没有合适的资源?快使用搜索试试~ 我知道了~
Data Reconstruction with Spatial and Temporal Correlation in Wir...
0 下载量 181 浏览量
2021-02-22
01:28:07
上传
评论
收藏 365KB PDF 举报
温馨提示
Data loss is a common phenomenon in the practical wireless sensor networks due to several observations, such as the node or link failure, the expensive cost of re-transmission, and so on. How to reconstruct the lost data is one important issue for many applications which concern heavily on the data gathering issues. Most of the current data reconstruction solutions are based on the spatial correlation and they adopt interpolation methods to approximate the data reconstruction process, which lead
资源推荐
资源详情
资源评论
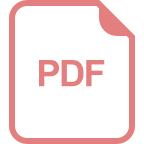
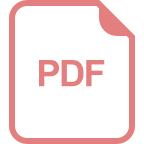
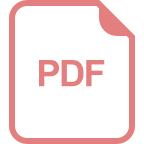
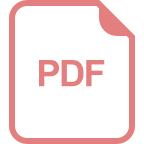
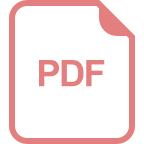
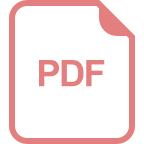
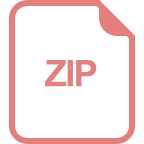
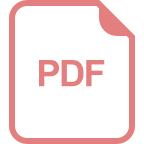
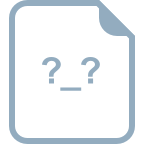
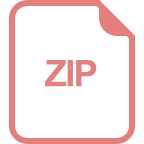
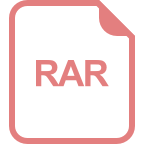
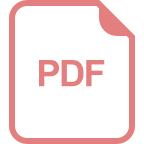
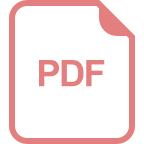
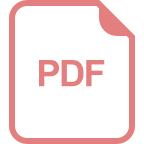
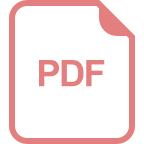
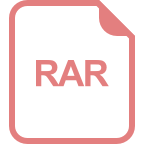
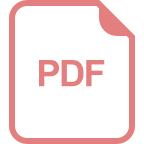
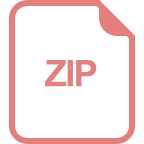
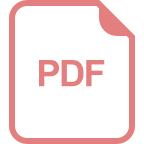
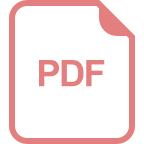
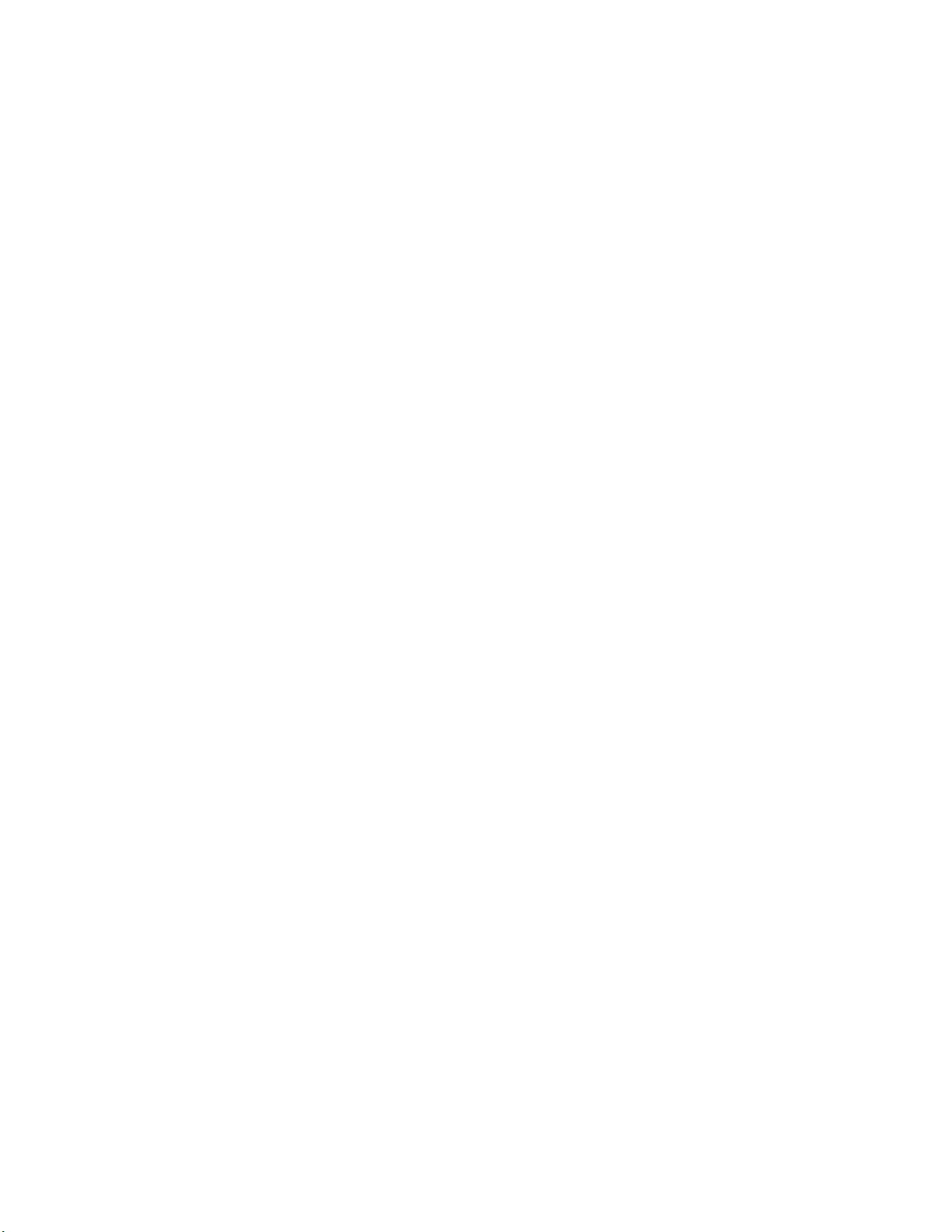
Data Reconstruction with Spatial and Temporal
Correlation in Wireless Sensor Networks
Yayun Zhang
College of Mathematics and
Computer Sciences
Fuzhou University,China
n140327044@fzu.edu.cn
Hongju Cheng
*
College of Mathematics and
Computer Sciences
Fuzhou University,China
cscheng@fzu.edu.cn
Dongyin Chen
College of Mathematics and
Computer Sciences
Fuzhou University,China
n140327035@fzu.edu.cn
ABSTRACT
Data loss is a common phenomenon in the practical wireless
sensor networks due to several observations, such as the node or
link failure, the expensive cost of re-transmission, and so on. How
to reconstruct the lost data is one important issue for many
applications which concern heavily on the data gathering issues.
Most of the current data reconstruction solutions are based on the
spatial correlation and they adopt interpolation methods to
approximate the data reconstruction process, which leads to bad
performance especially in case that the number of data loss
increases in the network. In this paper, we consider the spatial and
temporal correlation simultaneously, and propose a novel data
reconstruction algorithm to improve the data error ratio in the
wireless sensor networks. The proposed algorithm can utilize the
spatial correlation by using the curved face reconstruction, and it
is also helpful to improve the accuracy of data reconstruction by
