benchncnn can be used to test neural network inference performance
Only the network definition files (ncnn param) are required.
The large model binary files (ncnn bin) are not loaded but generated randomly for speed test.
More model networks may be added later.
---
Build
```shell
# assume you have already build ncnn library successfully
# uncomment the following line in <ncnn-root-dir>/CMakeLists.txt with your favorite editor
# add_subdirectory(benchmark)
cd <ncnn-root-dir>/<your-build-dir>
make -j4
# you can find benchncnn binary in <ncnn-root-dir>/<your-build-dir>/benchmark
```
Usage
```shell
# copy all param files to the current directory
./benchncnn [loop count] [num threads] [powersave] [gpu device] [cooling down]
```
run benchncnn on android device
```shell
# for running on android device, upload to /data/local/tmp/ folder
adb push benchncnn /data/local/tmp/
adb push <ncnn-root-dir>/benchmark/*.param /data/local/tmp/
adb shell
# executed in android adb shell
cd /data/local/tmp/
./benchncnn [loop count] [num threads] [powersave] [gpu device] [cooling down]
```
Parameter
|param|options|default|
|---|---|---|
|loop count|1~N|4|
|num threads|1~N|max_cpu_count|
|powersave|0=all cores, 1=little cores only, 2=big cores only|0|
|gpu device|-1=cpu-only, 0=gpu0, 1=gpu1 ...|-1|
|cooling down|0=disable, 1=enable|1|
Tips: Disable android UI server and set CPU and GPU to max frequency
```shell
# stopping android ui server, can be retarted later via adb shell start
adb root
adb shell stop
# executed in android adb shell
# set cpu performance mode
echo "performance" > /sys/devices/system/cpu/cpu0/cpufreq/scaling_governor
