没有合适的资源?快使用搜索试试~ 我知道了~
温馨提示
内容概要:本文利用自然语言处理技术和机器学习模型(如XGBoost和SHAP),探索福州市57个传统村落的建成环境对游客情感的影响。研究表明,开放度、绿色植物比例和色彩复杂度对游客的情感体验有显著正向影响,而地面曝光度和其他因素则有负面影响。通过对不同变量的交互分析,揭示了这些环境特征对游客情感的具体机制。 适合人群:城乡规划师、景观设计师、旅游研究人员以及相关领域的学者和从业人员。 使用场景及目标:本文的研究成果可为传统村落的可持续发展提供科学依据,特别是在提升游客情感体验方面。适用于优化传统村落的空间配置、改善基础设施建设、增强生态体验等方面。 其他说明:本研究采用了大量的社会媒体数据和图像数据进行量化分析,通过多种方法验证了研究成果的可靠性。同时,也为其他地区的农村环境评估提供了参考框架。-可实现的-有问题请联系博主,博主会第一时间回复!!!
资源推荐
资源详情
资源评论
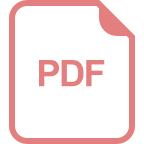
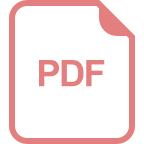
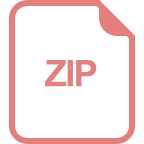
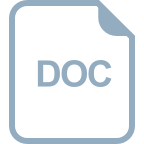
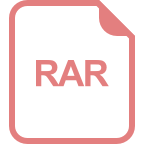
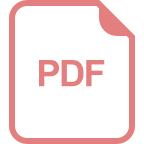
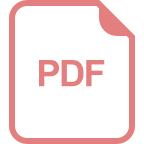
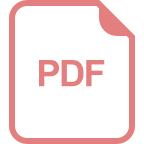
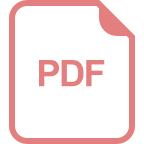
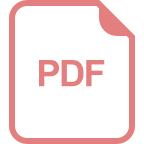
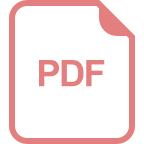
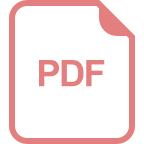
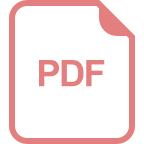
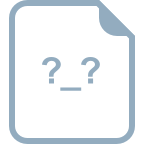
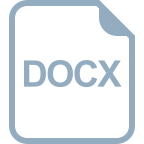
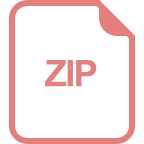
