# How Do Vision Transformers Work?
[[paper](https://openreview.net/forum?id=D78Go4hVcxO), [arxiv](https://arxiv.org/abs/2202.06709), [poster](https://github.com/xxxnell/how-do-vits-work-storage/blob/master/resources/how_do_vits_work_poster_iclr2022.pdf), [slide](https://github.com/xxxnell/how-do-vits-work-storage/blob/master/resources/how_do_vits_work_talk.pdf)]
This repository provides a PyTorch implementation of ["How Do Vision Transformers Work? (ICLR 2022 Spotlight)"](https://openreview.net/forum?id=D78Go4hVcxO) In the paper, we show that the success of multi-head self-attentions (MSAs) for computer vision is ***NOT due to their weak inductive bias and capturing long-range dependency***.
In particular, we address the following three key questions of MSAs and Vision Transformers (ViTs):
***Q1. What properties of MSAs do we need to better optimize NNs?***
A1. MSAs have their pros and cons. MSAs improve NNs by flattening the loss landscapes. A key feature is their data specificity (data dependency), not long-range dependency. On the other hand, ViTs suffers from non-convex losses.
***Q2. Do MSAs act like Convs?***
A2. MSAs and Convs exhibit opposite behaviorsâe.g., MSAs are low-pass filters, but Convs are high-pass filters. It suggests that MSAs are shape-biased, whereas Convs are texture-biased. Therefore, MSAs and Convs are complementary.
***Q3. How can we harmonize MSAs with Convs?***
A3. MSAs at the end of a stage (not a model) significantly improve the accuracy. Based on this, we introduce *AlterNet* by replacing Convs at the end of a stage with MSAs. AlterNet outperforms CNNs not only in large data regimes but also in small data regimes.
ð Let's find the detailed answers below!
### I. What Properties of MSAs Do We Need to Improve Optimization?
<p align="center">
<img src="resources/vit/loss-landscape.png" style="width:90%;">
</p>
MSAs improve not only accuracy but also generalization by flattening the loss landscapes. ***Such improvement is primarily attributable to their data specificity, NOT long-range dependency*** ð± On the other hand, ViTs suffers from non-convex losses. Their weak inductive bias and long-range dependency produce negative Hessian eigenvalues in small data regimes, and these non-convex points disrupt NN training. Large datasets and loss landscape smoothing methods alleviate this problem.
### II. Do MSAs Act Like Convs?
<p align="center">
<img src="resources/vit/fourier.png" style="width:90%;">
</p>
MSAs and Convs exhibit opposite behaviors. Therefore, MSAs and Convs are complementary. For example, MSAs are low-pass filters, but Convs are high-pass filters. Likewise, Convs are vulnerable to high-frequency noise but that MSAs are vulnerable to low-frequency noise: it suggests that MSAs are shape-biased, whereas Convs are texture-biased. In addition, Convs transform feature maps and MSAs aggregate transformed feature map predictions. Thus, it is effective to place MSAs after Convs.
### III. How Can We Harmonize MSAs With Convs?
<p align="center">
<img src="resources/vit/architecture.png" style="width:90%;">
</p>
Multi-stage neural networks behave like a series connection of small individual models. In addition, MSAs at the end of a stage (not the end of a model) play a key role in prediction. Based on these insights, we propose design rules to harmonize MSAs with Convs. NN stages using this design pattern consists of a number of CNN blocks and one (or a few) MSA block. The design pattern naturally derives the structure of the canonical Transformer, which has one MLP block for one MSA block.
<br />
<p align="center">
<img src="resources/vit/alternet.png" style="width:90%;">
</p>
Based on these design rules, we introduce AlterNet ([code](https://github.com/xxxnell/how-do-vits-work/blob/transformer/models/alternet.py)) by replacing Conv blocks at the end of a stage with MSA blocks. ***Surprisingly, AlterNet outperforms CNNs not only in large data regimes but also in small data regimes***, e.g., CIFAR. This contrasts with canonical ViTs, models that perform poorly on small amounts of data. For more details, see below (["How to Apply MSA to Your Own Model"](#how-to-apply-msa-to-your-own-model) section).
This repository is based on [the official implementation of "Blurs Behaves Like Ensembles: Spatial Smoothings to Improve Accuracy, Uncertainty, and Robustness"](https://github.com/xxxnell/spatial-smoothing). In this paper, we show that a simple (non-trainable) 2 â 2 box blur filter improves accuracy, uncertainty, and robustness simultaneously by ensembling spatially nearby feature maps of CNNs. MSA is not simply generalized Conv, but rather a generalized (trainable) blur filter that complements Conv. Please check it out!
