# Dynamic Sparse Network for Time Series Classification: Learning What to “See”
This is the offical implementation for the paper titled Dynamic Sparse Network for Time Series Classification: Learning What to “See”.
## Requirements
- PyTorch 1.4.0
- torchvision 0.2.1
- numpy
- pandas
## Dataset
- The univariate time series datasets (UCR) could be acquired from [here](http://www.cs.ucr.edu/%7Eeamonn/time_series_data/)
- The multivariate time series datasets (UEA) could be acquired from [here](http://www.timeseriesclassification.com/)
- Datasets from UCI could be found [here](https://github.com/titu1994/MLSTM-FCN/releases)
## Training
To train models for **UCR 85 Archive**, change the value of --root (e.g., UCR_TS_Archive_2015) and run this command:
```
python trainer_DSN.py --sparse True --density 0.2 --sparse_init remain_sort --fix False --growth random --depth 4 --ch_size 47 --c_size 3 --k_size 39
```
To train models for **UCI datasets**, change the value of --root (e.g., UCI) and run this command:
```
python trainer_DSN.py --sparse True --density 0.2 --sparse_init remain_sort --fix False --growth random --depth 4 --ch_size 47 --c_size 3 --k_size 39
```
To train models for **UEA 30 Archive**, change the value of --root (e.g., UEA_TS_Archive_2018) and run this command:
```
python trainer_DSN.py --sparse True --density 0.1 --sparse_init remain_sort --fix False --growth random --depth 4 --ch_size 59 --c_size 3 --k_size 39
```
## Other Implementations
The implementation is heavily based on [ITOP](https://github.com/Shiweiliuiiiiiii/In-Time-Over-Parameterization)
## Citation
```
@inproceedings{
xiao2022dynamic,
title={Dynamic Sparse Network for Time Series Classification: Learning What to {\textquotedblleft}See{\textquotedblright}},
author={Qiao Xiao and Boqian Wu and Yu Zhang and Shiwei Liu and Mykola Pechenizkiy and Elena Mocanu and Decebal Constantin Mocanu},
booktitle={Advances in Neural Information Processing Systems},
year={2022},
url={https://openreview.net/forum?id=ZxOO5jfqSYw}
}
```
没有合适的资源?快使用搜索试试~ 我知道了~
温馨提示
基于DSN(动态稀疏网络)的时间序列分类(Python完整源码和数据) 基于DSN(动态稀疏网络)的时间序列分类(Python完整源码和数据) 基于DSN(动态稀疏网络)的时间序列分类(Python完整源码和数据) DSN 动态稀疏网络 时间序列 时间序列分类
资源推荐
资源详情
资源评论
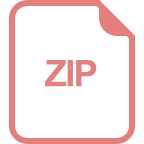
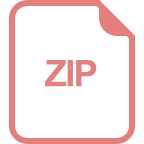
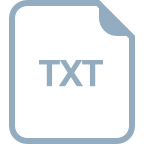
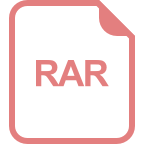
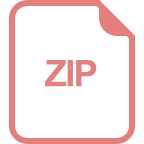
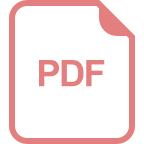
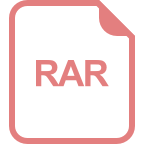
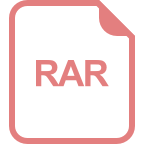
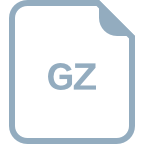
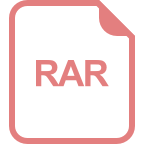
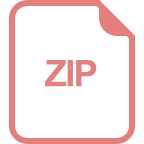
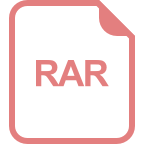
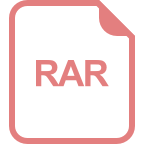
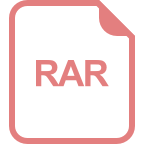
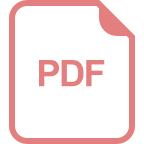
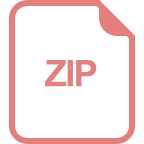
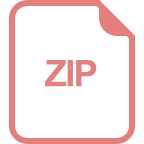
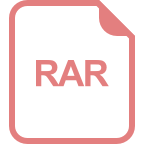
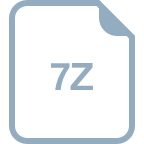
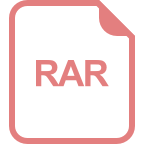
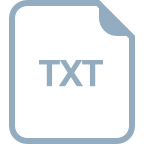
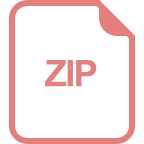
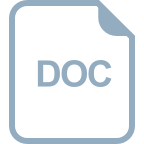
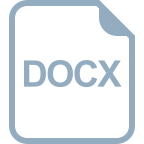
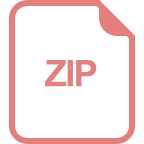
收起资源包目录