echo "performance" > /sys/devices/system/cpu/cpu1/cpufreq/scaling_governor
echo "performance" > /sys/devices/system/cpu/cpu2/cpufreq/scaling_governor
echo "performance" > /sys/devices/system/cpu/cpu3/cpufreq/scaling_governor
echo "performance" > /sys/devices/system/cpu/cpu4/cpufreq/scaling_governor
echo "performance" > /sys/devices/system/cpu/cpu5/cpufreq/scaling_governor
# set gpu performance mode (eg. RK3399)
echo "performance" > /sys/class/misc/mali0/device/devfreq/ff9a0000.gpu/governor
# set gpu performance mode (eg. Android Adreno)
echo 1 > /sys/class/kgsl/kgsl-3d0/force_clk_on
echo 10000000 > /sys/class/kgsl/kgsl-3d0/idle_timer
echo "performance" > /sys/class/kgsl/kgsl-3d0/devfreq/governor
echo <max freq> > /sys/class/kgsl/kgsl-3d0/gpuclk
```
---
Typical output (executed in android adb shell)
### NVIDIA Jetson AGX Orin (Cortex-A78AE 2.2 GHz x 12 + Ampere@1.3 GHz Tensor Cores 64)
```
i@orin:~/projects/ncnn/benchmark$ ./benchncnn 64 1 0 -1 0
loop_count = 64
num_threads = 1
powersave = 0
gpu_device = -1
cooling_down = 0
squeezenet min = 11.66 max = 11.80 avg = 11.74
squeezenet_int8 min = 12.24 max = 12.39 avg = 12.31
mobilenet min = 19.56 max = 19.73 avg = 19.65
mobilenet_int8 min = 16.06 max = 16.25 avg = 16.14
mobilenet_v2 min = 13.20 max = 13.41 avg = 13.29
mobilenet_v3 min = 11.39 max = 11.57 avg = 11.48
shufflenet min = 8.07 max = 8.18 avg = 8.11
shufflenet_v2 min = 8.41 max = 8.51 avg = 8.45
mnasnet min = 12.74 max = 12.91 avg = 12.79
proxylessnasnet min = 15.18 max = 15.32 avg = 15.25
efficientnet_b0 min = 26.86 max = 26.96 avg = 26.90
