# YOLOv5-Compression

2021.10.30 复现TPH-YOLOv5
2021.10.31 完成替换backbone为Ghostnet
2021.11.02 完成替换backbone为Shufflenetv2
2021.11.05 完成替换backbone为Mobilenetv3Small
2021.11.10 完成EagleEye对YOLOv5系列剪枝支持
2021.11.14 完成MQBench对YOLOv5系列量化支持
2021.11.16 完成替换backbone为EfficientNetLite-0
2021.11.26 完成替换backbone为PP-LCNet-1x
2021.12.12 完成SwinTrans-YOLOv5(C3STR)
2021.12.15 完成Slimming对YOLOv5系列剪枝支持
## Requirements
```shell
pip install -r requirements.txt
```
## Multi-Backbone Substitution for YOLOs
### 1、Base Model
Train on Visdrone DataSet (*Input size is 608*)
| No. | Model | mAP | mAP@50 | Parameters(M) | GFLOPs |
| ---- | ------- | ---- | ------ | ------------- | ------ |
| 1 | YOLOv5n | 13.0 | 26.20 | 1.78 | 4.2 |
| 2 | YOLOv5s | 18.4 | 34.00 | 7.05 | 15.9 |
| 3 | YOLOv5m | 21.6 | 37.80 | 20.91 | 48.2 |
| 4 | YOLOv5l | 23.2 | 39.70 | 46.19 | 108.1 |
| 5 | YOLOv5x | 24.3 | 40.80 | 86.28 | 204.4 |
### 2、Higher Precision Model
#### A、TPH-YOLOv5 
Train on Visdrone DataSet (*6-7 size is 640,8 size is 1536*)
| No. | Model | mAP | mAP@50 | Parameters(M) | GFLOPs |
| ---- | -------------- | ---- | ------ | ------------- | ------ |
| 6 | YOLOv5xP2 | 30.0 | 49.29 | 90.96 | 314.2 |
| 7 | YOLOv5xP2 CBAM | 30.1 | 49.40 | 91.31 | 315.1 |
| 8 | YOLOv5x-TPH | 40.7 | 63.00 | 112.97 | 270.8 |
###### Usage:
```shell
nohup python train.py --data VisDrone.yaml --weights yolov5n.pt --cfg models/yolov5n.yaml --epochs 300 --batch-size 8 --img 608 --device 0,1 --sync-bn >> yolov5n.txt &
```
###### Composition:
**P2 Head、CBAM、TPH、BiFPN、SPP**
<img src="https://github.com/Gumpest/YOLOv5-Multibackbone-Compression/blob/main/img/TPH-YOLOv5.png" alt="TPH-YOLOv5" width="600px" height="300px" />
1、TransBlock的数量会根据YOLO规模的不同而改变,标准结构作用于YOLOv5m
