# Yolo-v4 and Yolo-v3/v2 for Windows and Linux
### (neural network for object detection) - Tensor Cores can be used on [Linux](https://github.com/AlexeyAB/darknet#how-to-compile-on-linux) and [Windows](https://github.com/AlexeyAB/darknet#how-to-compile-on-windows-using-cmake-gui)
Paper Yolo v4: https://arxiv.org/abs/2004.10934
More details: [medium link](https://medium.com/@alexeyab84/yolov4-the-most-accurate-real-time-neural-network-on-ms-coco-dataset-73adfd3602fe?source=friends_link&sk=6039748846bbcf1d960c3061542591d7)
Discussion: [Reddit](https://www.reddit.com/r/MachineLearning/comments/gydxzd/p_yolov4_the_most_accurate_realtime_neural/)
About Darknet framework: http://pjreddie.com/darknet/
[](https://github.com/AlexeyAB/darknet/actions?query=workflow%3A%22Darknet+Continuous+Integration%22)
[](https://circleci.com/gh/AlexeyAB/darknet)
[](https://travis-ci.org/AlexeyAB/darknet)
[](https://github.com/AlexeyAB/darknet/graphs/contributors)
[](https://github.com/AlexeyAB/darknet/blob/master/LICENSE)
[](https://zenodo.org/badge/latestdoi/75388965)
[](https://arxiv.org/abs/2004.10934)
[](https://colab.research.google.com/drive/12QusaaRj_lUwCGDvQNfICpa7kA7_a2dE)
[](https://colab.research.google.com/drive/1_GdoqCJWXsChrOiY8sZMr_zbr_fH-0Fg)
* [YOLOv4 model zoo](https://github.com/AlexeyAB/darknet/wiki/YOLOv4-model-zoo)
* [Requirements (and how to install dependecies)](#requirements)
* [Pre-trained models](#pre-trained-models)
* [FAQ - frequently asked questions](https://github.com/AlexeyAB/darknet/wiki/FAQ---frequently-asked-questions)
* [Explanations in issues](https://github.com/AlexeyAB/darknet/issues?q=is%3Aopen+is%3Aissue+label%3AExplanations)
