没有合适的资源?快使用搜索试试~ 我知道了~
Task3 特征工程 一、数据预处理 在这一块,比较常用的包是sklearn.Processing data,主要包括以下操作: 异常值处理 使用箱型图(或小提琴图)发现离群点(off-group points)之后,为了不干扰实验结果,我们通常将离群点处理掉: #from DW阿泽 import the code def outliers_proc(data, col_name, scale=3): 用于清洗异常值,默认用 box_plot(scale=3)进行清洗 :param data: 接收 pandas 数据格式 :param col_name: pan
资源推荐
资源详情
资源评论
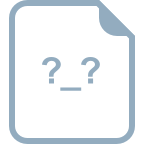
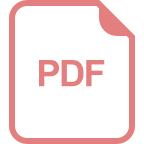
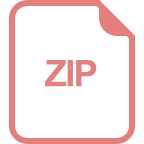
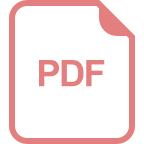
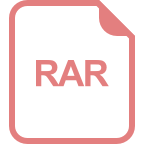
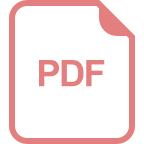
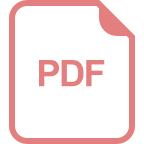
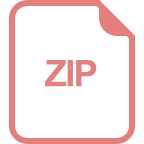
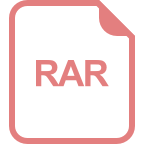
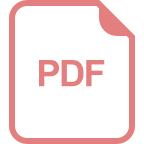
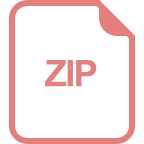
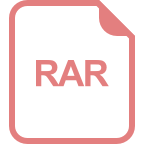
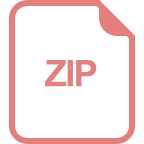
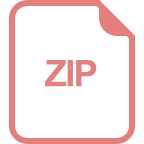
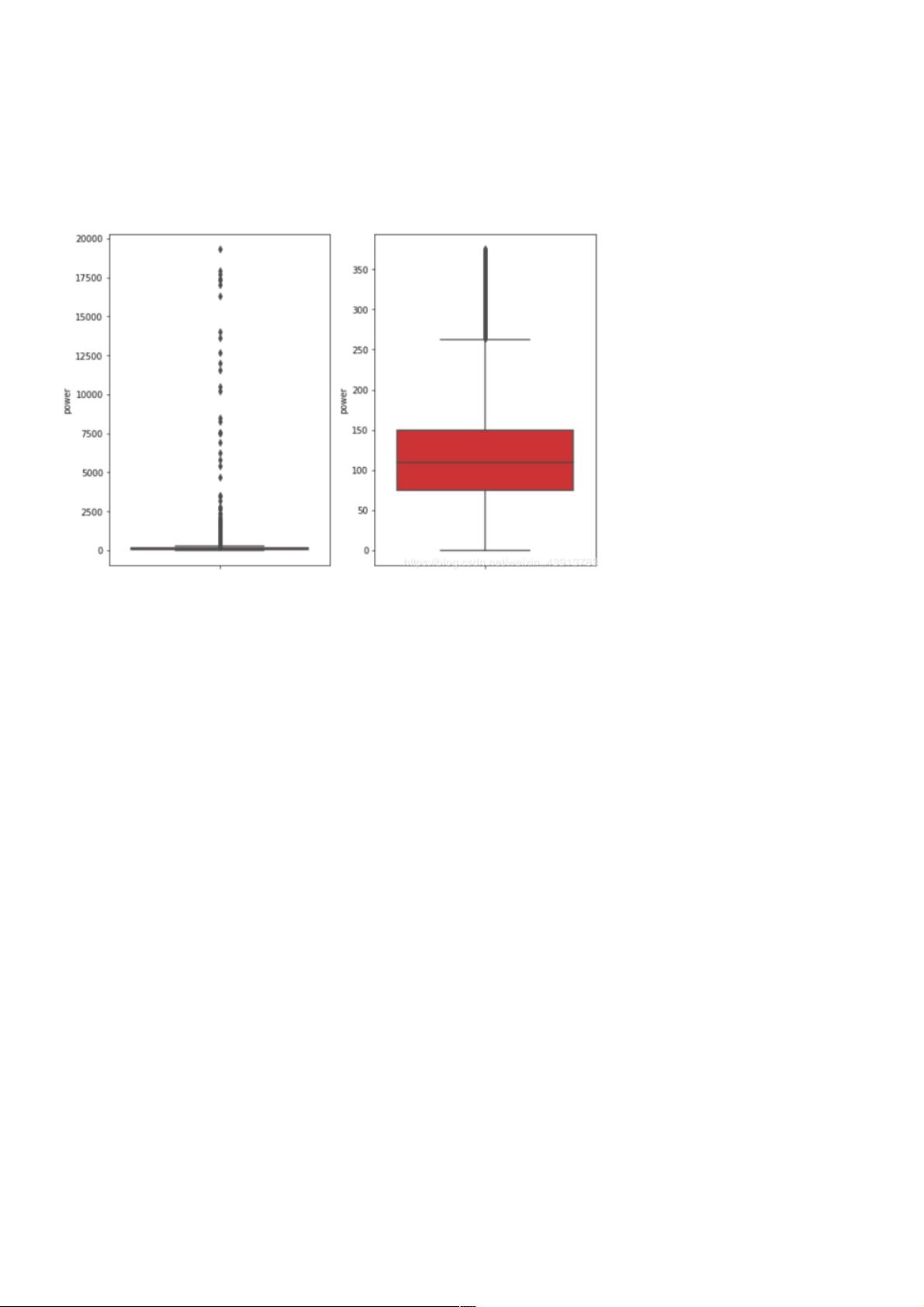
Task3 特征工程特征工程
Task3 特征工程特征工程
一、数据预处理一、数据预处理
在这一块,比较常用的包是sklearn.Processing data,主要包括以下操作:
异常值处理异常值处理
使用箱型图(或小提琴图)发现离群点(off-group points)之后,为了不干扰实验结果,我们通常将离群点处理掉:
#from DW阿泽 import the code
def outliers_proc(data, col_name, scale=3):
"""
用于清洗异常值,默认用 box_plot(scale=3)进行清洗
:param data: 接收 pandas 数据格式
:param col_name: pandas 列名
:param scale: 尺度
:return:
"""
def box_plot_outliers(data_ser, box_scale):
"""
利用箱线图去除异常值
:param data_ser: 接收 pandas.Series 数据格式
:param box_scale: 箱线图尺度,
:return:
"""
iqr = box_scale * (data_ser.quantile(0.75) - data_ser.quantile(0.25))