2、当YOLOv5x为主体与标准结构的区别是:(1)首先去掉14和19的CBAM模块(2)降低与P2关联的通道数(128)(3)在输出头之前会添加SPP模块,注意SPP的kernel随着P的像素减小而减小(4)在CBAM之后进行输出(5)只保留backbone以及最后一层输出的TransBlock(6)采用BiFPN作为neck
3、更改不同Loss分支的权重:如下图,当训练集的分类与置信度损失还在下降时,验证集的分类与置信度损失开始反弹,说明出现了过拟合,需要降低这两个任务的权重
消融实验如下:
| box | cls | obj | acc |
| ---- | ---- | ---- | --------- |
| 0.05 | 0.5 | 1.0 | 37.90 |
| 0.05 | 0.3 | 0.7 | **38.00** |
| 0.05 | 0.2 | 0.4 | 37.5 |
<img src="https://github.com/Gumpest/YOLOv5-Multibackbone-Compression/blob/main/img/image-20211109150606998.png" alt="loss" width="600px" />
#### B、SwinTrans-YOLOv5
```shell
pip install timm
```
###### Usage:
```shell
python train.py --data VisDrone.yaml --weights yolov5x.pt --cfg models/accModels/yolov5xP2CBAM-Swin-BiFPN-SPP.yaml --hyp data/hyps/hyp.visdrone.yaml --epochs 60 --batch-size 4 --img 1536 --nohalf
```
(1)Window size由***7***替换为检测任务常用分辨率的公约数***8***
(2)create_mask封装为函数,由在init函数执行变为在forward函数执行
(3)若分辨率小于window size或不是其公倍数时,在其右侧和底部Padding
*debug:在计算完之后需要反padding回去,否则与cv2支路的img_size无法对齐*
(4)forward函数前后对输入输出reshape
(5)验证C3STR时,需要手动关闭默认模型在half精度下验证(*--nohalf*)
### 3、Slighter Model
Train on Visdrone DataSet (*1 size is 608,2-6 size is 640*)
| No | Model | mAP | mAP@50 | Parameters(M) | GFLOPs | TrainCost(h) | Memory Cost(G) | PT File | FPS@CPU |
| ---- | ------------------------- | --------- | ------ | ------------- | -------- | ------------ | -------------- | ------------------------------------------------------------ | ------- |
| 1 | YOLOv5l | 23.2 | 39.7 | 46.19 | 108.1 | | | | |
| 2 | YOLOv5l-GhostNet | 18.4 | 33.8 | 24.27 | 42.4 | 27.44 | 4.97 | [PekingUni Cloud](https://disk.pku.edu.cn:443/link/35BD905E65DE091E2A58316B20BBE775) | |
| 3 | YOLOv5l-ShuffleNetV2 | 16.48 | 31.1 | 21.27 | 40.5 | 10.98 | 2.41 | [PekingUni Cloud](https://disk.pku.edu.cn:443/link/A5ED89B7B190FCF1C8187A0A8AF20C4F) | |
