没有合适的资源?快使用搜索试试~ 我知道了~
Neighborhood Correlation Analysis for Semi-paired Two-View Data
0 下载量 134 浏览量
2021-02-09
00:06:57
上传
评论
收藏 635KB PDF 举报
温馨提示
Canonical correlation analysis (CCA) is a widely used technique for analyzing two datasets (two views of the same objects). However, CCA needs that the samples of the two views are fully-paired. Actually, we are often faced up with the semi-paired scenario where the number of available paired samples is limited and yet the number of unpaired samples is sufficient. For such a scenario, CCA is generally prone to overfitting and thus performs poorly, since its definition itself makes it only able t
资源推荐
资源详情
资源评论
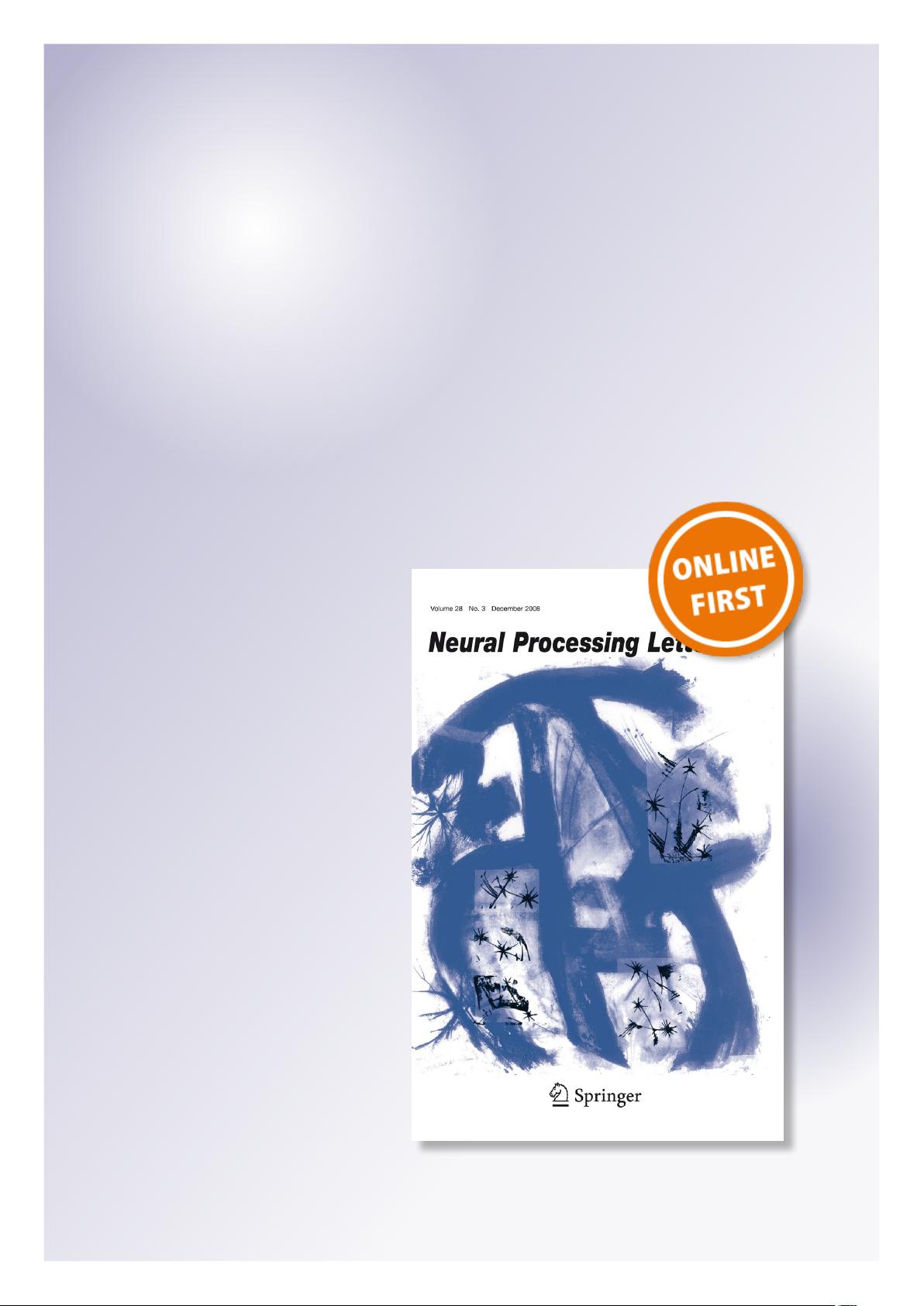
1 23
Neural Processing Letters
ISSN 1370-4621
Neural Process Lett
DOI 10.1007/s11063-012-9251-z
Neighborhood Correlation Analysis for
Semi-paired Two-View Data
Xudong Zhou, Xiaohong Chen &
Songcan Chen
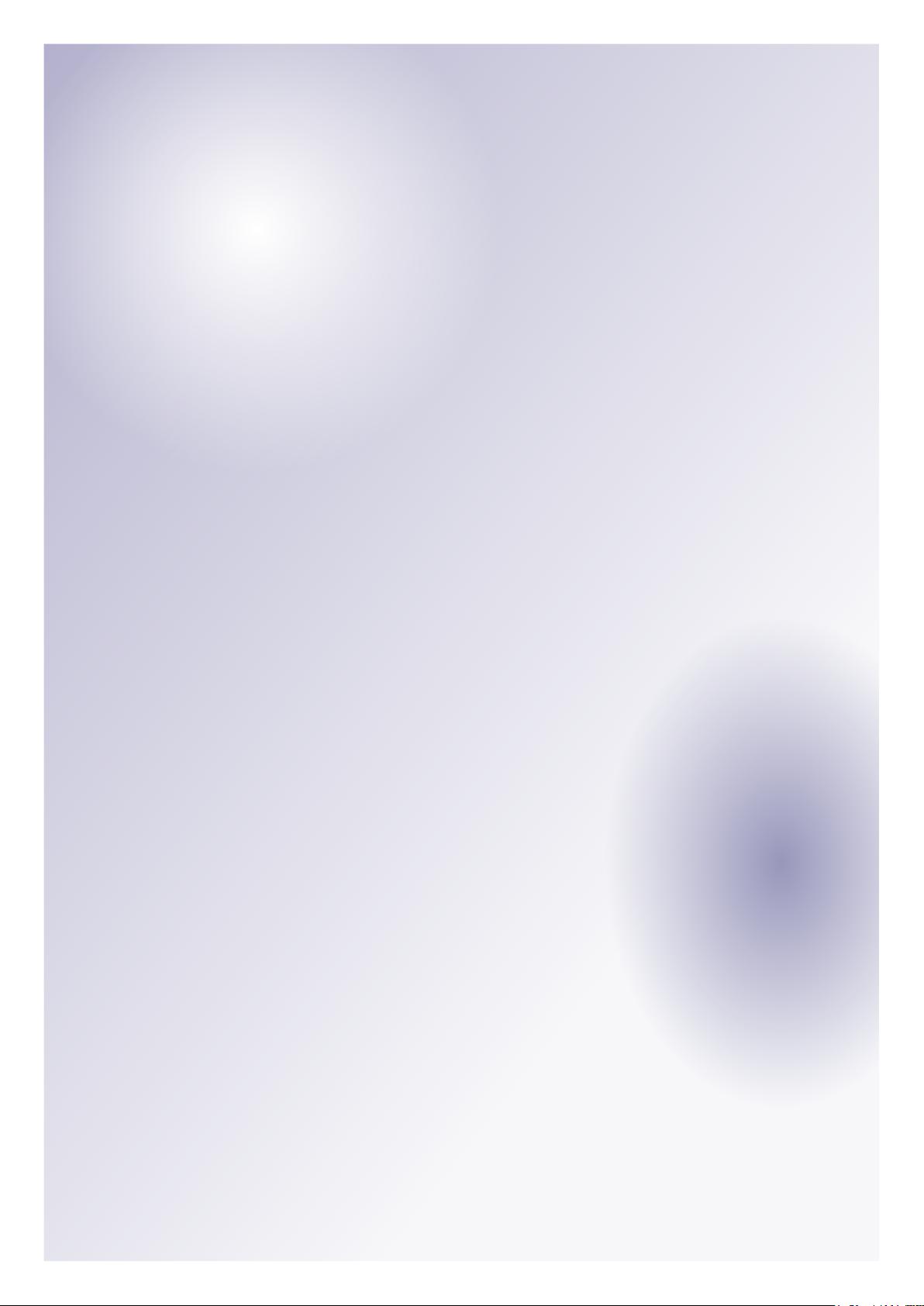
1 23
Your article is protected by copyright and all
rights are held exclusively by Springer Science
+Business Media New York. This e-offprint is
for personal use only and shall not be self-
archived in electronic repositories. If you
wish to self-archive your work, please use the
accepted author’s version for posting to your
own website or your institution’s repository.
