#include <stdio.h>
#include <stdlib.h>
#include <string.h>
#include <ctype.h>
#include "svm.h"
#include "mex.h"
#include "svm_model_matlab.h"
#define CMD_LEN 2048
#define Malloc(type,n) (type *)malloc((n)*sizeof(type))
void exit_with_help()
{
mexPrintf(
"Usage: model = svmtrain(training_label_vector, training_instance_matrix, 'libsvm_options');\n"
"libsvm_options:\n"
"-s svm_type : set type of SVM (default 0)\n"
" 0 -- C-SVC\n"
" 1 -- nu-SVC\n"
" 2 -- one-class SVM\n"
" 3 -- epsilon-SVR\n"
" 4 -- nu-SVR\n"
"-t kernel_type : set type of kernel function (default 2)\n"
" 0 -- linear: u'*v\n"
" 1 -- polynomial: (gamma*u'*v + coef0)^degree\n"
" 2 -- radial basis function: exp(-gamma*|u-v|^2)\n"
" 3 -- sigmoid: tanh(gamma*u'*v + coef0)\n"
"-d degree : set degree in kernel function (default 3)\n"
"-g gamma : set gamma in kernel function (default 1/k)\n"
"-r coef0 : set coef0 in kernel function (default 0)\n"
"-c cost : set the parameter C of C-SVC, epsilon-SVR, and nu-SVR (default 1)\n"
"-n nu : set the parameter nu of nu-SVC, one-class SVM, and nu-SVR (default 0.5)\n"
"-p epsilon : set the epsilon in loss function of epsilon-SVR (default 0.1)\n"
"-m cachesize : set cache memory size in MB (default 40)\n"
"-e epsilon : set tolerance of termination criterion (default 0.001)\n"
"-h shrinking: whether to use the shrinking heuristics, 0 or 1 (default 1)\n"
"-b probability_estimates: whether to train a SVC or SVR model for probability estimates, 0 or 1 (default 0)\n"
"-wi weight: set the parameter C of class i to weight*C, for C-SVC (default 1)\n"
"-v n: n-fold cross validation mode\n"
);
}
// svm arguments
struct svm_parameter param; // set by parse_command_line
struct svm_problem prob; // set by read_problem
struct svm_model *model;
struct svm_node *x_space;
int cross_validation;
int nr_fold;
double do_cross_validation()
{
int i;
int total_correct = 0;
double total_error = 0;
double sumv = 0, sumy = 0, sumvv = 0, sumyy = 0, sumvy = 0;
double *target = Malloc(double,prob.l);
double retval = 0.0;
// fix random seed to have same results for each run
srand(1);
svm_cross_validation(&prob,¶m,nr_fold,target);
if(param.svm_type == EPSILON_SVR ||
param.svm_type == NU_SVR)
{
for(i=0;i<prob.l;i++)
{
double y = prob.y[i];
double v = target[i];
total_error += (v-y)*(v-y);
sumv += v;
sumy += y;
sumvv += v*v;
sumyy += y*y;
sumvy += v*y;
}
mexPrintf("Cross Validation Mean squared error = %g\n",total_error/prob.l);
mexPrintf("Cross Validation Squared correlation coefficient = %g\n",
((prob.l*sumvy-sumv*sumy)*(prob.l*sumvy-sumv*sumy))/
((prob.l*sumvv-sumv*sumv)*(prob.l*sumyy-sumy*sumy))
);
retval = total_error/prob.l;
}
else
{
for(i=0;i<prob.l;i++)
if(target[i] == prob.y[i])
++total_correct;
mexPrintf("Cross Validation Accuracy = %g%%\n",100.0*total_correct/prob.l);
