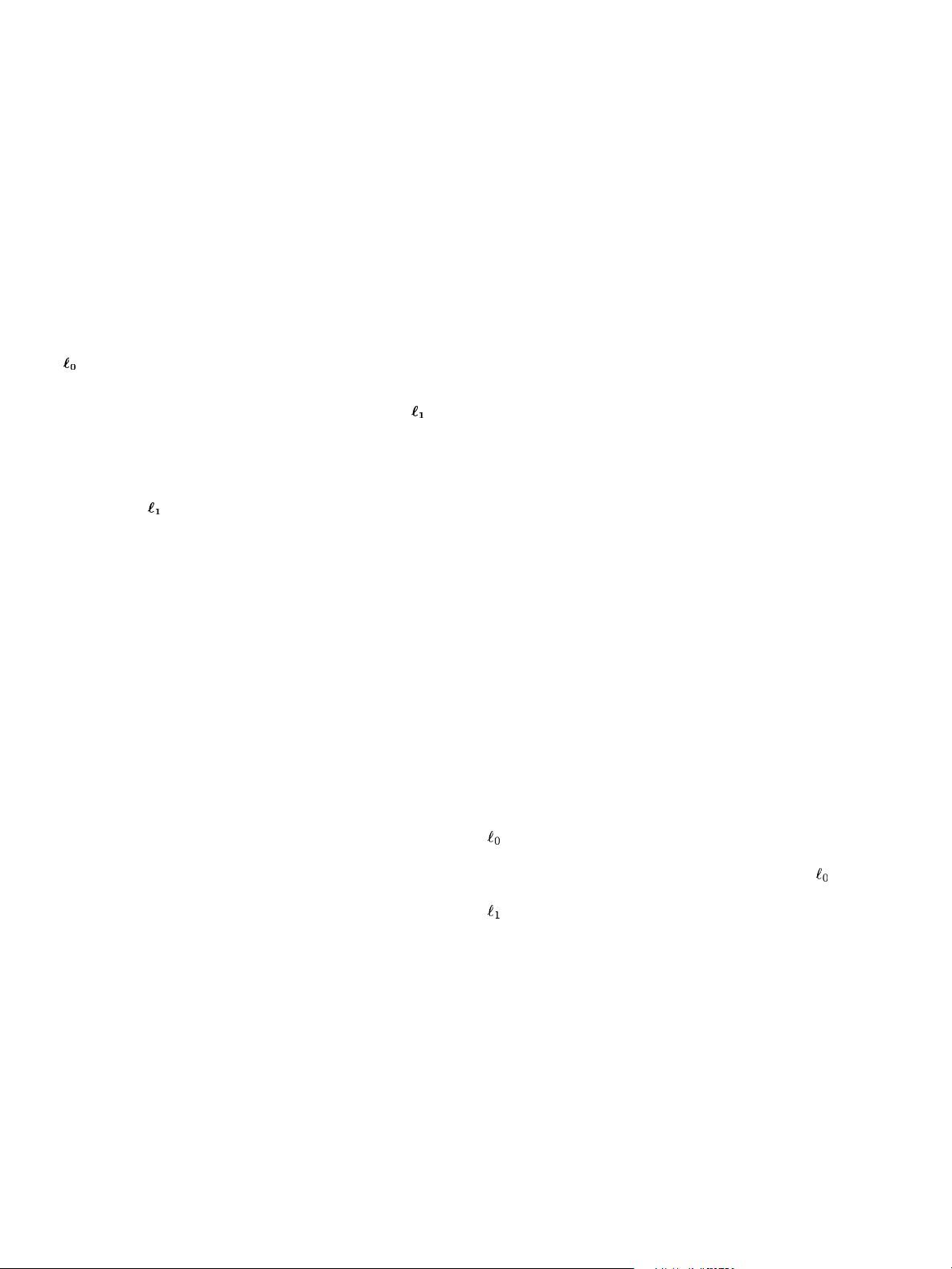
192 IEEE ANTENNAS AND WIRELESS PROPAGATION LETTERS, VOL. 11, 2012
Maximally Sparse Arrays Via Sequential Convex
Optimizations
Giancarlo Prisco and Michele D’Urso
Abstract—The d esign of sparse arrays able to radiate focused
beam patterns satisfying a given upper-bound power
mask with
the minimum number of sources is a research area of increasing
interest. The related synthesis problem can be formulated with
proper constraints on the cardinality of th
e solution space, i.e., its
-norm. Unfortunately, s uch a nonconvex constraint requires to
solve an NP-hard problem. Interesting ideas to relax the above
constraint in a convex way have been succe
ssfully proposed. A
possible solution is based on the minimization of the
-norm.