* [Yolo v4 in other frameworks (TensorRT, TensorFlow, PyTorch, OpenVINO, OpenCV-dnn, TVM,...)](#yolo-v4-in-other-frameworks)
* [Datasets](#datasets)
0. [Improvements in this repository](#improvements-in-this-repository)
1. [How to use](#how-to-use-on-the-command-line)
2. How to compile on Linux
* [Using cmake](#how-to-compile-on-linux-using-cmake)
* [Using make](#how-to-compile-on-linux-using-make)
3. How to compile on Windows
* [Using CMake-GUI](#how-to-compile-on-windows-using-cmake)
* [Using vcpkg](#how-to-compile-on-windows-using-vcpkg)
* [Legacy way](#how-to-compile-on-windows-legacy-way)
4. [Training and Evaluation of speed and accuracy on MS COCO](https://github.com/AlexeyAB/darknet/wiki#training-and-evaluation-of-speed-and-accuracy-on-ms-coco)
5. [How to train with multi-GPU:](#how-to-train-with-multi-gpu)
6. [How to train (to detect your custom objects)](#how-to-train-to-detect-your-custom-objects)
7. [How to train tiny-yolo (to detect your custom objects)](#how-to-train-tiny-yolo-to-detect-your-custom-objects)
8. [When should I stop training](#when-should-i-stop-training)
9. [How to improve object detection](#how-to-improve-object-detection)
10. [How to mark bounded boxes of objects and create annotation files](#how-to-mark-bounded-boxes-of-objects-and-create-annotation-files)
11. [How to use Yolo as DLL and SO libraries](#how-to-use-yolo-as-dll-and-so-libraries)
| 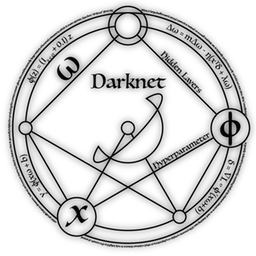 | 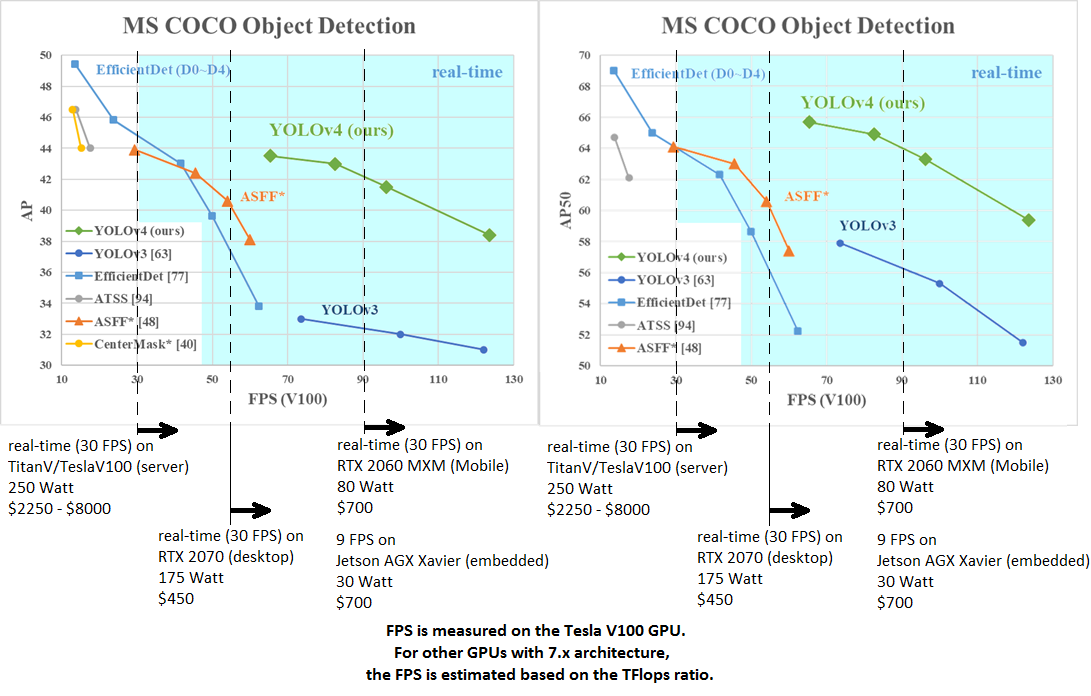 AP50:95 / AP50 - FPS (Tesla V100) Paper: https://arxiv.org/abs/2004.10934 |
|---|---|
tkDNN-TensorRT accelerates YOLOv4 **~2x** times for batch=1 and **3x-4x** times for batch=4.
