import cv2
import tensorrt as trt
import torch
import numpy as np
from collections import OrderedDict,namedtuple
class TRT_engine():
def __init__(self, weight) -> None:
self.imgsz = [640,640]
self.weight = weight
self.device = torch.device('cuda:0')
self.init_engine()
def init_engine(self):
# Infer TensorRT Engine
self.Binding = namedtuple('Binding', ('name', 'dtype', 'shape', 'data', 'ptr'))
self.logger = trt.Logger(trt.Logger.INFO)
trt.init_libnvinfer_plugins(self.logger, namespace="")
with open(self.weight, 'rb') as self.f, trt.Runtime(self.logger) as self.runtime:
self.model = self.runtime.deserialize_cuda_engine(self.f.read())
self.bindings = OrderedDict()
self.fp16 = False
for index in range(self.model.num_bindings):
self.name = self.model.get_binding_name(index)
self.dtype = trt.nptype(self.model.get_binding_dtype(index))
self.shape = tuple(self.model.get_binding_shape(index))
self.data = torch.from_numpy(np.empty(self.shape, dtype=np.dtype(self.dtype))).to(self.device)
self.bindings[self.name] = self.Binding(self.name, self.dtype, self.shape, self.data, int(self.data.data_ptr()))
if self.model.binding_is_input(index) and self.dtype == np.float16:
self.fp16 = True
self.binding_addrs = OrderedDict((n, d.ptr) for n, d in self.bindings.items())
self.context = self.model.create_execution_context()
def letterbox(self,im,color=(114, 114, 114), auto=False, scaleup=True, stride=32):
# Resize and pad image while meeting stride-multiple constraints
shape = im.shape[:2] # current shape [height, width]
new_shape = self.imgsz
if isinstance(new_shape, int):
new_shape = (new_shape, new_shape)
# Scale ratio (new / old)
self.r = min(new_shape[0] / shape[0], new_shape[1] / shape[1])
if not scaleup: # only scale down, do not scale up (for better val mAP)
self.r = min(self.r, 1.0)
# Compute padding
new_unpad = int(round(shape[1] * self.r)), int(round(shape[0] * self.r))
self.dw, self.dh = new_shape[1] - new_unpad[0], new_shape[0] - new_unpad[1] # wh padding
if auto: # minimum rectangle
self.dw, self.dh = np.mod(self.dw, stride), np.mod(self.dh, stride) # wh padding
self.dw /= 2 # divide padding into 2 sides
self.dh /= 2
if shape[::-1] != new_unpad: # resize
im = cv2.resize(im, new_unpad, interpolation=cv2.INTER_LINEAR)
top, bottom = int(round(self.dh - 0.1)), int(round(self.dh + 0.1))
left, right = int(round(self.dw - 0.1)), int(round(self.dw + 0.1))
self.img = cv2.copyMakeBorder(im, top, bottom, left, right, cv2.BORDER_CONSTANT, value=color) # add border
return self.img,self.r,self.dw,self.dh
def preprocess(self,image):
self.img,self.r,self.dw,self.dh = self.letterbox(image)
self.img = self.img.transpose((2, 0, 1))
self.img = np.expand_dims(self.img,0)
self.img = np.ascontiguousarray(self.img)
self.img = torch.from_numpy(self.img).to(self.device)
self.img = self.img.float()
return self.img
def predict(self,img,threshold):
img = self.preprocess(img)
self.binding_addrs['images'] = int(img.data_ptr())
self.context.execute_v2(list(self.binding_addrs.values()))
nums = self.bindings['num_dets'].data[0].tolist()
boxes = self.bindings['det_boxes'].data[0].tolist()
scores =self.bindings['det_scores'].data[0].tolist()
classes = self.bindings['det_classes'].data[0].tolist()
num = int(nums[0])
new_bboxes = []
for i in range(num):
if(scores[i] < threshold):
continue
xmin = (boxes[i][0] - self.dw)/self.r
ymin = (boxes[i][1] - self.dh)/self.r
xmax = (boxes[i][2] - self.dw)/self.r
ymax = (boxes[i][3] - self.dh)/self.r
new_bboxes.append([classes[i],scores[i],xmin,ymin,xmax,ymax])
return new_bboxes
def visualize(img,bbox_array):
for temp in bbox_array:
xmin = int(temp[2])
ymin = int(temp[3])
xmax = int(temp[4])
ymax = int(temp[5])
clas = int(temp[0])
score = temp[1]
cv2.rectangle(img,(xmin,ymin),(xmax,ymax), (105, 237, 249), 2)
img = cv2.putText(img, "class:"+str(clas)+" "+str(round(score,2)), (xmin,int(ymin)-5), cv2.FONT_HERSHEY_SIMPLEX, 0.5, (105, 237, 249), 1)
return img
trt_engine = TRT_engine("./trt_model/yolov7_tiny_fp16.engine")
img = cv2.imread("./pictures/zidane.jpg")
results = trt_engine.predict(img,threshold=0.5)
img = visualize(img,results)
cv2.imshow("img",img)
cv2.waitKey(0)
没有合适的资源?快使用搜索试试~ 我知道了~
基于YOLOv7的tensorrt部署(源码+说明文档).rar
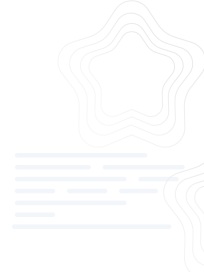
共8个文件
py:3个
png:2个
jpg:2个

