# -*- coding: utf-8 -*-
"""road_extraction.ipynb
Automatically generated by Colaboratory.
Original file is located at
https://colab.research.google.com/drive/1xmOO0tyPQDOEQ2zAFfk30hjND-moVqeR
## Setup and Dependencies
First, please make sure you are using a GPU runtime to run this notebook, so inference is much faster. If the following command fails, use the `Runtime` menu above and select `Change runtime type`.
"""
!nvidia-smi
!pip install opendatasets==0.1.22
!pip install diffusers==0.9.0
!pip install transformers==4.25.1
!pip install scipy==1.7.3
!pip install ftfy==6.1.1
!pip install "ipywidgets>=7,<8"
!pip install accelerate==0.15.0
import os
import sys
import numpy as np
import re
import cv2
import matplotlib.pyplot as plt
import tensorflow as tf
import torch
from diffusers import StableDiffusionInpaintPipeline
import PIL
from huggingface_hub import notebook_login
from google.colab import output
output.enable_custom_widget_manager()
INPUT_SHAPE = 128
"""## Login to HuggingFace
To use download the Stable Diffusion model, you need to sign in to https://huggingface.co/ then go to https://huggingface.co/settings/tokens and create
a token. Paste the token below:
"""
notebook_login()
"""## Download dataset
The dataset we'll be using for this notebook can be downloaded from Kaggle.com.
Sign into your Kaggle account and then go to http://bit.ly/kaggle-creds to create an API token. Then paste your username and API token below:
"""
import opendatasets as od
od.download("https://www.kaggle.com/datasets/balraj98/deepglobe-road-extraction-dataset", data_dir="./input")
def load_data(path, shape):
file_names = os.listdir(path)
file_names.sort()
if len(file_names) == 0:
raise ValueError("No files found at path: " + path)
frame_obj = {
'img': [],
'mask': []
}
for i, name in enumerate(file_names):
if not name.endswith('_sat.jpg'):
continue
base_name = name.split('_')[0]
img_path = path + '/' + name
mask_path = path + '/' + base_name + '_mask.png'
img = plt.imread(img_path)
mask = plt.imread(mask_path)
img = cv2.resize(img, (shape, shape))
mask = cv2.resize(mask, (shape, shape))
frame_obj['img'].append(img)
# For mask images, only retain channel 0 which represents the mask.
# Other channels are irrelevant.
# TODO(albrow): binarize mask with threshold of 128 as recommended on Kaggle?
frame_obj['mask'].append(mask[:,:,0])
return frame_obj
all_data = load_data('./input/deepglobe-road-extraction-dataset/train', shape=INPUT_SHAPE)
len(all_data['img'])
# Display sample images (helps make sure everything was loaded correctly)
plt.subplot(1,2,1)
plt.imshow(all_data['img'][0])
plt.subplot(1,2,2)
plt.imshow(all_data['mask'][0])
plt.show()
# Approximately 70/15/15 train/val/test split.
train_data = {'img': np.array(all_data['img'][0:4358]), 'mask': np.array(all_data['mask'][0:4358]) }
val_data = {'img': np.array(all_data['img'][4358:5292]), 'mask': np.array(all_data['mask'][4358:5292]) }
test_data = {'img': np.array(all_data['img'][5292:]), 'mask': np.array(all_data['mask'][5292:]) }
"""## Configure our U-Net model for image segmentation
We'll use a fairly standard U-Net model for image segmentation. As a reminder,
the model's task is to identify the parts of the image that should be considered
"roads".