* tkDNN: https://github.com/ceccocats/tkDNN
* OpenCV: https://gist.github.com/YashasSamaga/48bdb167303e10f4d07b754888ddbdcf
#### GeForce RTX 2080 Ti:
| Network Size | Darknet, FPS (avg)| tkDNN TensorRT FP32, FPS | tkDNN TensorRT FP16, FPS | OpenCV FP16, FPS | tkDNN TensorRT FP16 batch=4, FPS | OpenCV FP16 batch=4, FPS | tkDNN Speedup |
|:-----:|:--------:|--------:|--------:|--------:|--------:|--------:|------:|
|320 | 100 | 116 | **202** | 183 | 423 | **430** | **4.3x** |
|416 | 82 | 103 | **162** | 159 | 284 | **294** | **3.6x** |
|512 | 69 | 91 | 134 | **138** | 206 | **216** | **3.1x** |
|608 | 53 | 62 | 103 | **115**| 150 | **150** | **2.8x** |
|Tiny 416 | 443 | 609 | **790** | 773 | **1774** | 1353 | **3.5x** |
|Tiny 416 CPU Core i7 7700HQ | 3.4 | - | - | 42 | - | 39 | **12x** |
* Yolo v4 Full comparison: [map_fps](https://user-images.githubusercontent.com/4096485/80283279-0e303e00-871f-11ea-814c-870967d77fd1.png)
* Yolo v4 tiny comparison: [tiny_fps](https://user-images.githubusercontent.com/4096485/85734112-6e366700-b705-11ea-95d1-fcba0de76d72.png)
* CSPNet: [paper](https://arxiv.org/abs/1911.11929) and [map_fps](https://user-images.githubusercontent.com/4096485/71702416-6645dc00-2de0-11ea-8d65-de7d4b604021.png) comparison: https://github.com/WongKinYiu/CrossStagePartialNetworks
* Yolo v3 on MS COCO: [Speed / Accuracy (mAP@0.5) chart](https://user-images.githubusercontent.com/4096485/52151356-e5d4a380-2683-11e9-9d7d-ac7bc192c477.jpg)
* Yolo v3 on MS COCO (Yolo v3 vs RetinaNet) - Figure 3: https://arxiv.org/pdf/1804.02767v1.pdf
* Yolo v2 on Pascal VOC 2007: https://hsto.org/files/a24/21e/068/a2421e0689fb43f08584de9d44c2215f.jpg
