<div align="center">
<p>
<a align="center" href="https://ultralytics.com/yolov5" target="_blank">
<img width="100%" src="https://raw.githubusercontent.com/ultralytics/assets/main/yolov5/v70/splash.png"></a>
</p>
[English](README.md) | [ç®ä½ä¸æ](README.zh-CN.md)
<br>
<div>
<a href="https://github.com/ultralytics/yolov5/actions/workflows/ci-testing.yml"><img src="https://github.com/ultralytics/yolov5/actions/workflows/ci-testing.yml/badge.svg" alt="YOLOv5 CI"></a>
<a href="https://zenodo.org/badge/latestdoi/264818686"><img src="https://zenodo.org/badge/264818686.svg" alt="YOLOv5 Citation"></a>
<a href="https://hub.docker.com/r/ultralytics/yolov5"><img src="https://img.shields.io/docker/pulls/ultralytics/yolov5?logo=docker" alt="Docker Pulls"></a>
<br>
<a href="https://bit.ly/yolov5-paperspace-notebook"><img src="https://assets.paperspace.io/img/gradient-badge.svg" alt="Run on Gradient"></a>
<a href="https://colab.research.google.com/github/ultralytics/yolov5/blob/master/tutorial.ipynb"><img src="https://colab.research.google.com/assets/colab-badge.svg" alt="Open In Colab"></a>
<a href="https://www.kaggle.com/ultralytics/yolov5"><img src="https://kaggle.com/static/images/open-in-kaggle.svg" alt="Open In Kaggle"></a>
</div>
<br>
YOLOv5 ð is the world's most loved vision AI, representing <a href="https://ultralytics.com">Ultralytics</a> open-source research into future vision AI methods, incorporating lessons learned and best practices evolved over thousands of hours of research and development.
To request an Enterprise License please complete the form at <a href="https://ultralytics.com/license">Ultralytics Licensing</a>.
<div align="center">
<a href="https://github.com/ultralytics" style="text-decoration:none;">
<img src="https://github.com/ultralytics/assets/raw/main/social/logo-social-github.png" width="2%" alt="" /></a>
<img src="https://github.com/ultralytics/assets/raw/main/social/logo-transparent.png" width="2%" alt="" />
<a href="https://www.linkedin.com/company/ultralytics" style="text-decoration:none;">
<img src="https://github.com/ultralytics/assets/raw/main/social/logo-social-linkedin.png" width="2%" alt="" /></a>
<img src="https://github.com/ultralytics/assets/raw/main/social/logo-transparent.png" width="2%" alt="" />
<a href="https://twitter.com/ultralytics" style="text-decoration:none;">
<img src="https://github.com/ultralytics/assets/raw/main/social/logo-social-twitter.png" width="2%" alt="" /></a>
<img src="https://github.com/ultralytics/assets/raw/main/social/logo-transparent.png" width="2%" alt="" />
<a href="https://www.producthunt.com/@glenn_jocher" style="text-decoration:none;">
<img src="https://github.com/ultralytics/assets/raw/main/social/logo-social-producthunt.png" width="2%" alt="" /></a>
<img src="https://github.com/ultralytics/assets/raw/main/social/logo-transparent.png" width="2%" alt="" />
<a href="https://youtube.com/ultralytics" style="text-decoration:none;">
<img src="https://github.com/ultralytics/assets/raw/main/social/logo-social-youtube.png" width="2%" alt="" /></a>
<img src="https://github.com/ultralytics/assets/raw/main/social/logo-transparent.png" width="2%" alt="" />
<a href="https://www.facebook.com/ultralytics" style="text-decoration:none;">
<img src="https://github.com/ultralytics/assets/raw/main/social/logo-social-facebook.png" width="2%" alt="" /></a>
<img src="https://github.com/ultralytics/assets/raw/main/social/logo-transparent.png" width="2%" alt="" />
<a href="https://www.instagram.com/ultralytics/" style="text-decoration:none;">
<img src="https://github.com/ultralytics/assets/raw/main/social/logo-social-instagram.png" width="2%" alt="" /></a>
</div>
</div>
<br>
## <div align="center">YOLOv8 ð NEW</div>
We are thrilled to announce the launch of Ultralytics YOLOv8 ð, our NEW cutting-edge, state-of-the-art (SOTA) model
released at **[https://github.com/ultralytics/ultralytics](https://github.com/ultralytics/ultralytics)**.