"""
# Define Conv2d block for U-Net
# This block essentially performs 2 convolution
def conv_2d_block(input_tensor, num_filters, kernel_size = 3, do_batch_norm = True):
# First conv
x = tf.keras.layers.Conv2D(filters = num_filters, kernel_size = (kernel_size, kernel_size),
kernel_initializer = 'he_normal', padding = 'same') (input_tensor)
if do_batch_norm:
x = tf.keras.layers.BatchNormalization()(x)
x =tf.keras.layers.Activation('relu')(x)
# Second conv
x = tf.keras.layers.Conv2D(filters = num_filters, kernel_size = (kernel_size, kernel_size),
kernel_initializer = 'he_normal', padding = 'same') (x)
if do_batch_norm:
x = tf.keras.layers.BatchNormalization()(x)
x = tf.keras.layers.Activation('relu')(x)
return x
# Define U-Net
def create_unet(input_image, num_filters = 16, droupouts = 0.1, do_batch_norm = True):
# defining encoder Path
c1 = conv_2d_block(input_image, num_filters * 1, kernel_size = 3, do_batch_norm = do_batch_norm)
p1 = tf.keras.layers.MaxPooling2D((2,2))(c1)
p1 = tf.keras.layers.Dropout(droupouts)(p1)
c2 = conv_2d_block(p1, num_filters * 2, kernel_size = 3, do_batch_norm = do_batch_norm)
p2 = tf.keras.layers.MaxPooling2D((2,2))(c2)
p2 = tf.keras.layers.Dropout(droupouts)(p2)
c3 = conv_2d_block(p2, num_filters * 4, kernel_size = 3, do_batch_norm = do_batch_norm)
p3 = tf.keras.layers.MaxPooling2D((2,2))(c3)
p3 = tf.keras.layers.Dropout(droupouts)(p3)
c4 = conv_2d_block(p3, num_filters * 8, kernel_size = 3, do_batch_norm = do_batch_norm)
p4 = tf.keras.layers.MaxPooling2D((2,2))(c4)
p4 = tf.keras.layers.Dropout(droupouts)(p4)
c5 = conv_2d_block(p4, num_filters * 16, kernel_size = 3, do_batch_norm = do_batch_norm)
# defining decoder path
u6 = tf.keras.layers.Conv2DTranspose(num_filters*8, (3, 3), strides = (2, 2), padding = 'same')(c5)
u6 = tf.keras.layers.concatenate([u6, c4])
u6 = tf.keras.layers.Dropout(droupouts)(u6)
c6 = conv_2d_block(u6, num_filters * 8, kernel_size = 3, do_batch_norm = do_batch_norm)
u7 = tf.keras.layers.Conv2DTranspose(num_filters*4, (3, 3), strides = (2, 2), padding = 'same')(c6)
u7 = tf.keras.layers.concatenate([u7, c3])
u7 = tf.keras.layers.Dropout(droupouts)(u7)
c7 = conv_2d_block(u7, num_filters * 4, kernel_size = 3, do_batch_norm = do_batch_norm)
u8 = tf.keras.layers.Conv2DTranspose(num_filters*2, (3, 3), strides = (2, 2), padding = 'same')(c7)
u8 = tf.keras.layers.concatenate([u8, c2])
u8 = tf.keras.layers.Dropout(droupouts)(u8)
c8 = conv_2d_block(u8, num_filters * 2, kernel_size = 3, do_batch_norm = do_batch_norm)
u9 = tf.keras.layers.Conv2DTranspose(num_filters*1, (3, 3), strides = (2, 2), padding = 'same')(c8)
u9 = tf.keras.layers.concatenate([u9, c1])
u9 = tf.keras.layers.Dropout(droupouts)(u9)
c9 = conv_2d_block(u9, num_filters * 1, kernel_size = 3, do_batch_norm = do_batch_norm)
output = tf.keras.layers.Conv2D(1, (1, 1), activation = 'sigmoid')(c9)
model = tf.keras.Model(inputs = [input_image], outputs = [output])
return model
"""## Baseline performance: training on all available samples
First, we'll train the model on the full training set of ~4,000 images.
"""
# Instantiate and compile model
tf.random.set_seed(42)
np.random.seed(42)
inputs = tf.keras.layers.Input((INPUT_SHAPE, INPUT_SHAPE, 3))
baseline_unet = create_unet(inputs)
