本文主要讲解了如何使用Python语言结合卷积操作来提取图片的轮廓。这个过程涵盖了图像处理中的边缘检测技术,特别是使用了Sobel算子作为卷积核来实现。在实现这个功能的过程中,涉及到了Python的数值运算、图像加载显示以及使用TensorFlow框架来进行卷积操作的相关技巧。 Sobel算子是一种用来进行边缘检测的离散微分算子,它将一幅图像分解成两个不同方向的梯度信息——水平方向和垂直方向。Sobel算子通过卷积操作来计算图像强度的梯度近似值,从而检测图像中的边缘。 在Python中,可以使用多个库来实现图像的加载和显示,比如matplotlib.pyplot和matplotlib.image。加载图像后,通常需要将其转换为numpy数组形式以便进行进一步的数值运算。加载的图片维度信息(例如960x720x3)表明了图像的宽度、高度和颜色通道数。 在卷积操作之前,需要构建卷积核,也称为滤波器或掩膜。在TensorFlow中,可以使用tf.constant来初始化一个常量张量,作为卷积操作的卷积核。Sobel算子通常是一个3x3的矩阵,在彩色图像处理中,需要构建一个3x3x3的卷积核,这里的3表示有三个颜色通道(RGB)。 接下来是执行卷积操作,使用TensorFlow的tf.nn.conv2d函数来实现。这个函数需要指定输入数据、卷积核、步长(strides)和填充方式(padding)。在本例中,步长被设置为[1,1,1,1],表示在每个维度上移动一个像素,而填充方式为'SAME',意味着在边缘用零填充使得输出与输入具有相同的尺寸。 卷积操作之后的输出通常会包含负值,且值的范围可能会超出显示范围[0,255]。因此,需要进行归一化处理,将数据缩放到[0,1]区间,再乘以255转换为[0,255]的整数区间,以便用uint8类型进行存储,这样才能够用图像的形式正确显示。 使用matplotlib的imshow函数来显示处理后的图像,其中cmap='Greys_r'参数将图像以灰度形式展示出来,这样可以更清楚地看到边缘轮廓。 在实际操作中,可能会遇到依赖库未安装或者版本不兼容的问题。例如,TensorFlow框架尚未对Python 3.6提供支持,建议使用Python 3.5版本来避免兼容性问题。遇到pip安装错误时,可以使用python -m pip install --upgrade pip命令来更新pip到最新版本。 以上就是使用Python结合卷积操作提取图片轮廓的知识点总结,其中包括了图像处理的基本概念、Sobel算子、数值运算、TensorFlow框架的基本使用、图像的加载和显示以及数据归一化等技术要点。掌握这些内容对于进行图像处理和计算机视觉相关工作非常重要。