YOLOv8 is designed to be fast, accurate, and easy to use, making it an excellent choice for a wide range of
object detection, image segmentation and image classification tasks.
See the [YOLOv8 Docs](https://docs.ultralytics.com) for details and get started with:
```commandline
pip install ultralytics
```
<div align="center">
<a href="https://ultralytics.com/yolov8" target="_blank">
<img width="100%" src="https://raw.githubusercontent.com/ultralytics/assets/main/yolov8/yolo-comparison-plots.png"></a>
</div>
## <div align="center">Documentation</div>
See the [YOLOv5 Docs](https://docs.ultralytics.com) for full documentation on training, testing and deployment. See below for quickstart examples.
<details open>
<summary>Install</summary>
Clone repo and install [requirements.txt](https://github.com/ultralytics/yolov5/blob/master/requirements.txt) in a
[**Python>=3.7.0**](https://www.python.org/) environment, including
[**PyTorch>=1.7**](https://pytorch.org/get-started/locally/).
```bash
git clone https://github.com/ultralytics/yolov5 # clone
cd yolov5
pip install -r requirements.txt # install
```
</details>
<details>
<summary>Inference</summary>
YOLOv5 [PyTorch Hub](https://github.com/ultralytics/yolov5/issues/36) inference. [Models](https://github.com/ultralytics/yolov5/tree/master/models) download automatically from the latest
YOLOv5 [release](https://github.com/ultralytics/yolov5/releases).
```python
import torch
# Model
model = torch.hub.load("ultralytics/yolov5", "yolov5s") # or yolov5n - yolov5x6, custom
# Images
img = "https://ultralytics.com/images/zidane.jpg" # or file, Path, PIL, OpenCV, numpy, list
# Inference
results = model(img)
# Results
results.print() # or .show(), .save(), .crop(), .pandas(), etc.
```
</details>
<details>
<summary>Inference with detect.py</summary>
`detect.py` runs inference on a variety of sources, downloading [models](https://github.com/ultralytics/yolov5/tree/master/models) automatically from
the latest YOLOv5 [release](https://github.com/ultralytics/yolov5/releases) and saving results to `runs/detect`.
```bash
python detect.py --weights yolov5s.pt --source 0 # webcam
img.jpg # image
vid.mp4 # video
screen # screenshot
path/ # directory
list.txt # list of images
list.streams # list of streams
'path/*.jpg' # glob
'https://youtu.be/Zgi9g1ksQHc' # YouTube
'rtsp://example.com/media.mp4' # RTSP, RTMP, HTTP stream
```
</details>
<details>
<summary>Training</summary>
The commands below reproduce YOLOv5 [COCO](https://github.com/ultralytics/yolov5/blob/master/data/scripts/get_coco.sh)
results. [Models](https://github.com/ultralytics/yolov5/tree/master/models)
and [datasets](https://github.com/ultralytics/yolov5/tree/master/data) download automatically from the latest
YOLOv5 [release](https://github.com/ultralytics/yolov5/releases). Training times for YOLOv5n/s/m/l/x are
1/2/4/6/8 days on a V100 GPU ([Multi-GPU](https://github.com/ultralytics/yolov5/issues/475) times faster). Use the
largest `--batch-size` possible, or pass `--batch-size -1` for
YOLOv5 [AutoBatch](https://github.com/ultralytics/yolov5/pull/5092). Batch sizes shown for V100-16GB.
