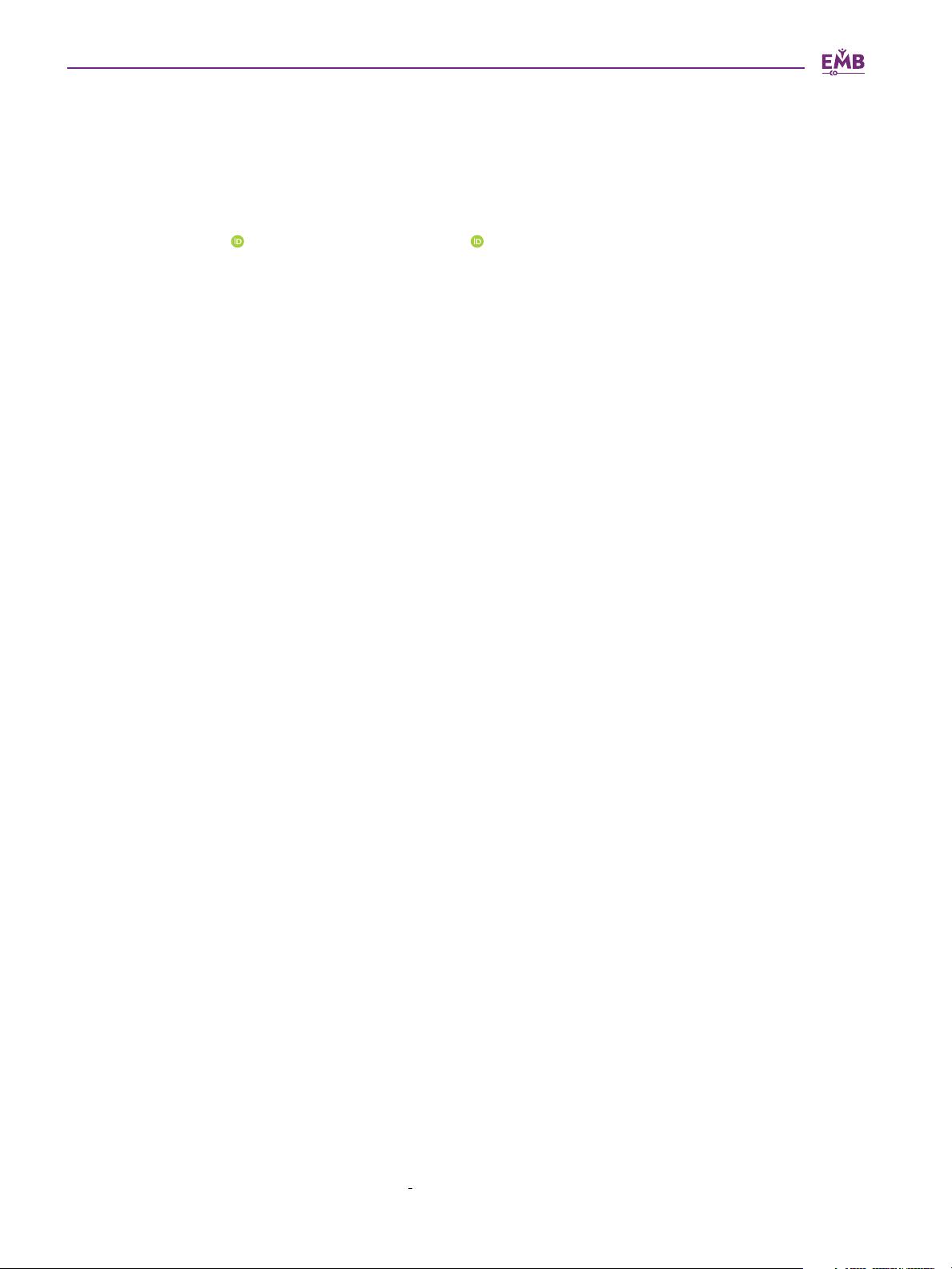
104 IEEE TRANSACTIONS ON BIOMEDICAL ENGINEERING, VOL. 65, NO. 1, JANUARY 2018
Enhancing Detection of SSVEPs for a
High-Speed Brain Speller Using Task-Related
Component Analysis
Masaki Nakanishi , Member, IEEE, Yijun Wang , Member, IEEE, Xiaogang Chen, Member, IEEE,
Yu-Te Wang, Member, IEEE, Xiaorong Gao, Member, IEEE, and Tzyy-Ping Jung, Fellow, IEEE
Abstract—Objective: This study proposes and evaluates
a novel data-driven spatial filtering approach for enhanc-
ing steady-state visual evoked potentials (SSVEPs) detec-
tion toward a high-speed brain-computer interface (BCI)
speller. Methods: Task-related component analysis (TRCA),
which can enhance reproducibility of SSVEPs across multi-
ple trials, was employed to improve the signal-to-noise ratio
(SNR) of SSVEP signals by removing background electroen-
cephalographic (EEG) activities. An ensemble method was
further developed to integrate TRCA filters corresponding
to multiple stimulation frequencies. This study conducted
a comparison of BCI performance between the proposed
TRCA-based method and an extended canonical correla-
tion analysis (CCA)-based method using a 40-class SSVEP
dataset recorded from 12 subjects. An online BCI speller
was further implemented using a cue-guided target selec-
tion task with 20 subjects and a free-spelling task with 10
of the subjects. Results: The offline comparison results in-
dicate that the proposed TRCA-based approach can signif-
icantly improve the classification accuracy compared with
the extended CCA-based method. Furthermore, the online
BCI speller achieved averaged information transfer rates
(ITRs) of 325.33 ± 38.17 bits/min with the cue-guided task
and 198.67 ± 50.48 bits/min with the free-spelling task.