efficientnetv2_b0 min = 35.99 max = 36.15 avg = 36.07
regnety_400m min = 16.81 max = 16.98 avg = 16.87
blazeface min = 4.25 max = 4.37 avg = 4.29
googlenet min = 48.73 max = 48.98 avg = 48.87
googlenet_int8 min = 47.39 max = 47.60 avg = 47.49
resnet18 min = 30.93 max = 31.24 avg = 31.08
resnet18_int8 min = 55.44 max = 55.70 avg = 55.56
alexnet min = 44.19 max = 44.43 avg = 44.33
vgg16 min = 173.94 max = 174.97 avg = 174.46
vgg16_int8 min = 475.10 max = 479.37 avg = 477.33
resnet50 min = 89.50 max = 90.11 avg = 89.80
resnet50_int8 min = 106.77 max = 107.14 avg = 106.96
squeezenet_ssd min = 37.78 max = 38.35 avg = 37.93
squeezenet_ssd_int8 min = 50.48 max = 50.88 avg = 50.74
mobilenet_ssd min = 45.62 max = 46.12 avg = 45.74
mobilenet_ssd_int8 min = 37.77 max = 38.00 avg = 37.88
mobilenet_yolo min = 90.23 max = 90.49 avg = 90.35
mobilenetv2_yolov3 min = 47.27 max = 47.48 avg = 47.33
yolov4-tiny min = 60.41 max = 60.75 avg = 60.57
nanodet_m min = 19.26 max = 19.43 avg = 19.35
yolo-fastest-1.1 min = 8.16 max = 8.31 avg = 8.20
yolo-fastestv2 min = 8.26 max = 8.39 avg = 8.32
i@orin:~/projects/ncnn/benchmark$ ./benchncnn 64 2 0 -1 0
loop_count = 64
num_threads = 2
powersave = 0
gpu_device = -1
cooling_down = 0
squeezenet min = 6.83 max = 6.98 avg = 6.90
squeezenet_int8 min = 7.39 max = 7.50 avg = 7.45
mobilenet min = 10.40 max = 10.50 avg = 10.45
mobilenet_int8 min = 8.92 max = 9.09 avg = 8.99
mobilenet_v2 min = 7.67 max = 7.80 avg = 7.74
mobilenet_v3 min = 6.86 max = 7.01 avg = 6.93
shufflenet min = 6.34 max = 6.44 avg = 6.39
shufflenet_v2 min = 5.71 max = 5.83 avg = 5.76
mnasnet min = 7.47 max = 7.58 avg = 7.53