retval = 100.0*total_correct/prob.l;
}
free(target);
return retval;
}
// nrhs should be 3
int parse_command_line(int nrhs, const mxArray *prhs[], char *model_file_name)
{
int i, argc = 1;
char cmd[CMD_LEN];
char *argv[CMD_LEN/2];
// default values
param.svm_type = C_SVC;
param.kernel_type = RBF;
param.degree = 3;
param.gamma = 0; // 1/k
param.coef0 = 0;
param.nu = 0.5;
param.cache_size = 40;
param.C = 1;
param.eps = 1e-3;
param.p = 0.1;
param.shrinking = 1;
param.probability = 0;
param.nr_weight = 0;
param.weight_label = NULL;
param.weight = NULL;
cross_validation = 0;
if(nrhs <= 1)
return 1;
if(nrhs == 2)
return 0;
// put options in argv[]
mxGetString(prhs[2], cmd, mxGetN(prhs[2]) + 1);
if((argv[argc] = strtok(cmd, " ")) == NULL)
return 0;
while((argv[++argc] = strtok(NULL, " ")) != NULL)
;
// parse options
for(i=1;i<argc;i++)
{
if(argv[i][0] != '-') break;
if(++i>=argc)
return 1;
switch(argv[i-1][1])
{
case 's':
param.svm_type = atoi(argv[i]);
break;
case 't':
param.kernel_type = atoi(argv[i]);
break;
case 'd':
param.degree = atof(argv[i]);
break;
case 'g':
param.gamma = atof(argv[i]);
break;
case 'r':
param.coef0 = atof(argv[i]);
break;
case 'n':
param.nu = atof(argv[i]);
break;
case 'm':
param.cache_size = atof(argv[i]);
break;
case 'c':
param.C = atof(argv[i]);
break;
case 'e':
param.eps = atof(argv[i]);
break;
case 'p':
param.p = atof(argv[i]);
break;
case 'h':
param.shrinking = atoi(argv[i]);
break;
case 'b':
param.probability = atoi(argv[i]);
break;
case 'v':
cross_validation = 1;
nr_fold = atoi(argv[i]);
if(nr_fold < 2)
{
mexPrintf("n-fold cross validation: n must >= 2\n");
return 1;
}
break;
case 'w':
++param.nr_weight;
param.weight_label = (int *)realloc(param.weight_label,sizeof(int)*param.nr_weight);
param.weight = (double *)realloc(param.weight,sizeof(double)*param.nr_weight);
param.weight_label[param.nr_weight-1] = atoi(&argv[i-1][2]);
param.weight[param.nr_weight-1] = atof(argv[i]);
break;
default:
mexPrintf("unknown option\n");
return 1;
}
}
return 0;
}
// read in a problem (in svmlight format)
void read_problem_dense(const mxArray *label_vec, const mxArray *instance_mat)
{
int i, j, k;
int elements, max_index, sc;
double *samples, *labels;
labels = mxGetPr(label_vec);
samples = mxGetPr(instance_mat);
sc = mxGetN(instance_mat);
elements = 0;
// the number of instance
prob.l = mxGetM(instance_mat);
for(i = 0; i < prob.l; i++)
{
for(k = 0; k < sc; k++)
if(samples[k * prob.l + i] != 0)
elements++;
// count the '-1' element
elements++;
}
prob.y = Malloc(double,prob.l);
prob.x = Malloc(struct svm_node *,prob.l);
x_space = Malloc(struct svm_node, elements);
max_index = sc;
j = 0;
for(i = 0; i < prob.l; i++)
{
prob.x[i] = &x_space[j];
prob.y[i] = labels[i];
for(k = 0; k < sc; k++)
{
if(samples[k * prob.l + i] != 0)
{
x_space[j].index = k + 1;
