没有合适的资源?快使用搜索试试~ 我知道了~
使用稀疏性以外的先验信息进行多变量追踪图像重建
0 下载量 47 浏览量
2021-03-10
13:37:41
上传
评论
收藏 1.42MB PDF 举报
温馨提示
图像的先验信息在降低CS反演的计算复杂度和提高重建质量方面起着重要作用。 本文提出了一种基于小波的多元追踪算法,该算法利用了图像的先验信息,而这些信息超越了简单稀疏性。 所提出的方法以多变量方式从多次测量中重建图像小波系数,并以提取的图像边缘作为先验信息来指导算法在CS恢复中的追踪过程。 通过边缘信息与多元联合恢复的交互作用,该算法显着提高了边缘明显,稀疏度高的CT,MRI等图像的重建质量。 数值实验表明,与其他最新的CS算法相比,所提出的算法具有更高的重构质量并保持更高的计算效率。
资源推荐
资源详情
资源评论
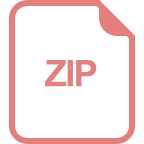
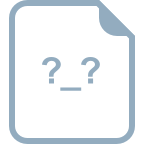
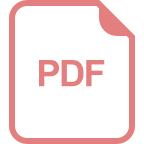
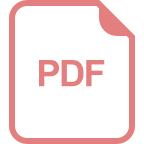
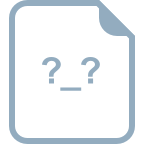
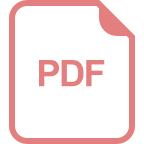
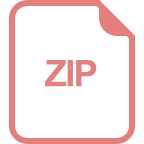
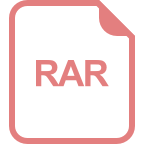
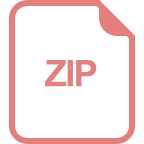
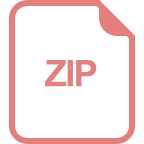
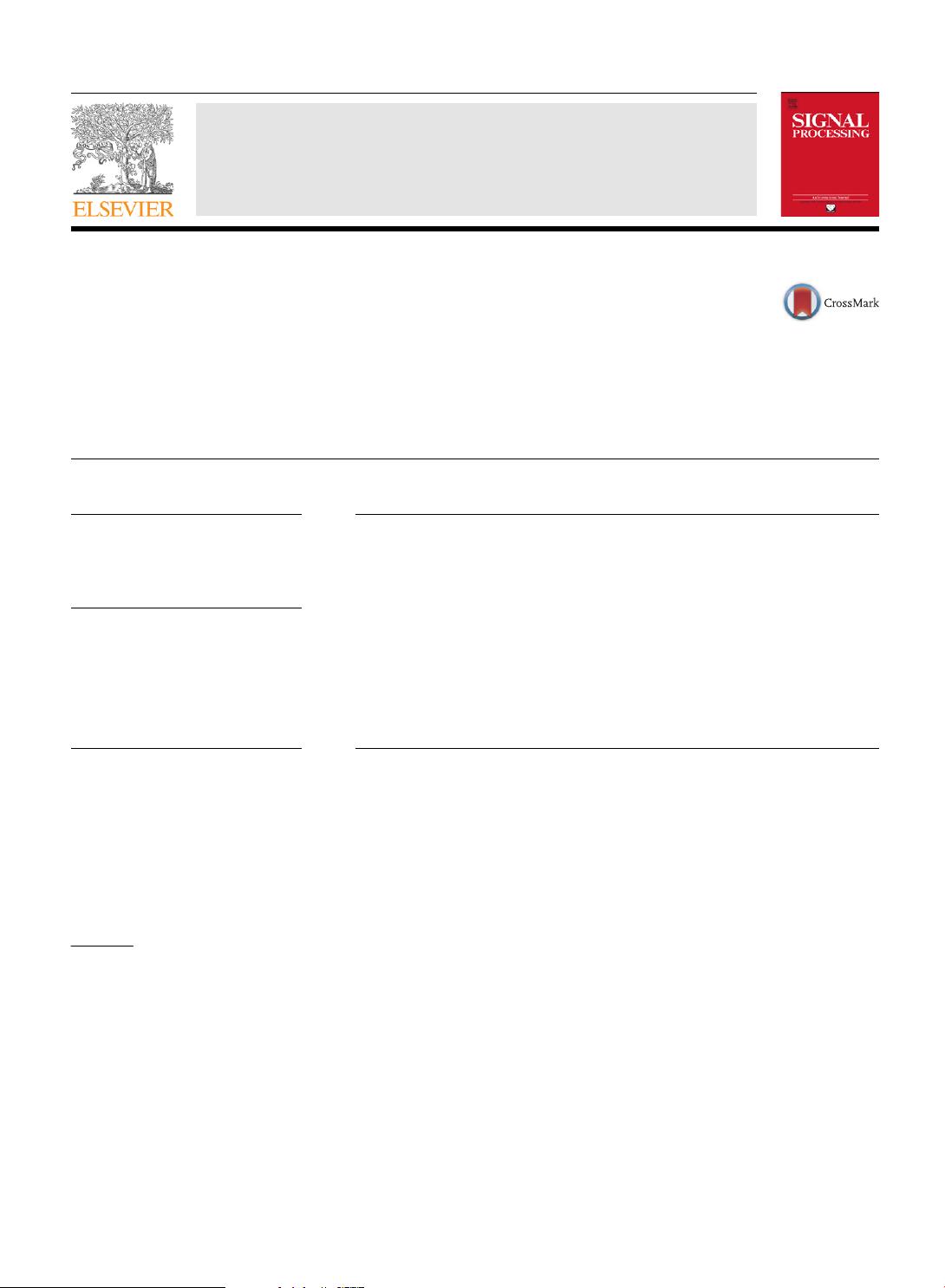
Multivariate pursuit image reconstruction using prior
information beyond sparsity
$
Jiao Wu
a,b,c,
n
, Fang Liu
a,b
, Lc Jiao
b
, Xiaodong Wang
a,b
a
School of Computer Science and Technology, Xidian University, Xi’an 710071, PR China
b
Key Laboratory of Intelligent Perception and Image Understanding of Ministry of School of Computer Science and Technology,
Xidian University, Xi’an 710071, PR China
c
College of Sciences, China Jiliang University, Hangzhou 310018, PR China
article info
Article history:
Received 25 January 2012
Received in revised form
10 September 2012
Accepted 16 September 2012
Available online 26 September 2012
Keywords:
Compressive sensing
Edge detection
Prior model
Wavelet transform
abstract
The prior information of images plays an important role in reducing the computational
complexity of CS inversion and improving the reconstruction quality. A wavelet-based
multivariate pursuit algorithm, which exploits the prior information of images that goes
beyond simple sparsity, is developed in this paper. The proposed method reconstructs the
image wavelet coefficients from the multiple measurements in a multivariate manner, and
uses the extracted image edge as the prior information to guide the pursuit process of
algorithm in CS recovery. By means of the interaction of edge information and multivariate
joint recovery, the proposed algorithm significantly improves the reconstruction quality
of those images with the obvious edges and high sparsity, such as CT, MRI images. Numerical
experiments demonstrate that the proposed algorithm returns superior reconstructed
quality and remains higher computational efficiency than other stat e-of-the-art CS
algorithms.
& 2012 Elsevie r B.V. All rights reserved.
