没有合适的资源?快使用搜索试试~ 我知道了~
金融投资组合优化中基于一致模糊环境的多期选择模型研究及其应用
1.该资源内容由用户上传,如若侵权请联系客服进行举报
2.虚拟产品一经售出概不退款(资源遇到问题,请及时私信上传者)
2.虚拟产品一经售出概不退款(资源遇到问题,请及时私信上传者)
版权申诉
0 下载量 148 浏览量
2025-01-06
20:48:38
上传
评论
收藏 1.47MB PDF 举报
温馨提示
内容概要:本文探讨了在一致性模糊环境下多期组合投资的选择问题,针对未来收益不确定性以及投资者对股市的态度异质性进行建模,提出了两个将财富最大化与风险最小化作为目标函数的一致模糊投资组合多周期优化模型。模型不仅考虑动态风险承受能力和预期收益率的变化路径,还综合现实交易成本等因素以模拟真实股票市场的复杂情况。文中提出的方法通过对两组大型数据集的经验研究验证其有效性,并通过多个投资评价指标评估模型性能,证明在大部分评估标准上优于传统静态模型。最终研究为不同的市场预期提供了长期投资决策的依据,并丰富了不确定条件下行为投资组合选择的研究内容。 适用人群:金融领域的研究人员及从业者,尤其是关注于投资策略制定和投资风险管理的专业人士 使用场景及目标:用于指导多期资产配置的实际操作流程并提供有效的风险管理解决方案;适用于长期投资者需要根据不同市场的反馈调整自身风险偏好的情形。 其他说明:此研究展示了基于最新技术成果的投资组合优化方法,在处理金融市场的不确定性方面有显著成效,但同时也承认某些局限如需要更多不同类型市场数据的支持。此外还强调未来可以进一步改进模型的方向比如纳入更多样化的评价目标(如投资效率)及考虑可信度测度的应用来增强模型的稳健性和适应范围。
资源推荐
资源详情
资源评论
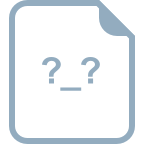
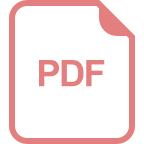
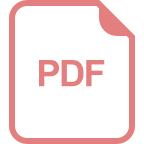
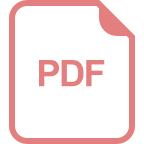
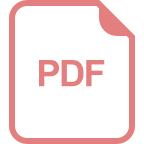
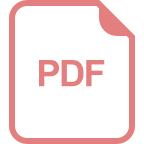
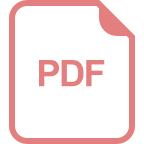
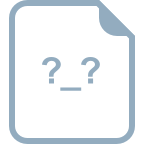
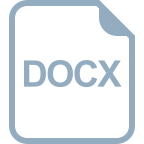
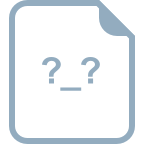
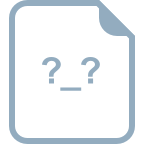
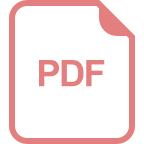
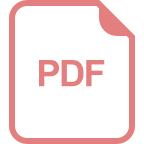
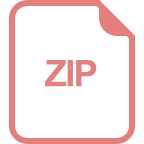
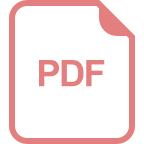
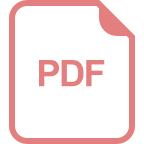
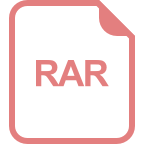
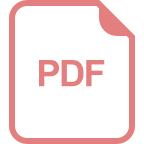
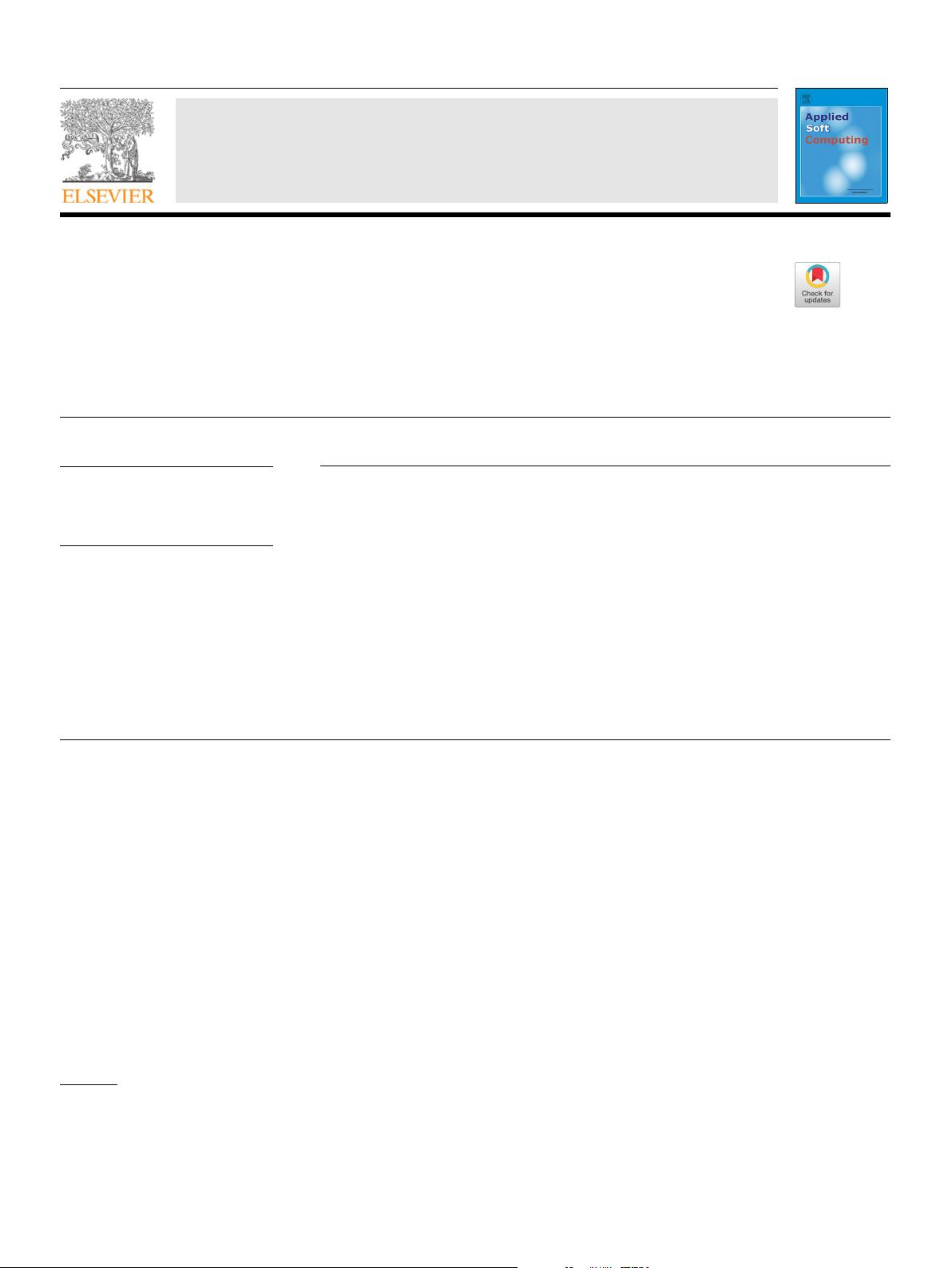
Applied Soft Computing 114 (2022) 108104
Contents lists available at ScienceDirect
Applied Soft Computing
journal homepage: www.elsevier.com/locate/asoc
Multi-period portfolio selection under the coherent fuzzy
environment with dynamic risk-tolerance and expected-return levels
Xiaomin Gong
a,b
, Liangyu Min
c,d,
∗
, Changrui Yu
d
a
Institute of Fintech, Shanghai University of Finance and Economics, Shanghai 200433, China
b
School of Finance, Shanghai University of Finance and Economics, Shanghai 200433, China
c
Faculty of Business Information, Shanghai Business School, 201400, China
d
School of Information Management and Engineering, Shanghai University of Finance and Economics, Shanghai 200433, China
a r t i c l e i n f o
Article history:
Received 13 January 2021
Received in revised form 12 September 2021
Accepted 20 October 2021
Available online 25 November 2021
Keywords:
Multi-period portfolio selection
Coherent fuzzy numbers
Heterogeneity
Dynamic risk-tolerance/expected-return
level
a b s t r a c t
We discuss the portfolio selection problems in which the uncertainty of future returns and the
heterogeneity of investor attitudes towards the stock market (optimistic–pessimistic–neutral) are
captured by coherent fuzzy numbers. Two coherent fuzzy multi-period portfolio selection models
are developed from the perspectives of wealth maximization and risk minimization. Given that the
constraint levels regarding risk and return of the current period tend to be influenced by the outcome
