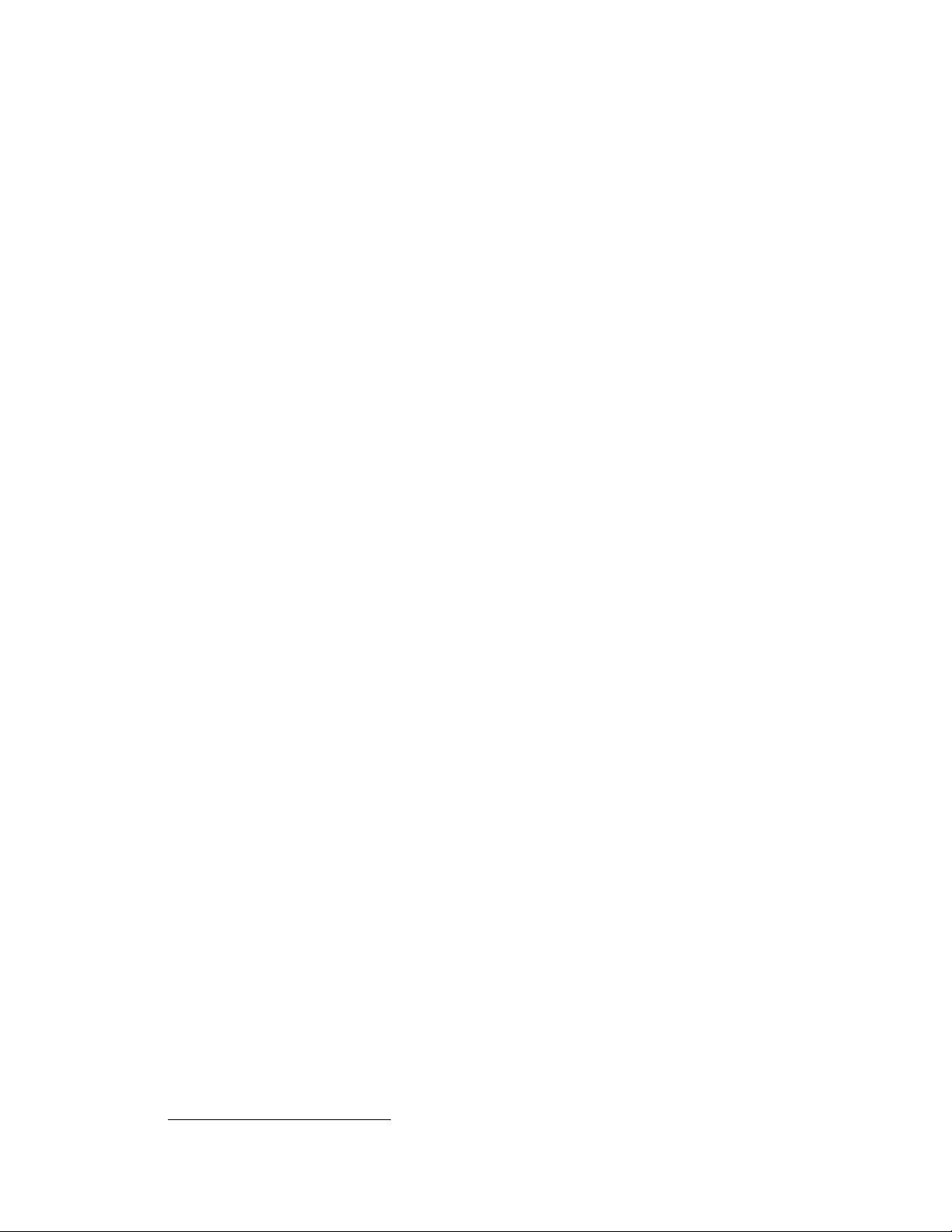
GAUSSIAN ERROR LINEAR UNITS (GELUS)
Dan Hendrycks
∗
University of California, Berkeley
hendrycks@berkeley.edu
Kevin Gimpel
Toyota Technological Institute at Chicago
kgimpel@ttic.edu
ABSTRACT
We propose the Gaussian Error Linear Unit (GELU), a high-performing neural
network activation function. The GELU activation function is xΦ(x), where Φ(x)
the standard Gaussian cumulative distribution function. The GELU nonlinearity
weights inputs by their value, rather than gates inputs by their sign as in ReLUs
(x1
x>0
). We perform an empirical evaluation of the GELU nonlinearity against
the ReLU and ELU activations and find performance improvements across all
considered computer vision, natural language processing, and speech tasks.
1 INTRODUCTION
Early artificial neurons utilized binary threshold units (Hopfield, 1982; McCulloch & Pitts, 1943).
These hard binary decisions are smoothed with sigmoid activations, enabling a neuron to have a “fir-
ing rate” interpretation and to train with backpropagation. But as networks became deeper, training
with sigmoid activations proved less effective than the non-smooth, less-probabilistic ReLU (Nair &
Hinton, 2010) which makes hard gating decisions based upon an input’s sign. Despite having less of
a statistical motivation, the ReLU remains a competitive engineering solution which often enables
faster and better convergence than sigmoids. Building on the successes of ReLUs, a recent modifi-
cation called ELUs (Clevert et al., 2016) allows a ReLU-like nonlinearity to output negative values
which sometimes increases training speed. In all, the activation choice has remained a necessary
architecture decision for neural networks lest the network be a deep linear classifier.
Deep nonlinear classifiers can fit their data so well that network designers are often faced with the
choice of including stochastic regularizer like adding noise to hidden layers or applying dropout (Sri-
vastava et al., 2014), and this choice remains separate from the activation function. Some stochastic
regularizers can make the network behave like an ensemble of networks, a pseudoensemble (Bach-
man et al., 2014), and can lead to marked accuracy increases. For example, the stochastic regular-
izer dropout creates a pseudoensemble by randomly altering some activation decisions through zero
multiplication. Nonlinearities and dropout thus determine a neuron’s output together, yet the two
innovations have remained distinct. More, neither subsumed the other because popular stochastic
regularizers act irrespectively of the input and nonlinearities are aided by such regularizers.
In this work, we introduce a new nonlinearity, the Gaussian Error Linear Unit (GELU). It relates
to stochastic regularizers in that it is the expectation of a modification to Adaptive Dropout (Ba &
Frey, 2013). This suggests a more probabilistic view of a neuron’s output. We find that this novel
nonlinearity matches or exceeds models with ReLUs or ELUs across tasks from computer vision,
natural language processing, and automatic speech recognition.
2 GELU FORMULATION
We motivate our activation function by combining properties from dropout, zoneout, and ReLUs.
First note that a ReLU and dropout both yield a neuron’s output with the ReLU deterministi-
cally multiplying the input by zero or one and dropout stochastically multiplying by zero. Also,
a new RNN regularizer called zoneout stochastically multiplies inputs by one (Krueger et al.,
2016). We merge this functionality by multiplying the input by zero or one, but the values of
this zero-one mask are stochastically determined while also dependent upon the input. Specif-
ically, we can multiply the neuron input x by m ∼ Bernoulli(Φ(x)), where Φ(x) = P (X ≤
∗
Work done while the author was at TTIC. Code available at github.com/hendrycks/GELUs
1
arXiv:1606.08415v4 [cs.LG] 8 Jul 2020
评论0