proxylessnasnet min = 8.73 max = 8.83 avg = 8.78
efficientnet_b0 min = 14.93 max = 15.13 avg = 15.03
efficientnetv2_b0 min = 20.17 max = 20.70 avg = 20.29
regnety_400m min = 12.50 max = 12.62 avg = 12.57
blazeface min = 2.95 max = 3.06 avg = 3.00
googlenet min = 26.25 max = 26.53 avg = 26.37
googlenet_int8 min = 26.54 max = 26.79 avg = 26.66
resnet18 min = 16.69 max = 16.90 avg = 16.80
resnet18_int8 min = 29.70 max = 29.93 avg = 29.81
alexnet min = 22.96 max = 23.12 avg = 23.03
vgg16 min = 88.39 max = 89.16 avg = 88.79
vgg16_int8 min = 245.86 max = 247.55 avg = 246.62
resnet50 min = 46.55 max = 46.86 avg = 46.70
resnet50_int8 min = 56.28 max = 56.63 avg = 56.43
squeezenet_ssd min = 23.65 max = 24.29 avg = 23.81
squeezenet_ssd_int8 min = 30.86 max = 31.27 avg = 30.99
mobilenet_ssd min = 25.17 max = 25.31 avg = 25.24
mobilenet_ssd_int8 min = 21.77 max = 21.97 avg = 21.84
mobilenet_yolo min = 48.03 max = 48.33 avg = 48.14
mobilenetv2_yolov3 min = 26.58 max = 26.81 avg = 26.66
yolov4-tiny min = 35.31 max = 35.53 avg = 35.41
nanodet_m min = 12.93 max = 13.08 avg = 13.01
yolo-fastest-1.1 min = 6.00 max = 6.10 avg = 6.04
yolo-fastestv2 min = 6.46 max = 6.61 avg = 6.52
i@orin:~/projects/ncnn/benchmark$ ./benchncnn 64 4 0 -1 0
loop_count = 64
num_threads = 4
powersave = 0
gpu_device = -1
cooling_down = 0
squeezenet min = 4.54 max = 4.84 avg = 4.61
squeezenet_int8 min = 4.96 max = 5.41 avg = 5.05
mobilenet min = 5.96 max = 6.23 avg = 6.04
mobilenet_int8 min = 5.21 max = 5.50 avg = 5.30
mobilenet_v2 min = 5.05 max = 5.26 avg = 5.15
mobilenet_v3 min = 4.83 max = 5.14 avg = 4.90