* Yolo v2 on Pascal VOC 2012 (comp4): https://hsto.org/files/3a6/fdf/b53/3a6fdfb533f34cee9b52bdd9bb0b19d9.jpg
#### Youtube video of results
[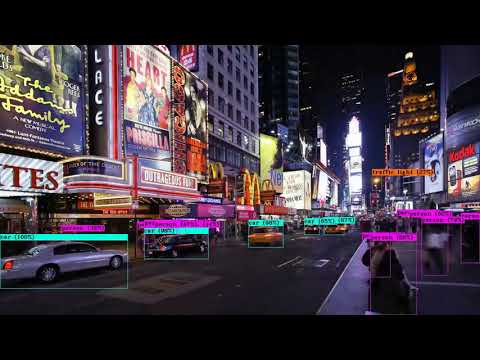](https://youtu.be/1_SiUOYUoOI "Yolo v4")
Others: https://www.youtube.com/user/pjreddie/videos
#### How to evaluate AP of YOLOv4 on the MS COCO evaluation server
1. Download and unzip test-dev2017 dataset from MS COCO server: http://images.cocodataset.org/zips/test2017.zip
2. Download list of images for Detection taks and replace the paths with yours: https://raw.githubusercontent.com/AlexeyAB/darknet/master/scripts/testdev2017.txt
3. Download `yolov4.weights` file: https://drive.google.com/open?id=1cewMfusmPjYWbrnuJRuKhPMwRe_b9PaT
4. Content of the file `cfg/coco.data` should be
```
classes= 80
train = <replace with your path>/trainvalno5k.txt
valid = <replace with your path>/testdev2017.txt
names = data/coco.names
backup = backup
eval=coco
```
5. Create `/results/` folder near with `./darknet` executable file
6. Run validation: `./darknet detector valid cfg/coco.data cfg/yolov4.cfg yolov4.weights`
7. Rename the file `/results/coco_results.json` to `detections_test-dev2017_yolov4_results.json` and compress it to `detections_test-dev2017_yolov4_results.zip`
8. Submit file `detections_test-dev2017_yolov4_results.zip` to the MS COCO evaluation server for the `test-dev2019 (bbox)`
#### How to evaluate FPS of YOLOv4 on GPU
1. Compile Darknet with `GPU=1 CUDNN=1 CUDNN_HALF=1 OPENCV=1` in the `Makefile` (or use the same settings with Cmake)