exploring the temporal correlation among the sensory data.
Extensive experiments demonstrate that the proposed data
reconstruction algorithm can significantly reduce the fitting data
error ratio compared with related works especially in case that
data loss is serious in the network.
CCS Concepts
• General and reference➝Estimation • Information systems➝
Sensor networks.
Keywords
Wireless sensor networks; spatial correlation; temporal correlation;
data reconstruction.
1. INTRODUCTION
A wireless sensor network generally consists of a large number of
nodes which are equipped with the sensing devices, micro-
processor, limited memory and wireless communications [1].
They can collect information from the local environment and send
the sensory data to the application center, i.e., the sink. However,
data loss might occur when data is gathered to the sink due to the
following observations. Firstly, the trend for cheaper sensor nodes
might result in increased probability of node failure. In this case,
the sensor node collect cannot obtain all the original data
especially when it works in a periodical manner. Secondly, the
congestion in the wireless communications, such as the exposed
terminal problem and hidden terminal problem, shows that the
data transmission is unreliable, which means that the sink will not
receive all the sensory data sent by nodes in the network. Thirdly,
data re-transmission is a little expensive in the wireless sensor
networks because the nodes are generally budged with un-
rechargeable battery. For example, the data loss ratio is about 23%
in the practical collected data set for Intel Indoor project [2]. How
to reconstruct the lost data is one important issue in the wireless
sensor networks.
Similar to the definition in [3], we synthesize several types of data
loss patterns by analyzing the data set in Intel Indoor project [2],
i.e., element random loss, block random loss and element
sequence loss. The element random loss describes the case that
data is lost randomly during any given collection period. In this
case, the probability of data loss for different nodes is independent.
Figure 1 demonstrates an example, in which the hollow circles
denote nodes whose data is lost (meaning that the sink does not
receive the data due to node/link failure), and the black solid
circles denote nodes whose data is correctly sent to the sink. The
block random loss is used to illustrate the case that a number of
nodes in the same block have lost all their data simultaneously at a
random time. As shown in Figure 2, nodes numbered from 1 to 6
are in the same block, and it is called block random loss if they
lose the data simultaneously (represented by hollow circles to
show the data loss). The element sequence loss shows that the
collected data for one single node is lost during the continuous
time duration. The sequential data loss might mean node failure in
the network when it is detected.