shufflenet min = 5.11 max = 5.34 avg = 5.18
shufflenet_v2 min = 4.13 max = 4.44 avg = 4.18
mnasnet min = 4.93 max = 5.27 avg = 5.01
proxylessnasnet min = 5.64 max = 5.89 avg = 5.72
efficient
没有合适的资源?快使用搜索试试~ 我知道了~
温馨提示
项目工程资源经过严格测试可直接运行成功且功能正常的情况才上传,可轻松复刻,拿到资料包后可轻松复现出一样的项目,本人系统开发经验充足(全领域),有任何使用问题欢迎随时与我联系,我会及时为您解惑,提供帮助。 【资源内容】:包含完整源码+工程文件+说明(如有)等。答辩评审平均分达到96分,放心下载使用!可轻松复现,设计报告也可借鉴此项目,该资源内项目代码都经过测试运行成功,功能ok的情况下才上传的。 【提供帮助】:有任何使用问题欢迎随时与我联系,我会及时解答解惑,提供帮助 【附带帮助】:若还需要相关开发工具、学习资料等,我会提供帮助,提供资料,鼓励学习进步 【项目价值】:可用在相关项目设计中,皆可应用在项目、毕业设计、课程设计、期末/期中/大作业、工程实训、大创等学科竞赛比赛、初期项目立项、学习/练手等方面,可借鉴此优质项目实现复刻,设计报告也可借鉴此项目,也可基于此项目来扩展开发出更多功能 下载后请首先打开README文件(如有),项目工程可直接复现复刻,如果基础还行,也可在此程序基础上进行修改,以实现其它功能。供开源学习/技术交流/学习参考,勿用于商业用途。质量优质,放心下载使用。
资源推荐
资源详情
资源评论
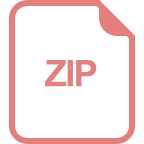
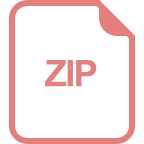
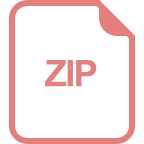
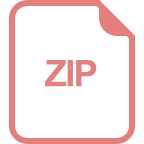
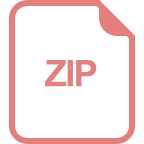
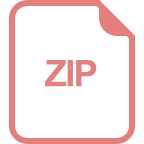
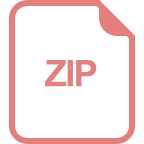
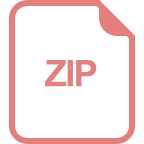
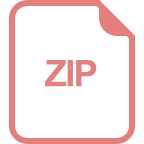
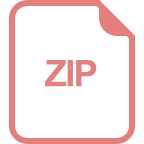
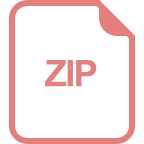
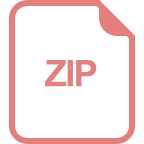
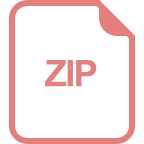
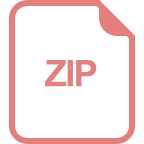
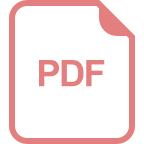
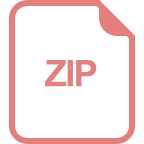
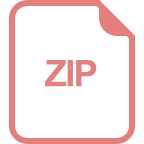
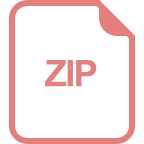
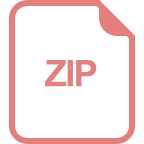
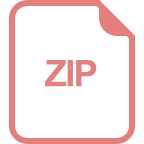
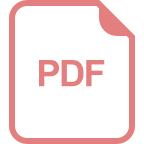
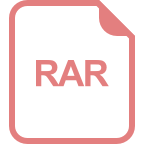