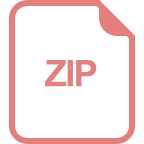
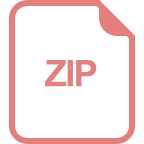
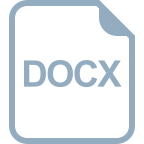
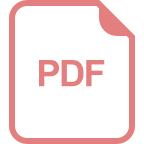
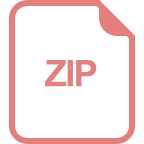
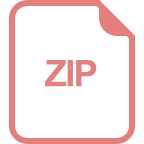
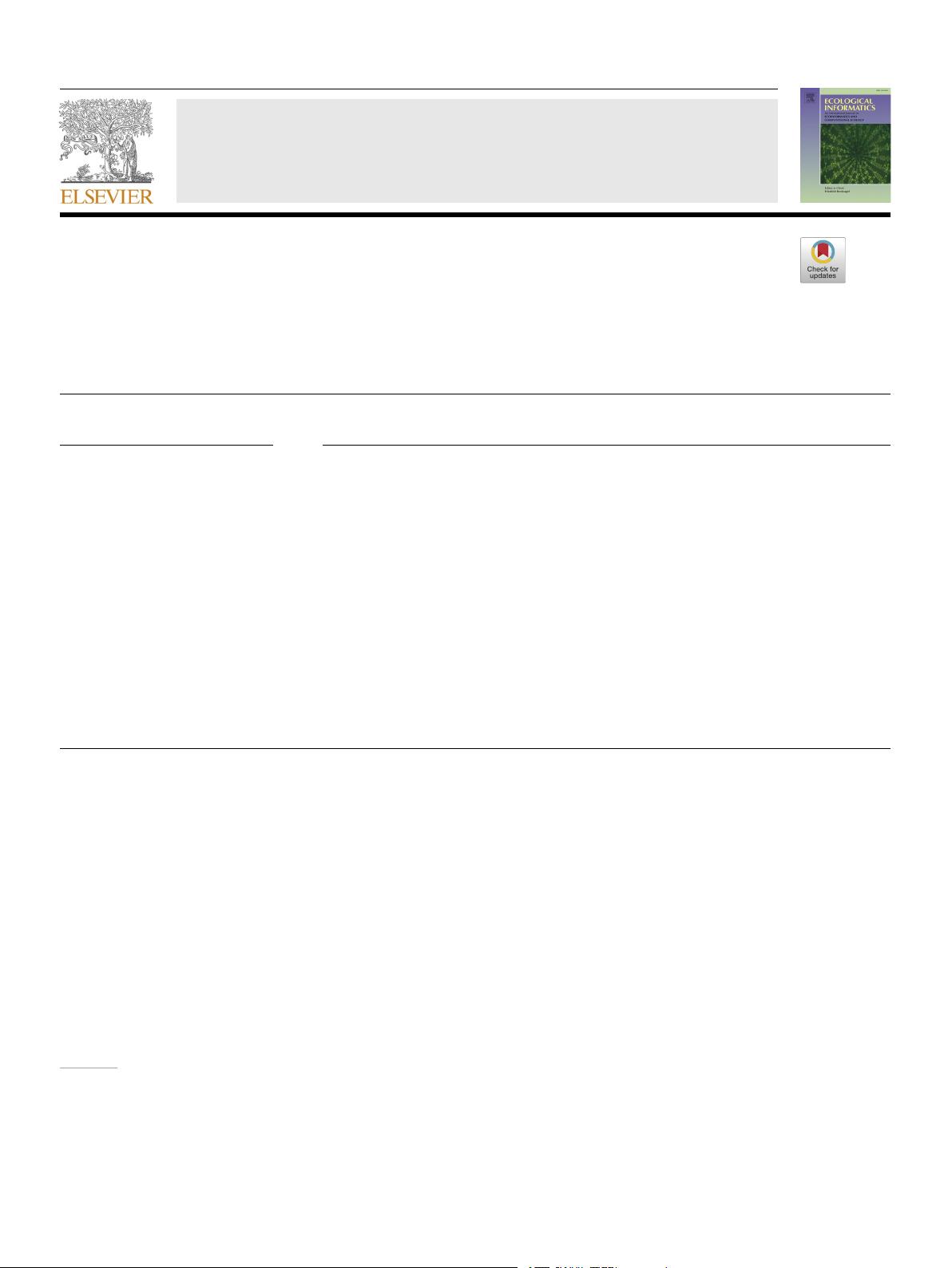
Ecological Informatics 80 (2024) 102465
Available online 11 January 2024
1574-9541/© 2024 The Authors. Published by Elsevier B.V. This is an open access article under the CC BY-NC license (http://creativecommons.org/licenses/by-
nc/4.0/).
Exploring the association between the built environment and positive
sentiments of tourists in traditional villages in Fuzhou, China
Zhengyan Chen , Honghui Yang , Yishan Lin , Jiahui Xie , Yuanqin Xie , Zheng Ding
*
College of Arts College of Landscape Architecture, Fujian Agriculture and Forestry University, Fuzhou 350100, Fujian Province, China
ARTICLE INFO
Keywords:
Traditional village
Tourist sentiments
Built environment
Natural language processing
Machine learning
ABSTRACT
Promoting positive emotional experiences for tourists is crucial for sustaining development in rural areas.