of the previous period, the dynamic risk-tolerance and expected-return levels are integrated into
the portfolio modeling. Practical constraints and transaction costs are also taken into account, which
enables the models more effective and lifelike in simulating the real-world trading of the stock market.
The empirical studies based on two large data sets are presented to illustrate the applicability of
the proposed models. To survey the models’ performance, several portfolio evaluation criteria are
used to conduct out-of-sample analysis. The results show outstanding performance of the presented
models with dynamic strategies over conventional ways (static risk-tolerance and expected-return
levels) on most of the indicators. This research offers references for investors with different attitudes to
make long-term investment decisions, and is an effective supplement to behavioral portfolio selection
research based on bounded rationality under uncertainty.
© 2021 Elsevier B.V. All rights reserved.
1. Introduction
Constructing the optimal portfolios by the selection of proper
financial assets is among the most vital and concerned decision-
making problems in the realm of financial management and
financial engineering, and it is also a basic mission for individual
and institutional investors. The pioneering work of Markowitz [1],
generally known as the mean–variance model, offers a brand
new perspective for the research of portfolio selection and lays
the foundation of modern portfolio theory. Mean–variance model
describes portfolio selection as a bi-objective optimization prob-
lem, with the aim of delivering an appropriate trade-off be-
tween portfolio return and risk [2–4]. In the model, portfolio
return is defined as the weighted linear combination of returns
of constituent assets, and portfolio risk is quantified by portfolio
variance, namely the function of correlations in assets compo-
nents [5]. Based on Markowitz’s framework, the literature has
seen a proliferation of several extended portfolio selection models
∗
Corresponding author.
E-mail addresses: gongxiaomin961@163.com (X. Gong),
minux@163.sufe.edu.cn (L. Min), yu.changrui@mail.shufe.edu.cn (C. Yu).
that are more aligned with the real-world portfolio management
requirements by considering the complex realistic constraints
and criteria [6–12].
Significantly, the traditional portfolio optimization models fo-
cus solely on a single period, that is, only the one-shot decision
is provided from the beginning to the end of the investment
period. However, such strategies cannot satisfy practical con-
cerns well since the real stock market is diverse and imperfect,
and investors will adjust their portfolio structure flexibly in re-
sponse to the dynamic and changeful market environment. It
is necessary to cast off the traditional modeling path and shift
the focus of the portfolio selection studies to multiple periods
to provide the best investment decisions for different periods.