val_low = data_ser.quantile(0.25) - iqr
val_up = data_ser.quantile(0.75) + iqr
rule_low = (data_ser val_up)
return (rule_low, rule_up), (val_low, val_up)
data_n = data.copy()
data_series = data_n[col_name] rule, value = box_plot_outliers(data_series, box_scale=scale)
index = np.arange(data_series.shape[0])[rule[0] | rule[1]] print("Delete number is: {}".format(len(index)))
data_n = data_n.drop(index)
data_n.reset_index(drop=True, inplace=True)
print("Now column number is: {}".format(data_n.shape[0]))
index_low = np.arange(data_series.shape[0])[rule[0]] outliers = data_series.iloc[index_low] print("Description of data less than the lower bound is:")
print(pd.Series(outliers).describe())
index_up = np.arange(data_series.shape[0])[rule[1]] outliers = data_series.iloc[index_up] print("Description of data larger than the upper bound is:")
print(pd.Series(outliers).describe())
fig, ax = plt.subplots(1, 2, figsize=(10, 7))
sns.boxplot(y=data[col_name], data=data, palette="Set1", ax=ax[0])
sns.boxplot(y=data_n[col_name], data=data_n, palette="Set1", ax=ax[1])
return data_n
缺失值处理缺失值处理
像IRIS数据集没有缺失值,故对数据集新增一个特征,4个特征均赋值为NaN,表示数据缺失;
用均值、众数、中位数填充;
用正态分布进行填充;
sklearn.processing import Imputer 这是sklearn中的处理特征缺失的类;
资源评论
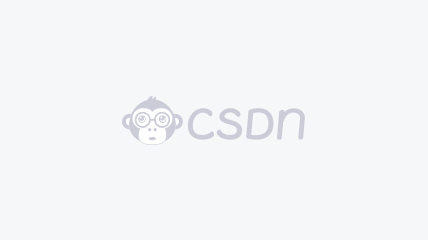

weixin_38626179
- 粉丝: 4
- 资源: 959
上传资源 快速赚钱
我的内容管理 展开
我的资源 快来上传第一个资源
我的收益
登录查看自己的收益我的积分 登录查看自己的积分
我的C币 登录后查看C币余额
我的收藏
我的下载
下载帮助

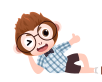
安全验证
文档复制为VIP权益,开通VIP直接复制