However, existing research has limited focus on the rural built environment, particularly in developing a
framework to evaluate environmental sentiment on a small to medium scale with detailed indicators. This study
addresses this gap by examining the impact of the rural built environment on tourists’ emotions. Natural Lan-
guage Processing (NLP) technologies are employed to analyze web text data and determine the average senti-
ment index for traditional villages in Fuzhou, China. Additionally, data on the built environment were acquired
through the HRnet segmentation model and Matlab. To assess the association between environmental indicators
and the sentiment index, we used eXtreme Gradient Boosting (XGBoost), the SHapley Additive exPlanation
(SHAP) model, and ArcMap software. The study demonstrated that (1) the spatial distribution of the average
sentiment index was signicant. Houfu Village (9.91), Qianhu Village (9.88), and Ximen Village (9.75) had the
highest scores, while Doukui Village (− 0.85), Jiji Village (0.2), and Qiaodong Village (0.55) had the lowest. (2)
The indicators that have the most signicant impact on sentiment are Openness, Greenness, and Color
Complexity, with a contribution value above 0.7—followed by Enclosure, Visual Entropy, and Ground Exposure,
with a contribution between 0.5 and 0.7. Furthermore, analyzing the interaction mechanism of the indicators
showed a non-linear relationship. The environmental characteristics associated with high emotional index scores
are openness in the range of 0.2 to 0.5, greenness in the range of 0.4 to 0.6, and color complexity in the range of
0.3 to 0.5. This study provides observations pertinent to the sustainable development of traditional village en-
vironments. The ndings contribute to an understanding of how these environmental elements might be effec-
tively designed to improve tourists’ sentiment in rural settings.
1. Introduction
In recent years, rural development in China has undergone signi-
cant changes, primarily due to national policies focusing on the National
Agrotourism Demonstration Site, Beautiful Countryside Construction,
and Rural Revitalization strategy, thereby elevating tourism to a critical
developmental factor in traditional villages (Dai et al., 2023; Wang
et al., 2023). Rural tourism has emerged as a sustainable livelihood
strategy (Su et al., 2019), playing a pivotal role in reshaping rural
economies, social structures, and spatial dynamics. It has become a vital
conduit for alleviating rural poverty and enhancing built environments.
Notably, both personal and environmental factors greatly affect tourists’
overall emotional experiences during their travels (Chen, 2022).
Furthermore, positive tourism experiences can bolster sustainable con-
sumption motivations in rural tourism (Chen et al., 2023a), thereby
inuencing the economic earnings and sustainability of the destinations.
Consequently, scholars are increasingly exploring the interplay between
tourism emotions and the rural built environment, recognizing its
signicance.
Emotions, serving as adaptive responses to events in daily life, reveal
the psychological changes experienced in response to stimuli (Ekman,
1992). Anderson and Smith (2001) establish a link between emotions
and space, suggesting that spatial emotions illustrate social relations.
This concept forms the basis of affective geography, which examines the
relationship between emotions and physical environments. Kim and
Fesenmaier (2015) have shown that varying environments signicantly
impact human emotions and subsequently affect the overall experience
of an activity. However, the quality of the built environment has a sig-
nicant impact on public depression and other sentiment symptoms,
according to the 2016 edition of the China Family Panel Studies (Liu
* Corresponding author.
E-mail address: 000Q151039@fafu.edu.cn (Z. Ding).