x_space[j].value = samples[k * prob.l + i];
j++;
}
}
x_space[j++].index = -1;
}
if(param.gamma == 0)
param.gamma = 1.0/max_index;
}
void read_problem_sparse(const mxArray *label_vec, const mxArray *instance_mat)
{
int i, j, k, low, high;
int *ir, *jc;
int elements, max_index, num_samples;
double *samples, *labels;
mxArray *instance_mat_tr; // transposed instance sparse matrix
// transpose instance matrix
{
mxArray *prhs[1], *plhs[1];
prhs[0] = mxDuplicateArray(instance_mat);
if (mexCallMATLAB(1, plhs, 1, prhs, "transpose")) {
mexPrintf("Error: cannot transpose training instance matrix\n");
return;
}
instance_mat_tr = plhs[0];
}
// each column is one instance
labels = mxGetPr(label_vec);
samples = mxGetPr(instance_mat_tr);
ir = mxGetIr(instance_mat_tr);
jc = mxGetJc(instance_mat_tr);
num_samples = mxGetNzmax(instance_mat_tr);
// the number of instance
prob.l = mxGetN(instance_mat_tr);
elements = num_samples + prob.l;
max_index = mxGetM(instance_mat_tr);
prob.y = Malloc(double,prob.l);
prob.x = Malloc(struct svm_node *,prob.l);
x_space = Malloc(struct svm_node, elements);
j = 0;
for(i=0;i<prob.l;i++)
{
prob.x[i] = &x_space[j];
prob.y[i] = labels[i];
low = jc[i], high = jc[i+1];
for(k=low;k<high;k++)
{
x_space[j].index = ir[k] + 1;
x_space[j].value = samples[k];
j++;
}
x_space[j++].index = -1;
}
if(param.gamma == 0)
param.gamma = 1.0/max_index;
}
static void fake_answer(mxArray *plhs[])
{
plhs[0] = mxCreateDoubleMatrix(0, 0, mxREAL);
}
// Interface function of matlab
// now assume prhs[0]: label prhs[1]: features
void mexFunction( int nlhs, mxArray *plhs[],
int nrhs, const mxArray *prhs[] )
{
const char *error_msg;
// Translate the input Matrix to the format such that svmtrain.exe can recognize it
if(nrhs > 0 && nrhs < 4)
{
if(parse_command_line(nrhs, prhs, NULL))
{
exit_with_help();
svm_destroy_param(¶m);
fake_answer(


阿里matlab建模师
- 粉丝: 4255
- 资源: 2843
最新资源
- 基于Go和React的高质量企业微信私域流量管理系统 。遵守Apache2.0协议,全网唯一免费商用。企业微信、私域流量、SCRM。详细文档+优秀项目+全部资料.zip
- 基于Go和React的企业微信的开源 SCRM 系统。企微,个微,微信,聚合聊天,自动回复,加好友。详细文档+优秀项目+全部资料.zip
- 基于go语言的搜索引擎,信息检索系统详细文档+优秀项目+全部资料.zip
- 基于Go语言,Beego框架开发的简单的文章发布管理系统。详细文档+优秀项目+全部资料.zip
- 基于Go语言的轻量级高性能的分布式日志系统详细文档+优秀项目+全部资料.zip
- 基于go语言简单的用户管理系统详细文档+优秀项目+全部资料.zip
- 基于Go语言的一个秒杀系统详细文档+优秀项目+全部资料.zip
- 基于Go语言的一个简易blog系统详细文档+优秀项目+全部资料.zip
- 基于Go语言开发,具备高安全性、高性能和易扩展性的企业级内容管理系统,详细文档+优秀项目+全部资料.zip
- 基于Go语言实现的单点登录系统详细文档+优秀项目+全部资料.zip
- 基于Go语言实现的微服务电商系统详细文档+优秀项目+全部资料.zip
- 基于python和sql server2014做的商品学生信息管理系统,有界面,利用的sql server数据库详细文档+优秀项目+全部资料.zip
- 基于OWTP协议库,封装所有与openw-server钱包服务API交互方法。用于集成到go语言开发下的应用方系统。详细文档+优秀项目+全部资料.zip
- 基于Quasar和Go语言的功能全面的管理系统详细文档+优秀项目+全部资料.zip
- 基于SpringBoot 开发的员工的季度绩效考核系统详细文档+优秀项目+全部资料.zip
- 四足机器人技术发展及其应用场景概述
资源上传下载、课程学习等过程中有任何疑问或建议,欢迎提出宝贵意见哦~我们会及时处理!
点击此处反馈


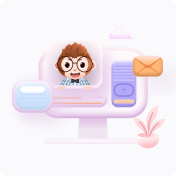
- 1
- 2
前往页