# yolov8_ros
ROS 2 wrap for [Ultralytics YOLOv8](ultralytics/ultralytics) to perform object detection and tracking, instance segmentation, human pose estimation and Oriented Bounding Box (OBB). There are also 3D versions of object detection, including instance segmentation, and human pose estimation based on depth images.
## Table of Contents
1. [Installation](#installation)
2. [Models](#models)
3. [Usage](#usage)
4. [Demos](#demos)
## Installation
```shell
$ cd ~/ros2_ws/src
$ git clone .git
$ pip3 install -r yolov8_ros/requirements.txt
$ cd ~/ros2_ws
$ rosdep install --from-paths src --ignore-src -r -y
$ colcon build
```
## Models
The available models for yolov8_ros are the following:
- [YOLOv8](https://docs.ultralytics.com/models/yolov8/)
- [YOLOv9](https://docs.ultralytics.com/models/yolov9/)
- [YOLOv10](https://docs.ultralytics.com/models/yolov10/)
- [YOLOv11](https://docs.ultralytics.com/models/yolo11/)
- [YOLO-NAS](https://docs.ultralytics.com/models/yolo-nas/)
## Usage
### YOLOv8 / YOLOv9 / YOLOv10 / YOLOv11 / YOLO-NAS
```shell
$ ros2 launch yolov8_bringup yolov8.launch.py
```
```shell
$ ros2 launch yolov8_bringup yolov9.launch.py
```
```shell
$ ros2 launch yolov8_bringup yolov10.launch.py
```
```shell
$ ros2 launch yolov8_bringup yolov11.launch.py
```
```shell
$ ros2 launch yolov8_bringup yolo-nas.launch.py
```
<p align="center">
<img src="./docs/rqt_graph_yolov8.png" width="100%" />
</p>
#### Topics
- **/yolo/detections**: Objects detected by YOLO using the RGB images. Each object contains a bounding boxes and a class name. It may also include a mak or a list of keypoints.
- **/yolo/tracking**: Objects detected and tracked from YOLO results. Each object is assigned a tracking ID.
- **/yolo/debug_image**: Debug images showing the detected and tracked objects. They can be visualized with rviz2.
#### Parameters
- **model_type**: Ultralytics model type (default: YOLO)
- **model**: YOLOv8 model (default: yolov8m.pt)
- **tracker**: Tracker file (default: bytetrack.yaml)
- **device**: GPU/CUDA (default: cuda:0)
- **enable**: Wether to start YOLOv8 enabled (default: True)
- **threshold**: Detection threshold (default: 0.5)
- **input_image_topic**: Camera topic of RGB images (default: /camera/rgb/image_raw)
- **image_reliability**: Reliability for the image topic: 0=system default, 1=Reliable, 2=Best Effort (default: 2)
### YOLOv8 3D
```shell
$ ros2 launch yolov8_bringup yolov8_3d.launch.py
```
<p align="center">
<img src="./docs/rqt_graph_yolov8_3d.png" width="100%" />
</p>
#### Topics
- **/yolo/detections**: Objects detected by YOLO using the RGB images. Each object contains a bounding boxes and a class name. It may also include a mak or a list of keypoints.
- **/yolo/tracking**: Objects detected and tracked from YOLO results. Each object is assigned a tracking ID.
- **/yolo/detections_3d**: 3D objects detected. YOLO results are used to crop the depth images to create the 3D bounding boxes and 3D keypoints.
- **/yolo/debug_image**: Debug images showing the detected and tracked objects. They can be visualized with rviz2.
#### Parameters
- **model_type**: Ultralytics model type (default: YOLO)
- **model**: YOLOv8 model (default: yolov8m.pt)
- **tracker**: tracker file (default: bytetrack.yaml)
- **device**: GPU/CUDA (default: cuda:0)
- **enable**: wether to start YOLOv8 enabled (default: True)
- **threshold**: detection threshold (default: 0.5)
- **input_image_topic**: camera topic of RGB images (default: /camera/rgb/image_raw)
- **image_reliability**: reliability for the image topic: 0=system default, 1=Reliable, 2=Best Effort (default: 2)
- **input_depth_topic**: camera topic of depth images (default: /camera/depth/image_raw)
- **depth_image_reliability**: reliability for the depth image topic: 0=system default, 1=Reliable, 2=Best Effort (default: 2)
- **input_depth_info_topic**: camera topic for info data (default: /camera/depth/camera_info)
- **depth_info_reliability**: reliability for the depth info topic: 0=system default, 1=Reliable, 2=Best Effort (default: 2)
- **depth_image_units_divisor**: divisor to convert the depth image into metres (default: 1000)
- **target_frame**: frame to transform the 3D boxes (default: base_link)
- **maximum_detection_threshold**: maximum detection threshold in the z axis (default: 0.3)
## Lifecycle nodes
Previous updates add Lifecycle Nodes support to all the nodes available in the package.
This implementation tries to reduce the workload in the unconfigured and inactive states by only loading the models and activating the subscriber on the active state.
These are some resource comparisons using the default yolov8m.pt model on a 30fps video stream.
| State | CPU Usage (i7 12th Gen) | VRAM Usage | Bandwidth Usage |
| -------- | ----------------------- | ---------- | --------------- |
| Active | 40-50% in one core | 628 MB | Up to 200 Mbps |
| Inactive | ~5-7% in one core | 338 MB | 0-20 Kbps |
## Demos
## Object Detection
This is the standard behavior of YOLOv8, which includes object tracking.
```shell
$ ros2 launch yolov8_bringup yolov8.launch.py
```
[](https://drive.google.com/file/d/1gTQt6soSIq1g2QmK7locHDiZ-8MqVl2w/view?usp=sharing)
## Instance Segmentation
Instance masks are the borders of the detected objects, not the all the pixels inside the masks.