You may further deposit the accepted author’s
version on a funder’s repository at a funder’s
request, provided it is not made publicly
available until 12 months after publication.
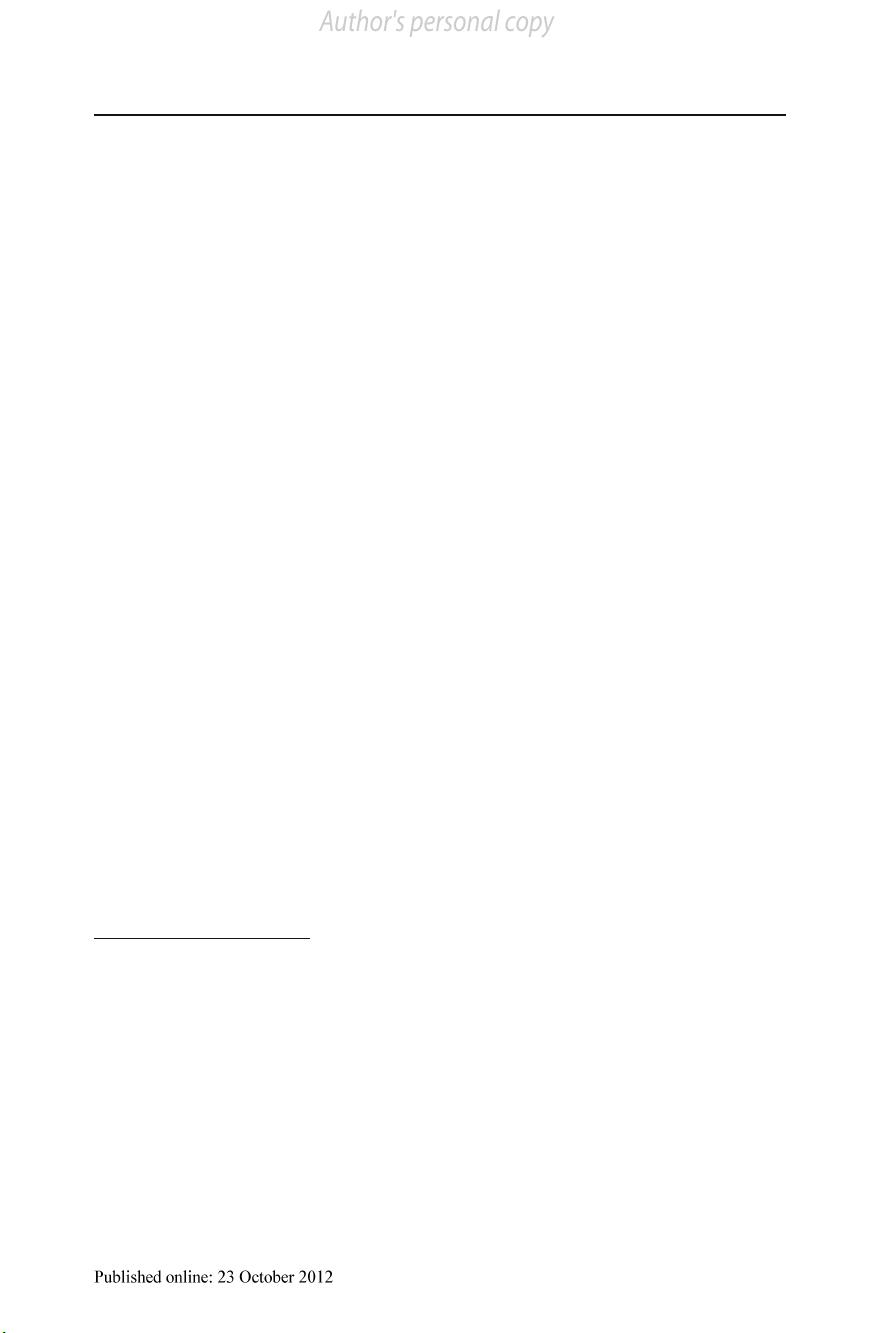
Neural Process Lett
DOI 10.1007/s11063-012-9251-z
Neighborhood Correlation Analysis for Semi-paired
Two-View Data
Xudong Zhou · Xiaohong Chen · Songcan Chen
© Springer Science+Business Media New York 2012
Abstract Canonical correlation analysis (CCA) is a widely used technique for analyzing
two datasets (two views of the same objects). However, CCA needs that the samples of the
two views are fully-paired. Actually, we are often faced up with the semi-paired scenario
where the number of available paired samples is limited and yet the number of unpaired
samples is sufficient. For such a scenario, CCA is generally prone to overfitting and thus
performs poorly, since its definition itself makes it only able to utilize those paired samples.
To overcome such a shortcoming, several semi-paired variants of CCA have been proposed.
However, unpaired samples in these methods are just used in the way of single-view leaning
to capture individual views’ structure information for regularizing CCA. Intuitively, using
unpaired samples in the way of two-view learning should be more natural and more attrac-
tive since CCA itself is a two-view learning method. As a result, a novel CCAs semi-paired