收起资源包目录

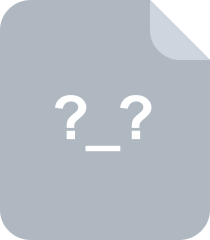
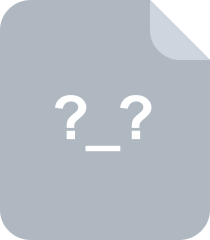
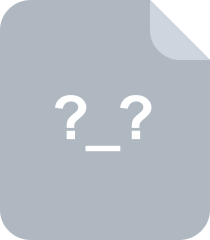
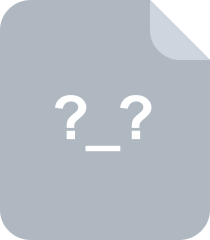
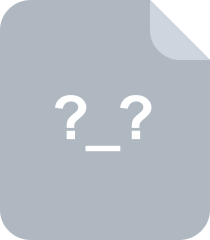
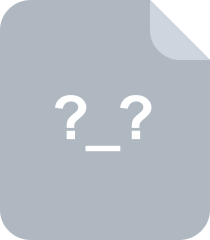
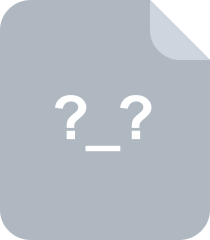
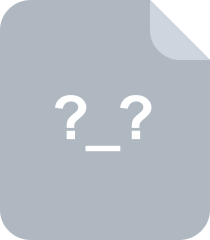
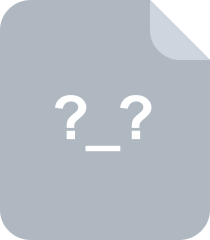
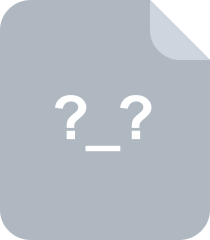
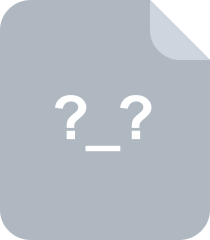
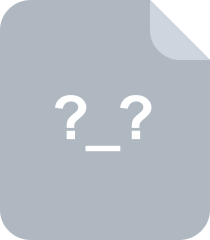
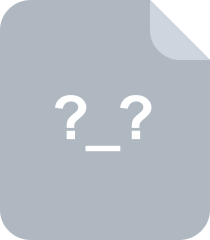
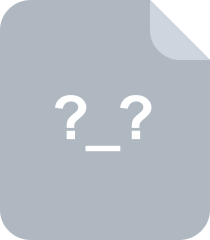
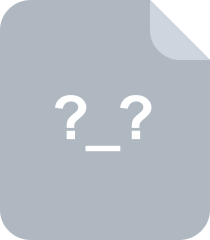
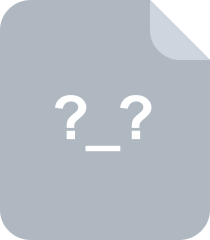
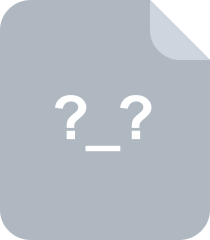
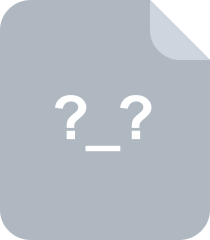
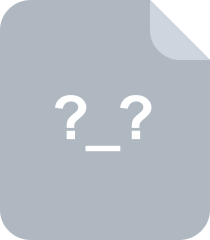
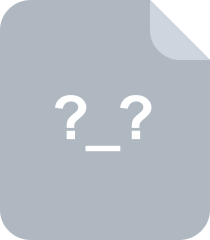
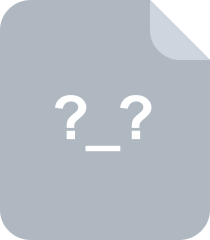
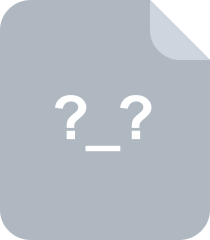
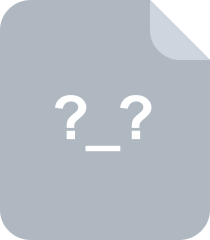
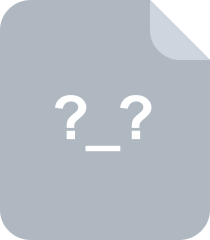
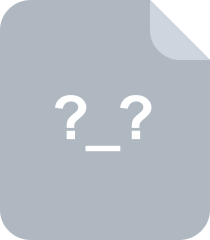
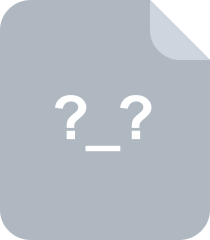
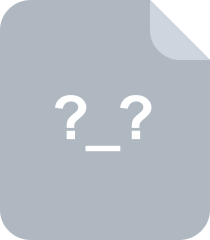
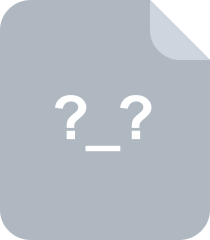
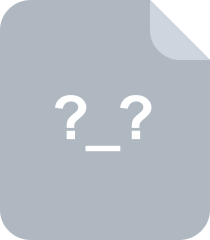
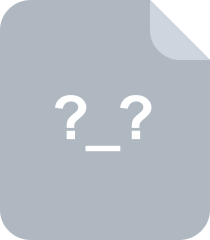
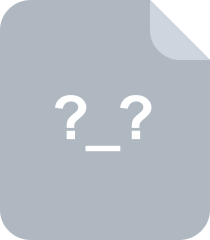
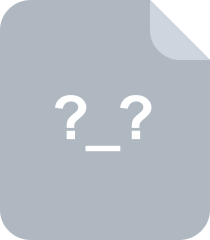
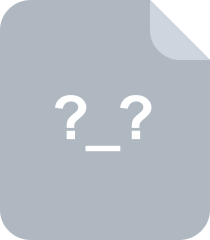
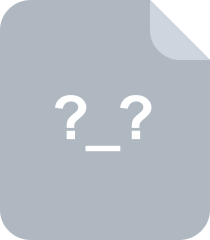
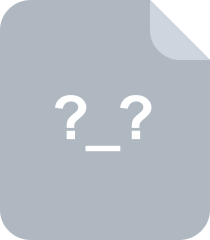
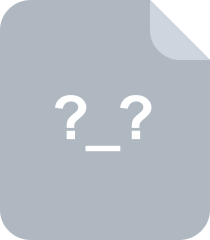
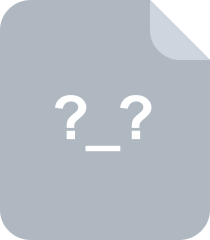
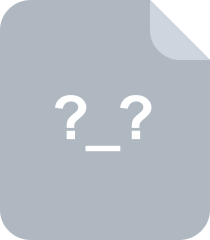
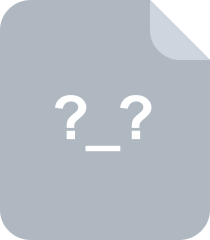