```bash
python train.py --data coco.yaml --epochs 300 --weights '' --cfg yolov5n.yaml --batch-size 128
没有合适的资源?快使用搜索试试~ 我知道了~
温馨提示
<项目介绍> 基于YOLOv5的路面病害识别模型,转化为tensflow lite模型并部署到移动端 使用基于pytorch的框架yolov5进行模型训练,数据集使用了RDDC2020数据集 训练完毕后的pt文件移植为Tensflow Lite格式,以便于移植到移动端 将转化后的TFLite模型文件,通过Tensflow的app Demo说明,移植到自己的app - 不懂运行,下载完可以私聊问,可远程教学 该资源内项目源码是个人的毕设,代码都测试ok,都是运行成功后才上传资源,答辩评审平均分达到96分,放心下载使用! 1、该资源内项目代码都经过测试运行成功,功能ok的情况下才上传的,请放心下载使用! 2、本项目适合计算机相关专业(如计科、人工智能、通信工程、自动化、电子信息等)的在校学生、老师或者企业员工下载学习,也适合小白学习进阶,当然也可作为毕设项目、课程设计、作业、项目初期立项演示等。 3、如果基础还行,也可在此代码基础上进行修改,以实现其他功能,也可用于毕设、课设、作业等。 下载后请首先打开README.md文件(如有),仅供学习参考, 切勿用于商业用途。 --------
资源推荐
资源详情
资源评论
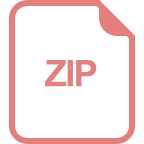
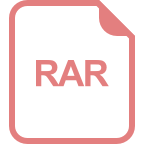
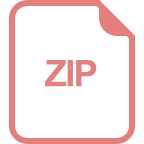
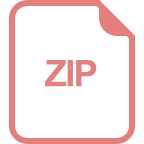
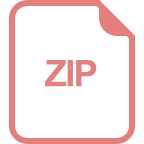
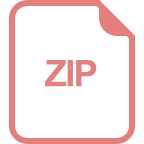
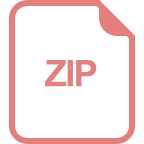
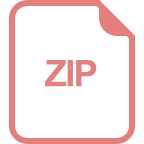