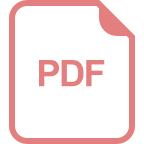
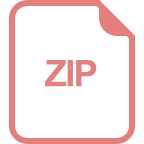
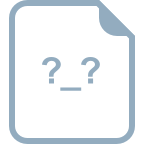
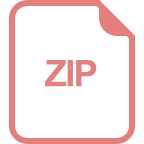
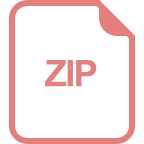
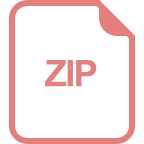
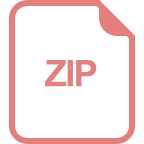
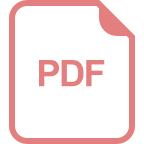
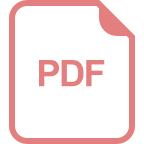
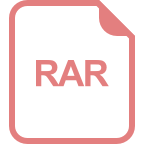
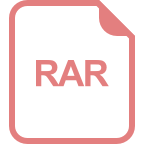
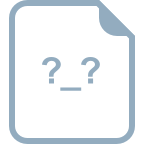
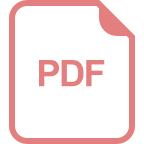
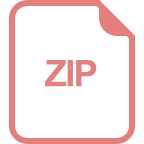
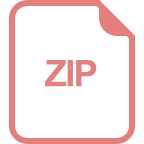
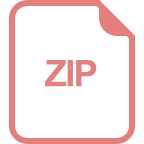
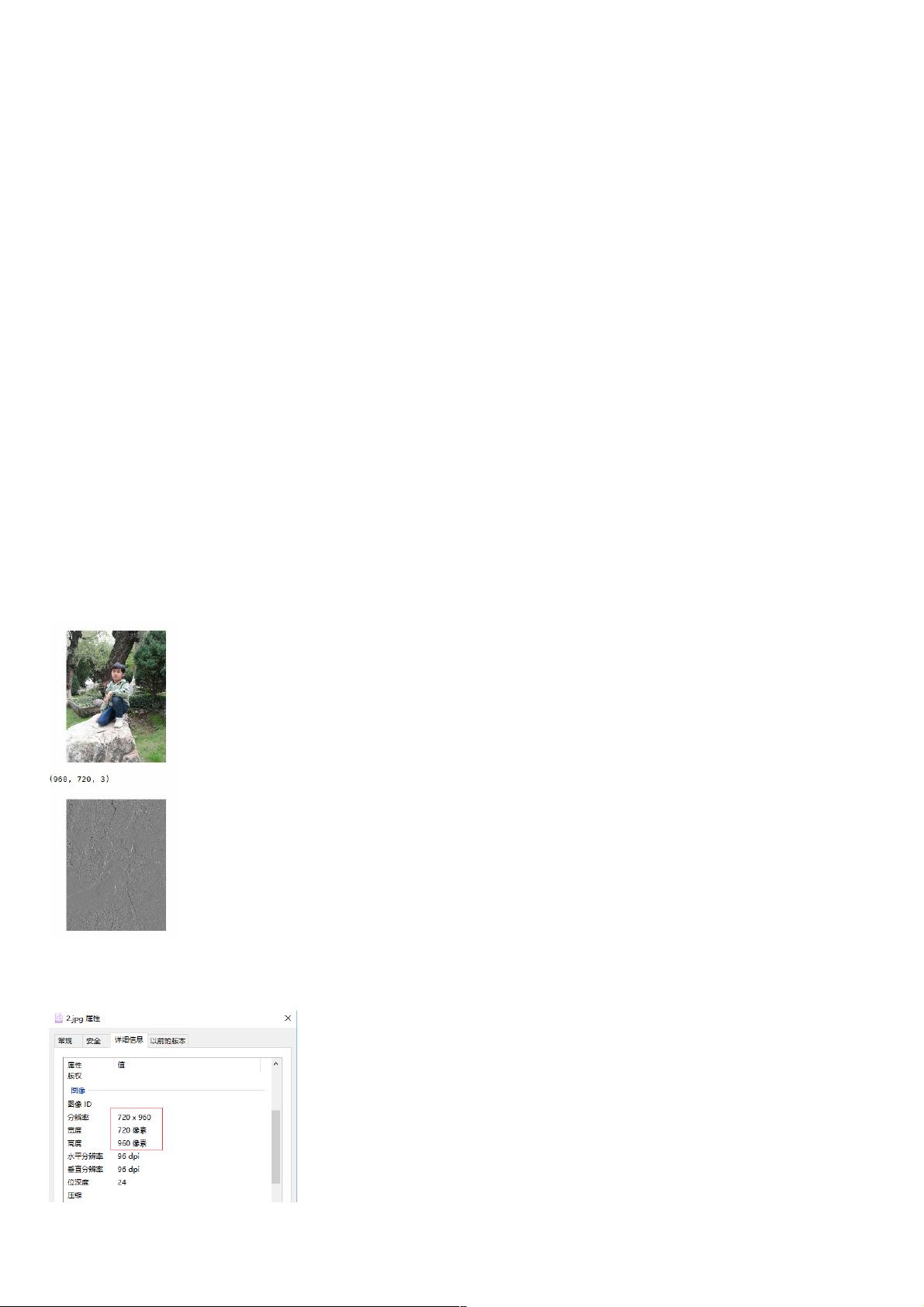
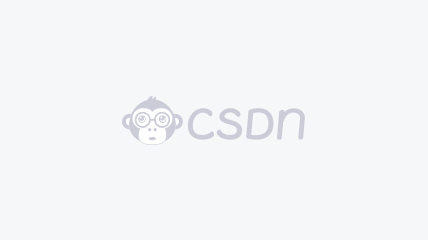

- 粉丝: 8
- 资源: 894
我的内容管理 展开
我的资源 快来上传第一个资源
我的收益
登录查看自己的收益我的积分 登录查看自己的积分
我的C币 登录后查看C币余额
我的收藏
我的下载
下载帮助

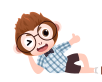
