## Prepare the PANDA-IMAGE dataset
First step is reorganize the dataset into COCO format. Please refer to `Task1_utils.py`.
## Prepare config files of MMDetection
The second step is to prepare config files thus the dataset could be successfully loaded. Assume that we want to use Faster R-CNN, the config to train the detector on PANDA IMAGE dataset is as below.
The **first config** need to be overwritten is the file `configs/_base_/datasets/coco_detection.py`, the config is as below.
We train only one category of box during one training process.
- Change the **CATE_ID** in the above config to select target category for training
- Change the **data_root** and the **anno_root** to your training data path
```python
CATE_ID = '1'
classes_dict = {'1': 'visible body', '2': 'full body', '3': 'head', '4': 'vehicle'}
json_pre_dict = {'1': 'person_visible', '2': 'person_full', '3': 'person_head', '4':'vehicle'}
data_root = 'YOUR/PATH/split_' + json_pre_dict[CATE_ID].split('_')[0] +'_train/'
anno_root = 'YOUR/PATH/coco_format_json/'
classes = (classes_dict[CATE_ID],)
json_pre = json_pre_dict[CATE_ID]
dataset_type = 'CocoDataset'
img_norm_cfg = dict(
mean=[123.675, 116.28, 103.53], std=[58.395, 57.12, 57.375], to_rgb=True)
train_pipeline = [
dict(type='LoadImageFromFile'),
dict(type='LoadAnnotations', with_bbox=True),
dict(type='Resize', img_scale=(1333, 800), keep_ratio=True),
dict(type='RandomFlip', flip_ratio=0.5),
dict(type='Normalize', **img_norm_cfg),
dict(type='Pad', size_divisor=32),
dict(type='DefaultFormatBundle'),
dict(type='Collect', keys=['img', 'gt_bboxes', 'gt_labels']),
]
test_pipeline = [
dict(type='LoadImageFromFile'),
dict(
type='MultiScaleFlipAug',
img_scale=(1333, 800),
flip=False,
transforms=[
dict(type='Resize', keep_ratio=True),
dict(type='RandomFlip'),
dict(type='Normalize', **img_norm_cfg),
dict(type='Pad', size_divisor=32),
dict(type='ImageToTensor', keys=['img']),
dict(type='Collect', keys=['img']),
])
]
data = dict(
samples_per_gpu=2,
workers_per_gpu=16,
train=dict(
type=dataset_type,
classes=classes,
ann_file=anno_root + json_pre + '_train.json',
img_prefix=data_root + 'image_train',
pipeline=train_pipeline),
val=dict(
type=dataset_type,
classes=classes,
ann_file=anno_root + json_pre + '_val.json',
img_prefix=data_root + 'image_train',
pipeline=test_pipeline),
test=dict(
type=dataset_type,
classes=classes,
ann_file=anno_root + json_pre + '_val.json',
img_prefix=data_root + 'image_train',
pipeline=test_pipeline))
evaluation = dict(interval=1, metric='bbox')
```
The **second config** need to be overwritten is the file `configs/_base_/models/faster_rcnn_r50_fpn.py`, the config is as below.
```python
model = dict(
type='FasterRCNN',
pretrained='torchvision://resnet50',
backbone=dict(
type='ResNet',
depth=50,
num_stages=4,
out_indices=(0, 1, 2, 3),
frozen_stages=1,
norm_cfg=dict(type='BN', requires_grad=True),
norm_eval=True,
style='pytorch'),
neck=dict(
type='FPN',
in_channels=[256, 512, 1024, 2048],
out_channels=256,
num_outs=5),
rpn_head=dict(
type='RPNHead',
in_channels=256,
feat_channels=256,
anchor_generator=dict(
type='AnchorGenerator',
scales=[8],
ratios=[0.5, 1.0, 2.0],