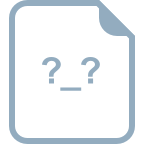
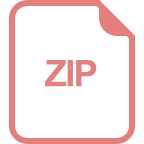
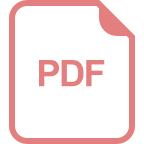
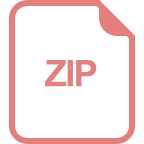
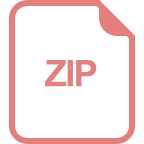
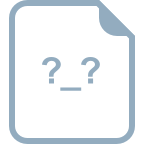
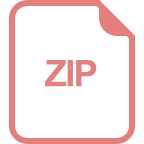
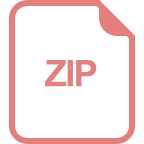
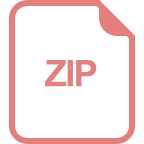
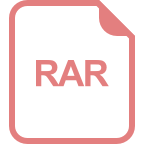
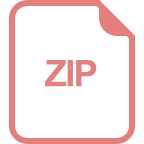
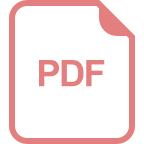
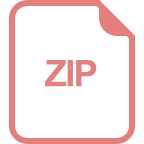
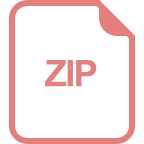
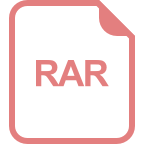
收起资源包目录

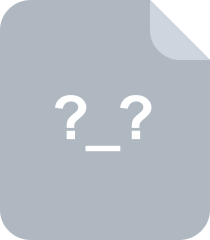
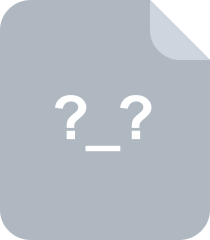
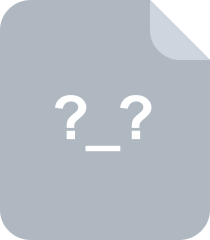
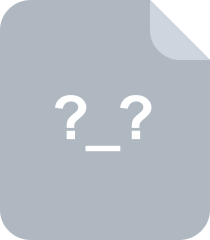
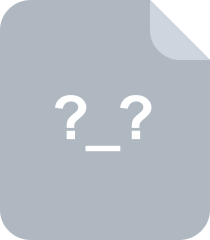
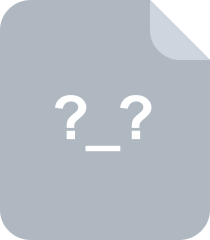
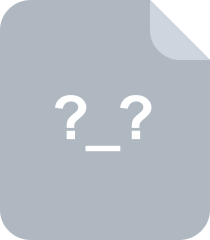
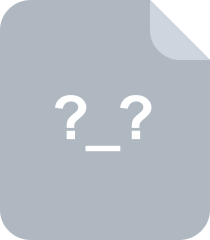
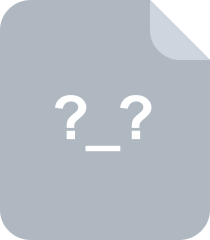