1.该资源内容由用户上传,如若侵权请联系客服进行举报
2.虚拟产品一经售出概不退款(资源遇到问题,请及时私信上传者)
2.虚拟产品一经售出概不退款(资源遇到问题,请及时私信上传者)
版权申诉

温馨提示
1、资源内容: 2、适用人群:计算机,电子信息工程、数学等专业的大学生课程设计、期末大作业或毕业设计,作为“参考资料”使用。 3、更多仿真源码和数据集下载列表(自行寻找自己需要的):https://blog.csdn.net/m0_62143653?type=download 4、免责声明:本资源作为“参考资料”而不是“定制需求”不一定能够满足所有人的需求,需要有一定的基础能够看懂代码,能够自行调试,能够自行添加功能修改代码。由于作者大厂工作较忙,不提供答疑服务,如不存在资源缺失问题概不负责,谢谢理解。
资源推荐
资源详情
资源评论
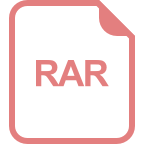
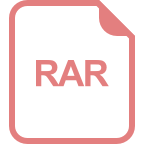
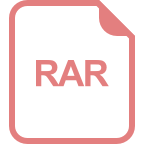
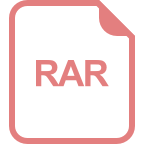
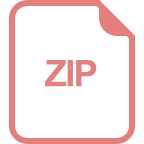
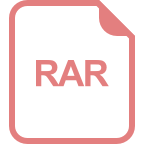
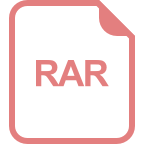
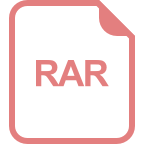
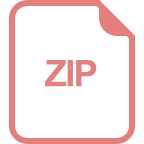
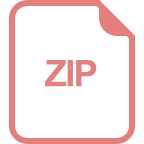
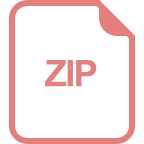
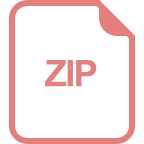
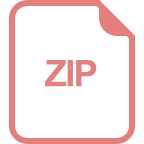
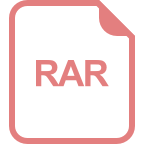
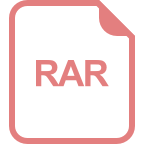
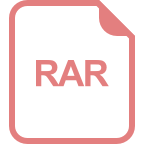
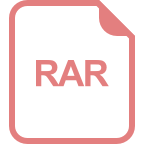
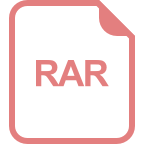
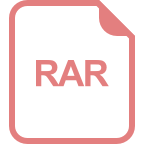
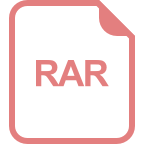
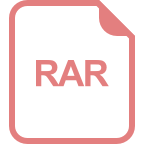
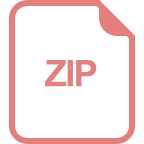
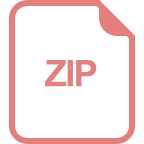
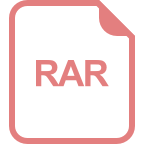
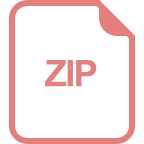
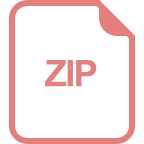
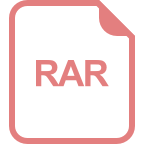
收起资源包目录