| 4 | YOLOv5l-MobileNetv3Small | 16.55 | 31.2 | **20.38** | **38.4** | **10.19** | 5.30 | [PekingUni Cloud](https://disk.pku.edu.cn:443/link/EE375ED30AAD3F2B3FA5055DD6F4964C) | |
| 5 | YOLOv5l-EfficientNetLite0 | **19.12** | **35** | 23.01 | 43.9 | 13.94 | 2.04 | [PekingUni Cloud](https://disk.pku.edu.cn:443/link/45E65A080C4574036EE274B7BD83B7EA) | |
| 6 | YOLOv5l-PP-LCNet | 17.63 | 32.8 | 21.64 | 41.7 | 18.52 | **1.66** | [PekingUni Cloud](https://disk.pku.edu.cn:443/link/7EBE07BA6D7985C7053BF0A8F2591464) | |
#### A、GhostNet-YOLOv5 
<img src="https://github.com/Gumpest/YOLOv5-Multibackbone-Compression/blob/main/img/GhostNet.jpg" alt="GhostNet" width="500px" height="250px" />
(1)为保持一致性,下采样的DW的kernel_size均等于3
(2)neck部分与head部分沿用YOLOv5l原结构
(3)中间通道人为设定(expand)
#### B、ShuffleNetV2-YOLOv5 
<img src="https://github.com/Gumpest/YOLOv5-Multibackbone-Compression/blob/main/img/Shffulenet.png" alt="Shffulenet" width="400px" />
(1)Focus Layer不利于芯片部署,频繁的slice操作会让缓存占用严重
(2)避免多次使用C3 Leyer以及高通道的C3 Layer(违背G1与G3准则)
(3)中间通道不变
#### C、MobileNetv3Small-YOLOv5 
<img src="https://github.com/Gumpest/YOLOv5-Multibackbone-Compression/blob/main/img/Mobilenetv3s.jpg" alt="Mobilenetv3s" width="500px" />
(1)原文结构,部分使用Hard-Swish激活函数以及SE模块
(2)Neck与head部分嫁接YOLOv5l原结构
(3)中间通道人为设定(expand)
#### D、EfficientNetLite0-YOLOv5 
<img src="https://github.com/Gumpest/YOLOv5-Multibackbone-Compression/blob/main/img/efficientlite.jpg" alt="efficientlite" width="500px" />
(1)使用Lite0结构,且不使用SE模块
(2)针对dropout_connect_rate,手动赋值(随着idx_stage变大而变大)
(3)中间通道一律*6(expand)
#### E、PP-LCNet-YOLOv5 
<img src="https://github.com/Gumpest/YOLOv5-Multibackbone-Compression/blob/main/img/PP-LCNet.png" alt="PP-LCNet" width="500px" />
(1)使用PP-LCNet-1x结构,在网络末端使用SE以及5*5卷积核
