没有合适的资源?快使用搜索试试~ 我知道了~

markb
- 粉丝: 2
- 资源: 2
上传资源 快速赚钱
我的内容管理 展开
我的资源 快来上传第一个资源
我的收益
登录查看自己的收益我的积分 登录查看自己的积分
我的C币 登录后查看C币余额
我的收藏
我的下载
下载帮助

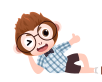
最新资源
- .archivetemp04 - 继承和多态 作业.doc
- 基于运动学车辆模型的开放式驾驶MPC横向控制算法Analyzed commaai Openpilot MPC lateral c
- STM32F103单片机源码STM32-74HC595串转并控制数码管显示
- python语言教程项目案例
- 斐波那契数列java代码 FibonacciProblem
- 服务器的概要介绍与分析
- logseq-linux包
- 蓝色简历首页的微信小程序模板源码
- 基于MPC的仿真轨迹跟踪模块MPC-based Simulink trajectory tracking module
- 递归地求解Fibonacci数列.pdf
资源上传下载、课程学习等过程中有任何疑问或建议,欢迎提出宝贵意见哦~我们会及时处理!
点击此处反馈


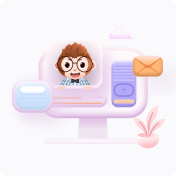
安全验证
文档复制为VIP权益,开通VIP直接复制