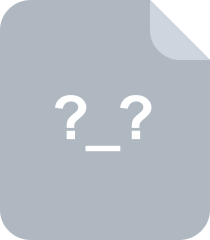
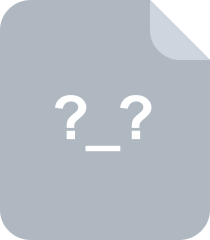

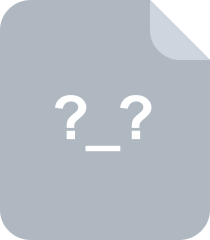
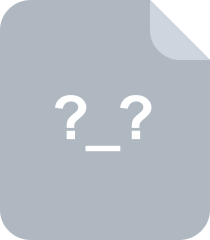
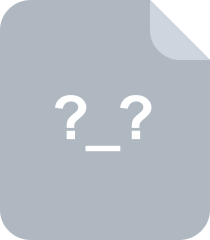
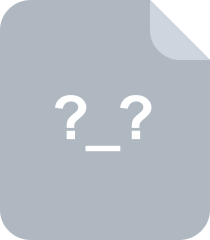

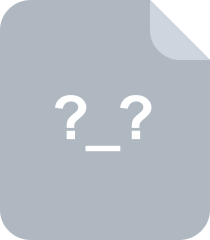
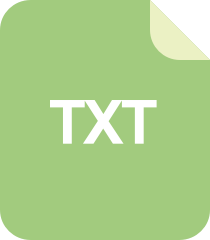
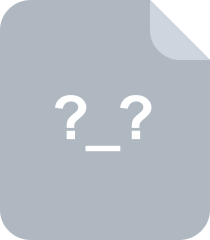

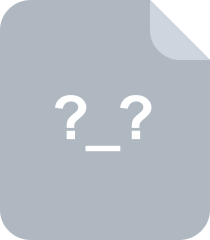
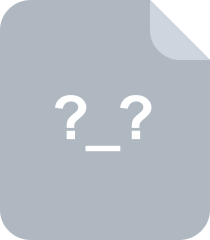
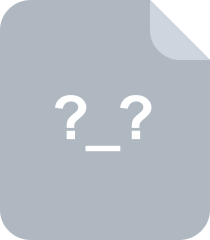
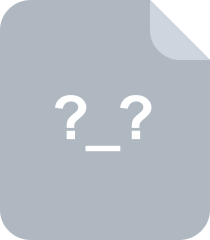
共 13 条
- 1
资源评论
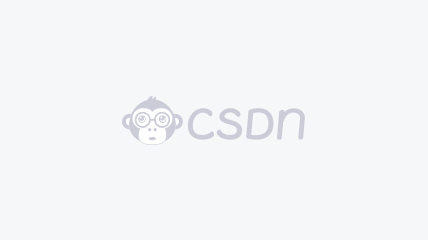
- kefuxiaobai2023-03-22感谢资源主的分享,这个资源对我来说很有用,内容描述详尽,值得借鉴。

前程算法屋
- 粉丝: 5460
- 资源: 782
上传资源 快速赚钱
我的内容管理 展开
我的资源 快来上传第一个资源
我的收益
登录查看自己的收益我的积分 登录查看自己的积分
我的C币 登录后查看C币余额
我的收藏
我的下载
下载帮助

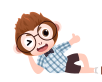
最新资源
资源上传下载、课程学习等过程中有任何疑问或建议,欢迎提出宝贵意见哦~我们会及时处理!
点击此处反馈


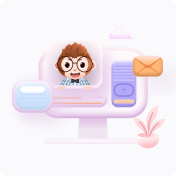
安全验证
文档复制为VIP权益,开通VIP直接复制