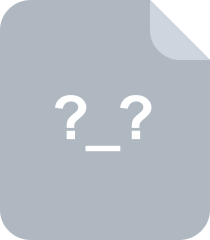
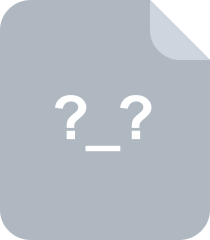
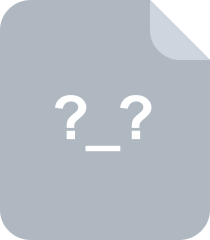
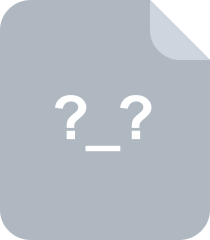
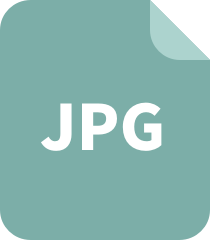
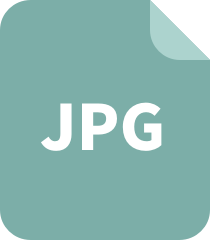
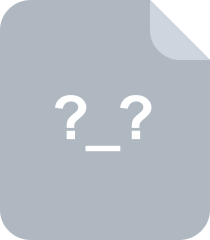
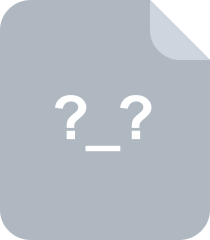
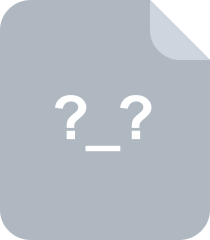
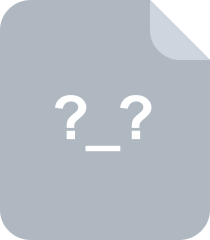
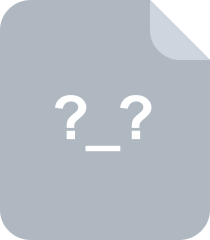
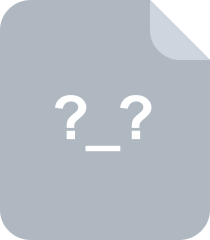
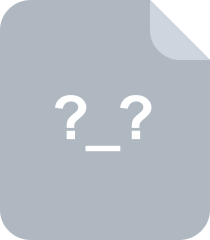
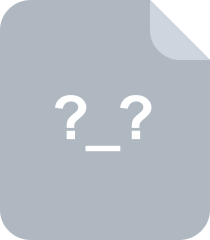
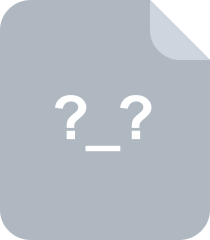
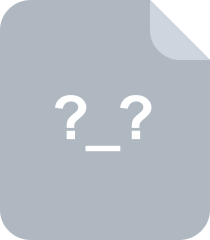
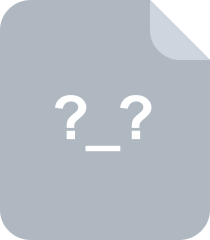
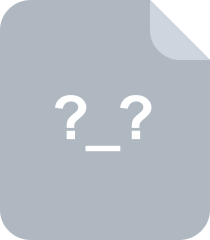
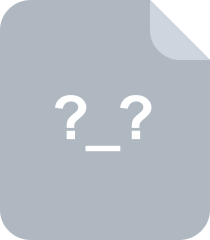
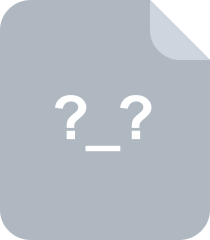
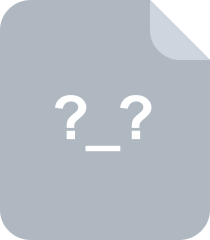
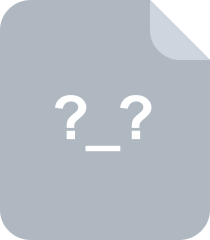
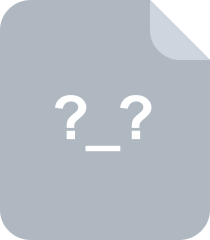
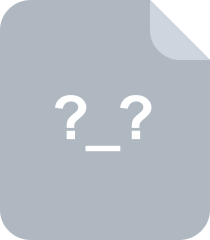
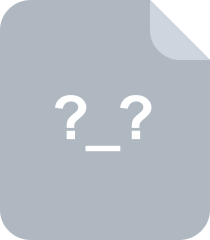