Contents lists available at ScienceDirect
Ecological Informatics
journal homepage: www.elsevier.com/locate/ecolinf
https://doi.org/10.1016/j.ecoinf.2024.102465
Received 24 November 2023; Received in revised form 5 January 2024; Accepted 6 January 2024
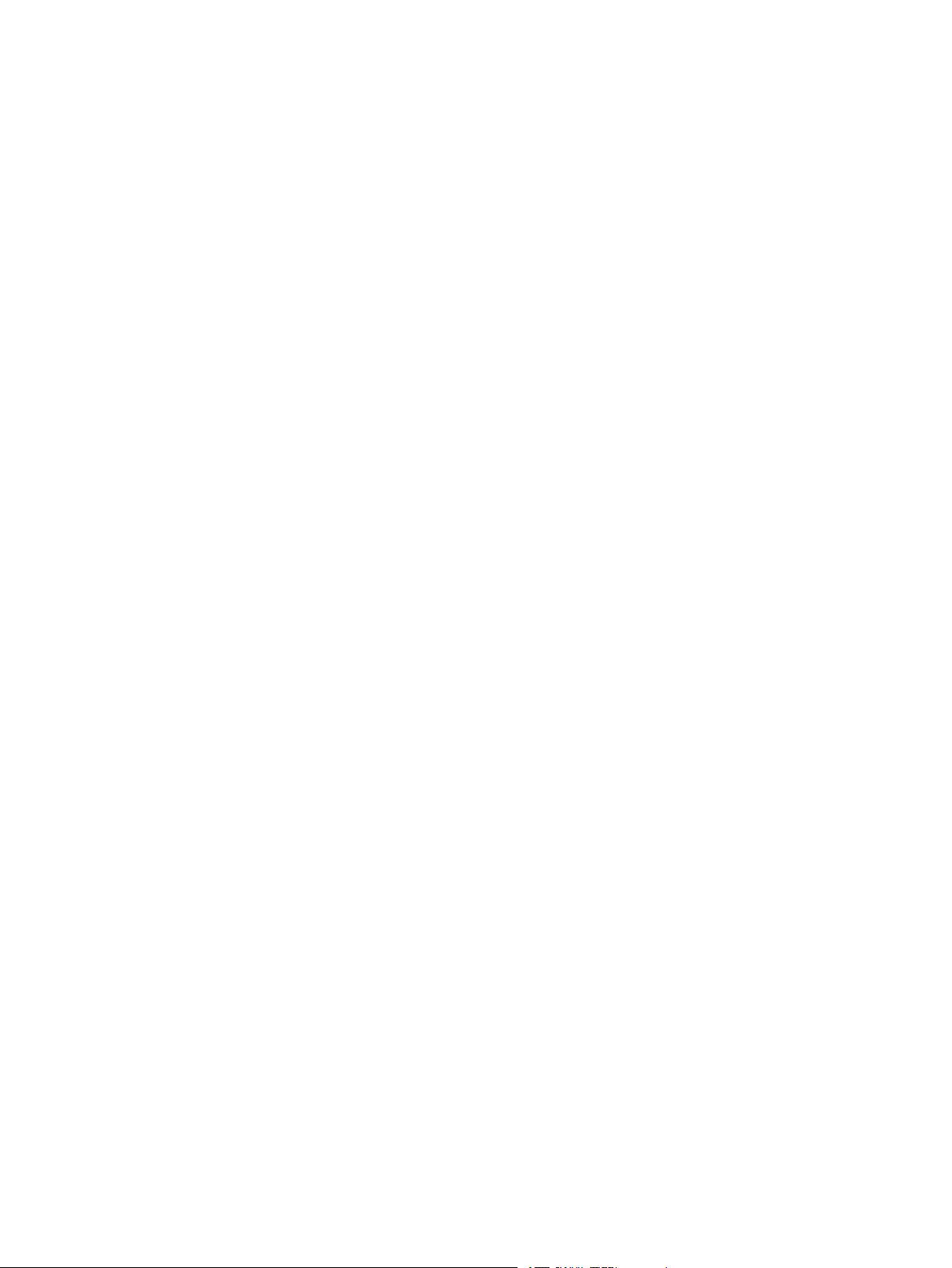
Ecological Informatics 80 (2024) 102465
2
et al., 2021). Therefore, promoting positive emotions among individuals
in the environment is crucial for achieving sustainable development in
planning and design (Rui, 2023). This initiative also supports the United
Nations World Tourism Organization’s (UNWTO) Sustainable Develop-
ment Goals for Sustainable Cities and Communities as well as Health and
Well-Being (United Nations, 2015).