variant named Neighborhood Correlation Analysis (NeCA), which uses unpaired samples
in the two-view learning way, is developed through incorporating between-view neighbor-
hood relationships into CCA. The relationships are acquired through leveraging within-view
neighborhood relationships of each view’s all data (including paired and unpaired data) and
between-view paired information. Thus, it can take more sufficient advantage of the unpaired
samples and then mitigate overfitting effectively caused by the limited paired data. Promising
X. Zhou · S. Chen (
B
)
College of Computer Science and Technology, Nanjing University of Aeronautics & Astronautics,
Nanjing 210016, China
e-mail: s.chen@nuaa.edu.cn
X. Zhou
e-mail: xdzhou@nuaa.edu.cn
X. Zhou
Information Engineering College, Yangzhou University, Yangzhou 225127, China
X. Chen
College of Science, Nanjing University of Aeronautics & Astronautics, Nanjing 210016, China
S. Chen
National Key Laboratory for Novel Software Technology, Nanjing University, Nanjing 210093, China
123
Author's personal copy
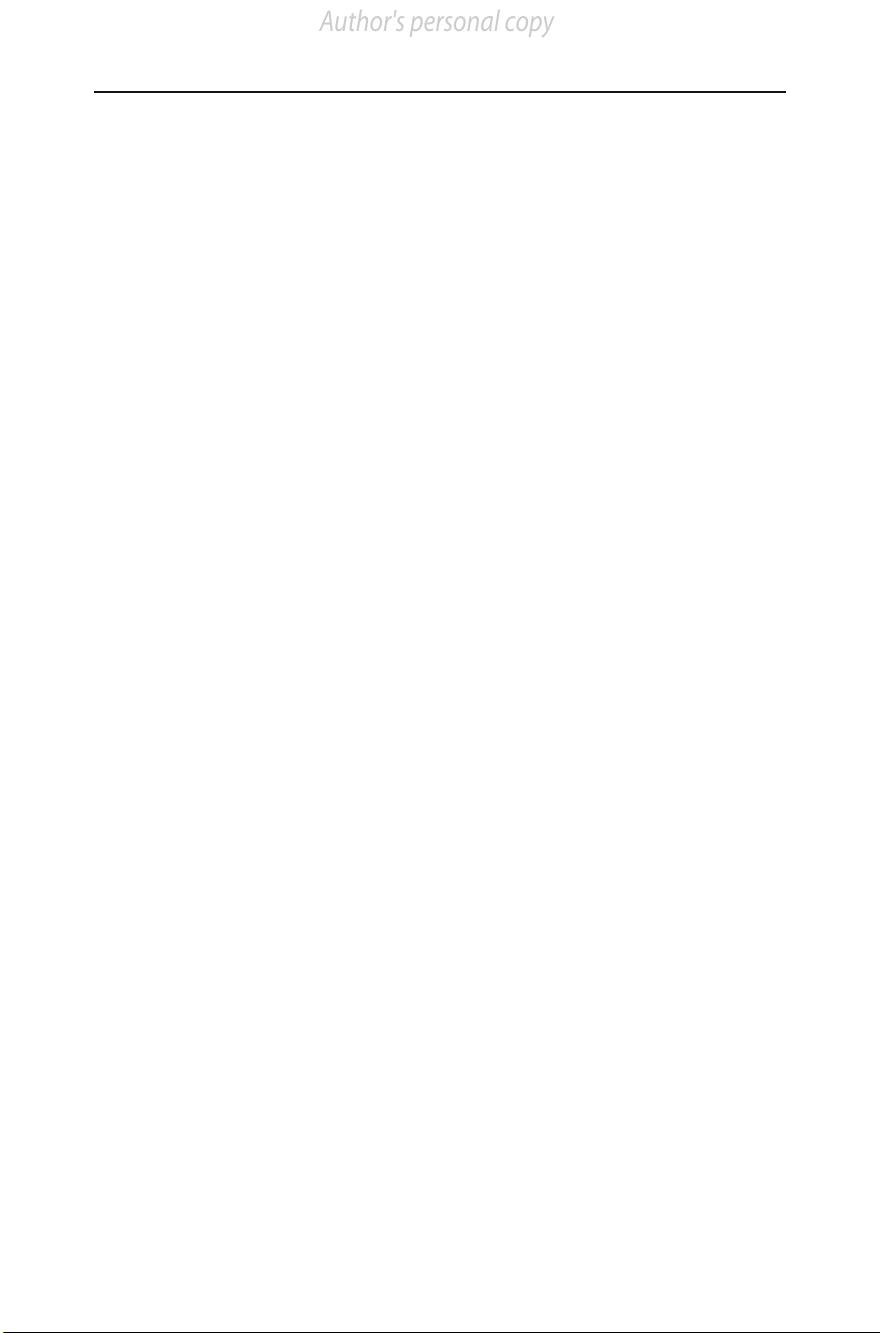
X. Zhou et al.
experiments results on several popular multi-view datasets show its feasibility and effective-
ness.
Keywords Canonical correlation analysis · Semi-paired learning · Two-view learning ·
Neighborhood relationship · Neighborhood correlation
1 Introduction
High-dimensional co-occurring data associated with an object frequently and abundantly
emerge in the real world. For example, an Internet web page as an object can be repre-
sented as (co-occurring) page text and links to the page, and a human can be represented as
co-occurring visual and audio contents. A lot of works have been done for analyzing this
kind of data [1–7]. Among these works, canonical correlation analysis (CCA) is one of the
most widely adopted methods [8–12].
CCA is a classical but useful multivariate statistical analysis method [13]. It aims to find
maximally correlated projections between two sets of variables, which can be considered
as two views (views x and y) or representations of the same set of objects. However CCA
requires that such two views be fully-paired, i.e., each sample in view x should have a corre-
spondence in view y, and vice versa. Conversely, we are often faced such a scenario where
most samples in view x have no correspondences in view y, and vice versa, thus forming
the semi-paired scenario called here. For such a scenario, CCA is generally prone to overfit-
ting and thus performs poorly, since its definition itself makes it only suitful for the paired
scenario, so its applications are limited in the real world. Actually, abundant unpaired sam-
ples (i.e. x-andy-only samples) often contain much useful information which will benefit
the learning task, just as the unlabeled samples benefit semi-supervised leaning [14,15]by
exploiting the intrinsic data structure under clustering assumption or manifold assumption.