This strategy is not always able to achieve a maximally sparse
solution. In the following, an innovat
ive synthesis scheme that
optimizes both excitation weights and sensor positions of an
array radiating pencil beam-patterns is discussed. The solution
algorithm is based on sequentia
l convex optimizations including
a reweighted
-norm minimization. Numerical tests, referred to
benchmark problems, show that the proposed synthesis method is
able to achieve maximally spa
rse linear arrays, also compared to
the best results reported in the literature, obtained by means of
global optimization schemes.
Index Terms—Compressed sensing, global optimizations,
sequential convex optimi
zation, sparse array.
I. INTRODUCTION
A
GOOD deal of attention has been devoted to the problem
of synthesizing arb
itrary far-field pattern subject to given
far-field constraints by using the minimal number of sensors.
The problem is of particular interest in t hose application s where
the anten na weigh
t and size m ust be limited [1], [2]. The tools
and algorithms proposed in the open literature can be subdi-
vided in two classes: deterministic and stochastic approaches.
To the first clas
s belong the methods proposed in [1 ]–[7], where
simple formulas are derived for synthesizing given far-field pat-
terns by using uniform amplitude sparse arrays. In [1]–[3], in
particular
, a large num ber of sensors is often required to ful-
fill the design constraints. The number of senso rs is an input
of the solution methods, and it is n o t derived once th e far-field
performan
ces to ach ieve have been given [1]–[3]. A novel al-
gorithm has been proposed in [ 4]–[6]. The proposed approach
allows to determine the minimum number of sensors to fit, with
a fixed a
ccuracy, a reference pattern, given in am plitude and
phase. Concerning [5] and [6], note that th e field distributio n
satisfying the given design constraints is n ot un iqu e, and dif-
feren
t choices for the reference field pattern conduct to different
results in terms of number of required sensors. In particular, the
field distribution to achieve the minimum num ber of sen sors is
Manuscript received November 03, 2011; revised January 06, 2012; accepted
January 27, 2012. Date of publication February 03, 2012; date of current version
March 19, 2012.
The authors are with the SELEX Sistemi Integrati S.p.A., 80014 Giugliano,
Italy (e-mail: gprisco@selex-si.com; mdurso@selex-si.com).
Dig
ital Object Identifier 10.1109/LAWP.2012.2186626
aprioriunknown, and its determination requires to apply the
procedure in [5] and [6] for each fiel d distributio n b
elonging to
the solution set. Furthermore, regarding the reference field to a
set achieved by arbitrarily fixing the sensor positions (equally
spaced half-wavelength array; see [5] and [
6]), there is no guar-
antee that the number of antenn as of the resulting array is the
minimum one.
Therefore, the problem to aprioriestabli
sh the reference
far-field p attern, so as to achieve the solution with the minimum
number of sensor, arises. Such a problem represents the main
drawback also for the compressive s
ensing (CS)- and Bayesian
CS (BCS)-based approaches [8]–[10], wherein the reference
field to fit in order to obtain the maximally sparse array is
aprioriassumed known.
To the second class belong the stochastic ap-
proaches [11]–[14], where different global opti mi zation
methods are exploited for syn
thesizing given radiation patterns
while minimizing the number of required sensors. A nonconvex
properly weighted cost functional is introduced and minimized,
whose global solution, wh
en reached, gives back the minimum
number of required sensors and the corresponding complex
excitations.
In this letter, the synthe
sis problem involving the minimum
number of sensors is addressed from a new perspective. The
array weights can be seen as a sup erposition of spikes, located
in the sensor positio
ns on the array supp ort [15]. The sparse
array i s then synthesized by extracting the sensor positions and
weights from a predefined dense grid, resembling a continu ous
source. Herein, th
e number of required sensors is minimized by
minimizing the cardinality of the current distribution through its
-norm. On the other hand, such a constraint leads to compu-
tationally int
ractable problem s; the p rop osed method is based
on the minimization of a convex measure of the
-norm, as
introduced in [16]. Such a measure outperforms the common
-norm, l a rg
ely exploited in the framework of t he CS-based
optimization prob lems. As a furth er contribution, note that t he
existence of a non-su per-directive source whose radiation pat-
tern fulfil
ls the design constr a int s is verified by taking advantage
from the results in [17] before applying the proposed procedure.
Notably, if the feasibility of the problem is not aprioriveri-
fied, a su
per-directive array may be found as a result, as in [18].
In the proposed synthesis a pproach, differently from [5], [6],
and [8]–[10], only the upper boun d of the p ower pattern is as-
signe
d (and not a reference field, in amplitude and phase), thus
allowing to determine the minimum number of required sensors
independently on the particular reference field.
This l
etter is organized as follows. In Section II, the addressed
synthesis p rob lem is formulated. In Section III, the prop osed
solution method is presented. In Section IV, numerical synthesis
res
ults are p resented and discussed. Conclusions follow.
1536-1225/$31.00 © 2012 IEEE