Environmental impact assessment is one of the most critical com-
ponents in sustainability surveys (Nabavi-Pelesaraei et al., 2021), In the
early stages of researching the correlation between environment and
emotion, traditional methods like questionnaires, interviews, and eld
surveys had notable limitations. Recently, however, the rise of social
networking platforms has made social media data a signicant source
for understanding consumer travel choices (Ghermandi et al., 2023; Shin
et al., 2019). This data is easy to obtain and has a high potential for
utilization. For example, Wang et al. (2021) developed an analytical
framework to examine geographically themed sentiment using social
media data. Studies like those by Liu et al. (2019) and Yu et al. (2023)
have shown that analyzing tourists’ emotions can provide insights into
destination image scores and satisfaction. The wealth of User Generated
Content (UGC) available on social media platforms provides researchers
with a valuable resource for studying various aspects of human-
environment interactions. These resources facilitate research into the
dynamics of human-nature relationships (Chai-allah et al., 2023a),
monitoring environmental states (Hartmann et al., 2022), understand-
ing environmental perception (Madjar et al., 2023), and quantifying
landscape preferences (Chai-allah et al., 2023b). These ndings under-
score the value of social media data in evaluating crowd sentiment and
aspects of the built environment. However, prior research has predom-
inantly leveraged the substantial volume and ready accessibility of so-
cial media data for quantitative analyses of extensive urban settings.
This focus has often overshadowed the potential applicability of such
data in examining smaller and medium-scale areas. Moreover, there has
been a notable paucity of studies dedicated to investigating the built
environment within rural contexts. In addition, previous related studies
have mainly used broad area quantitative data, such as standardized
questionnaire (Nabavi-Pelesaraei and Damgaard, 2023), Street Map
View Imag (SVI), Normalized Difference Vegetation Index (NDVI), land
use, and POI (Point of Interest) data for analysis (Chen et al., 2023e; Ma,
2023; Richards and Lavorel, 2022). Moreover, there is a requirement to
explore the spatial correlation between User-generated content and
quantitative data, such as Points of Interest (POIs), road networks, and
land use, reveal a gap in the current data framework for urban imagery,
public perception, and micro-scale spatial assessment research. This gap
emphasizes the need for a more rened and matched data framework in
these domains.
In response to the increasing demand for processing multi-source
data in built environment research, researchers have increasingly
adopted machine learning models. Notably, the open-source eXtreme
Gradient Boosting (XGBoost) model, rooted in GBDT, has gained
prominence for its robust explanatory and predictive capabilities
(Todorovic et al., 2023; T. Chen and Guestrin, 2016). Employed across
various elds, XGBoost has been particularly useful in addressing
complex problems (Chen et al., 2023c). In recent years, scholars in
various elds have begun employing the XGBoost model and integrating
multi-source data for built environment studies. Their research spans
topics like spatial vitality (Liu et al., 2023; Zhang et al., 2023a), spatial
heterogeneity (J. Zhang et al., 2023b), landscape impacts on ecological
vulnerability (Xu et al., 2022), and implications for human health
(Zhang et al., 2022c). This method is extensively utilized in large-scale
research, notably in urban and transportation planning sectors. How-
ever, the development of a standardized assessment indicator system for
this methodology is still in progress. More research indicators with high
emotional matching with tourists need to be added in the experiment.