strides=[4, 8, 16, 32, 64]),
bbox_coder=dict(
type='DeltaXYWHBBoxCoder',
target_means=[.0, .0, .0, .0],
target_stds=[1.0, 1.0, 1.0, 1.0]),
loss_cls=dict(
type='CrossEntropyLoss', use_sigmoid=True, loss_weight=1.0),
loss_bbox=dict(type='L1Loss', loss_weight=1.0)),
roi_head=dict(
type='StandardRoIHead',
bbox_roi_extractor=dict(
type='SingleRoIExtractor',
roi_layer=dict(type='RoIAlign', output_size=7, sampling_ratio=0),
out_channels=256,
featmap_strides=[4, 8, 16, 32]),
bbox_head=dict(
type='Shared2FCBBoxHead',
in_channels=256,
fc_out_channels=1024,
roi_feat_size=7,
num_classes=1,
bbox_coder=dict(
type='DeltaXYWHBBoxCoder',
target_means=[0., 0., 0., 0.],
target_stds=[0.1, 0.1, 0.2, 0.2]),
reg_class_agnostic=False,
loss_cls=dict(
type='CrossEntropyLoss', use_sigmoid=False, loss_weight=1.0),
loss_bbox=dict(type='L1Loss', loss_weight=1.0))),
# model training and testing settings
train_cfg=dict(
rpn=dict(
assigner=dict(
type='MaxIoUAssigner',
pos_iou_thr=0.7,
neg_iou_thr=0.3,
min_pos_iou=0.3,
match_low_quality=True,
ignore_iof_thr=-1),
sampler=dict(
type='RandomSampler',
num=256,
pos_fraction=0.5,
neg_pos_ub=-1,
add_gt_as_proposals=False),
allowed_border=-1,
pos_weight=-1,
debug=False),
rpn_proposal=dict(
nms_across_levels=False,
nms_pre=2000,
nms_post=1000,
max_num=1000,
nms_thr=0.7,
min_bbox_size=0),
rcnn=dict(
assigner=dict(
type='MaxIoUAssigner',
pos_iou_thr=0.5,
neg_iou_thr=0.5,
min_pos_iou=0.5,
match_low_quality=False,
ignore_iof_thr=-1),
sampler=dict(
type='RandomSampler',
num=512,
pos_fraction=0.25,
neg_pos_ub=-1,
add_gt_as_proposals=True),
pos_weight=-1,
debug=False)),
test_cfg=dict(
rpn=dict(
nms_across_levels=False,
nms_pre=1000,
nms_post=1000,
max_num=1000,
nms_thr=0.7,
min_bbox_size=0),
rcnn=dict(
score_thr=0.05,
nms=dict(type='nms', iou_threshold=0.5),
max_per_img=100)
# soft-nms is also supported for rcnn testing
# e.g., nms=dict(type='soft_nms', iou_threshold=0.5, min_score=0.05)
))
```
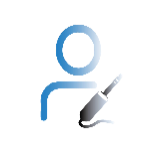
好家伙VCC
- 粉丝: 2827
- 资源: 9136
最新资源
- 【毕业设计】基于SpringBoot+Vue的图书管理系统【源码+论文+答辩ppt+开题报告+任务书】.zip
- 【毕业设计】基于springboot+sureness的面向REST API资源无状态认证权限管理系统【源码+论文+答辩ppt+开题报告+任务书】.zip
- 【毕业设计】基于springboot+mybatis学生管理系统【源码+论文+答辩ppt+开题报告+任务书】.zip
- 【毕业设计】基于SpringBoot的物业管理系统【源码+论文+答辩ppt+开题报告+任务书】.zip
- 【毕业设计】基于springboot的后台管理系统基本框架【源码+论文+答辩ppt+开题报告+任务书】.zip
- 【毕业设计】Python的Django-html企业物流管理系统源码(完整前后端+mysql+说明文档+LW+PPT).zip
- 【毕业设计】Python的Django-html某医院体检挂号系统源码(完整前后端+mysql+说明文档+LW+PPT).zip
- 基于一致性算法的直流微电网均流均压二级控制策略,涵盖分布式电源与非线性负载,实现电压电流恢复,仿真验证优秀性能与鲁棒性 顶刊复现,有意者加好友,粉丝价优惠 ,基于一致性算法的直流微电网均流均压二级控制
- 【毕业设计】基于SpringBoot的人事管理系统【源码+论文+答辩ppt+开题报告+任务书】.zip
- 【毕业设计】基于SpringBoot开发的酒店管理系统【源码+论文+答辩ppt+开题报告+任务书】.zip
- 【毕业设计】基于vue + springboot的学生成绩管理系统【源码+论文+答辩ppt+开题报告+任务书】.zip
- 【毕业设计】Python的Django-html人脸表情的分类算法的设计源码(完整前后端+mysql+说明文档+LW+PPT).zip
- 【毕业设计】基于vue+springboot的图书馆管理系统【源码+论文+答辩ppt+开题报告+任务书】.zip
- 【毕业设计】酒店管理系统(vue+springboot)【源码+论文+答辩ppt+开题报告+任务书】.zip
- 【毕业设计】图书管理系统(SpringBoot,thymeleaf) (2018, Archived)【源码+论文+答辩ppt+开题报告+任务书】.zip
- 【毕业设计】微人事是一个前后端分离的人力资源管理系统,项目采用SpringBoot+Vue开发。【源码+论文+答辩ppt+开题报告+任务书】.zip
资源上传下载、课程学习等过程中有任何疑问或建议,欢迎提出宝贵意见哦~我们会及时处理!
点击此处反馈


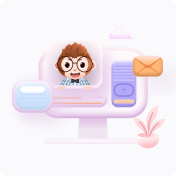