(2)SeBlock压缩维度为原1/16
(3)中间通道不变
## Pruning for YOLOs
| Model | mAP |
没有合适的资源?快使用搜索试试~ 我知道了~
YOLOv5系列多主干(TPH-YOLOv5、Ghostnet、ShuffleNetv2、Mobilenetv3Small、Ef...
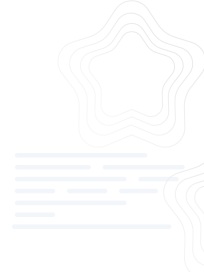
共25个文件
yaml:11个
py:8个
txt:3个

1.该资源内容由用户上传,如若侵权请联系客服进行举报
2.虚拟产品一经售出概不退款(资源遇到问题,请及时私信上传者)
2.虚拟产品一经售出概不退款(资源遇到问题,请及时私信上传者)
版权申诉
0 下载量 116 浏览量
2024-11-26
12:19:41
上传
评论
收藏 61KB ZIP 举报
温馨提示
YOLOv5-压缩2021.10.30 复现TPH-YOLOv52021.10.31完成替换主干网为Ghostnet2021.11.02 完成替换主干网为Shufflenetv22021.11.05 完成替换主干网为Mobilenetv3Small2021.11.10 完成EagleEye对YOLOv5系列剪枝支持2021.11.14 完成MQBench对YOLOv5系列量化支持2021.11.16完成替换主干网为EfficientNetLite-02021.11.26 完成替换主干网为PP-LCNet-1x2021.12.12完成SwinTrans-YOLOv5(C3STR)2021.12.15 完成瘦身对YOLOv5系列剪枝支持要求pip install -r requirements.txtYOLO 的多主干替代1、基础模型在 Visdrone 数据集上训练(输入大小为 608)不。 模型 地图 地图@50 参数(米) 每秒千兆次浮点运算1 YOLOv5n 13.0 26.20 1.78 4.22 YOLOv5s 18
资源推荐
资源详情
资源评论
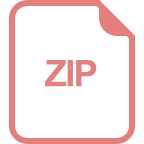
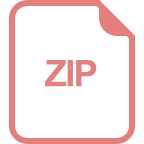
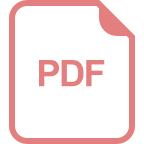
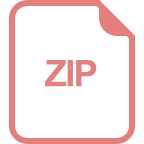
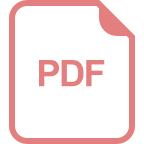
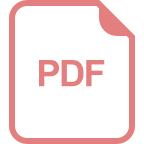
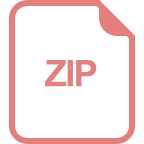
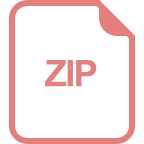
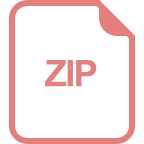
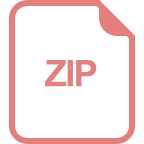
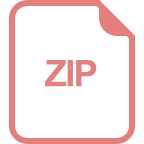
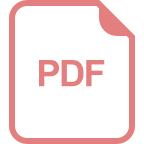
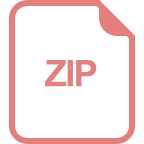
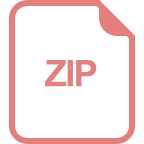
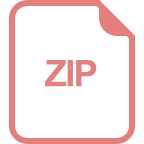
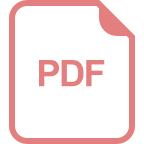
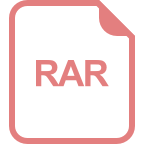
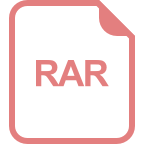
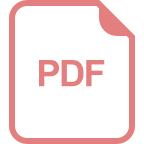
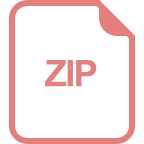
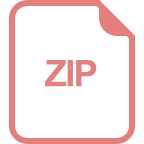