Solving the multi-period portfolio problems also has a signifi-
cant history of research [13]. In particular, based on the mean–
variance framework, Çelikyurt and Özekici [14] presented some
mathematical optimization models under stochastic markets for
multi-period investment decisions. Fu et al. [15] explored the
mean–variance portfolio selection problem under continuous-
time conditions considering the constraint of higher borrow-
ing rates. Rocha and Kuhn [16] proposed a multistage model
in the mean–variance framework for portfolio management of
https://doi.org/10.1016/j.asoc.2021.108104
1568-4946/© 2021 Elsevier B.V. All rights reserved.
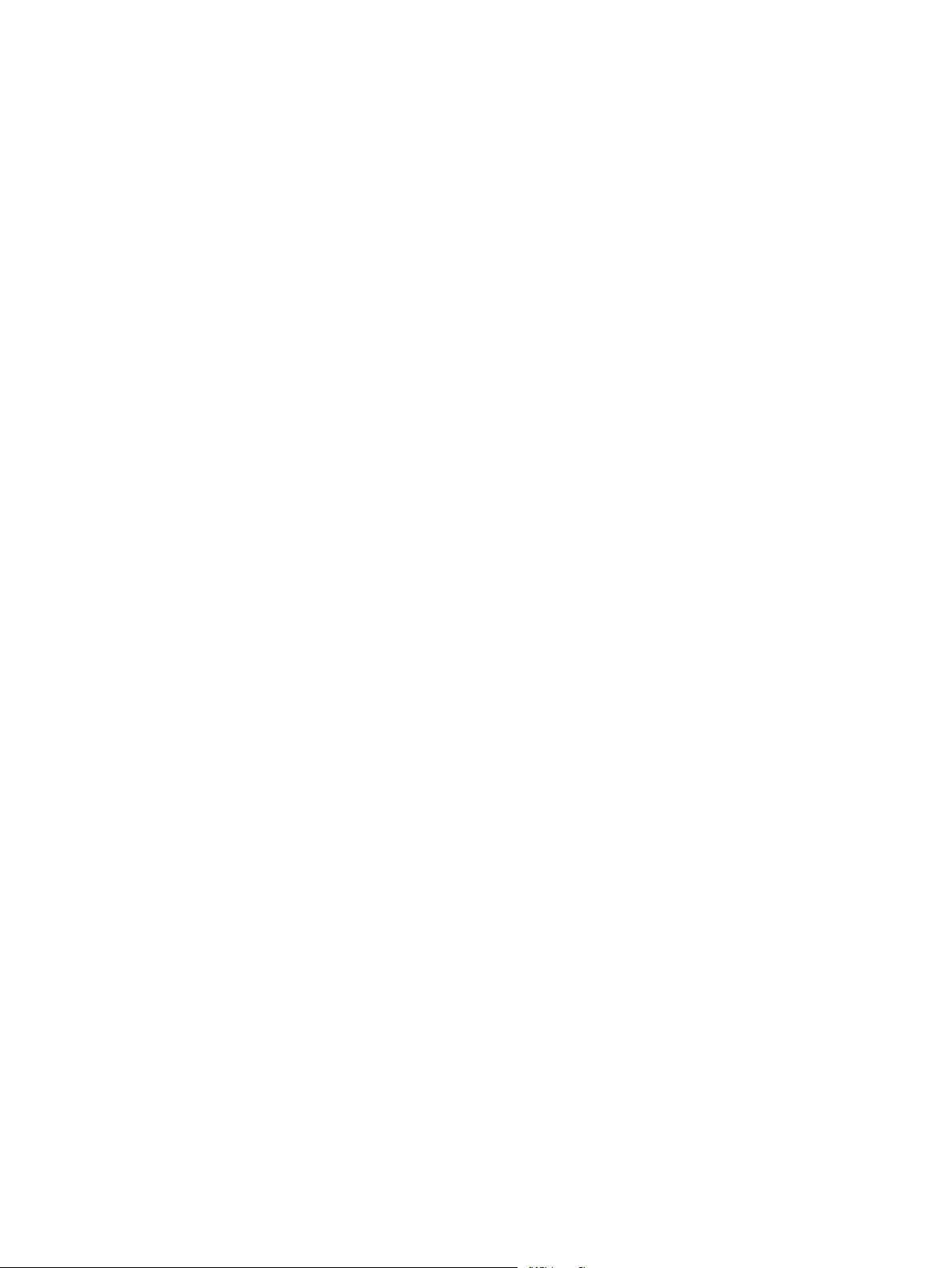
X. Gong, L. Min and C. Yu Applied Soft Computing 114 (2022) 108104
the power market. Wu and Li [17] established a multi-period
portfolio selection model in the mean–variance framework with
regime-switching, which assumes that cash flows are random.
Fu et al. [18] studied the optimal asset allocation issue under
the continuous-time regime-switching market. Cui et al. [19]
developed the time-consistent and self-coordination strategies
to address the time inconsistency of the multi-period mean-
Conditional Value-at-Risk portfolio selection problem.
However, the above-cited works on multi-period asset alloca-
tion are based on the assumption that future return information
associated with various assets could be exactly reflected through
past performance (data) and characterized by random variables.
