The main goal of this research is to demonstrate how probabilistic graphs may be used for mod- eling and assessment of credit concentration risk. The destructive power of credit concentrations essentially depends on the amount of correlation among borrowers. However, borrower companies correlation and concentration of credit risk exposures have been difficult for the banking industry to measure in an objective way as they are riddled with uncertainty. As a result, banks do not man- age to make a quantitative link to the correlation driving risks and fail to prevent concentrations from accumulating. In this paper, we argue that Bayesian networks provide an attractive solution to these problems and we show how to apply them in representing, quantifying and managing the uncertain knowledge in concentration of credits risk exposures. We suggest the stepwise Bayesian network model building and show how to incorporate expert-based prior beliefs on the risk ex- posure of a group of related borrowers, and then update these beliefs through the whole model with the new information. We then explore a specific graph structure, a tree-augmented Bayesian network and show that this model provides better understanding of the risk accumulating due to business links between borrowers. We also present two strategies of model assessment that exploit the measure of mutual information and show that the constructed Bayesian network is a reliable model that can be implemented to identify and control threat from concentration of credit expo- sures. Finally, we demonstrate that suggested tree-augmented Bayesian network is also suitable for stress-testing analysis, in particular, it can provide the estimates of the posterior risk of losses related to the unfavorable changes in the financial conditions of a group of related borrowers. 信用风险模型化是金融风险管理领域的一个重要课题,尤其是在当前复杂的经济环境中,信用风险的管理和评估对于银行及金融机构的稳定运行至关重要。信用风险,简单来说,是指债务人未能履行合同规定的义务,导致债权人遭受损失的可能性。其中,信用集中风险指的是由于金融机构对某一特定债务人或特定行业、地区、信贷产品的集中暴露导致的风险。 在这篇文章中,作者提出使用贝叶斯网络(Bayesian Networks)来模拟和评估信用集中风险。贝叶斯网络,又称信念网络,是一种图形化的概率模型,它通过概率推理能够处理不确定性,适用于复杂的因果关系建模。利用贝叶斯网络的图形化结构,可以直观地表示出变量之间的依赖关系和条件概率,这为处理信用风险中普遍存在的不确定性和信息不完全问题提供了一种有力工具。 文章的主要贡献在于它提出了一种逐步构建贝叶斯网络模型的方法,首先将专家的知识或先验信念以概率的形式融入模型中,然后通过新信息更新这些信念。这种专家知识与数据驱动相结合的方法能够有效地在模型中体现和量化信用风险相关知识的不确定性。 文章还探讨了一种特殊的图结构——树增强贝叶斯网络(tree-augmented Bayesian network),与传统的贝叶斯网络相比,树增强贝叶斯网络能更好地理解和量化由于借款人之间业务关联所引起的风险积累。树增强贝叶斯网络通过在原有的网络结构中加入树结构,可以表示变量之间更为复杂的依赖关系,这使得模型能够捕捉和反映现实世界中更为复杂的信用风险动态。 在模型评估方面,文章提出了两种策略:一种是利用互信息量度(mutual information)的方法,另一种是后验风险评估,即在相关借款人的财务状况发生变化时,对潜在的损失风险进行估计。这两种评估策略证明了构建的贝叶斯网络模型是一个可靠的工具,可用于识别和控制信用风险暴露的威胁。 此外,文章还证明了所提出的树增强贝叶斯网络模型非常适合进行压力测试分析,尤其是在需要估计一组相关借款人财务状况不利变化时,它能够提供关于后验风险损失的估计。 银行在评估信用风险时面临的一个主要挑战是如何客观地量化借款人之间的相关性以及信用风险暴露的集中度。传统的风险管理方法往往难以准确地测量这些因素,因为它们常常隐藏在不确定性之中。贝叶斯网络提供了一种新的解决方案,它通过图形化和概率推理的方式,将不确定性纳入考虑范围,使得银行能够更准确地衡量信用风险暴露,并提前采取措施以防止风险的积累。 总结来说,这篇文章不仅深化了贝叶斯网络在信用风险管理中的应用,而且通过具体的建模和评估策略,为银行和金融机构提供了一种更为科学和有效的信用风险量化工具。这为风险管理部门在应对信用风险集中问题时提供了更加丰富的分析手段和决策支持,有助于提高金融系统的整体稳健性。
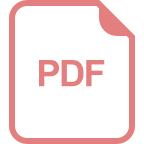
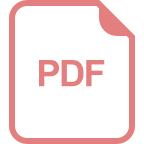
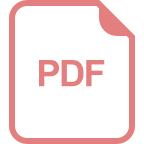
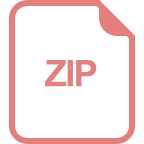
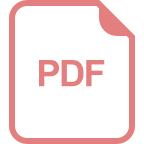
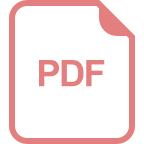
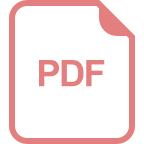
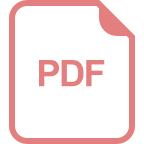
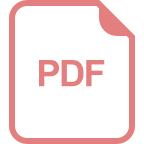
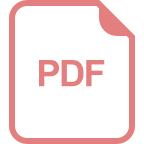
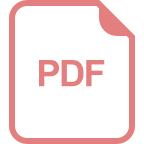
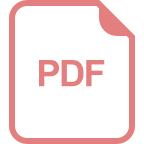
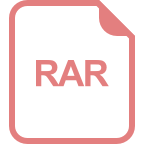
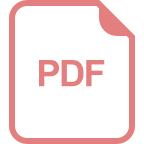
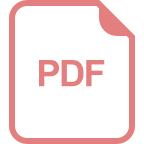
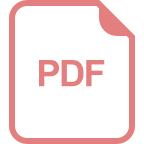
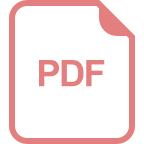
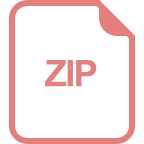
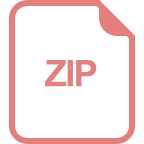
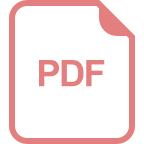
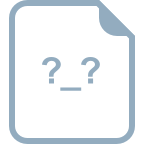
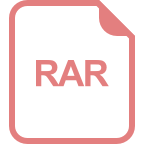
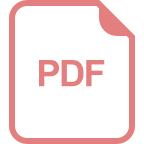
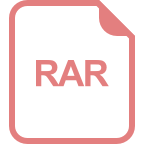
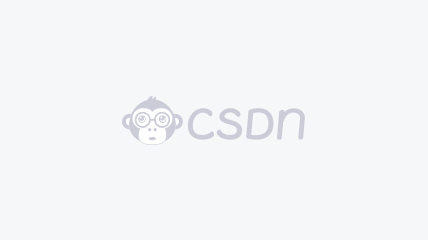

- 粉丝: 0
- 资源: 2
我的内容管理 展开
我的资源 快来上传第一个资源
我的收益
登录查看自己的收益我的积分 登录查看自己的积分
我的C币 登录后查看C币余额
我的收藏
我的下载
下载帮助

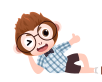
最新资源

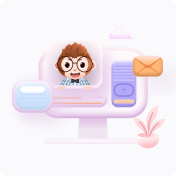