baseline_unet.compile(optimizer='Adam', loss='binary_crossentropy', metrics=tf.keras.metrics.BinaryIoU(name="binary_iou"))
# Note: We're just using the EarlyStopping callback to restore best weights. Because we set the patience very high,
# training will never actually stop early.
restore_best_weights = tf.keras.callbacks.EarlyStopping(monitor='val_loss', patience=99999999, restore_best_weights=True)
results = baseline_unet.fit(train_data['img'], train_data['mask'], validation_data=(val_data['img'], val_data['mask']), epochs=50, verbose=1, callbacks=[restore_best_weights])
plt.figure(figsize=(16, 9))
plt.plot(results.history['loss'], label='loss')
plt.plot(results.history['val_loss'], label='val_loss')
plt.legend()
plt.grid(True)
baseline_unet.evaluate(test_data['img'], test_data['mask'])
def batch_predict(model, images, masks):
images = np.array(images)
masks = np.array(masks)
return model.predict(images)
def plot_pred(img, pred_mask, actual_mask):
没有合适的资源?快使用搜索试试~ 我知道了~
温馨提示
Stable Diffusion是 Stability AI 在今年早些时候发布的一种非常强大的文本到图像模型。在这篇博文中,我们将探索一种使用稳定扩散来增强训练数据的技术,以提高图像分割任务的性能。这种方法在数据有限或需要繁琐的人工标记的应用程序中特别强大。 在计算机视觉模型的上下文中,图像分割是指根据图像的内容将图像分成两个或多个部分。与“图像分类”相比,分割的目标不仅是识别图像包含什么,而且图像的哪些 部分对应于每个类。 Stable Diffusion是 Stability AI 在今年早些时候发布的一种非常强大的文本到图像模型。在这篇博文中,我们将探索一种使用稳定扩散来增强训练数据的技术,以提高图像分割任务的性能。这种方法在数据有限或需要繁琐的人工标记的应用程序中特别强大。
资源推荐
资源详情
资源评论
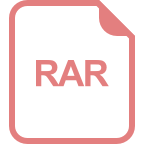
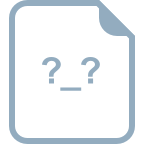
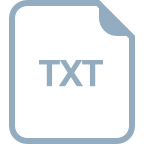
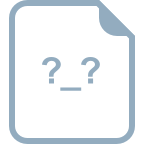
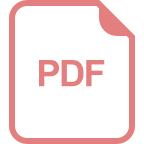
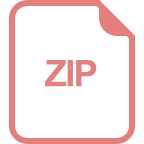
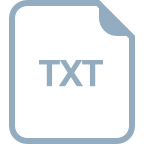
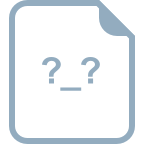
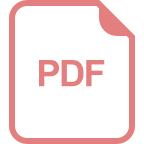
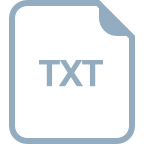
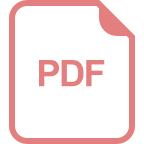
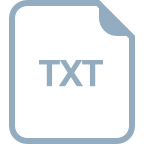
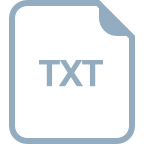
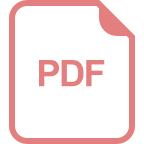
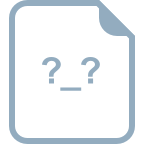
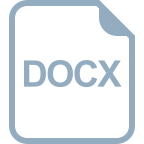
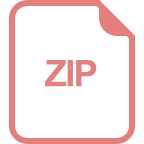
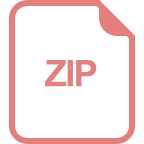
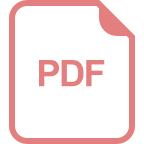
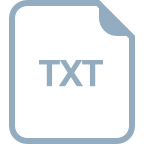
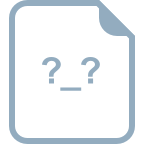
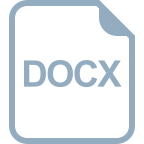
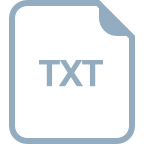
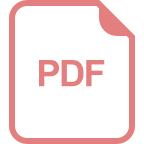
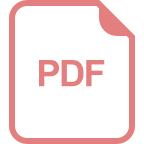
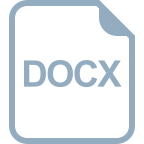
收起资源包目录


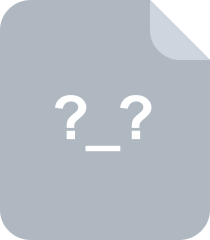
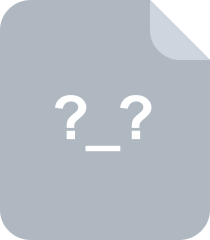
共 2 条
- 1
资源评论
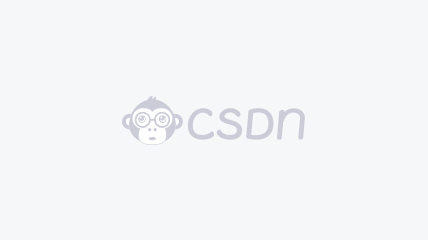


小风飞子
- 粉丝: 368
- 资源: 1962
上传资源 快速赚钱
我的内容管理 展开
我的资源 快来上传第一个资源
我的收益
登录查看自己的收益我的积分 登录查看自己的积分
我的C币 登录后查看C币余额
我的收藏
我的下载
下载帮助

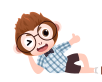
最新资源
- STM32参考资料文档应用文档深入解析STM32-USB-FS-Device-Lib库
- 公司本周销售数据.xlsx
- STM32参考资料文档应用文档利用ST提供的USB例程实现USB IAP功能
- 毕业设计《基于协同过滤算法SSM个性化推荐购物商城设计(带支付宝支付接口)》+JavaScript源码+文档说明
- STM32参考资料文档应用文档基于STM32的USB程序开发笔记
- STM32参考资料文档应用文档UM0427-基于ARM的32位MCU STM32F101xx 和 STM32F103xx固件库
- STM32参考资料文档应用文档UM0424-STM32F10xxx USB开发工具包
- STM32参考资料文档应用文档UM0306-STM32F10XXX使用手册
- STM32参考资料文档应用文档UIP协议的中文介绍
- STM32参考资料文档应用文档uip1.0
资源上传下载、课程学习等过程中有任何疑问或建议,欢迎提出宝贵意见哦~我们会及时处理!
点击此处反馈


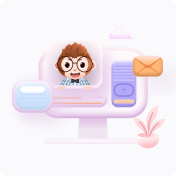
安全验证
文档复制为VIP权益,开通VIP直接复制