In fact, financial markets involve high uncertainty, and there are
various factors that deviate from probabilistic analysis, such as
social, economic, political, investor’s psychology, and data uncer-
tainty, which usually affect the market and cannot be resolved
with probability theory [20–22]. Concerned with this issue, an
increasing number of scholars turn their attention to the fuzzy set
theory [23], which is referred to as an appropriate and powerful
means for processing uncertainty and vagueness that are the
inherent nature of any financial market [24]. Research on single-
period/multi-period portfolio decision and optimization in the
context of fuzzy logic is enriching and extending gradually. For in-
stance, Mehlawat et al. [25] developed a multi-objective portfolio
selection model integrating higher moments and efficiency. The
asset data in their work are modeled with (λ, ρ) interval-valued
fuzzy numbers to reflect the nondeterminacy as well as fuzziness
of the real-life stock market. Mansour et al. [26] presented a
financial portfolio selection method considering return, risk, and
liquidity criteria, which determines the possibility distributions of
fuzzy return according to the information provided by the deci-
sion environment. Liu et al. [27] studied the issue of fuzzy multi-
period portfolio selection that involves various aspects, such as
return, transaction cost, risk, and skewness. Liu and Zhang [28]
examined what time-varying loss aversion does to fuzzy multi-
period portfolio decision. Borovička [29] proposed a complicated
and rational procedure for portfolio formulation by utilizing the
fuzzy multiobjective programming technique with a unique in-
teractive program. Taking stock returns as fuzzy variables, Guo
et al. [30] formulated the fuzzy multi-period portfolio selection
approach in the view of different investment horizons. Liagk-
ouras and Metaxiotis [31] examined the multi-period portfolio
optimization problem involving transaction costs, in which the
asset-related information in every investment period is expressed
as trapezoidal fuzzy variables.
In real financial markets, investors exhibit substantial hetero-
geneity [32]. The heterogeneity of investor attitudes (optimistic–
pessimistic–neutral) undoubtedly plays a significant role in the
price movement of individual stocks and is revealed through the
trading behavior [33]. Even faced with the same historical data
of a stock, investors may hold different attitudes (someone may
be optimistic about it, someone maybe not) [34]. Investors will
show different preferences under the influence of their attitudes,
which is in favor of understanding portfolio allocation decisions.
Gupta et al. [35] also mentioned that the stock market would not
be operational if every investor had a consistent attitude towards
every asset. Based on this fact, how to integrate investor attitudes
into the portfolio selection model reasonably and effectively is
a fascinating issue and has taken on new significance in the
context of a remarkable function on behavioral finance in recent
years. Laengle et al. [36] focused on the mean–variance portfo-
lio selection model with the ordered weighted average, which
takes into account varying levels of optimism and pessimism,
thus providing a novel perspective in analyzing the attitudes
of decision-makers in the process of portfolio selection. Li and
Yi [37] developed a new fuzzy number with an adaptive index,
known as the coherent fuzzy number, which can overestimate
the membership degrees for the favorable returns and meanwhile
underestimate those for the unfavorable returns for the optimistic
investors by appropriate transformation, and reverse the trans-
formation for the pessimistic. Therefore, coherent fuzzy numbers
not only retain the advantages of traditional fuzzy numbers in
expressing and dealing with uncertainty, but also provide greater
flexibility in modeling the heterogeneity of investor attitudes in
the investment process as closely as possible. Although coherent
fuzzy numbers have more flexibility and applicability compared
with traditional fuzzy numbers, the research on portfolio selec-
tion under a coherent fuzzy environment is still in the initial
exploration stage. The models constructed in the above work [37]
are also limited to a single period of asset allocation, which is
not applicable for long-term investment and decision-making. For
the above reasons, this paper will try to introduce coherent fuzzy
numbers into the modeling of multi-period portfolio selection to
describe the possibilistic distribution of asset returns and capture
the attitude characteristics of investors.
With regard to multi-period portfolio optimization, the exist-
ing studies usually treat the risk-tolerance/expected-return level
of the whole investment horizon as a constant, that is, the risk-
tolerance/expected-return threshold in each investment period
does not vary over time. For instance, Zhang and Zhang [38] dealt
with a multi-period mean absolute deviation portfolio selection
problem considering risk control and cardinality constraints in a
fuzzy environment, where the risk control level is an invariant
constant. Mehlawat [39] discussed fuzzy multi-period portfolio
problems that integrate multi-choice aspirations for wealth and
risk respectively, in which the minimum return threshold for
each trading period is also the same. Under the credibility theory
framework, Gupta et al. [35] proposed two multi-period portfolio
optimization models using coherent fuzzy numbers, in which
portfolio risks are quantified by mean absolute semi-deviation
and Conditional Value-at-Risk. These two models show great
advantages in multiple aspects, but the risk tolerance level over
the entire investment horizon remains fixed under the same risk
measure. Furthermore, Liu and Zhang [40] formulated a fuzzy
mean-semivariance portfolio selection model based on possibility
theory, which takes the terminal wealth and cumulative risk
as the main goal of decision-making. In their model, although
the risk-tolerance and expected-return levels of each period are
inconsistent, they are given directly and full of subjectivity. Of
note, the optimal solution of the discussed portfolio selection
problems relies closely on the selection of the upper limit of risk
and the lower limit of return [41]. Considering that in the real
world, the risk-tolerance and expected-return levels are suscep-
tible to the outcomes from the portfolio in the previous period,
it is necessary to design a reasonable updating process for these
values rather than setting them statically or subjectively. Specif-
ically, if the portfolio profit in period t is larger (smaller) than
the original expectation on return, the investor will feel that
the portfolio has performed well (poorly), and then his/her risk-
tolerance level is increased (decreased) in constructing portfolios
in the following period, that is, investor is more inclined to risk-
seeking (risk aversion). According to this train of thought, Wang
et al. [42] concentrated on the problems of multi-period portfolio
selection with dynamic risk and expected-return levels in the
mean–variance frame to discuss the actual behaviors of traders
and performance of portfolios.