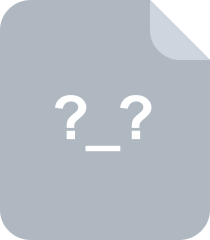
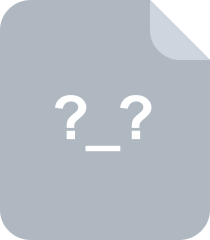
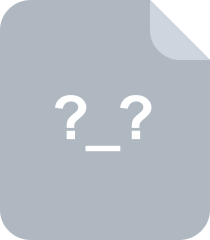
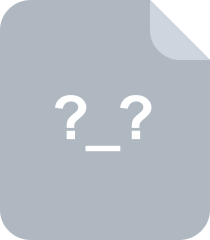
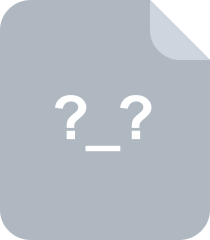
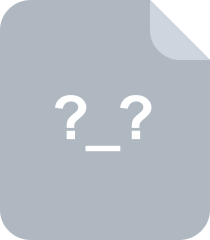
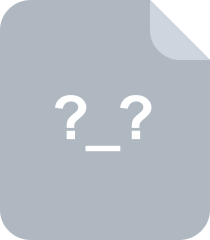
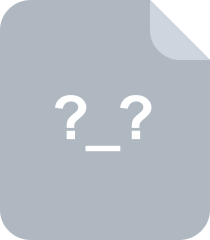
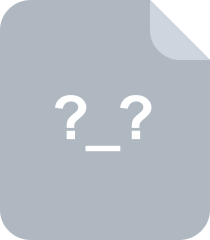
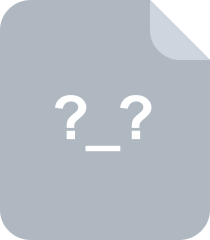
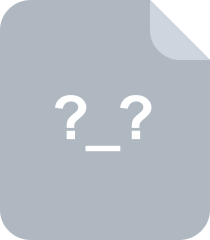
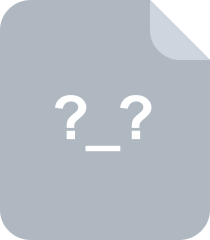
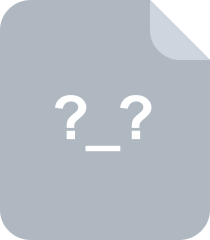
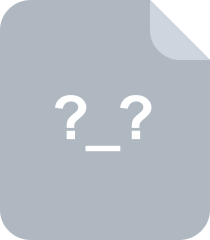
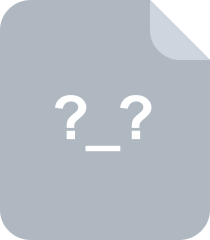
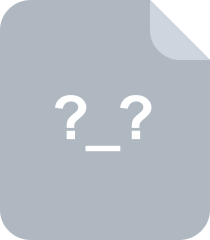
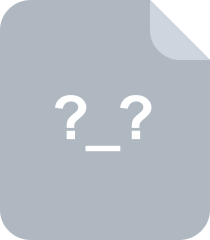
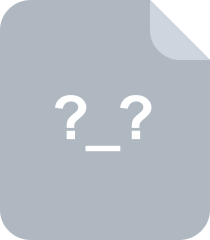
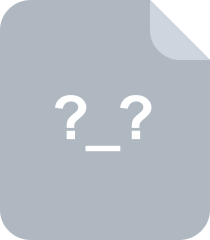
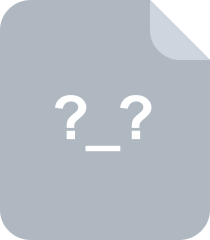
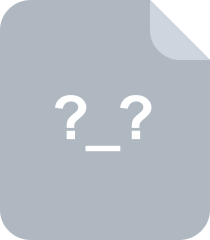
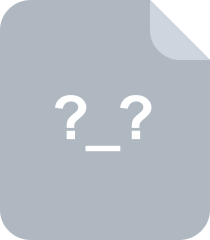
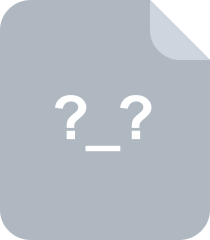
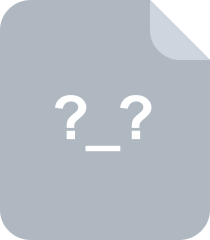
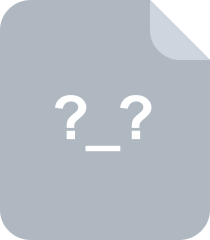
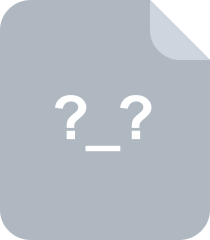
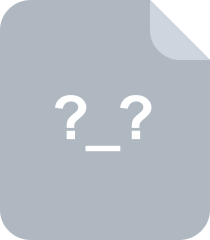
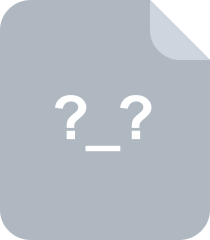
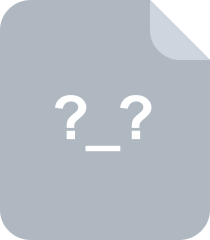
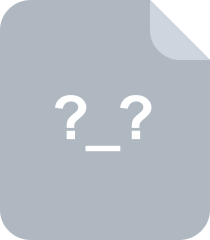
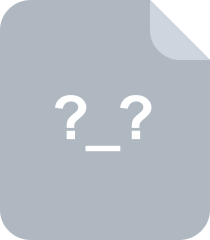
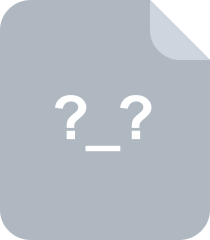
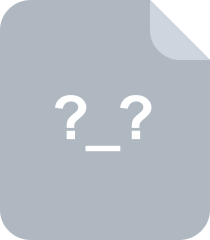
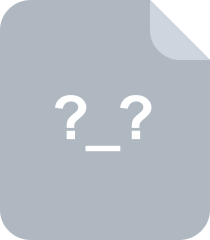
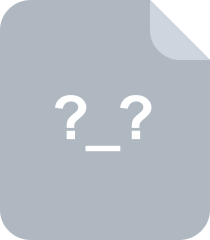
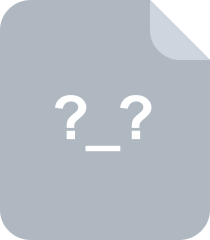
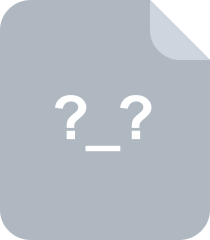
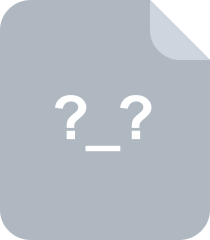
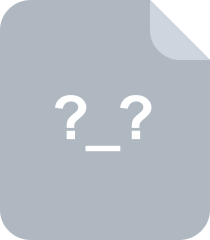