Most of the applications in the wireless sensor networks depend
on data analysis by gathering information from nodes in the
networks to the sink [4, 5]. The presented data loss may influence
the efficiency or feasibility of data analysis in these applications.
Some works aim at providing the approximate query in which
data accuracy is not the key issue and thus the application/sink
only needs to know the approximate data in the network. However,
data loss is not considered and solved in these works, which
means approximate query will encounter with the problems if too
much data is lost.
Most of the current data reconstruction algorithms, such as the
traditional KNN [6], the inverse-distance weighting [7] and the
Delaunay Triangulation [8], depend on the spatial data correlation
in the sensor networks. However, the physical phenomenon
observed in the network is both spatial and temporal correlated
generally. The different data observed by adjacent nodes is similar
and it has the same variation tendency. At the same time, the
value of sequential sensing data for one node is generally
continuous when the collection duration is small enough. Some
Permission to make digital or hard copies of all or part of this work for
personal or classroom use is granted without fee provided that copies are
not made or distributed for profit or commercial advantage and that
copies bear this notice and the full citation on the first page. To copy
otherwise, or republish, to post on servers or to redistribute to lists,
requires prior specific permission and/or a fee. Request permissions
from Permissions@acm.org.
MSCC'16, July 05 2016, Paderborn, Germany
© 2016 ACM. ISBN 978-1-4503-4343-5/16/07…$15.00
DOI: http://dx.doi.org/10.1145/2940353.2940360
40
资源评论
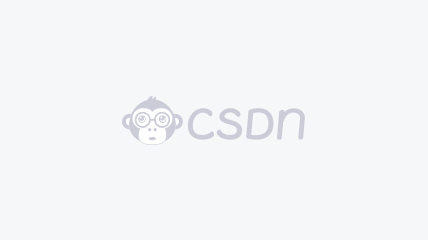

weixin_38515573
- 粉丝: 8
- 资源: 939
上传资源 快速赚钱
我的内容管理 展开
我的资源 快来上传第一个资源
我的收益
登录查看自己的收益我的积分 登录查看自己的积分
我的C币 登录后查看C币余额
我的收藏
我的下载
下载帮助

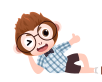
最新资源
- 软件测试的分类.xmind
- MATLAB GUI三阶魔方三维还原仿真程序,可以呈现出魔方的动态还原过程,方便验证魔方还原步骤的正确性 还送C++解魔方程序 有这个GUI就太省事了,GUI生成乱序魔方状态字符串,C++程序生成
- Java毕设项目:基于spring+mybatis+maven+mysql实现的校园资讯智能推荐系统【含源码+数据库+开题报告+毕业论文】
- 三段式电流保护方案设计及仿真分析,MATLAB Simulink 原始参数、要求见图1 利用Simulink搭建仿真模型见图2,验证过电流保护(③段保护),仿真结果见图3 说明书完整,包括:三段式
- 一个基于python和sql server2014做的商品学生信息管理系统,有界面,利用的sql server数据库
- COMSOL声学-超声波无损检测 模型介绍:本模型主要利用静电、固体力学以及压电效应多物理场三个模块 本模型包括压电单元(PZT-5H)和被检测材料(不锈钢)两个部分
- Java毕设项目:基于spring+mybatis+maven+mysql实现的固定资产管理系统【含源码+数据库+开题报告+毕业论文】
- 一个基于基于python的人脸识别签到系统.zip
- BLDC(直流无刷电机)反电动势测量 观测模型-simulink A1 暂无文档
- ESP32-S3深度休眠模式与自动浅度休眠模式的正交测试代码
- 一个基于python的图书推荐系统
- 最新2024.10更新!研究生导师综合评价数据集
- Java毕设项目:基于spring+mybatis+maven+mysql实现的实验室考勤管理系统【含源码+数据库+毕业论文】
- STM32 AT32 GD32 串口 IAP bootloader 远程升级 源码 RS485升级 有说明文档
- 数据编织价值评估指南2024
- 全混合动力电动汽车模型 该simulink模型代表混合动力电动汽车的整车模型,可用于研究不同的控制策略以提高燃油经济性 需要Matlab 2020b来打开该模型
资源上传下载、课程学习等过程中有任何疑问或建议,欢迎提出宝贵意见哦~我们会及时处理!
点击此处反馈


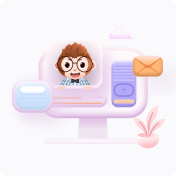
安全验证
文档复制为VIP权益,开通VIP直接复制
