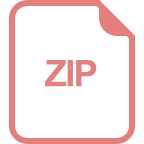
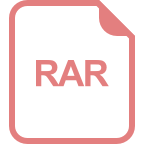
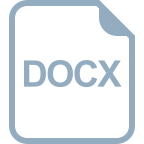
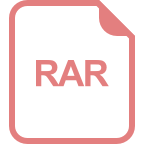
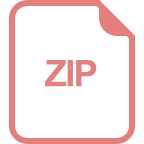
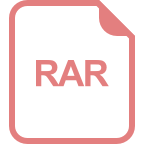
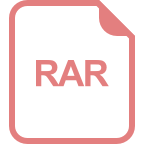
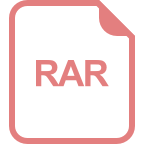
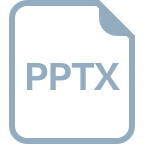
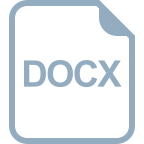
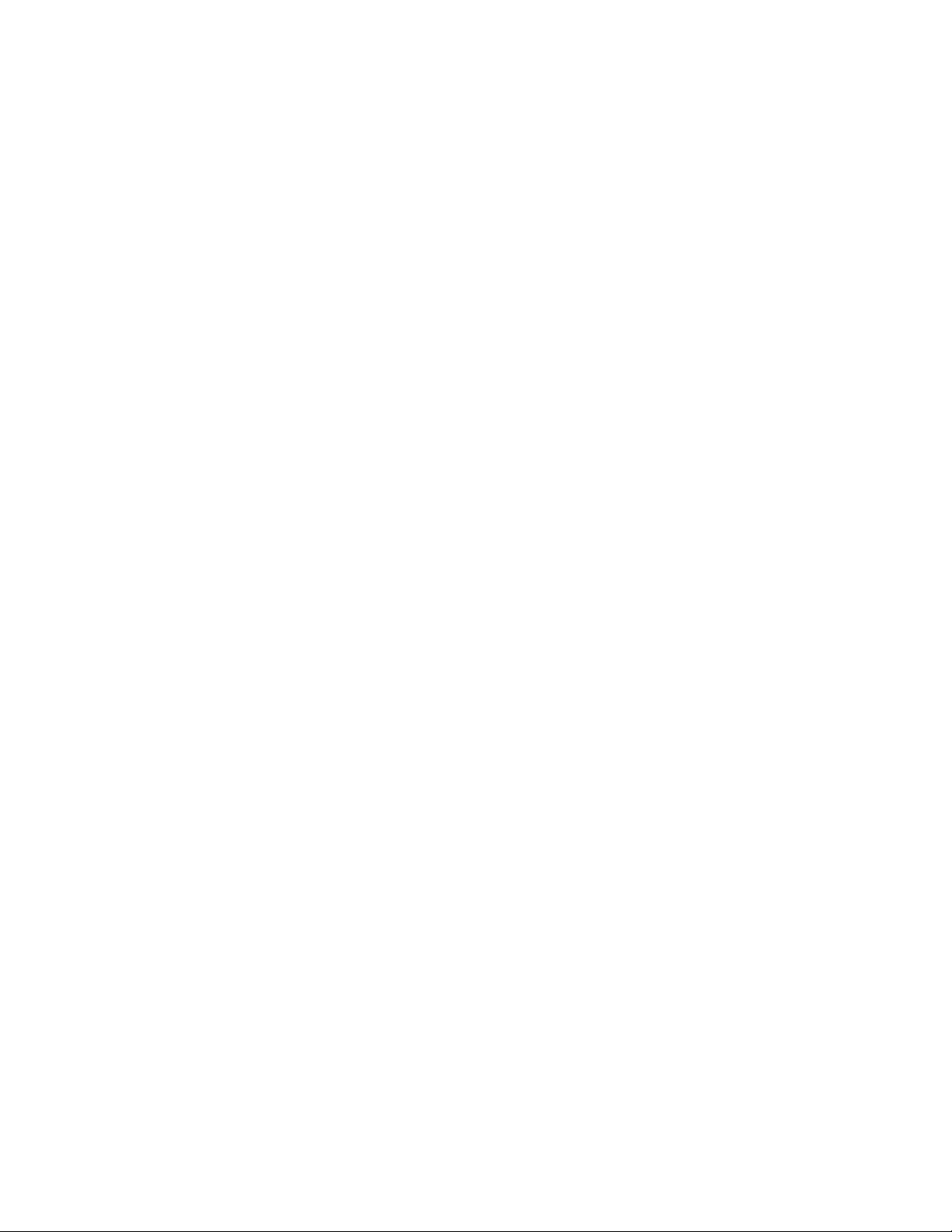
A Fast Graphic-based Information Valuation
Algorithm for Cooperative Information Sharing
Haixiao Hu, Yang Xu*, Yulin Zhang, Ming Liu
School of Computer Science and Engineering, University of Electronic Science and Technology of China
Chengdu, Sichuan, 611731, P.R.China
Email: xuyang@uestc.edu.cn
Abstract—Information sharing is critical to multi-agent team
for cooperative decision making in dynamic and partially ob-
servable environments. Other than building a full information
coverage, if agents in a team can be self-directed to valuate a
potential receiver and where to communicate, the coordination
efficiency will be greatly enhanced. Although intensive studies
of information valuation approaches have been developed in
ontology graph matching and natural language processing, these
models fail to perform fast reasoning for large-scale decentralized
agents dynamic coordination. In this paper, we propose a fast
information valuation approach based on a complex network
graph model, which helps to indicate the information importance.
Similar to vague information valuation by human, the key is that
important information always significantly changes their complex
information graph with its incorporation. Therefore, we calculate
the semantic based value of this new information in a graph
model and build a local graph evaluation algorithm to estimate
information graph evolution, instead of performing expensive
complete graph search. The advantage is that the local valuation
algorithm can be easily transformed into efficient queries in
agents’ information base so that they can make fast decisions.
Although the decision may not be precise, similar to human
communication, the information sharing performance is good
enough to disseminate valuable information in the multi-agent
team. We demonstrate the feasibility of the proposed information
valuation approach in a multi-agent cooperation case study.
I. INTRODUCTION
Multi-agent coordination is popular in various application
domains including disaster response, anti-terrorism and explo-
ration [8]. In those systems, agents are required to complete
tasks cooperatively to achieve the common goal. However,
when the number of agents scale up, they are highly distributed
and have to operate in a dynamic and partially observable
environment. In this case, information sharing is critical for
distributed agents to make rational decisions [11]. Although
intensive studies have been carried out, state of the art fails to
build practical algorithms for large-scale multi-agent systems,
where agents are required to evaluate how a potential receiver
could benefit from a given information whilst considering
the communication cost. With decision-theoretic approaches,
decentralized agents inherit the constraint of partial observ-
ability and their valuation falls within DEC-POMDP model,