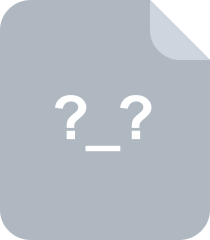
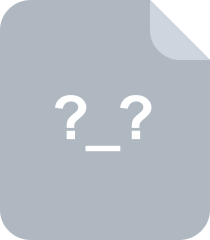
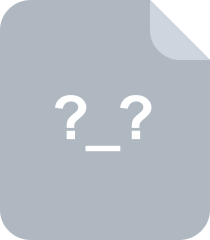
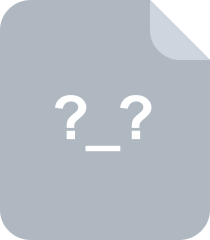
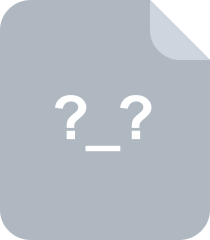
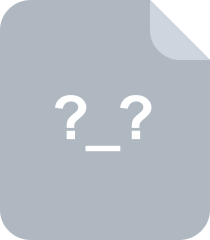
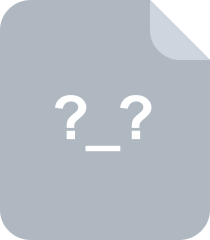
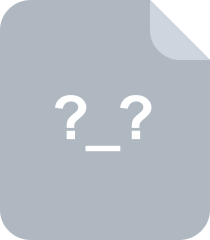
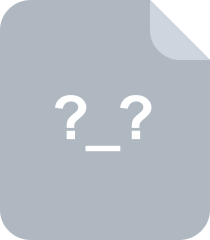
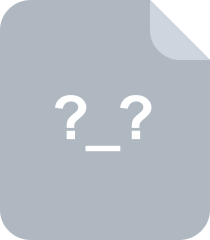
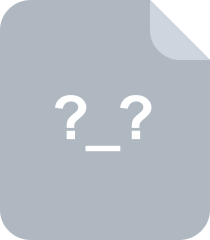
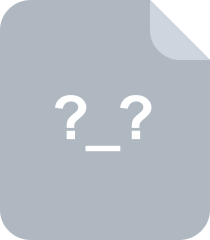
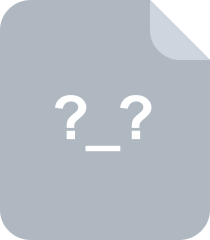
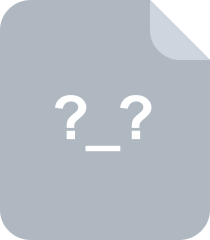
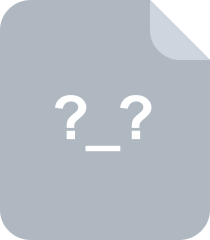
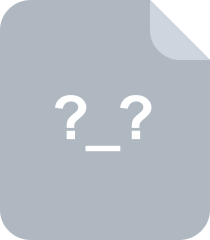
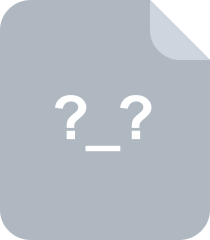
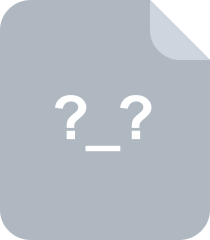
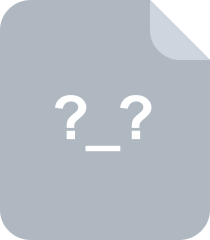
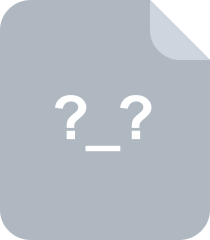
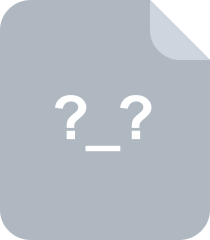
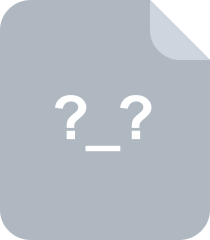
共 2003 条
- 1
- 2
- 3
- 4
- 5
- 6
- 21
资源评论
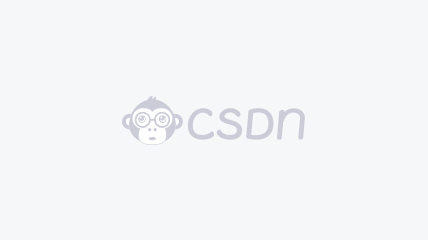

热爱技术。
- 粉丝: 2935
- 资源: 7864
上传资源 快速赚钱
我的内容管理 展开
我的资源 快来上传第一个资源
我的收益
登录查看自己的收益我的积分 登录查看自己的积分
我的C币 登录后查看C币余额
我的收藏
我的下载
下载帮助

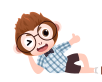
最新资源
- 精选毕设项目-蒙台梭利幼教.zip
- 精选毕设项目-母婴商城.zip
- 精选毕设项目-面包旅行.zip
- 精选毕设项目-柠檬树婚纱照.zip
- 精选毕设项目-企业OA系统小程序.zip
- 精选毕设项目-平安保险小程序.zip
- 凹凸社区APP源码蜜桃社区源码+视频搭建教程
- 精选毕设项目-汽车测评小程序.zip
- 精选毕设项目-企业版商城小程序.zip
- 精选毕设项目-汽车维修保养商店小程序.zip
- 精选毕设项目-仁怀酱酒宝:酒类商城模板.zip
- 精选毕设项目-扫码购物商城.zip
- 精选毕设项目-人民好公仆小程序(生活+便民+政务).zip
- 精选毕设项目-谁是杀手小程序游戏.zip
- 精选毕设项目-世博展会小程序.zip
- 精选毕设项目-守望先锋资讯小程序.zip
资源上传下载、课程学习等过程中有任何疑问或建议,欢迎提出宝贵意见哦~我们会及时处理!
点击此处反馈


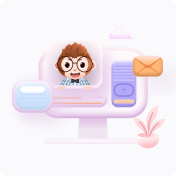
安全验证
文档复制为VIP权益,开通VIP直接复制