Recently, several works have concerned such new scenario [16–18]. Blaschko et al. [16]pro-
posed a semi-supervised Laplacian regularization of kernel CCA (SemiLRKCCA), which
utilizes intrinsic geometry structure of each view to regularize kernel CCA (KCCA) [19].
As a result, SemiLRKCCA can find a set of meaningful directions which not only make the
two view’s paired samples highly correlated but also capture each view’s manifold struc-
ture. SemiCCA [17] utilizes global structure of each view’s whole training samples (paired
and unpaired samples together) to regularize CCA in order to bridge CCA and principal
component analysis (PCA) [20,21] seamlessly. Both SemiLRKCCA and SemiCCA can take
sufficient advantage of unpaired samples in addition to paired samples, and consequently
achieve better results than CCA just based on the paired samples. It is necessary to mention
that the actual meaning of “semi-” in SemiLRKCCA and SemiCCA is “semi-paired” rather
than “semi-supervised” in popular semi-supervised learning literature [14,15]. Compared
with SemiLRKCCA and SemiCCA, more recent work termed as semi-paired and semi-
supervised generalized correlation analysis (S
2
GCA) [18] make further research for dealing
with semi-paired and semi-supervised scenario. S
2
GCA utilizes within-view structural infor-
mation and within-view discriminant information jointly, to preserve the individual view’s
structure of unlabeled data and separate labeled data in different classes from each other
simultaneously. Without semi-supervised information, S
2
GCA is similar to SemiLRKCCA
and SemiCCA.
In SemiLRKCCA, SemiCCA and S
2
GCA, unpaired samples are just used in the way of
single-view leaning to capture individual views’ structure information for regularizing KCCA
or CCA. Consequently, CCA and its variants (SemiLRKCCA, SemiCCA and S
2
GCA) only
123
Author's personal copy
剩余21页未读,继续阅读
资源评论
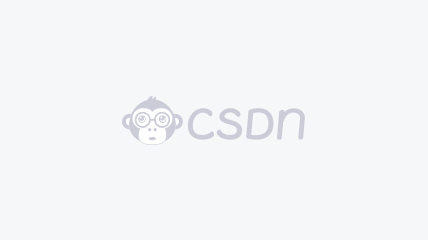

weixin_38663197
- 粉丝: 8
- 资源: 926
上传资源 快速赚钱
我的内容管理 展开
我的资源 快来上传第一个资源
我的收益
登录查看自己的收益我的积分 登录查看自己的积分
我的C币 登录后查看C币余额
我的收藏
我的下载
下载帮助

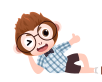
最新资源
- bdwptqmxgj11.zip
- onnxruntime-win-x86
- onnxruntime-win-x64-gpu-1.20.1.zip
- vs2019 c++20 语法规范 头文件 <ratio> 的源码阅读与注释,处理分数的存储,加减乘除,以及大小比较等运算
- 首次尝试使用 Win,DirectX C++ 中的形状渲染套件.zip
- 预乘混合模式是一种用途广泛的三合一混合模式 它已经存在很长时间了,但似乎每隔几年就会被重新发现 该项目包括使用预乘 alpha 的描述,示例和工具 .zip
- 项目描述 DirectX 引擎支持版本 9、10、11 库 Microsoft SDK 功能相机视图、照明、加载网格、动画、蒙皮、层次结构界面、动画控制器、网格容器、碰撞系统 .zip
- 项目 wiki 文档中使用的代码教程的源代码库.zip
- 面向对象的通用GUI框架.zip
- 基于Java语言的PlayerBase游戏角色设计源码
资源上传下载、课程学习等过程中有任何疑问或建议,欢迎提出宝贵意见哦~我们会及时处理!
点击此处反馈


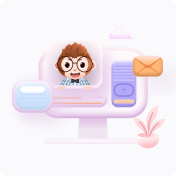
安全验证
文档复制为VIP权益,开通VIP直接复制