The utilization of macroscopic data, including Points of Interest (POIs)
and remotely sensed imagery, is often constrained by the lack of detailed
information about the built environment (Cao et al., 2018; Huang et al.,
2022). This limitation is particularly challenging in traditional villages,
characterized by complex planning scenarios. To delve deeper into how
the environment inuences individual emotions, specic environmental
indicators must be integrated into the research framework. The combi-
nation of the XGBoost model and the SHAP interpretation method fa-
cilitates the understanding of interactions among various variables. It
explains the extent to which detailed environmental indicators
contribute to the model, enabling effective evaluation of small- to
medium-sized spaces. The integration of visual and textual features in
studies establishes a novel framework (Rogers, 2021), addressing the
gap observed in previous research between macro-environmental data
and human emotional responses. This innovative approach opens new
avenues for future research, suggesting a more holistic methodology
that can bridge the divide between large-scale environmental data and
nuanced emotional experiences in built environments.
Fuzhou, as one of the pioneering cities in urban agriculture, is
renowned for its rich cultural heritage and abundant traditional village
resources (Wang et al., 2022b). However, the city faces challenges of
urban-rural development disparities and environmental issues due to
rapid industrialization (Chen et al., 2023b). In response to these chal-
lenges and in alignment with China’s “14th Five-Year Plan” and the
Five-Year Action Plan for Rural Habitat Improvement and Enhancement
(2021–2025), Fuzhou City is undertaking signicant measures. These
measures aim to address environmental concerns in traditional villages,
fostering sustainable rural development (Hao et al., 2023; Wei et al.,
2021). This study examines the correlation between built environment
variables and tourists’ emotions in the countryside by using social media
data and machine learning methods. Specically, the study focuses on
57 traditional villages in Fuzhou City, China. The experimental steps are
as follows: (1) Quantitatively analyze the text data and pictures obtained
from Dianping, Ctrip, and Weibo platforms by cleaning and organizing
them. (2) Score social media data for villages and determine the average
sentiment index using NLP technology based on LSTM and CNN. (3)
Organize corresponding image datasets into text data, quantify them
using semantic segmentation based on Hrnet and image complexity
calculation based on Matlab, and visualize the average quantied data
of different villages using ArcMap software. (4) The dataset was divided
into a training set and a test set before constructing the XGBoost model.
(5) The SHAP method was utilized to examine how elements of the built
environment, as captured in photographs, inuence the sentiment
index. This research provides a scientic foundation for rening rural
spatial congurations in Fujian, enriching tourist experiences in tradi-
tional villages, and acting as a guideline for the sustainable development
of other traditional villages.