which is intrinsically NEXP-COMPLETE. Therefore, precise
computation of information utilities is either infeasible or
computational costly in a large system [2].
Heuristic approaches for the information sharing problem
always start from the assumption that communication cost can
be rationally estimated [4]. Given a good knowledge of the
potential receiver, e.g., in [10], the receivers’ knowledge can
be built solely from their incoming messages, the agent should
be able to score the importance of the new information if it is
incorporated into the receiver’s knowledge. There have been
many approaches. For example, most straightforwardly, the
information importance can be crudely counted by checking
whether it is already known by the receiver explicitly or
implicitly [7]. Some other researches calculate agent’s belief,
and approximate the information importance with two criteria:
improvement to reach a synchronized belief [9] or reduction
of the uncertainty [3]. However, those algorithms are of high
computation cost to perform intensive information base scan
throughout to build statistical or probability inference model,
and have poor performance in a large multi-agent system. In
the semantic web community, the information base is always
abstracted into a semantic graph [5]. Based on this graph,
either statistic or graph searching algorithms are put forward
[12]. In such models, agents in a team have to maintain a
complete view of knowledge graphs for each potential receiver
so that information valuation can be performed. However,
it is of high memory cost as well as long decision making
times, due to its computational complexity. Therefore, a fast
information valuation method that matches the needs of large-
scale multi-agent information sharing decision is still very
necessary.
II. PROBLEM STATEMENT AND MODELING
A. Information Sharing Problem
In large teams, decentralized agents have to make decisions
about how to share information based on their limited obser-
vation of the team. Due to their heterogeneities, not all agents
in the system are interested in a given piece of information
[13]. In this case, other than building full information coverage
around the team, information sharing aims at ensuring that
agents get the information they are interested in at a timely
manner. For example, in a city rescue domain, an agent
driving the fire track cares more about the city traffic condition
than the news of finding a victim. Therefore, to effectively
share information, agents have to estimate their teammates’
information needs from their own knowledge.
More generally, let agent a be the candidate receiver of
information I, and IB = {I
1
, I
2
, ..., I
k
, ...} denotes its infor-
mation base, which contains a set of information known or
2017 IEEE International Conference on Systems, Man, and Cybernetics (SMC)
Banff Center, Banff, Canada, October 5-8, 2017
978-1-5386-1644-4/17/$31.00 ©2017 IEEE 1892
资源评论
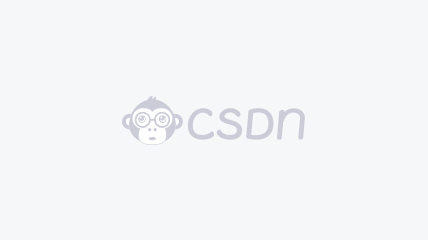

weixin_38707153
- 粉丝: 7
- 资源: 949
上传资源 快速赚钱
我的内容管理 展开
我的资源 快来上传第一个资源
我的收益
登录查看自己的收益我的积分 登录查看自己的积分
我的C币 登录后查看C币余额
我的收藏
我的下载
下载帮助

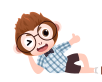
最新资源
资源上传下载、课程学习等过程中有任何疑问或建议,欢迎提出宝贵意见哦~我们会及时处理!
点击此处反馈


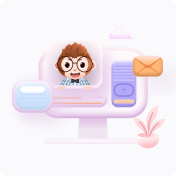
安全验证
文档复制为VIP权益,开通VIP直接复制