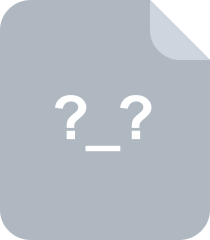
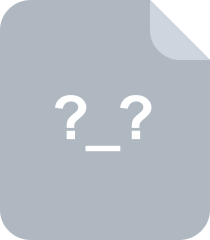
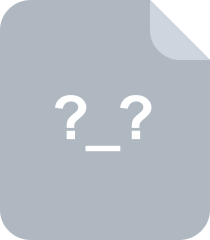
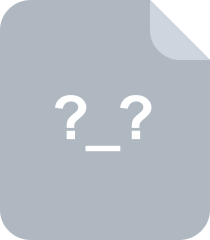
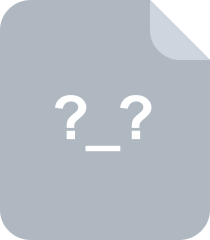
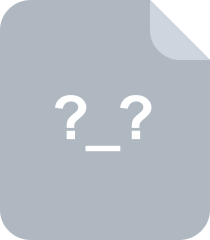
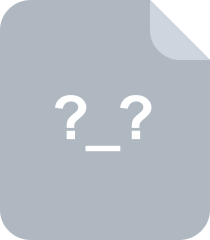
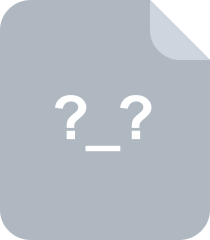
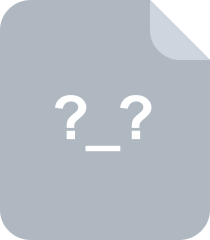
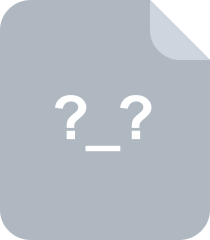
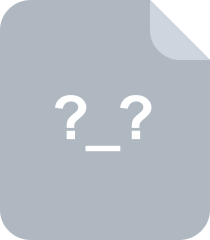
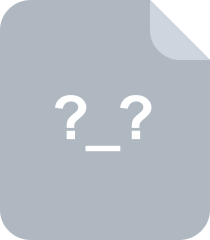
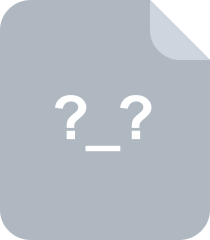
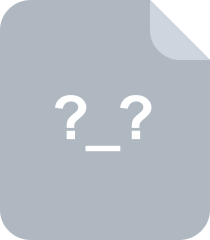
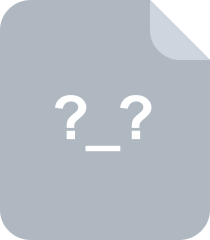
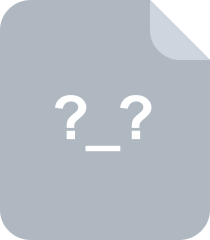
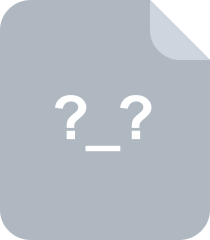
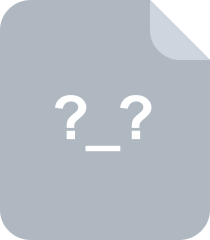
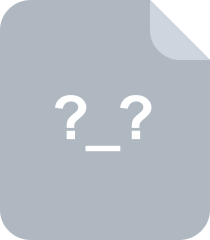
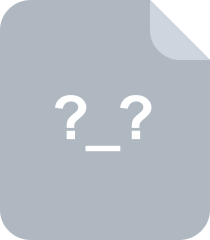
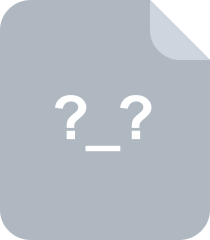
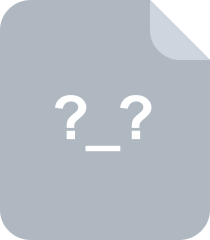
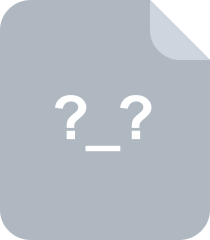
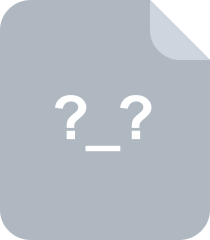