To the best of our knowledge, apart from the above research,
portfolio selection with dynamic risk-tolerance/expected-return
level is still uncharted territory. To supply the vacancy in this
research, this paper attempts to establish two fuzzy optimization
models subject to some realistic constraints for multi-period
portfolio selection under the coherent fuzzy environment, in
2
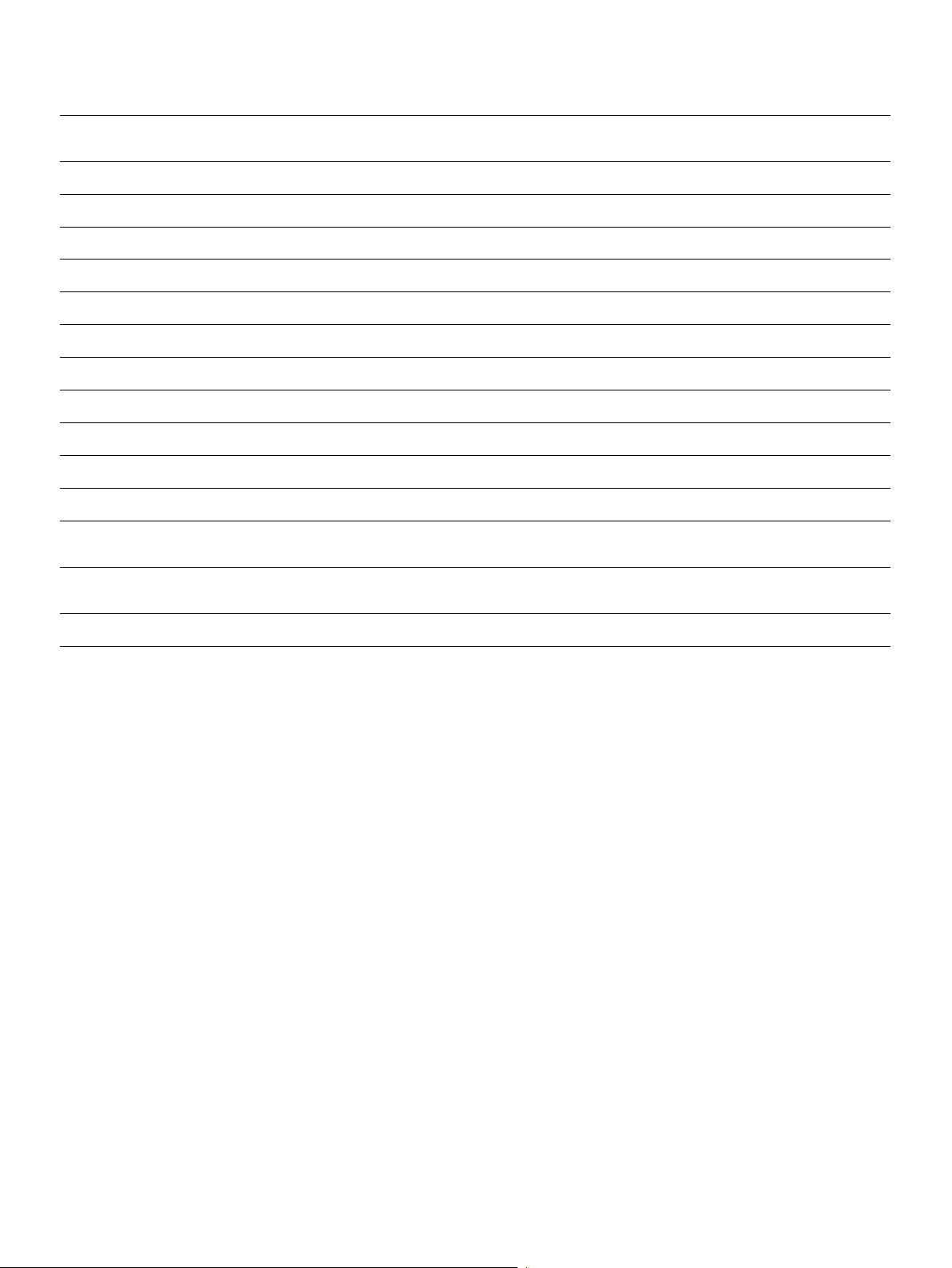
X. Gong, L. Min and C. Yu Applied Soft Computing 114 (2022) 108104
Table 1
Comparison with existing literature on portfolio selection.
Attributes Guo et al.
(2016) [30]
Liagkouras
& Metaxiotis
(2018) [31]
Zhou et al.
(2018) [34]
Zhang &
Zhang (2014)
[38]
Mehlawat
(2016) [39]
Liu & Zhang
(2015) [40]
Li and Yi
(2019) [37]
Gupta et al.
(2020) [35]
Proposed
method
Objective
functions
Linear,
non-linear
Non-linear Linear,
non-linear
Non-linear Linear Linear,
non-linear
Linear,
non-linear
Non-linear Non-linear
Environment Fuzzy Fuzzy Fuzzy Fuzzy Fuzzy Fuzzy Coherent
fuzzy
Coherent
fuzzy
Coherent
fuzzy
Investment
period
Multiple Multiple Single Multiple Multiple Multiple Single Multiple Multiple
Risk measure Fuzzy
variance
Fuzzy variance LAD Absolute
deviation
Entropy Fuzzy
semivariance
Fuzzy
variance
MASD and
CVaR
Fuzzy
variance
Fuzzy
framework
Credibilistic Possibilistic Me measure Possibilistic Credibilistic Possibilistic Possibilistic Credibilistic Possibilistic
Behavioral
factors
× × Conservative
-aggressive
× × × Optimistic–
pessimistic
Optimistic–
pessimistic
Optimistic–
pessimistic
Expected-
return level
Static Invariant Static × Invariant Variant and
given directly
Static × Dynamic
update
Risk-tolerance
level
Static × Static Invariant × Variant and
given directly
Static Invariant Dynamic
update
Transaction
cost
× ×
Full utilization
of capita
×
Cardinality
constraint
× × ×
No short
selling
constraint
× ×
Lower–upper
bounds
constraints
× ,
upper bound
× ×
Number of
data set tested
Three One One One One One Two Two Two
Acronyms-LAD: Lower absolute deviation; Var-OWA: Variance-ordered weighted average; MASD: Mean absolute semi-deviation; CVaR: Conditional Value-at-Risk.
which we expend the risk-tolerance and expected-return levels to
dynamic analysis. These two multi-period portfolio models take
wealth maximization and risk minimization as objective func-
tions, respectively. The uncertainty of the proposed models and
heterogeneity of investor attitudes (optimistic, pessimistic, and
neutral) are modeled by regarding the asset returns as indepen-
dent coherent fuzzy variables. The return and risk of the asset are
quantitatively determined with the aid of the possibilistic mean
and possibilistic variance of coherent fuzzy return, respectively.
