没有合适的资源?快使用搜索试试~ 我知道了~
温馨提示
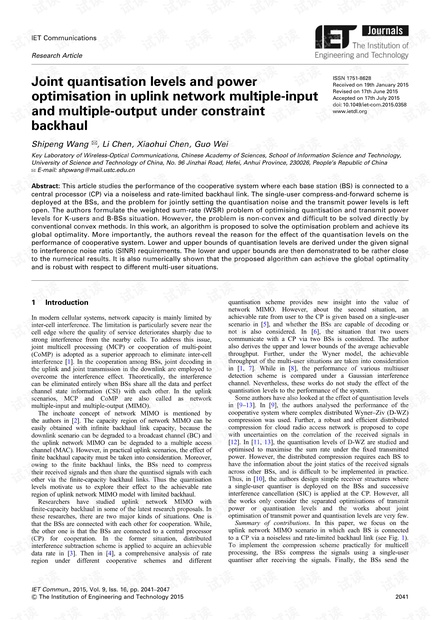

试读
7页
This article studies the performance of the cooperative system where each base station (BS) is connected to a<br>central processor (CP) via a noiseless and rate-limited backhaul link. The single-user compress-and-forward scheme is<br>deployed at the BSs, and the problem for jointly setting the quantisation noise and the transmit power levels is left<br>open. The authors formulate the weighted sum-rate (WSR) problem of optimising quantisation and transmit power<br>levels for K-users and B
资源推荐
资源评论
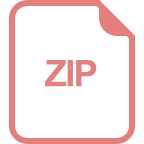
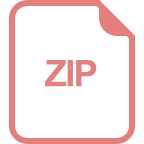
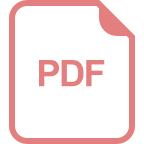
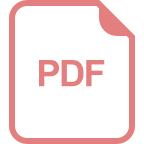
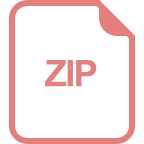
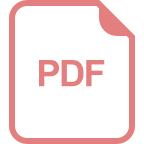
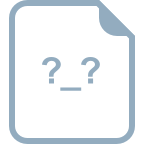
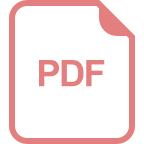
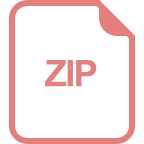
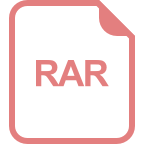
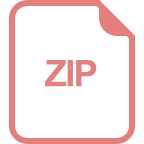
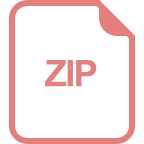
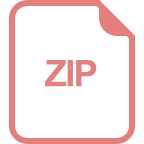
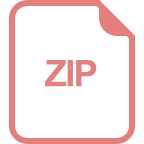
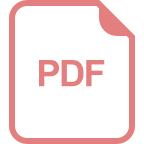
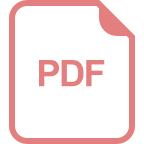
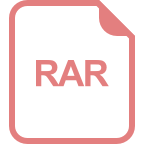
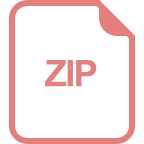
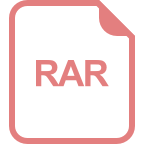
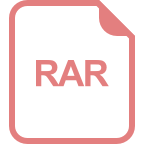
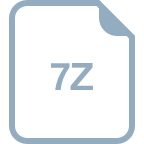
资源评论
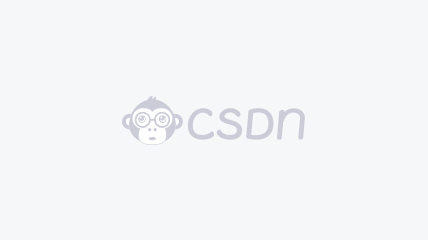

weixin_38571759
- 粉丝: 6
- 资源: 897
上传资源 快速赚钱
我的内容管理 展开
我的资源 快来上传第一个资源
我的收益
登录查看自己的收益我的积分 登录查看自己的积分
我的C币 登录后查看C币余额
我的收藏
我的下载
下载帮助

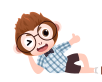
安全验证
文档复制为VIP权益,开通VIP直接复制