1. Introduction
Compressive sensing (CS) [1,2] demonstrates that sparse
images can be recovered from fewer random measure-
ments by taking advantage of the sparsity inherent in
real world images. In other words, if an image f 2
R
M
can be converted into one having relatively few values
significantly different from zero under a certain orthogonal
transform
W
2
R
MM
,thatisf ¼
W
x, one may reconstruct
the transform coefficient vector x from fewer linear mea-
surements of f by solving the following l
0
-problem:
min
x
JxJ
0
s:t: y ¼
H
f ¼
U
x, ð1Þ
where y 2
R
K
ðK 5MÞ is the linear measurements of f under
the given measurement matrix
H
2
R
KM
,and
U
¼
HW
.
However, l
0
-problem is NP-hard . Thus designing the
effective and low computational complexity CS reconstruc-
tion method is of great significance.
Theorthogonalbasisforsparserepresentationofimages
is one of the key factors of CS, with the wavelet transform [3]
being an important exampl e. Under the wavele t transform,
the main energ y of image is concentrated in the low
frequency part, and the singularity characteristics show the
significant sparsity in the high frequency parts, that is, the
wavelet coefficients that correspond to the edge and ridge
are significan tly differe nt from zero. G eneral ly, there ar e
some particular structures (such as the tree structure and
Contents lists available at SciVerse ScienceDirect
journal homepage: www.elsevier.com/locate/sigpro
Signal Processing
0165-1684/$ - see front matter & 2012 Elsevier B.V. All rights reserved.
http://dx.doi.org/10.1016/j.sigpro.2012.09.010
$
This work was supported in part by the National Natural Science
Foundation of China (Grant nos. 60971112, 60971128, 60970067,
61072106, 61072108, 61173090), in part by the Fund for Foreign Scholars
in University Research and Teaching Programs (the 111 Project) (Grant no.
B07048), in part by the Program for Cheung Kong Scholars and Innovative
Research Team in University (Grant no. IRT1170), and in part by the Funded
By Open Research Fund Program of Key Lab of Intelligent Perception and
Image Understanding of Ministry of Education of China (Grant no.