The efficient investment strategies are derived via the genetic
algorithm introduced in [40]. Table 1 provides the characteristic
comparison of our research with the existing literature on port-
folio selection. The main contributions in this study include the
following five-folds.
(i) Based on the mean–variance framework and bounded ratio-
nality of investors, we propose two coherent fuzzy multi-period
portfolio selection models, in which uncertain asset information
and the optimistic–pessimistic–neutral attitudes of investors are
characterized by coherent fuzzy numbers. (ii) We consider the
dynamic risk-tolerance and expected-return levels in portfolio
construction by designing corresponding update paths, which
offer avenues in analyzing the impact of the previous investment
results on investors’ decision behavior in the next period. (iii)
Several real-world trading constraints and transaction costs are
included in the presented models. These constraints enable our
models to simulate the stock trading storyline more realistically
and to better satisfy the diversified requirements of investors.
(iv) The empirical study with the in-sample and out-of-sample
analysis is carried out to evaluate the performance of the re-
sulting portfolios. The results on two different data sets show
that the presented models outperform the conventional ways
(static risk-tolerance and expected-return levels) on most of the
evaluation indicators. (v) In contrast to most research on this field
that considers test data between 10 and 20 stocks [39,43–45],
the data sets tested in the current study are relatively larger,
reaching 74 and 101 risky assets, respectively, thereby improving
the authenticity of the examined models.
The remainder of the current paper is structured as follows.
We first review the coherent trapezoidal fuzzy numbers in Sec-
tion 2. In Section 3, two multi-period coherent fuzzy portfolio se-
lection models with dynamic risk-tolerance and expected-return
levels are developed. In Section 4, the numerical experiments are
presented to test the proposed models. A detailed discussion of
the achieved results is also made in this section. The final section
concludes the paper.
2. Preliminaries
The financial market is a time-varying and complex system.
Its significant feature is provided with tremendous uncertainty,
which brings difficulty in accurately estimating future returns
related to assets. Fuzzy logic is considered to be an effective
and appropriate way to express ambiguity and nondeterminacy.
The membership function is the cornerstone of fuzzy set theory,
through which asset returns can be transformed into fuzzy vari-
ables for research. The coherent fuzzy number is an extended
form of traditional fuzzy number. To better understand this paper,
this section introduces the coherent trapezoidal fuzzy number.
3
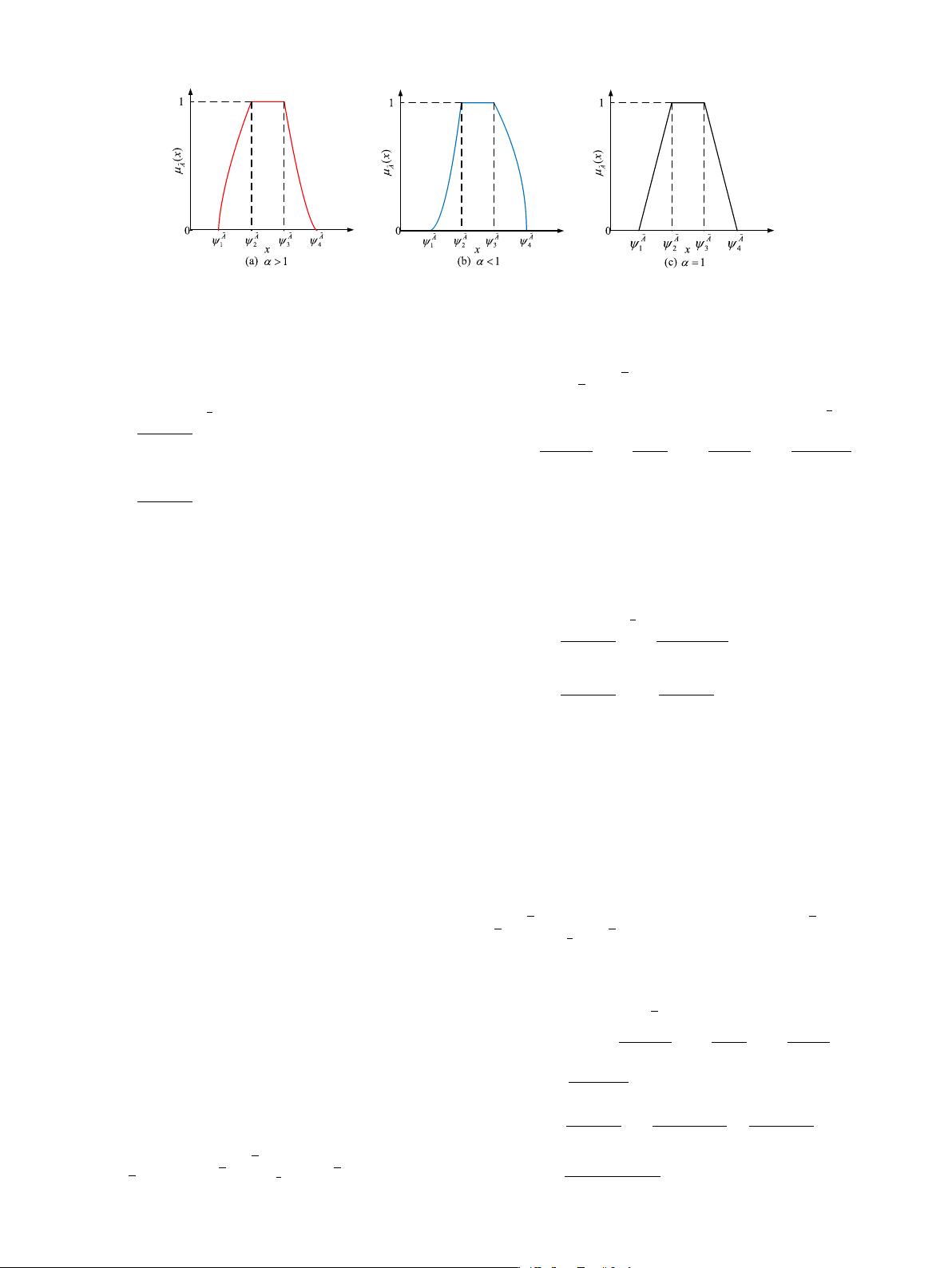
X. Gong, L. Min and C. Yu Applied Soft Computing 114 (2022) 108104
Fig. 1. Possibility distributions of
˜
A = (ψ
˜
A
1
, ψ
˜
A
2
, ψ
˜
A
3
, ψ
˜
A
4
)
α
with α > 1, α < 1, and α = 1.