2. Download `yolov4.weights` file 245 MB: [yolov4.weights](https://github.com/AlexeyAB/darknet/releases/download/darknet_yolo_v3_optimal/yolov4.weights) (Google-drive mirror [yolov4.weights](https://drive.google.com/open?id=1cewMfusmPjYWbrnuJRuKhPMwRe_b9PaT) )
3. Get any .avi/.mp4 video file (preferably not more than 1920x1080 to avoid bottlenecks in CPU performance)
4. Run one of two commands and look at the AVG FPS:
* include video_capturing + NMS + drawing_bboxes:
`./darknet detector demo cfg/coco.data
没有合适的资源?快使用搜索试试~ 我知道了~
YOLO4源码,学习算法结构和开发结构c-plus-plus-study-master.zip
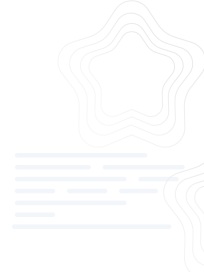
共2000个文件
png:1520个
cfg:156个
c:70个

需积分: 5 0 下载量 161 浏览量
2024-06-28
07:57:39
上传
评论
收藏 7.88MB ZIP 举报
温馨提示
YOLO4源码,学习算法结构和开发结构c_plus_plus-study-master.zip
资源推荐
资源详情
资源评论
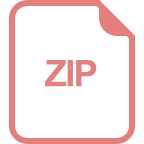
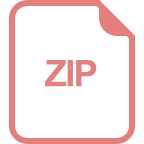
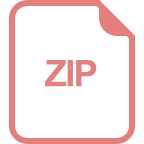
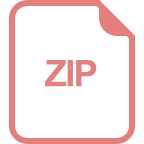
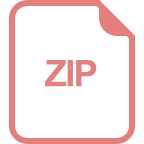
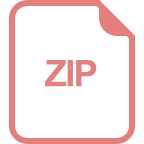
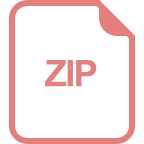
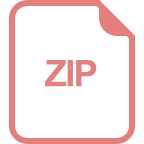
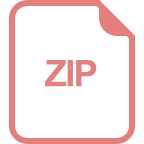
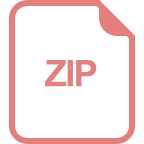
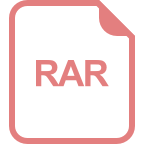
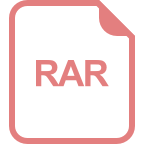
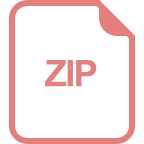
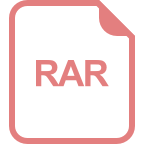
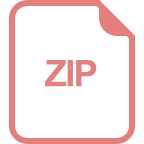
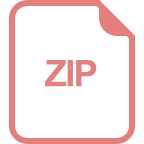
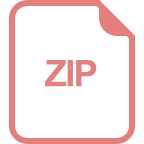
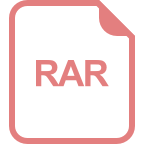
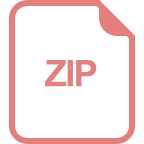
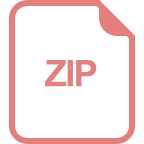
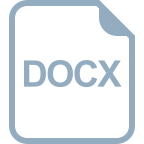
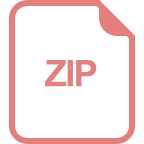
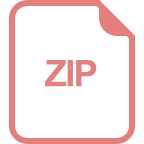