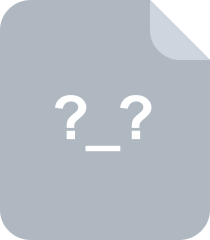
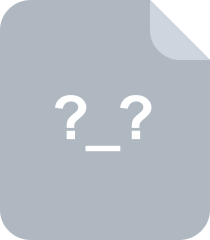
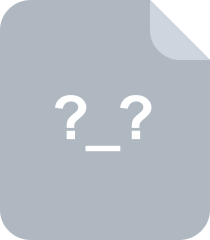
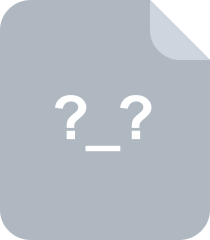
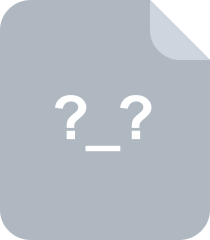
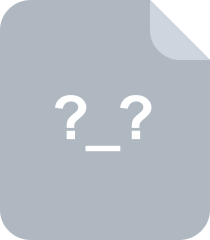
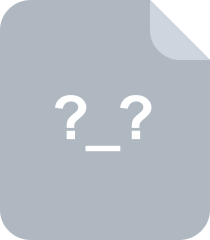
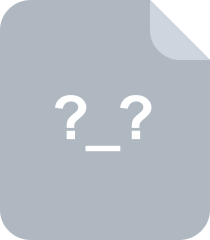
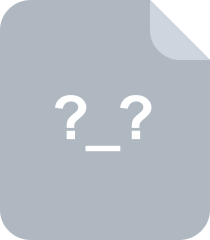
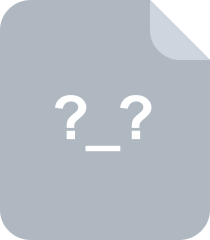
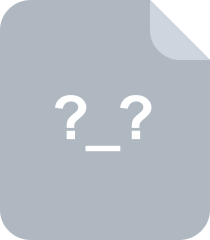
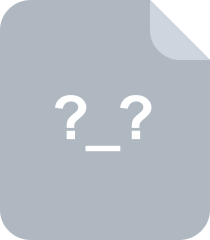
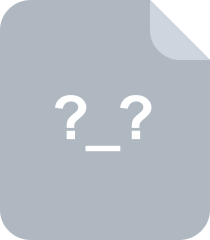
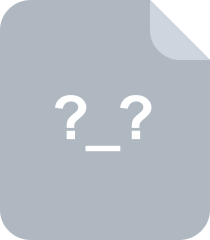
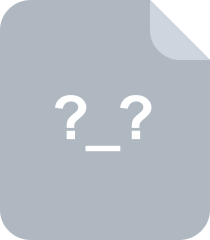
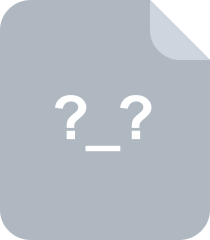
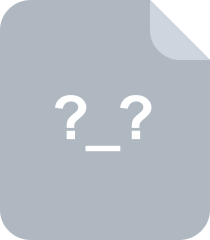
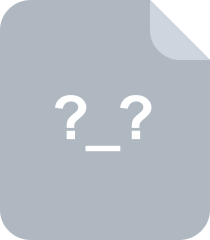
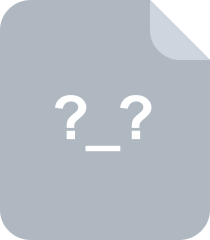
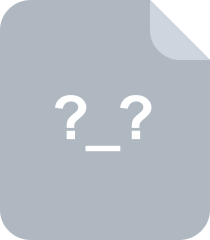
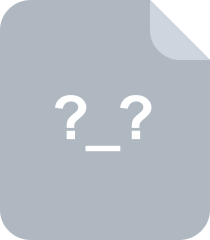
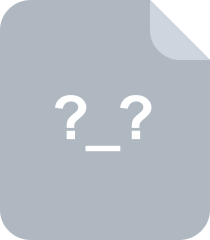
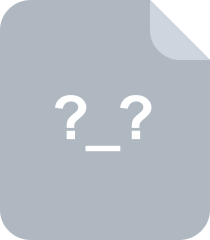
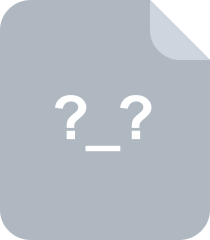