Definition 1 ([37]). A fuzzy set
˜
A = (ψ
˜
A
1
, ψ
˜
A
2
, ψ
˜
A
3
, ψ
˜
A
4
)
α
is called
a coherent trapezoidal fuzzy number (CTrFN) with core interval
[ψ
˜
A
2
, ψ
˜
A
3
], left endpoint ψ
˜
A
1
, and right endpoint ψ
˜
A
4
. The member-
ship function of
˜
A is denoted as µ
˜
A
(x):
µ
˜
A
(x) =
x − ψ
˜
A
1
ψ
˜
A
2
− ψ
˜
A
1
1
α
, ψ
˜
A
1
< x ≤ ψ
˜
A
2
;
1, ψ
˜
A
2
< x ≤ ψ
˜
A
3
;
ψ
˜
A
4
− x
ψ
˜
A
4
− ψ
˜
A
3
α
, ψ
˜
A
3
< x ≤ ψ
˜
A
4
;
0, else.
(1)
where µ
˜
A
(x) → [0, 1], and α is an adaptive index with α > 0.
˜
A =
(ψ
˜
A
1
, ψ
˜
A
2
, ψ
˜
A
3
, ψ
˜
A
4
)
α
is degenerated into the coherent triangular
fuzzy number in the case of ψ
˜
A
2
= ψ
˜
A
3
.
Assuming that the future return of an asset for portfolio se-
lection is characterized by a CTrFN
˜
A = (ψ
˜
A
1
, ψ
˜
A
2
, ψ
˜
A
3
, ψ
˜
A
4
)
α
, the
interval [ψ
˜
A
2
, ψ
˜
A
3
] is regarded as the most likely return on the
asset, and the intervals [ψ
˜
A
1
, ψ
˜
A
2
] and [ψ
˜
A
3
, ψ
˜
A
4
] represent the un-
favorable and favorable returns respectively. The adaptive index
α is an indicator for measuring investors’ expectations (attitudes).
According to variant values of α, we have the following three
cases: (1) When α > 1, the relatively higher membership degree
is allocated to the unfavorable returns located within [ψ
˜
A
1
, ψ
˜
A
2
],
while the relatively lower membership degree is obtained within
[ψ
˜
A
3
, ψ
˜
A
4
]. Therefore, α > 1 is used as a signal that reflects the
pessimistic attitude of investors (i.e., pessimistic scenario), and
a larger α value states the more pessimistic investor sentiment.
(2) When α < 1, the relatively higher membership degree is
allocated to the attractive returns located within [ψ
˜
A
3
, ψ
˜
A
4
], while
the returns within [ψ
˜
A
1
, ψ
˜
A
2
] have a smaller membership degree.
Therefore, α < 1 can be treated as a signal reflecting the op-
timistic attitude for investors (i.e., optimistic scenario), and a
smaller α value means investors are more optimistic. (3) When
α = 1, the CTrFN degenerates to the traditional trapezoidal fuzzy
number, and the linearity within [ψ
˜
A
1
, ψ
˜
A
2
] and [ψ
˜
A
3
, ψ
˜
A
4
] is a re-
flection of investor neutrality. The possibility distributions of the
CTrFN in the above three scenarios are represented graphically in
Fig. 1.
Based on Carlsson and Fullér [46], the possibilistic mean and
variance of the CTrFN can be defined as below.
Definition 2. Let
˜
A = (ψ
˜
A
1
, ψ
˜
A
2
, ψ
˜
A
3
, ψ
˜
A
4
)
α
be a CTrFN with the
membership function µ
˜
A
(x) (see Eq. (1)), the ξ − level set of
˜
A can
be expressed as [
˜
A]
ξ
= [a(ξ ), a(ξ )], where a(ξ ) = ψ
˜
A
1
+ (ψ
˜
A
2
−
ψ
˜
A
1
)ξ
α
and a(ξ ) = ψ
˜
A
4
−(ψ
˜
A
4
−ψ
˜
A
3
)ξ
1
α
. Then, the possibilistic mean
of
˜
A can be given by:
E(
˜
A) =
1
0
ξ
a(ξ) + a(ξ )
dξ
=
1
0
ξ
ψ
˜
A
1
+ (ψ
˜
A
2
− ψ
˜
A
1
)ξ
α
+ ψ
˜
A
4
− (ψ
˜
A
4
− ψ
˜
A
3
)ξ
1
α
dξ
=
α
2(α + 2)
ψ
˜
A
1
+
1
α + 2
ψ
˜
A
2
+
α
2α + 1
ψ
˜
A
3
+
1
2(2α + 1)
ψ
˜
A
4
(2)
Li and Yi [37] define the possibility density function of
˜
A =
(ψ
˜
A
1
, ψ
˜
A
2
, ψ
˜
A
3
, ψ
˜
A
4
)
α
, which is stated as:
f
˜
A
(x) = µ
˜
A
(x)
µ
′
˜
A
(x)
=
x − ψ
˜
A
1
ψ
˜
A
2
− ψ
˜
A
1
2
α
−1
1
k(ψ
˜
A
2
− ψ
˜
A
1
)
, ψ
˜
A
1
< x < ψ
˜
A
2
;
ψ
˜
A
4
− x
ψ
˜
A
4
− ψ
˜
A
3
2α−1
k
ψ
˜
A
4
− ψ
˜
A
3
, ψ
˜
A
3
< x < ψ
˜
A
4
;
0, else;
(3)
where f
˜
A
(x) satisfies: f
˜
A
(x) ≥ 0 and
+∞
−∞
f
˜
A
(x)dx = 1.