```shell
$ ros2 launch yolov8_bringup yolov8.launch.py model:=yolov8m-seg.pt
```
[](https://drive.google.com/file/d/1dwArjDLSNkuOGIB0nSzZR6ABIOCJhAFq/view?usp=sharing)
## Human Pose
Online persons are detected along with their keypoints.
```shell
$ ros2 launch yolov8_bringup yolov8.launch.py model:=yolov8m-pose.pt
```
[](https://drive.google.com/file/d/1pRy9lLSXiFEVFpcbesMCzmTMEoUXGWgr/view?usp=sharing)
## 3D Object Detection
The 3D bounding boxes are calculated filtering the depth image data from an RGB-D camera using the 2D bounding box. Only objects with a 3D bounding box are visualized in the 2D image.
```shell
$ ros2 launch yolov8_bringup yolov8_3d.launch.py
```
[](https://drive.google.com/file/d/1ZcN_u9RB9_JKq37mdtpzXx3b44tlU-pr/view?usp=sharing)
## 3D Object Detection (Using Instance Segmentation Masks)
In this, the depth image data is filtered using the max and min values obtained from the instance masks. Only objects with a 3D bounding box are visualized in the 2D image.
```shell
$ ros2 launch yolov8_bringup yolov8_3d.launch.py model:=yolov8m-seg.pt
```
[](https://drive.google.com/file/d/1wVZgi5GLkAYxv3GmTxX5z-vB8RQdwqLP/view?usp=sharing)
## 3D Human Pose
Each keypoint is projected in the depth image and visualized using purple spheres. Only objects with a 3D bounding box are visualized in the 2D image.
```shell
$ ros2 launch yolov8_bringup yolov8_3d.launch.py model:=yolov8m-pose.pt
```
[](https://drive.google.com/file/d/1j4VjCAsOCx_mtM2KFPOLkpJogM0t227r/view?usp=sharing)
免责声明:
1.本资源仅供学习和交流使用,不保证其准确性、完整性、及时性或适用性。
2.本资源仅包含一般信息,不构成专业建议。在使用本资源时,请务必自行研究并谨慎决策。
3.我已尽力确保本资源的正确性和合法性,但不对其准确性、完整性和及时性做出保证。
4.本资源不应用于商业用途。
5.在使用本资源的过程中,用户应自行承担所有风险和责任,并遵守相关法律法规。
6.对于因使用本资�

苹果酱0567
- 粉丝: 2045
- 资源: 1102
最新资源
- 麻雀优化算法SSA优化BP做多特征输入单个因变量输出的分类模型 程序内注释详细直接替数据就可以用 想要的加好友我
- 麻雀优化算法SSA优化深度学习机DELM,建立多特征输入单个因变量输出的拟合预测模型 程序内注释详细直接替数据就可以用 程序语言为matlab
- mmexport1736592575149.jpg
- NFC线圈设计#HFSS分析设计13.56MHz RFID天线及其匹配电路 ①在HFSS中创建参数化的线圈天线模型...... ②使用HFSS分析查看天线在13.56GHz工作频率上的等效电感值、等生
- Hands-On-Machine-Learning-with-Scikit-Learn-and-TensorFlow-3rd-Edition
- 针对速度环的滑模控制器永磁同步电机矢量控制仿真模型,PMSM滑模速度控制器算法,使用matlab simulink搭建,以供参考学习
- Video-2024-09-28下午-聊天1.0案例基础引导逻辑.wmv
- A星路径规划算法,Matlab实现A星算法,可自己改变地图和障碍物,自定义起点坐标和终点坐标
- 基于核极限学习机KELM、在线顺序极限学习机OS-ELM、在线贯序核极限学习机OSKELM、遗忘因子的在线贯列核极限学习F-OSKELM和自适应遗忘因子的在线贯列核极限学习AF-OSKELM数据预测
- 考虑 化成本的混合储能微电网双层能量管理系统(复现) 本文的主要贡献如下:1)提出了一种新型的包含混合ESS的两层微电网EMS 电力调度的目标是上层的运行成本最小,下层的预测不确定性和电力波动最小
- bms电池管理系统 锂电池算法SOC代码 获取锂电池SOC采用的是电流积分法,电化学阻抗法 电流积分法又称为安时积分法或库伦计数,通过将电池电流对时间进行积分来计算电池的荷电状态 这种方法对于计算
- 基于SSM的“软件缺陷管理系统”的设计与实现(源码+数据库+文档+PPT).zip
- 西门子S7-1200PLC双轴定位算法电池焊接控制博图程序案例,触摸屏画面采用威纶触摸屏 程序设计结构灵活,采用SCL语言+梯形图结构,项目包括: 1.博图V15PLC程序 2.威纶通触摸屏程序
- 基于遗传算法的微电网储能配置方法 搭建以储能配置综合成本最低和供电可靠性最高为目标函数,并考虑DG电源约束、储能充放电约束和负荷平衡约束的多目标优化模型 在传统建立成本目标函数时只考虑单一投资成本的
- 基于matlab的二维小波相干分析,以空气质量数据为例 进行二维小波相干分析
- 基于simulink直流无刷电机仿真模型 速度电流闭环PID反馈控制 另外还有一个三相电机模型(图4)模型良好,调试完美 如图
资源上传下载、课程学习等过程中有任何疑问或建议,欢迎提出宝贵意见哦~我们会及时处理!
点击此处反馈


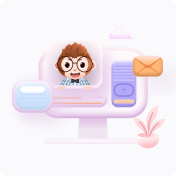