function [bestX,Convergence_curve,result]=ssaforlstm(P_train,T_train,P_test,T_test)
%% 参数设置
pop=5; % 种群数
M=5; % 最大迭代次数
dim=4;%一共有4个参数需要优化
lb=[1 1 1 0.001];%分别对两个lstm隐含层节点 训练次数与学习率寻优
ub=[100 100 50 0.01];%这个分别代表4个参数的上下界,比如第一个参数的范围就是1-100
P_percent = 0.2; %producers 在全部种群的占比
pNum = round( pop * P_percent ); % producers的数量
%初始化种群
for i = 1 : pop
for j=1:dim
if j==4%除了学习率 其他的都是整数
x( i, j ) = (ub(j)-lb(j))*rand+lb(j);
else
x( i, j ) = round((ub(j)-lb(j))*rand+lb(j));
end
end
fit( i )=fitness(x(i,:),P_train,T_train,P_test,T_test);
end
pFit = fit;
pX = x;
fMin=fit(1);
bestX = x( i, : );
for t = 1 : M
[ ~, sortIndex ] = sort( pFit );% Sort.从小到大
[fmax,B]=max( pFit );
worse= x(B,:);
r2=rand(1);
%%%%%%%%%%%%%5%%%%%%这一部位为发现者(探索者)的位置更新%%%%%%%%%%%%%%%%%%%%%%%%%
if(r2<0.8)%预警值较小,说明没有捕食者出现
for i = 1 : pNum %r2小于0.8的发现者的改变(1-20) % Equation (3)
r1=rand(1);
x( sortIndex( i ), : ) = pX( sortIndex( i ), : )*exp(-(i)/(r1*M));%对自变量做一个随机变换
x( sortIndex( i ), : ) = Bounds( x( sortIndex( i ), : ), lb, ub );%对超过边界的变量进行去除
fit( sortIndex( i ) )=fitness(x(sortIndex( i ),:),P_train,T_train,P_test,T_test);
end
else %预警值较大,说明有捕食者出现威胁到了种群的安全,需要去其它地方觅食
for i = 1 : pNum %r2大于0.8的发现者的改变
x( sortIndex( i ), : ) = pX( sortIndex( i ), : )+randn(1)*ones(1,dim);
x( sortIndex( i ), : ) = Bounds( x( sortIndex( i ), : ), lb, ub );
fit( sortIndex( i ) )=fitness(x(sortIndex( i ),:),P_train,T_train,P_test,T_test);
end
end
[ ~, bestII ] = min( fit );
bestXX = x( bestII, : );
%%%%%%%%%%%%%5%%%%%%这一部位为加入者(追随者)的位置更新%%%%%%%%%%%%%%%%%%%%%%%%%
for i = ( pNum + 1 ) : pop %剩下的个体的变换 % Equation (4)
% i
% sortIndex( i )
A=floor(rand(1,dim)*2)*2-1;
if( i>(pop/2))%这个代表这部分麻雀处于十分饥饿的状态(因为它们的能量很低,也是是适应度值很差),需要到其它地方觅食
x( sortIndex(i ), : )=randn(1,dim).*exp((worse-pX( sortIndex( i ), : ))/(i)^2);
else%这一部分追随者是围绕最好的发现者周围进行觅食,其间也有可能发生食物的争夺,使其自己变成生产者
x( sortIndex( i ), : )=bestXX+(abs(( pX( sortIndex( i ), : )-bestXX)))*(A'*(A*A')^(-1))*ones(1,dim);
end
x( sortIndex( i ), : ) = Bounds( x( sortIndex( i ), : ), lb, ub );%判断边界是否超出
fit( sortIndex( i ) )=fitness(x(sortIndex( i ),:),P_train,T_train,P_test,T_test);
end
%%%%%%%%%%%%%5%%%%%%这一部位为意识到危险(注意这里只是意识到了危险,不代表出现了真正的捕食者)的麻雀的位置更新%%%%%%%%%%%%%%%%%%%%%%%%%
c=randperm(numel(sortIndex));%%%%%%%%%这个的作用是在种群中随机产生其位置(也就是这部分的麻雀位置一开始是随机的,意识到危险了要进行位置移动,
%处于种群外围的麻雀向安全区域靠拢,处在种群中心的麻雀则随机行走以靠近别的麻雀)
b=sortIndex(c(1:3));
for j = 1 : length(b) % Equation (5)
if( pFit( sortIndex( b(j) ) )>(fMin) ) %处于种群外围的麻雀的位置改变
x( sortIndex( b(j) ), : )=bestX+(randn(1,dim)).*(abs(( pX( sortIndex( b(j) ), : ) -bestX)));
else
%处于种群中心的麻雀的位置改变
x( sortIndex( b(j) ), : ) =pX( sortIndex( b(j) ), : )+(2*rand(1)-1)*(abs(pX( sortIndex( b(j) ), : )-worse))/ ( pFit( sortIndex( b(j) ) )-fmax+1e-50);
end
x( sortIndex(b(j) ), : ) = Bounds( x( sortIndex(b(j) ), : ), lb, ub );
fit( sortIndex( b(j) ) )=fitness(x(sortIndex( b(j) ),:),P_train,T_train,P_test,T_test);
end
for i = 1 : pop
if ( fit( i ) < pFit( i ) )
pFit( i ) = fit( i );
pX( i, : ) = x( i, : );
end
if( pFit( i ) < fMin )
fMin= pFit( i );
bestX = pX( i, : );
end
end
Convergence_curve(t)=fMin;
result(t,:)=bestX;
end

智能算法及其模型预测
- 粉丝: 2526
- 资源: 871
最新资源
- 带载流子密度的双温模型matlab,电子晶格温度,电子密度,飞秒激光源模拟,有限元法解偏微分方程 德鲁德模型,带载流子密度变化
- GP026-仓库系统.zip
- HttpCanary_3.3.6.apk
- 线控制动系统仿真 Carsim和Simulink联合仿真线控制动系统BBW-EMB系统 包含简单的制动力分配和四个车轮的线控制动机构 四个车轮独立BLDCM三环PID闭环制动控制,最大真实还原线
- Comsol脉冲涡流无损检测仿真 图一:脉冲涡流仿真,检出电压信号 图二:脉冲涡流模型 图三:磁通密度模 图四:磁通密度模
- CC2530无线zigbee裸机代码实现光敏和热敏传感器数值读取.zip
- CC2530无线zigbee裸机代码实现继电器的控制.zip
- CC2530无线zigbee裸机代码实现看门口狗Watch Dog使用.zip
- CC2530无线zigbee裸机代码实现控制步进电机正反转.zip
- CC2530无线zigbee裸机代码实现人体红外传感器数值读取.zip
- CC2530无线zigbee裸机代码实现睡眠定时器唤醒系统.zip
- CC2530无线zigbee裸机代码实现外部中断控制LED开关.zip
- CC2530无线zigbee裸机代码实现外部中断控制流水灯.zip
- 基于51单片机的污水处理厂气体检测报警系统(protues仿真)-毕业设计
- CC2530无线zigbee裸机代码实现温度传感器DS18B20数值读取.zip
- CC2530无线zigbee裸机代码实现温湿度传感器DHT11数值读取.zip
资源上传下载、课程学习等过程中有任何疑问或建议,欢迎提出宝贵意见哦~我们会及时处理!
点击此处反馈


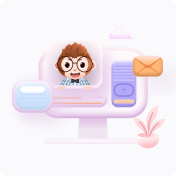