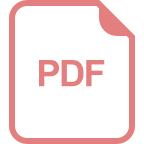
收起资源包目录

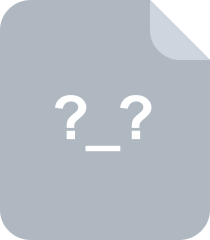
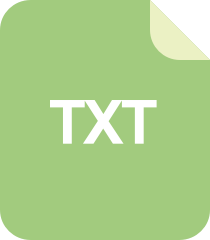

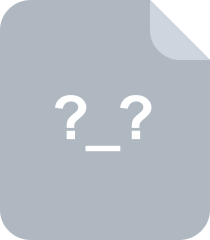
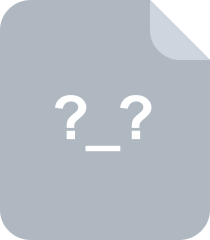
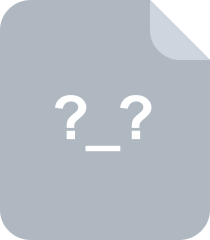
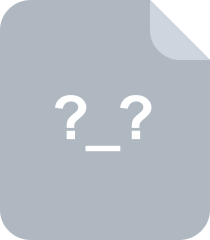
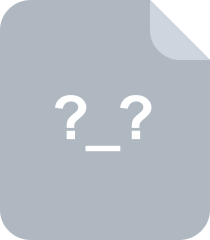
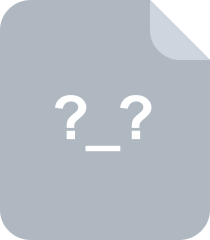
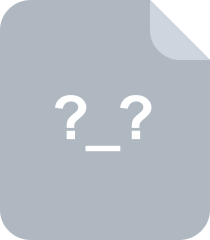
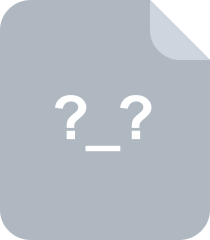
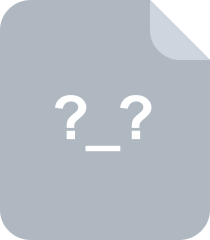

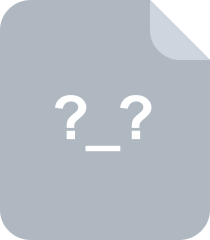
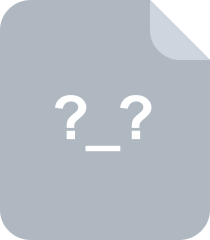
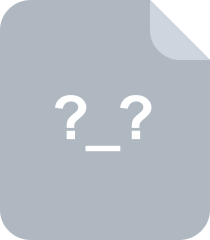
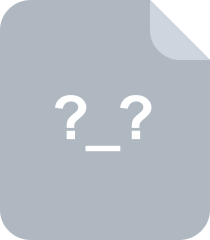
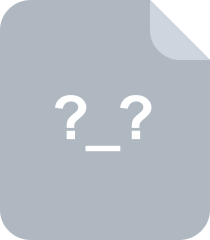
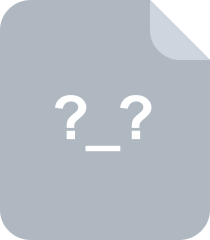
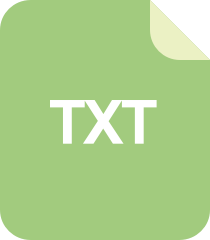
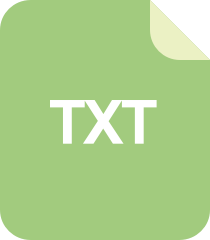
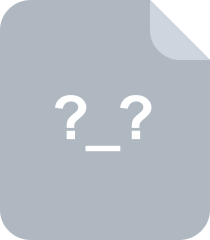
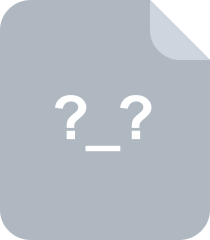
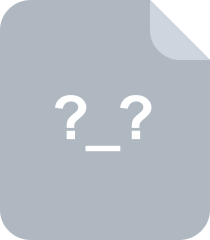
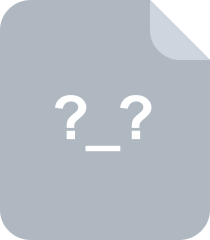
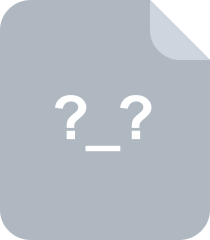
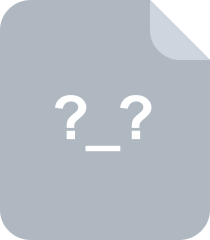
共 25 条
- 1
资源评论
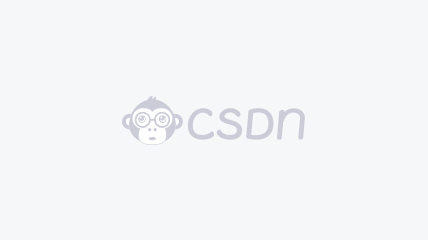

徐浪老师
- 粉丝: 8102
- 资源: 8096
上传资源 快速赚钱
我的内容管理 展开
我的资源 快来上传第一个资源
我的收益
登录查看自己的收益我的积分 登录查看自己的积分
我的C币 登录后查看C币余额
我的收藏
我的下载
下载帮助

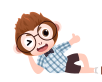
最新资源
- C++类型萃取技术:深入探究与实践指南
- 在 NVIIDIA 1060 上以 37 fps 的速度运行搭载最新 TensorRT6.0 的 YoloV3 .zip
- 202409010304 吴羡 职业生涯规划书.pdf
- 在 NVIDIA Jetson Nano 上使用 Yolov5 和 openCV 进行摄像头安装、软件和硬件设置以及物体检测的简单过程 .zip
- 知识问答中文微调训练数据集
- 微信小程序-毕设基于SSM的项目申报小程序【代码+论文+PPT】
- 数学运算相关的指令微调数据集
- YOLOv3的多尺度预测机制:技术解析与代码实践
- 在 Keras 中使用 YOLO 进行车辆检测的运行速度为 21FPS.zip
- 在 c++ 中部署 yolov5.zip
资源上传下载、课程学习等过程中有任何疑问或建议,欢迎提出宝贵意见哦~我们会及时处理!
点击此处反馈


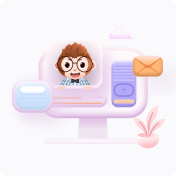
安全验证
文档复制为VIP权益,开通VIP直接复制
