import numpy as np
from utils import feature_split, calculate_gini
### 定义树结点
class TreeNode():
def __init__(self, feature_i=None, threshold=None,
leaf_value=None, left_branch=None, right_branch=None):
# 特征索引
self.feature_i = feature_i
# 特征划分阈值
self.threshold = threshold
# 叶子节点取值
self.leaf_value = leaf_value
# 左子树
self.left_branch = left_branch
# 右子树
self.right_branch = right_branch
### 定义二叉决策树
class BinaryDecisionTree(object):
### 决策树初始参数
def __init__(self, min_samples_split=2, min_gini_impurity=999,
max_depth=float("inf"), loss=None):
# 根结点
self.root = None
# 节点最小分裂样本数
self.min_samples_split = min_samples_split
# 节点初始化基尼不纯度
self.min_gini_impurity = min_gini_impurity
# 树最大深度
self.max_depth = max_depth
# 基尼不纯度计算函数
self.gini_impurity_calculation = None
# 叶子节点值预测函数
self._leaf_value_calculation = None
# 损失函数
self.loss = loss
### 决策树拟合函数
def fit(self, X, y, loss=None):
# 递归构建决策树
self.root = self._build_tree(X, y)
self.loss = None
### 决策树构建函数
def _build_tree(self, X, y, current_depth=0):
# 初始化最小基尼不纯度
init_gini_impurity = 999
# 初始化最佳特征索引和阈值
best_criteria = None
# 初始化数据子集
best_sets = None
if len(np.shape(y)) == 1:
y = np.expand_dims(y, axis=1)
# 合并输入和标签
Xy = np.concatenate((X, y), axis=1)
# 获取样本数和特征数
n_samples, n_features = X.shape
# 设定决策树构建条件
# 训练样本数量大于节点最小分裂样本数且当前树深度小于最大深度
if n_samples >= self.min_samples_split and current_depth <= self.max_depth:
# 遍历计算每个特征的基尼不纯度
for feature_i in range(n_features):
# 获取第i特征的所有取值
feature_values = np.expand_dims(X[:, feature_i], axis=1)
# 获取第i个特征的唯一取值
unique_values = np.unique(feature_values)
# 遍历取值并寻找最佳特征分裂阈值
for threshold in unique_values:
# 特征节点二叉分裂
Xy1, Xy2 = feature_split(Xy, feature_i, threshold)
# 如果分裂后的子集大小都不为0
if len(Xy1) > 0 and len(Xy2) > 0:
# 获取两个子集的标签值
y1 = Xy1[:, n_features:]
y2 = Xy2[:, n_features:]
# 计算基尼不纯度
impurity = self.impurity_calculation(y, y1, y2)
# 获取最小基尼不纯度
# 最佳特征索引和分裂阈值
if impurity < init_gini_impurity:
init_gini_impurity = impurity
best_criteria = {"feature_i": feature_i, "threshold": threshold}
best_sets = {
"leftX": Xy1[:, :n_features],
"lefty": Xy1[:, n_features:],
"rightX": Xy2[:, :n_features],
"righty": Xy2[:, n_features:]
}
# 如果计算的最小不纯度小于设定的最小不纯度
if init_gini_impurity < self.min_gini_impurity:
# 分别构建左右子树
left_branch = self._build_tree(best_sets["leftX"], best_sets["lefty"], current_depth + 1)
right_branch = self._build_tree(best_sets["rightX"], best_sets["righty"], current_depth + 1)
return TreeNode(feature_i=best_criteria["feature_i"], threshold=best_criteria["threshold"], left_branch=left_branch, right_branch=right_branch)
# 计算叶子计算取值
leaf_value = self._leaf_value_calculation(y)
return TreeNode(leaf_value=leaf_value)
### 定义二叉树值预测函数
def predict_value(self, x, tree=None):
if tree is None:
tree = self.root
# 如果叶子节点已有值,则直接返回已有值
if tree.leaf_value is not None:
return tree.leaf_value
# 选择特征并获取特征值
feature_value = x[tree.feature_i]
# 判断落入左子树还是右子树
branch = tree.right_branch
if isinstance(feature_value, int) or isinstance(feature_value, float):
if feature_value >= tree.threshold:
branch = tree.left_branch
elif feature_value == tree.threshold:
branch = tree.right_branch
# 测试子集
return self.predict_value(x, branch)
### 数据集预测函数
def predict(self, X):
y_pred = [self.predict_value(sample) for sample in X]
return y_pred
class ClassificationTree(BinaryDecisionTree):
### 定义基尼不纯度计算过程
def _calculate_gini_impurity(self, y, y1, y2):
p = len(y1) / len(y)
gini = calculate_gini(y)
gini_impurity = p * calculate_gini(y1) + (1-p) * calculate_gini(y2)
return gini_impurity
### 多数投票
def _majority_vote(self, y):
most_common = None
max_count = 0
for label in np.unique(y):
# 统计多数
count = len(y[y == label])
if count > max_count:
most_common = label
max_count = count
return most_common
# 分类树拟合
def fit(self, X, y):
self.impurity_calculation = self._calculate_gini_impurity
self._leaf_value_calculation = self._majority_vote
super(ClassificationTree, self).fit(X, y)
### CART回归树
class RegressionTree(BinaryDecisionTree):
# 计算方差减少量
def _calculate_variance_reduction(self, y, y1, y2):
var_tot = np.var(y, axis=0)
var_y1 = np.var(y1, axis=0)
var_y2 = np.var(y2, axis=0)
frac_1 = len(y1) / len(y)
frac_2 = len(y2) / len(y)
# 计算方差减少量
variance_reduction = var_tot - (frac_1 * var_y1 + frac_2 * var_y2)
return sum(variance_reduction)
# 节点值取平均
def _mean_of_y(self, y):
value = np.mean(y, axis=0)
return value if len(value) > 1 else value[0]
# 回归树拟合
def fit(self, X, y):
self.impurity_calculation = self._calculate_variance_reduction
self._leaf_value_calculation = self._mean_of_y
super(RegressionTree, self).fit(X, y)

正义的彬彬侠
- 粉丝: 3661
- 资源: 13
最新资源
- 《登飞来峰》教学设计.docx
- 《登飞来峰》教学设计与反思.docx
- 《登幽州台歌》课件.pptx
- (178914818)基于STM32的DS18B20温度传感器应用程序
- (177818802)基于Django和Hadoop集群进行的大数据分析平台.zip
- rocketmq-client-cpp-2.2.0编译的5个文件
- (179049424)CNN卷积神经网络Python的代码实现
- PM的matlab代码
- IMG_20241226_170144.jpg
- html+css 圣诞树html网页代码 圣诞节代码html飘雪花
- (177098236)可直接运行,脉冲雷达测速测距的matlab程序,雷达测距matlab代码
- 经典力学教材:Goldstein, Poole, Safko 第三版的详细解析与应用
- (176438242)毕业设计,采用Hadoop+Hive构建数据仓库,使用django+echarts构建前端web网站对业务指标进行可视化呈现
- Java基础知识点总结与实战指南PDF版
- (179458240)鲁棒优化- C&CG算法求解两阶段鲁棒优化
- chrom Axure插件
资源上传下载、课程学习等过程中有任何疑问或建议,欢迎提出宝贵意见哦~我们会及时处理!
点击此处反馈


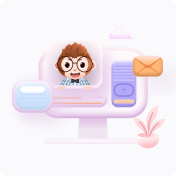