IPIU012011002).
n
Corresponding author at: School of Computer Science and Technol-
ogy, Xidian University, Xi’an 710071, PR China.
E-mail addresses: wu_jiao@yahoo.cn (J. Wu),
lf204310@163.com (F. Liu), jlc1023@163.com (L. Jiao),
xdwang@mail.xidian.edu.cn (X. Wang).
Signal Processing 93 (2013) 1662–1672
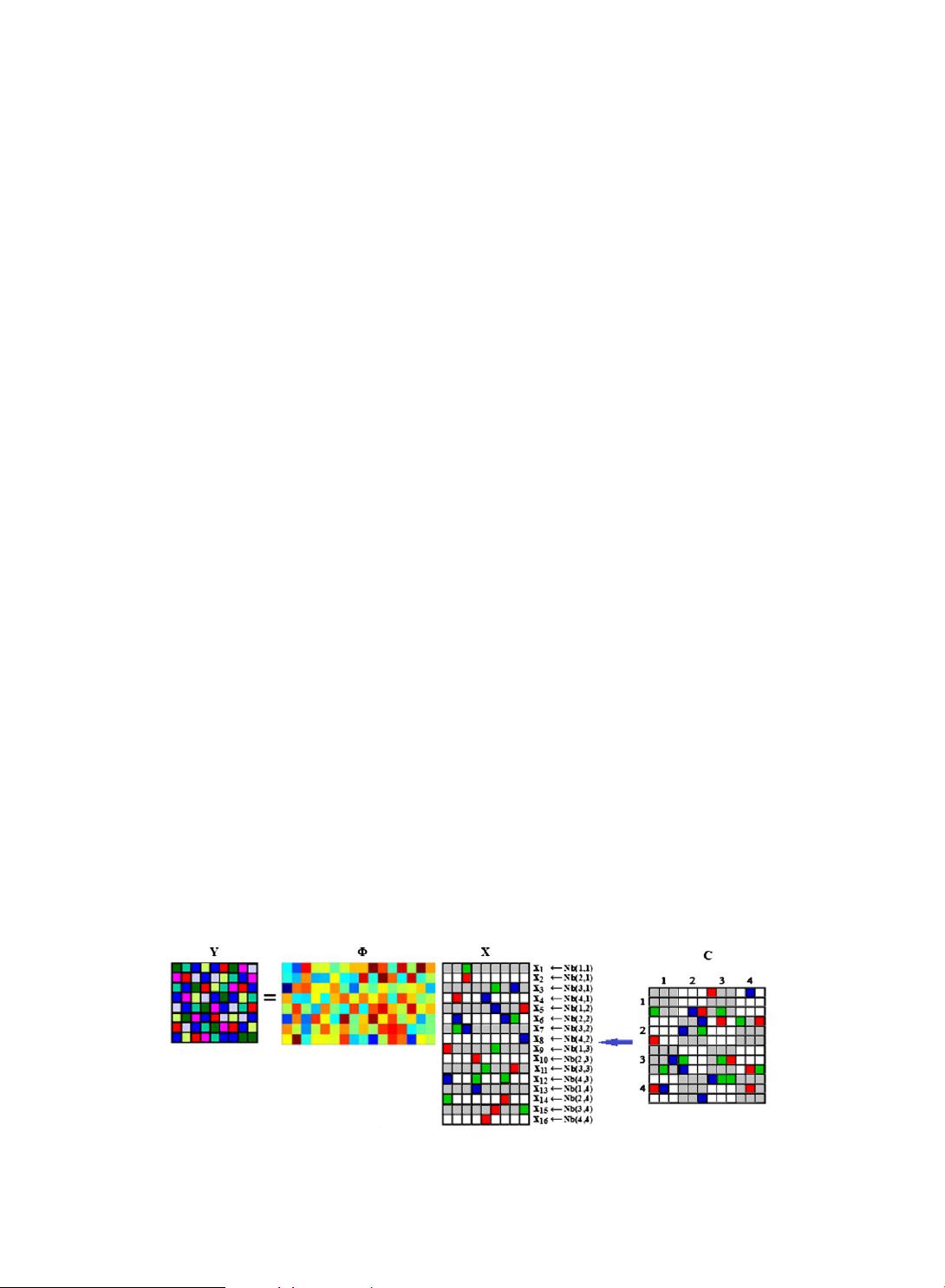
the dependence structure) within the wavelet coefficients of
images. The expl icit use of these structures has appeared in
image compressi on and denoi sing proble ms [4–9].
A multiscale CS scheme [10] is developed based on the
multiscale decomposition by using conventional linear
sampling and reconstruction for low frequency information,
and compressed sampling on high frequency information.
Under this framework, more realistic prior information
(such as tree structures and dependencies of image wavelet
coefficients) can be exploited to wavelet-based CS recovery.
Examples of such techniques include the tree structure-
based methods [11–16] and the dependence structure-
based method [17]. The tree-based approaches enforce
the additional tree-structured dependencies in CS recovery
by exploiting the sparse tree representation or HMT mod-
els. In our previous work [17], the developed multivariate
pursuit algorithm (MPA) jointly recovers the wavelet coef-
ficients of images from the multiple CS measurements by
integrating the statistical dependence models of wavelet
neighborhood coefficients in CS recovery. In addition, the
edge also is one of the important prior information which
exists in the image to be recovered. As mentioned above,
under the wavelet transform, the edges of i mage corre-
spond to those non-zero wavelet coefficients to be recov-
ered. An edge guided MRI recovery algorithm in Fourier
domain [18] produces the state-of-art reconstruction
results by performing an iterative reweighed TV norm
minimization where the weights are dependent on edge
information. The theoretical and numerical results of the
aforementioned methods demonstrate that the superior
performance can be obtained with the fewer measurements
by leveraging the prior models of images.
