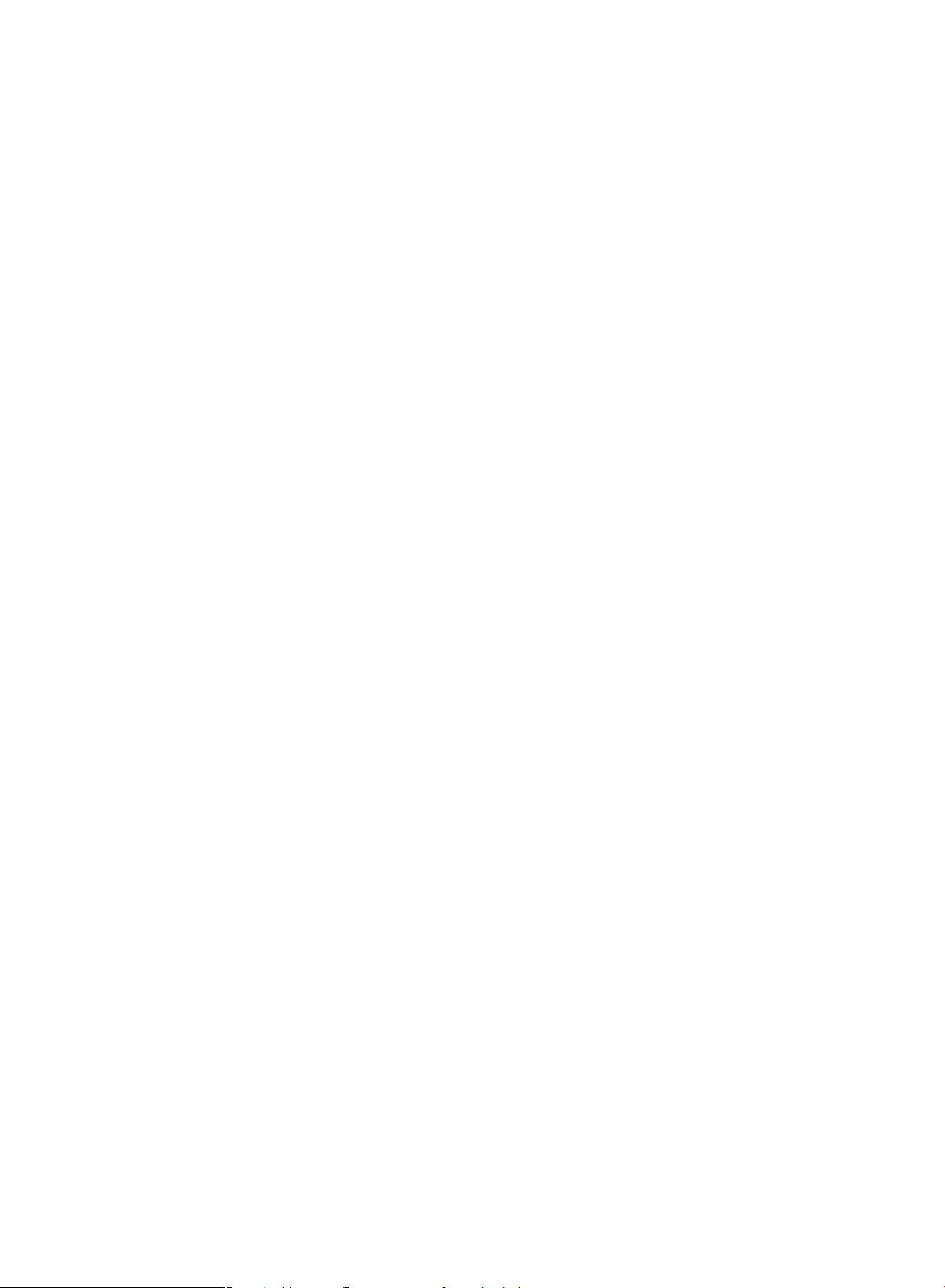
1. Introduction
The spectral kurtosis (SK) was first introduced by Dwyer in [1], as a statistical tool which ‘‘can
indicate not only non-Gaussian components in a signal, but also their locations in the frequency
domain’’. Dwyer initially used it as a complement to the power spectral density, and demonstrated
how it efficiently supplements the latter in problems concerned with the detection of transients in
noisy signals [2,3].
Dwyer originally defined the SK as the normalised fourth-order moment of the real part of the
short-time Fourier transform, and suggested using a similar definition on the imaginary part in
parallel.
Since then, the SK had been seldom brought into play [5], until Pagnan and Ottonello proposed
a modified definition based on the normalised fourth-order moment of the magnitude of the
short-time Fourier transform [6,7]. This led to considerably simplified properties. Pagnan and
Ottonello also showed that the SK could be used as a filter to recover randomly occurring signals
severely corrupted by additive stationary noise.
The SK was lately given a more formal definition in [8] in light of the theory of higher-order
statistics. Capdevielle defined the SK as the normalised fourth-order cumulant of the Fourier
transform, i.e. as a slice of the tricoherence spectrum, and used it as a measure of distance of a
process from Gaussianity. Her definition applied well to stationary signals, but encountered some
difficulties with non-stationary signals. The stationary case was recently investigated in more
depth by Vabrie, who proposed some interesting applications to the characterisation of harmonic
processes [9,10].
There is still a need today for a correct formalisation of the SK of non-stationary processes. We
believe that filling this gap is necessary for the SK to really capture the interest it deserves.
Unfortunately, this task has been hindered by some theoretical difficulties:
how can the SK—which is estimated by time averaging—detect non-stationary signals?
why is the SK—which is inherently a tool for non-Gaussian signals—so well adapted to
characterising non-stationary signals?
can the correct definition of the SK of non-stationary signals be based on the assumption of
circularity, which theoretically holds only for stationary signals?
In this paper, we propose a formalisation of the SK by means of the Wold–Crame
´
r decomposition
of ‘‘conditionally non-stationary’’ (CNS) processes. The paradigm of CNS is a natural idea we
introduce here for convenience to solve the aforementioned difficulties. It basically makes it
possible to establish under which conditions a ‘‘non-stationary’’ process (i) generates a non-
Gaussian distribution, and (ii) can be described by time-averaged—i.e. stationarised—statistics. In
contrast to earlier references, we will also provide an interpretation of the SK as a measure of
temporal dispersion of the time–frequency energy density of a process. This point of view will shed
new light on the comprehension of the SK and on some of its properties.
Overall, this paper brings together a number of fully original results in order to provide a more
comprehensive view of some previously published material. Most of the content of Sections 2
(starting from the concept of conditional non-stationarity) to 5 is new—or at least generalises
earlier works—unless specifically stated otherwise. All the proofs presented in the appendix are
fully original.
ARTICLE IN PRESS
J. Antoni / Mechanical Systems and Signal Processing 20 (2006) 282–307 283