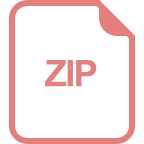
收起资源包目录

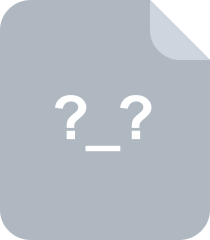
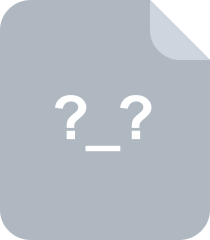
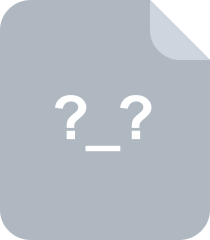
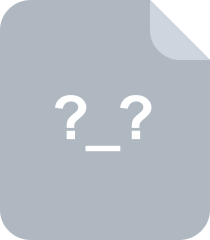
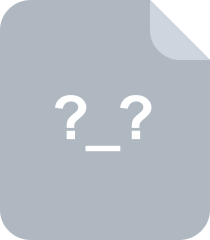
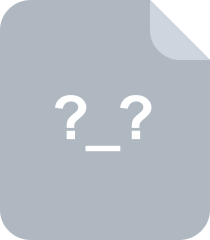
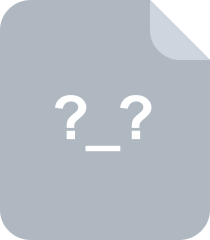
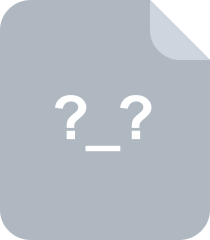
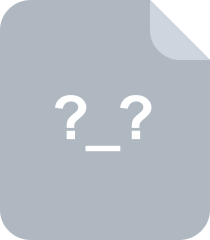
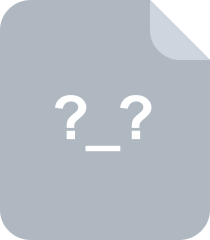
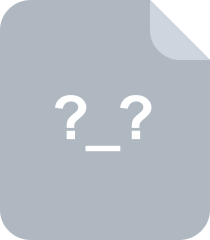
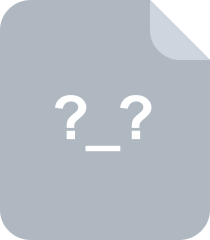
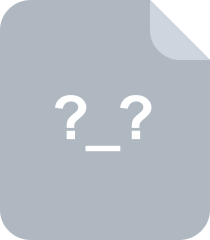
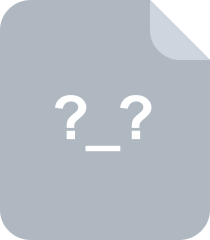
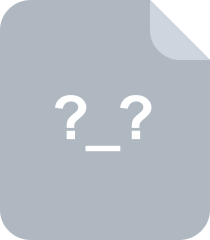
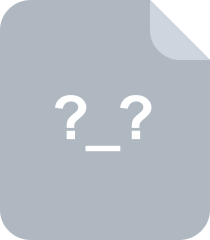
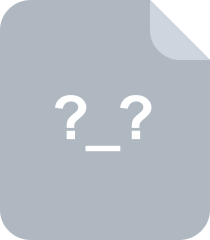
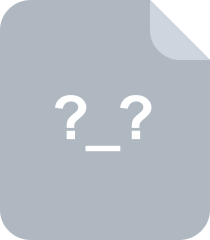
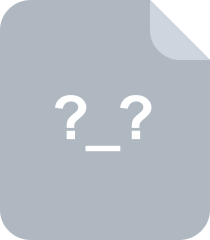
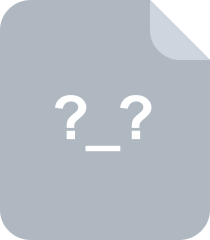
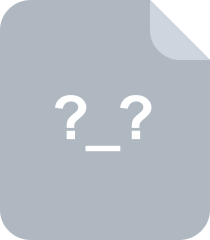
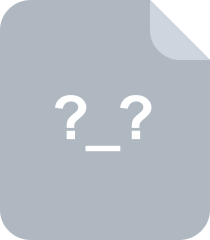
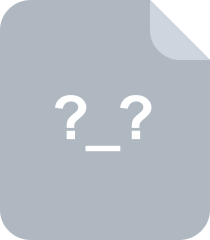
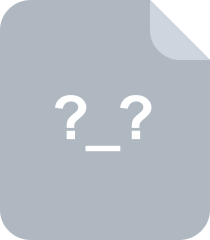
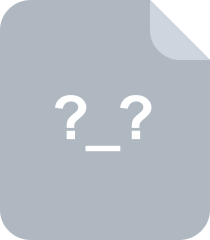
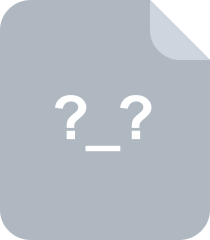
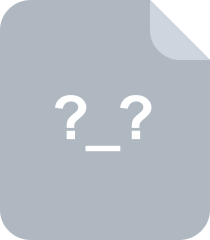
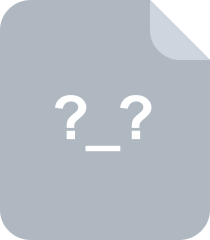
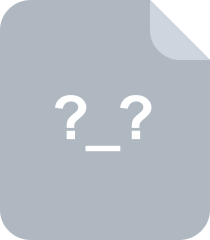
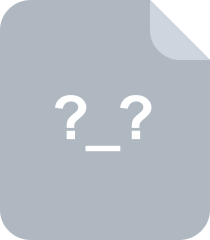
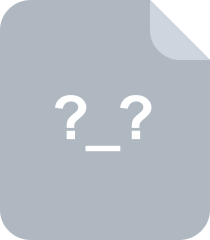
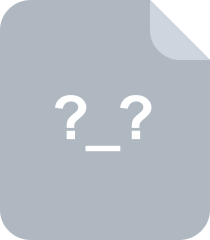
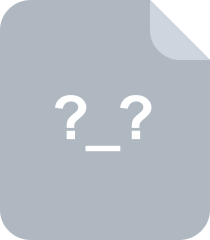
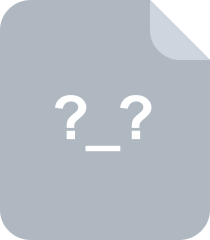
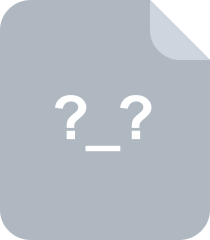
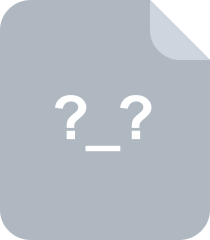
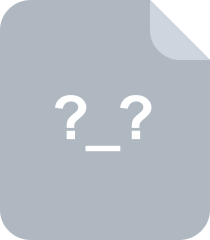
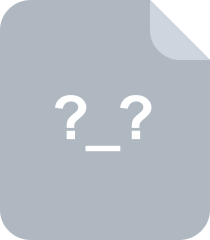
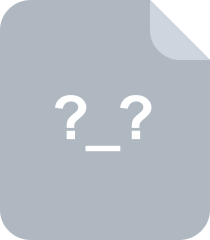