2. Data and methodology
2.1. Study area
Fuzhou, the capital of Fujian Province, China, holds a pivotal posi-
tion in politics, economy, and culture, being one of the rst coastal
development cities. This experiment’s research scope entails examining
traditional villages found throughout Fuzhou City, with a representation
of their distribution depicted in Fig. 1. The city, divided into six central
districts (Gulou, Taijiang, Cangshan, Jinan, Mawei, and Changle) as well
as seven counties (Fuqing, Minhou, Lianjiang, Luoyuan, Minqing,
Yongtai, and Pingtan). The climate in Fuzhou is a typical subtropical
monsoon climate characterized by warmth and humidity throughout the
year, abundant sunshine, and precipitation. Fuzhou faces challenges of
uneven spatial patterns and traditional village landscape resources due
to its complex topography and geomorphology. (Pengyi, 2011). Tradi-
tional villages in Fuzhou lack adaptation to evolving production and
living requirements, necessitating professional environmental planning
guidance to prevent environmental decay and loss of historical and
cultural heritage (Ou and Xiao, 2020). This study, therefore, chose
Fuzhou City as a test site to provide recommendations for enhancing the
Z. Chen et al.
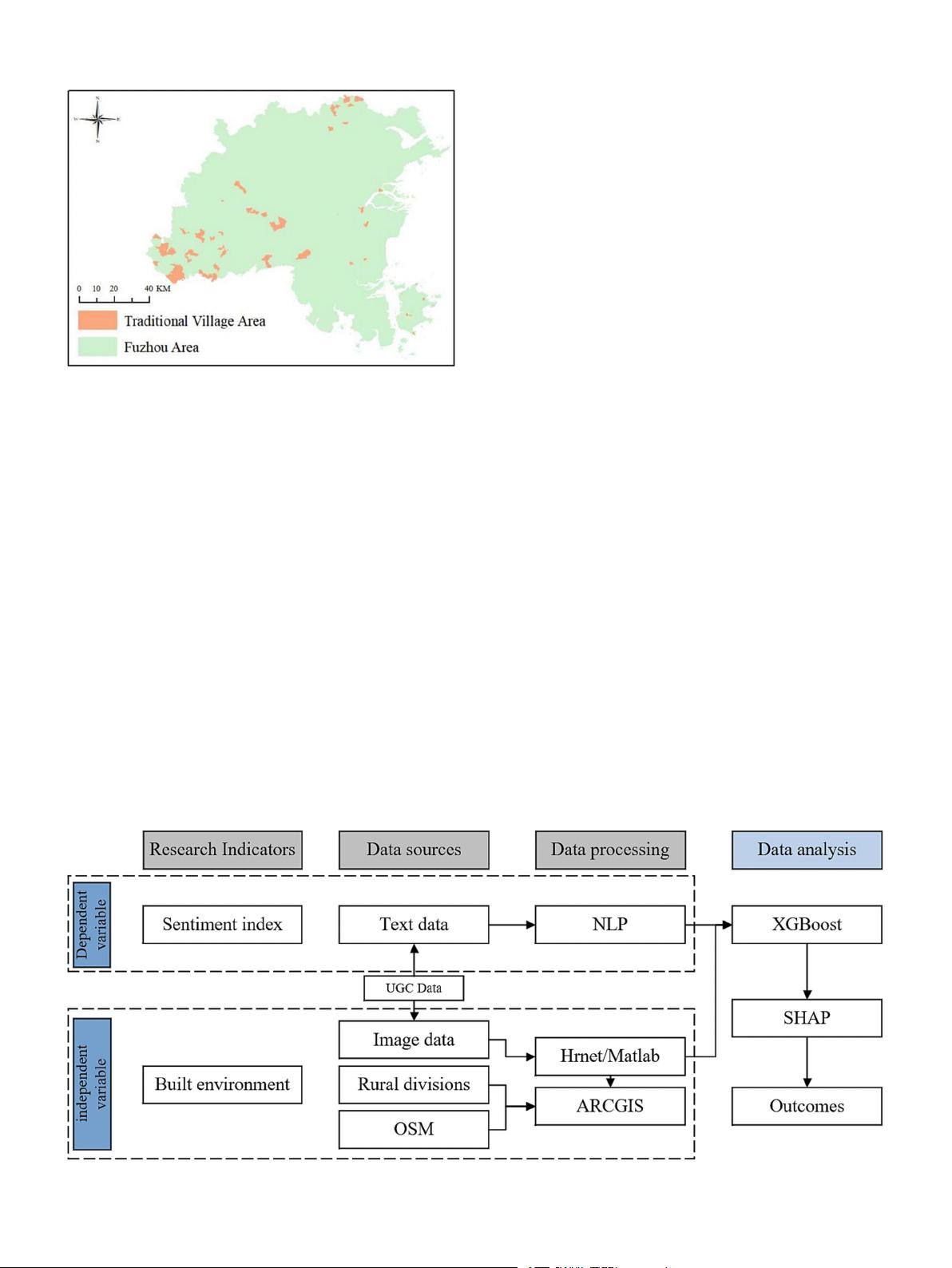
Ecological Informatics 80 (2024) 102465
3
emotional environment of traditional villages.
2.2. Theoretical framework
The experimental design is illustrated in Fig. 2. Our study aims to
explore the correlation between constructed environments and emotions
in rural areas. To achieve this, we propose utilizing a machine learning
and NLP-based evaluation model with three main stages. Firstly, we
extract text and photo information from relevant social media data. The
sentiment index is selected as the dependent variable, while the inde-
pendent variable comprises built environment data calculated during
subsequent experiments. Secondly, the data is sorted and cleaned before
conducting photo data calculations through a semantic segmentation
model based on Hrnet, used for identifying landscape elements in the
photographs. The visual and color complexity of the images is computed
using Matlab. The text data is evaluated using the NLP model of LSTM
with CNN framework pre-trained using Ernie to score sentiment indices.