The purpose of this paper is to design an edge-based
multivariate pursuit algorithm (EMPA) for wavelet-based
CS reconstruction of images. The developed algorithm can
be viewed as an improvement of MPA. The main con-
tributions of this paper are as follows:
Under multiscale CS framework, the multivariate CS
sampling scheme [17] is used to make the multiple CS
measurements, then the reconstruction problem of
images from CS is converted to a sparse multiple
measurement vectors (MMV) problem [19–23], while
the traditional CS corresponds to the single measure-
ment vector (SMV) problem. The theoretical analysis
[20,23] demonstrates that the performance of jointly
sparse recovery for MMV is higher than that of SMV
with the fewer measurements.
MPA solves MMV problem by successively estimating
the wavelet coefficients in neighborhoods and itera-
tively updating the residuals. However, in this manner
the supports of coefficients (i.e. the location of non-
zero coefficients) cannot be identified, that is to say,
the sparse solution of MMV problem cannot be cor-
rectly recovered. To this end, we design an extraction
scheme of image edge information from the complete
low frequency measurements, and use the extracted
edge information as the prior of coefficient supports to
guide the pursuit process of MPA, thus obtain the
sparse solution of MMV problem.
The combination of multivariate joint recovery and edge
information guide contributes a lot to the improvement of
reconstruction performance, especially for those images
with the obvious edges and hi gh sparsity, such as CT, MRI
images.
As the incorporation of image edge information requires
edge detection step, comparing with MPA, the running
time of EMPA increases slightly. However, EMPA has
higher computational efficiency than many other state-
of-the-art CS algorithms.
The remainder of this paper is organized as follows.
Section 2 reviews the multivariate compressive sample
scheme and describe s the prob lem formulat ion. The idea
and main procedu re of MPA is reviewed in Section 3.In
Section 4, we first give the general framework of EMPA and
detail how to extract edge information from CS measure-
ments. Then we interpret how to use the edge information to
guide MPA in CS recovery, thus derive EMPA and several vari-
ant algorithms. Section 5 reports the experimental results.
Conclusions and future work are discussed in Section 6.
2. Multivariate compressive sampling and problem
formulation
2.1. Multivariate compressive sampling
A multivariate compressive sampling scheme [17] has
been designed for taking multiple CS measurements. This
method first rearranges the wavelet coefficients according
Fig. 1. Example of multivariate CS sampling. Given the size of small neighborhood 3 3, a 12 12 image coefficient matrix C (in the right) is partitioned
into 16 small neighborhoods. The ðj
1
, j
2
Þth small neighborhood Nbðj
1
, j
2
Þðj
1
, j
2
¼1, 2, 3, 4Þ is represented as a 9-dimension vector x
i
¼ðx
i1
, ...,
x
i9
Þ
T
ði ¼ j
1
þ4 ðj
2
1ÞÞ. Then C is represented as a 16 9 multivariate coefficient matrix X ¼ðx
1
, ..., x
16
Þ
T
. With an 8 16 measurement matrix
U
,an
8 9 multiple measurement matrix is taken as Y ¼
U
X.
J. Wu et al. / Signal Processing 93 (2013) 1662–1672 1663
剩余10页未读,继续阅读
资源评论
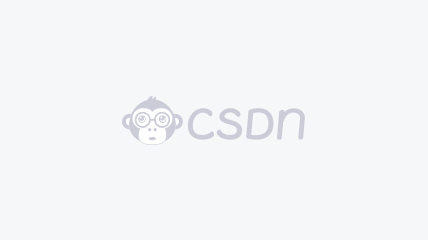

weixin_38654944
- 粉丝: 2
- 资源: 943
上传资源 快速赚钱
我的内容管理 展开
我的资源 快来上传第一个资源
我的收益
登录查看自己的收益我的积分 登录查看自己的积分
我的C币 登录后查看C币余额
我的收藏
我的下载
下载帮助

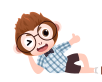
安全验证
文档复制为VIP权益,开通VIP直接复制