Conclusion: This study validated the efficiency of the pro-
posed TRCA-based method in implementing a high-speed
SSVEP-based BCI. Significance: The high-speed SSVEP-
based BCIs using the TRCA method have great potential
for various applications in communication and control.
Manuscript received November 21, 2016; revised February 25, 2017;
accepted April 6, 2017. Date of publication April 19, 2016; date of cur-
rent version December 20, 2017. This work was supported in part by
Army Research Laboratory (W911NF-10-2-0022) and National Institute
of Healths under Grant 1R21EY025056-01. The work of Y. Wang was
also supported by the Recruitment Program for Young Professionals and
National Natural Science Foundation of China under Grant 61671424.
The work of X. Chen was supported by Young Talents Lift Project of
Chinese Association of Science and Technology, and National Natural
Science Foundation of China under Grant 61603416. (Corresponding
author: Yijun Wang.)
M. Nakanishi, Y.-T. Wang, and T.-P. Jung are with the Swartz Cen-
ter for Computational Neuroscience, Institute for Neural Computation,
University of California.
Y. Wang is with the State Key Laboratory on Integrated Optoelectron-
ics, Institute of Semiconductors, Chinese Academy of Sciences, Beijing
100083, China (e-mail: wangyj@semi.ac.cn).
X. Chen is with the Institute of Biomedical Engineering, Chinese
Academy of Medical Sciences and Peking Union Medical College.
X. Gao is with the Department of Biomedical Engineering, Tsinghua
University.
Digital Object Identifier 10.1109/TBME.2017.2694818
Index Terms—Brain-computer interfaces (BCI), electroen-
cephalography (EEG), steady-state visual evoked potentials
(SSVEP), task-related component analysis (TRCA).
I. INTRODUCTION
B
RAIN-COMPUTER interfaces (BCIs) based on steady-
state visual evoked potentials (SSVEPs) have been attract-
ing much attention due to high communication rates [1]–[3]. In
a recent study, a 40-target SSVEP-based BCI achieved an infor-
mation transfer rate (ITR) of 267 bits/min, to our knowledge, the
highest ITR reported in BCI research to date [2]. To improve the
performance of SSVEP-based BCIs, researchers are working on
two main directions: (1) increasing the number of classes (i.e.,
visual stimuli), ( 2) improving target identification algorithms
[4]. Although the number of frequencies that can be presented
on a computer monitor is limited by its refresh rate, various
stimulus presentation approaches inspired by multiple access
(MA) technologies used in telecommunication have succeeded
in rendering a large number of visual flickers on a computer
monitor [2], [5]–[8]. For example, the frequency shift keying
(FSK) method has been applied to increase the number of vi-
sual stimuli with different properties using the limited number
of available frequencies [5]. A hybrid paradigm which combines
SSVEP with other electroencephalogram (EEG) features (e.g.,
P300) is another way to increase the number of selections [6].
In several other studies, frequency-approximation approaches
make it possible to render a large number of flickers with flexible
frequencies on a computer monitor [7]–[9]. More recently, the
efficacy of a joint frequency-phase modulation (JFPM) method
has been demonstrated in our previous studies [2], [4], [10].
Target identification methods also play an important role in
enhancing the performance of SSVEP-based BCIs [4]. The
methods based on power spectrum density analysis (PSDA)
such as discrete Fourier transform (DFT) were widely used to
detect target frequency of SSVEPs from single-channel EEGs
[11], [12]. In recent studies, temporal features of SSVEPs have
been employed to develop more efficient methods than PSDA
[13]. For example, canonical correlation analysis (CCA) be-
tween SSVEPs and sine-cosine reference signals has been used
to improve the classification accuracy due to its ability to en-
hance the signal-to-noise ratio (SNR) of SSVEPs [13], [14].
Although the robustness of CCA-based method in detecting
0018-9294 © 2017 IEEE. Personal use is permitted, but republication/redistribution requires IEEE permission.
See http://www.ieee.org/publications
standards/publications/rights/index.html for more information.