Finally, the independent variables derived are matched with the
response variables to create an XGBoost model. The SHAP method is
then employed to interpret the calculations. The ndings are visualized
and analyzed through the ArcMap software.
2.3. Data collection
The data regarding traditional villages presented in this study is
derived from the 2023 list published by the Ministry of Housing and
Urban-Rural Development and other pertinent departments in China,
encompassing a total of 57 traditional villages. The social media data
were collected from Weibo (https://weibo.com/), Dianping (https:
//www.dianping.com/), and Ctrip (https://you.ctrip.com/) through
web crawlers. We used specic keywords associated with the traditional
villages to obtain information. We retrieved time information from
comments with images posted sometime between September 2018 and
September 2023. We collected information such as posting ID, text in-
formation, corresponding image URL, and the number of retweets. After
gathering and arranging data from 57 villages, The data cleaning process
ensured the removal of irrelevant information, maintaining the accuracy
and objectivity of experimental results.
2.4. Selection of variables
Based on the perspective of the impact of the built environment on
human emotions in traditional villages, this study integrates previous
studies on the impact of the built environment on human emotions
(Asgarzadeh et al., 2014; Kang et al., 2020; Xu et al., 2023). The in-
dicators were selected by combining the characteristics of traditional
villages, the practical needs of the study, and the availability of data.
Greenness, Openness, and Aquatic rate were selected as natural in-
dicators, while Enclosure, Pedestrian proportion, and Transportation
were chosen as human indicators among the built environment in-
dicators. Additionally, to further examine the impact of roads on peo-
ple’s emotions, Ground exposure and Paving degree were included in
the measurement of human indicators. Previous studies have yet to fully
consider the potential inuence of landscape features on visual quality
(Qi et al., 2023). As a result, this study will investigate how measurable
visual stimuli in the environment affect human emotions. For this pur-
pose, visual sensory metrics, such as Entropy value and color
complexity, were combined (Stamps, 2004; Montero et al., 2020). The
relevant meaning of each indicator and the quantication method are
shown in Table 1.
The semantic segmentation model based on Hrnet quanties various
aspects of the built environment data, including Greenness, Openness,
Enclosure, Ground exposure, Paving degree, Aquatic rate, Pedestrian
proportion, and Transportation. The Entropy value and Color
complexity are computed using MATLAB, and the Sentiment index is
Fig. 1. Study area.
Fig. 2. Theoretical framework.
Z. Chen et al.
剩余14页未读,继续阅读
资源评论
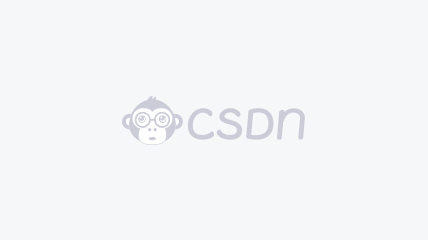

pk_xz123456
- 粉丝: 2598
- 资源: 3652

下载权益

C知道特权

VIP文章

课程特权

开通VIP
上传资源 快速赚钱
我的内容管理 展开
我的资源 快来上传第一个资源
我的收益
登录查看自己的收益我的积分 登录查看自己的积分
我的C币 登录后查看C币余额
我的收藏
我的下载
下载帮助

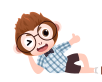
最新资源
资源上传下载、课程学习等过程中有任何疑问或建议,欢迎提出宝贵意见哦~我们会及时处理!
点击此处反馈


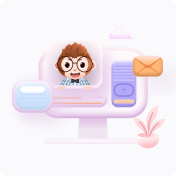
安全验证
文档复制为VIP权益,开通VIP直接复制