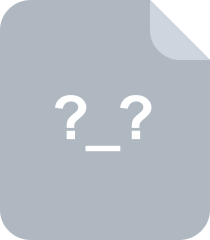
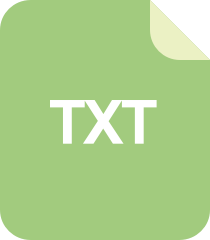
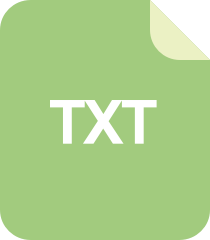
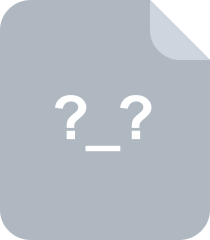
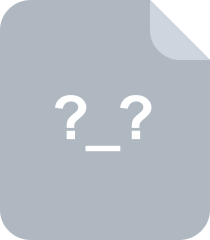
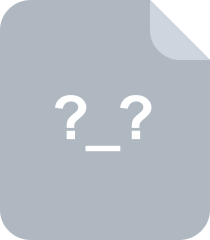
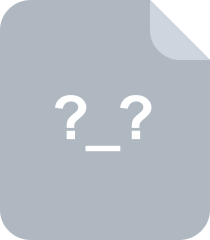
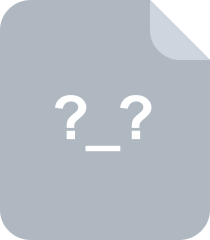
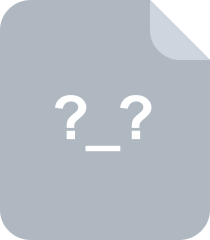
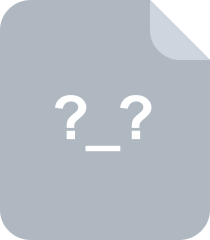
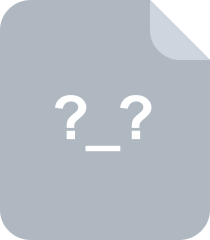
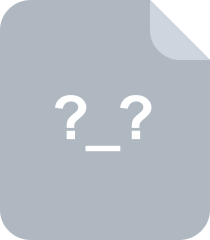
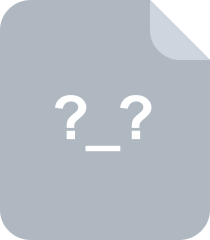
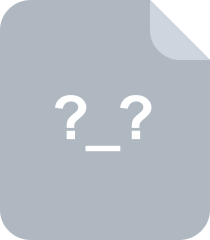
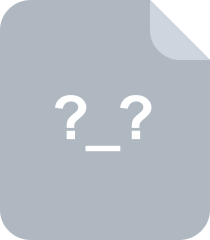
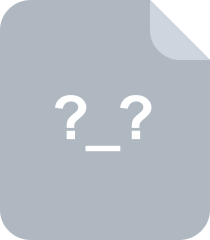
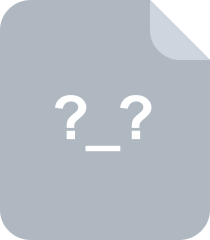
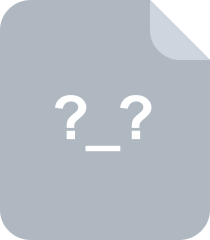
共 133 条
- 1
- 2
资源评论
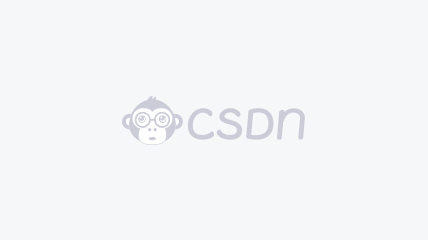

机智的程序员zero
- 粉丝: 1952
- 资源: 4202
上传资源 快速赚钱
我的内容管理 展开
我的资源 快来上传第一个资源
我的收益
登录查看自己的收益我的积分 登录查看自己的积分
我的C币 登录后查看C币余额
我的收藏
我的下载
下载帮助

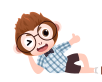
安全验证
文档复制为VIP权益,开通VIP直接复制