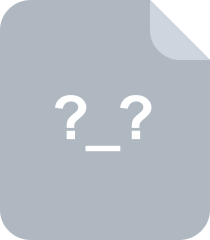

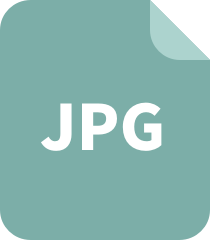
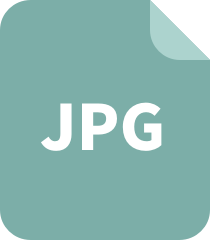

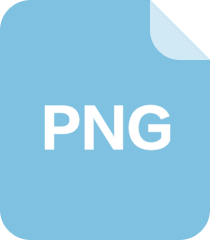
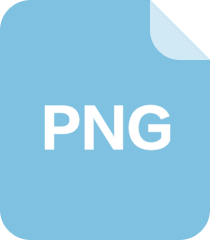
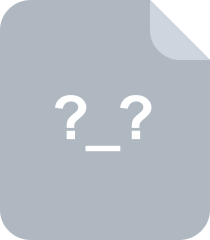
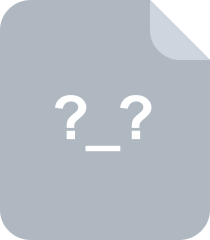
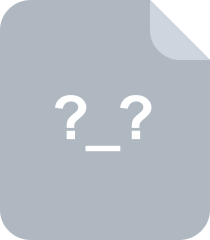
共 8 条
- 1
资源评论
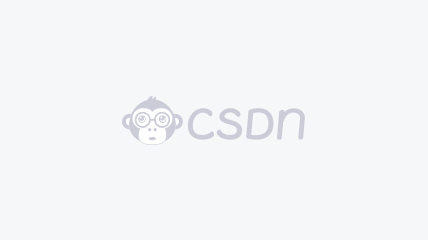
- sinbin002023-08-09骗人,千万别下 #毫无价值
- m0_751126362023-07-26资源很实用,对我启发很大,有很好的参考价值,内容详细。
- @susu2023-10-31资源有很好的参考价值,总算找到了自己需要的资源啦。
- qxjie_7724562023-05-31这个资源对我启发很大,受益匪浅,学到了很多,谢谢分享~
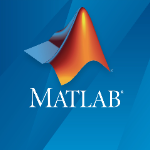
Matlab仿真实验室
- 粉丝: 3w+
- 资源: 2405
上传资源 快速赚钱
我的内容管理 展开
我的资源 快来上传第一个资源
我的收益
登录查看自己的收益我的积分 登录查看自己的积分
我的C币 登录后查看C币余额
我的收藏
我的下载
下载帮助

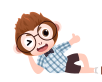
最新资源
资源上传下载、课程学习等过程中有任何疑问或建议,欢迎提出宝贵意见哦~我们会及时处理!
点击此处反馈


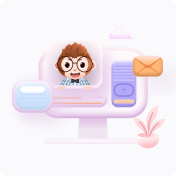
安全验证
文档复制为VIP权益,开通VIP直接复制