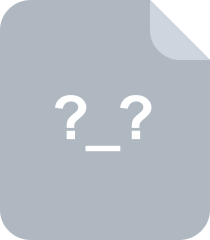
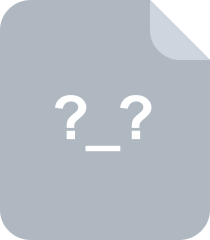
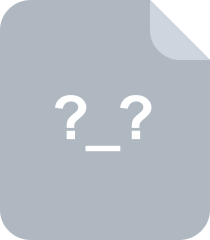
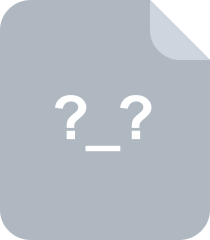
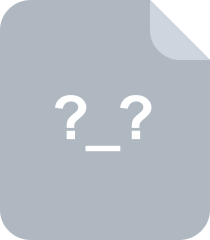
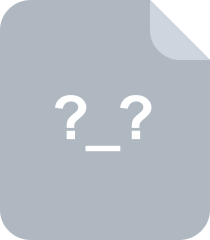
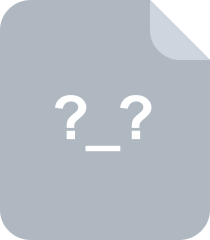
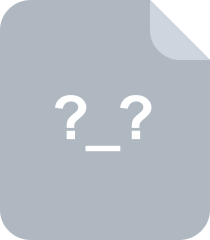
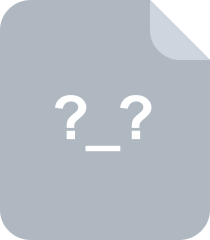
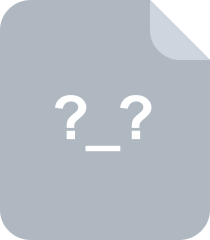
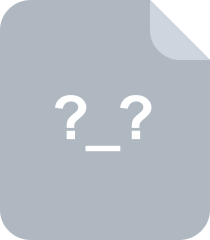
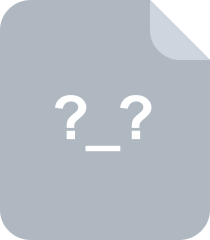
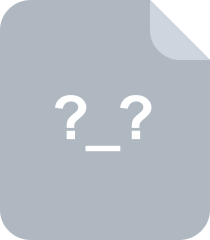
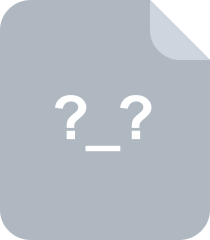
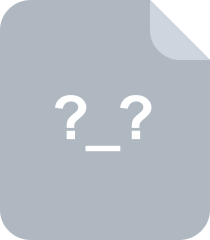
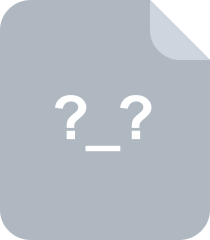
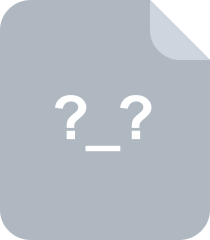
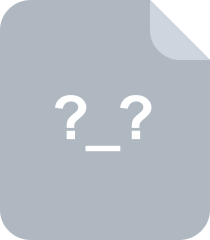
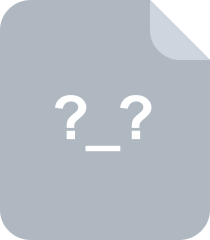
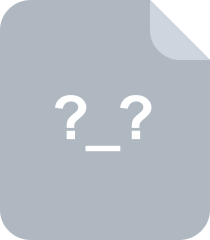
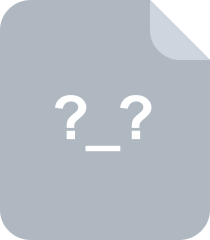
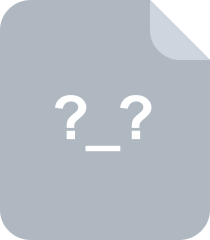
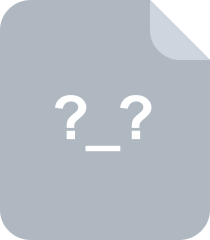
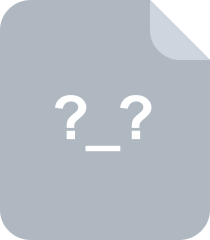
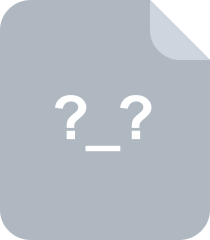
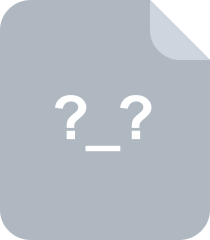
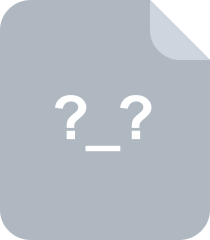
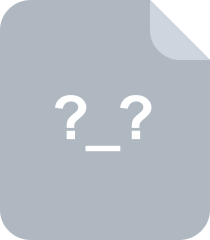
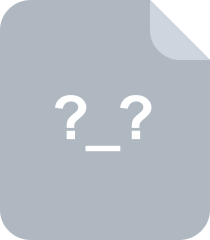
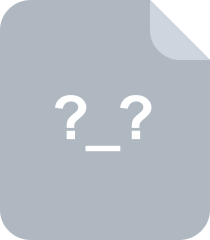
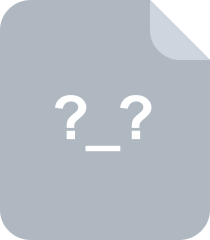
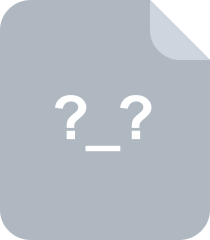
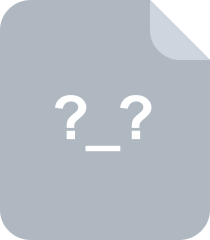
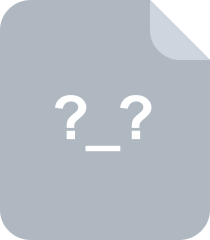
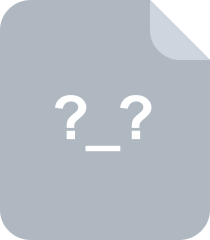
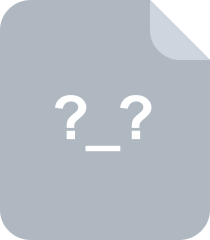
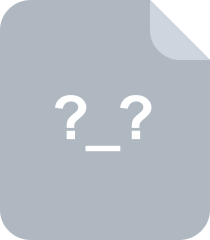
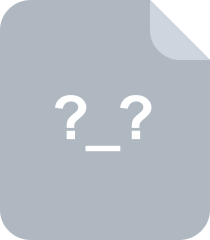
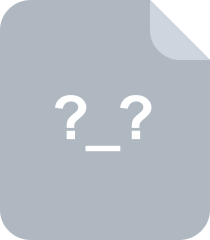
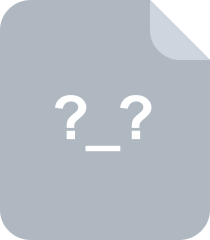
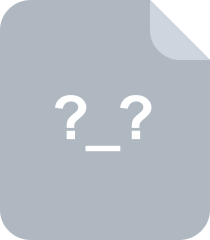