Based on f
˜
A
(x), the possibilistic mean of
˜
A can also be ex-
pressed as E(
˜
A) =
+∞
−∞
xf
˜
A
(x)dx (see [37]), and the corresponding
result is the same as that of Eq. (2).
Definition 3. Let
˜
A = (ψ
˜
A
1
, ψ
˜
A
2
, ψ
˜
A
3
, ψ
˜
A
4
)
α
be a CTrFN with the
membership function µ
˜
A
(x), the ξ − level set of
˜
A is [
˜
A]
ξ
=
[a(ξ), a(ξ )], where a(ξ ) = ψ
˜
A
1
+ (ψ
˜
A
2
− ψ
˜
A
1
)ξ
α
and a(ξ ) = ψ
˜
A
4
−
(ψ
˜
A
4
− ψ
˜
A
3
)ξ
1
α
. Then, we have the lower and upper possibilistic
semivariances of
˜
A (Va
−
(
˜
A) and Va
+
(
˜
A)) with the following forms:
Va
−
(
˜
A) = 2
1
0
ξ
E(
˜
A) − a(ξ )
2
dξ
= 2
1
0
ξ
α
2(α + 2)
ψ
˜
A
1
+
1
α + 2
ψ
˜
A
2
+
α
2α + 1
ψ
˜
A
3
+
1
2(2α + 1)
ψ
˜
A
4
−
ψ
˜
A
1
+ (ψ
˜
A
2
− ψ
˜
A
1
)ξ
α
2
dξ
=
ψ
˜
A
3
− ψ
˜
A
2
2
+
α(ψ
˜
A
2
− ψ
˜
A
1
)
2(α + 2)
+
(ψ
˜
A
4
− ψ
˜
A
3
)
2(2α + 1)
2
+
α
2
(ψ
˜
A
2
− ψ
˜
A
1
)
2
(α + 1)(α + 2)
2
(4)
4
剩余18页未读,继续阅读
资源评论
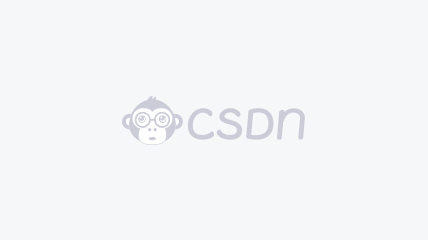

pk_xz123456
- 粉丝: 2863
- 资源: 4045
上传资源 快速赚钱
我的内容管理 展开
我的资源 快来上传第一个资源
我的收益
登录查看自己的收益我的积分 登录查看自己的积分
我的C币 登录后查看C币余额
我的收藏
我的下载
下载帮助

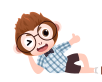
最新资源
- opc da 转opc ua 、opc 隧道软件 注意:这是两个软件,安装在同一个机器上,可以实现opc da转 opc ua 安装在两个计算机上就可以实现opc tunnel功能,不需要配置d
- java Springboot网上音乐商城(源码+sql+论文)-springboot毕业项目,适合计算机毕-设、实训项目、大作业学习.zip
- 档案管理系统_g2p7x--论文-springboot毕业项目,适合计算机毕-设、实训项目、大作业学习.zip
- ffmpeg and EasyDarwin
- elk-demo 代码例子,123 45678
- 大学生创新创业训练项目管理系统设计与实现-springboot毕业项目,适合计算机毕-设、实训项目、大作业学习.zip
- 大健康养老公寓管理系统_to14d-springboot毕业项目,适合计算机毕-设、实训项目、大作业学习.zip
- 大学新生报到系统的设计与实现-springboot毕业项目,适合计算机毕-设、实训项目、大作业学习.zip
- 基于Hadoop的高校固定资产管理系统研究与实现_hot14-springboot毕业项目,适合计算机毕-设、实训项目、大作业学习.zip
- 基于Java的大学生迎新系统-springboot毕业项目,适合计算机毕-设、实训项目、大作业学习.zip
- JavaSpringboot+vue图书购物商城管理系统(源码+sql+论文)-springboot毕业项目,适合计算机毕-设、实训项目、大作业学习.rar
- JavaSpringboot学生教务管理系统(源码+sql+文档)-springboot毕业项目,适合计算机毕-设、实训项目、大作业学习.zip
- 个性化电影推荐系统-springboot毕业项目,适合计算机毕-设、实训项目、大作业学习.zip
- 儿童性教育网站-springboot毕业项目,适合计算机毕-设、实训项目、大作业学习.zip
- JavaSpringboot+vur前后端分离党员信息管理系统(源码+sql+论文)-springboot毕业项目,适合计算机毕-设、实训项目、大作业学习.rar
- 430大神asp.net基于三层商品进销存管理系统毕业课程源码设计
资源上传下载、课程学习等过程中有任何疑问或建议,欢迎提出宝贵意见哦~我们会及时处理!
点击此处反馈


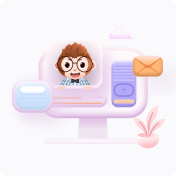
安全验证
文档复制为VIP权益,开通VIP直接复制