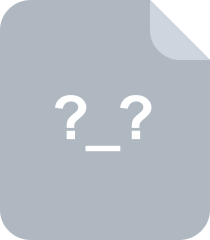
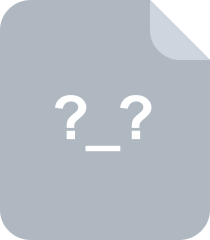
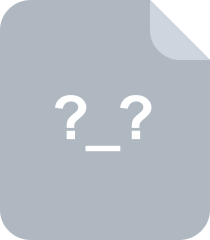
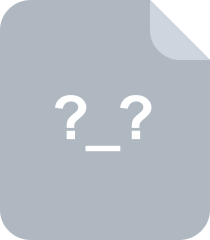
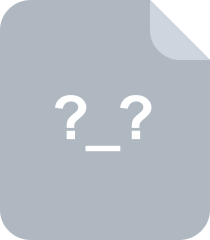
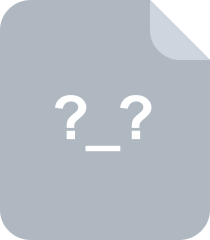
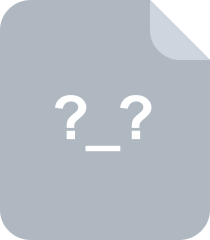
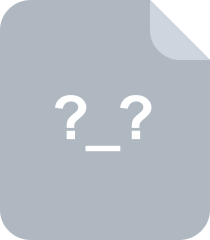
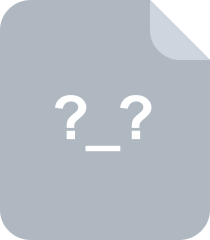
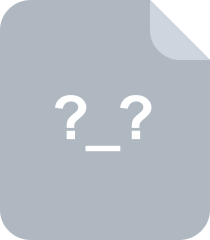
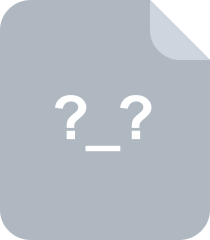
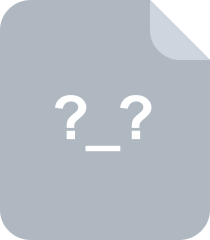
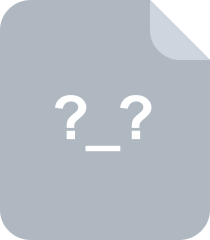
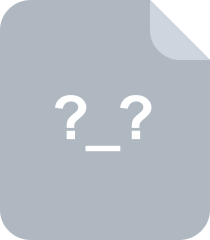
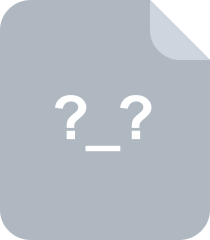
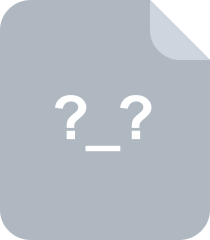
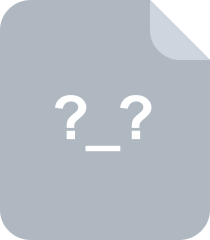
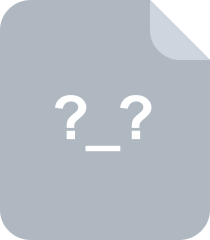
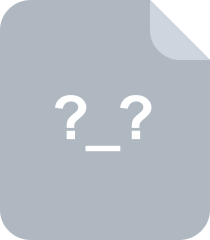
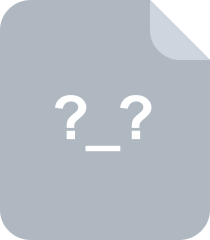
共 2000 条
- 1
- 2
- 3
- 4
- 5
- 6
- 20
资源评论
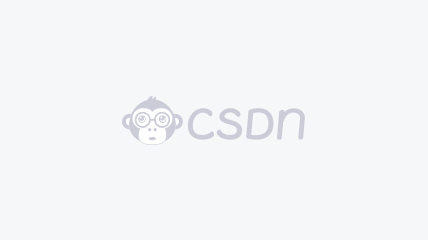

蜡笔小流
- 粉丝: 1618
- 资源: 955
上传资源 快速赚钱
我的内容管理 展开
我的资源 快来上传第一个资源
我的收益
登录查看自己的收益我的积分 登录查看自己的积分
我的C币 登录后查看C币余额
我的收藏
我的下载
下载帮助

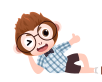
最新资源
- 光伏储能+三相并离网逆变切换运行模型含笔记 包含Boost、Buck-boost双向DCDC、并网逆变器控制、离网逆变器控制
- 车辆轨迹自适应预瞄跟踪控制和自适应p反馈联合控制,自适应预苗模型和基于模糊p控制均在simulink中搭建 个人觉得跟踪效果相
- HarmonyOs 应用基础-ArkTS-核心-基础 -抽奖案例代码
- SVPWM+死区补偿(基于电流极性)+高频注入法辨识PMSM的dq轴电感(离线辨识)-simulink
- testtest0001
- bollqbollqbollqbollqbollqbollqbollqbollqbollq
- jiguo.zipkjdfkjads
- anno_overview.zip
- 光伏发电三相并网 光伏加+Boost+三相并网逆变器 PLL锁相环 MPPT最大功率点跟踪控制(扰动观察法) dq解耦控制, 电
- 储能选址定容,33节点,matpower潮流计算,计算目标函数 考虑储能SOC、储能额定容量、功率约束 NSGA2多目标:储
资源上传下载、课程学习等过程中有任何疑问或建议,欢迎提出宝贵意见哦~我们会及时处理!
点击此处反馈


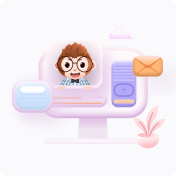
安全验证
文档复制为VIP权益,开通VIP直接复制