## Getting Started
The following packages are required:
* pytorch
* matplotlib
* notebook
* ipywidgets
* timm
* einops
* tensorboard
* seaborn (optional)
We mainly use docker images `pytorch/pytorch:1.9.0-cuda11.1-cudnn8-runtime` for the code.
See [```classification.ipynb```](classification.ipynb) ([Colab notebook](https://colab.research.google.com/github/xxxnell/how-do-vits-work/blob/transformer/classification.ipynb)) for image classification. Run all cells to train and test models on CIFAR-10, CIFAR-100, and ImageNet.
**Metrics.** We provide several metrics for measuring accuracy and uncertainty: Acuracy (Acc, â) and Acc for 90% certain results (Acc-90, â), negative log-likelihood (NLL, â), Expected Calibration Error (ECE, â), Intersection-over-Union (IoU, â) and IoU for certain results (IoU-90, â), Unconfidence (Unc-90, â), and Frequency for certain results (Freq-90, â). We also define a method to plot a reliability diagram for visualization.
**Models.** We provide AlexNet, VGG, pre-activation VGG, ResNet, pre-activation ResNet, ResNeXt, WideResNet, ViT, PiT, Swin, MLP-Mixer, and Alter-ResNet by default. timm implementations also can be used.
<details>
<summary>
Four pretrained models for CIFAR-100 are also provided: <a href="https://github.com/xxxnell/how-do-vits-work-storage/releases/download/v0.1/resnet_50_cifar100_691cc9a9e4.pth.tar">ResNet-50</a>, <a href="https://github.com/xxxnell/how-do-vits-work-storage/releases/download/v0.1/vit_ti_cifar100_9857b21357.pth.tar">ViT-Ti</a>, <a href="https://github.com/xxxnell/how-do-vits-work-storage/releases/download/v0.1/pit_ti_cifar100_0645889efb.pth.tar">PiT-Ti</a>, and <a href="https://github.com/xxxnell/how-do-vits-work-storage/releases/download/v0.1/swin_ti_cifar100_ec2894492b.pth.tar">Swin-Ti</a>. We recommend using <a href="https://github.com/rwightman/pytorch-image-models">timm</a> for ImageNet-1K (e.g., please refer to <code><a href="https://github.com/xxxnell/how-do-vits-work/blob/transformer/fourier_analysis.ipynb">fourier_analysis.ipynb</a></code>).
</summary>
<br/>
The codes below are snippets for (a) loading pretrained models and (b) converting them into block sequences.
<br/>
```python
# ResNet-50
import models
# a. download and load a pretrained model for CIFAR-100
url = "https://github.com/xxxnell/how-do-vits-work-storage/releases/download/v0.1/resnet_50_cifar100_691cc9a9e4.pth.tar"
path = "checkpoints/resnet_50_cifar100_691cc9a9e4.pth.tar"
models.download(url=url, path=path)
name = "resnet_50"
model = models.get_model(name, num_classes=num_classes, # timm does not provide a ResNet for CIFAR
stem=model_args.get("stem", False))
map_location = "cuda" if torch.cuda.is_available() else "cpu"
checkpoint = torch.load(path, map_location=map_location)
model.load_state_dict(checkpoint["state_dict"])
# b. model â blocks. `blocks` is a sequence of blocks
blocks = [
model.layer0,
*model.layer1,
*model.layer2,
*model.layer3,
*model.layer4,
model.classifier,
]
```
```python
# ViT-Ti
import copy
import timm
import torch
import torch.nn as nn
import models
# a. download and load a pretrained model for CIFAR-100
url = "
没有合适的资源?快使用搜索试试~ 我知道了~
基于Vision Transformer的图像去雾算法研究与实现源码+文档说明(python高分项目).zip
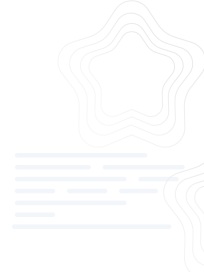
共338个文件
py:204个
png:39个
yaml:16个

1.该资源内容由用户上传,如若侵权请联系客服进行举报
2.虚拟产品一经售出概不退款(资源遇到问题,请及时私信上传者)
2.虚拟产品一经售出概不退款(资源遇到问题,请及时私信上传者)
版权申诉

温馨提示
基于Vision Transformer的图像去雾算法研究与实现源码+文档说明.zip预处理数据 --- 把训练数据图像切分成大小为256*256的小图 下载数据集存放在: /home/dell/桌面/TPAMI2022/Dehazing/#dataset/NH_haze/ 内含两个文件夹:train test 对训练数据集处理: python3 generate_patches_SIDD.py --src_dir /home/dell/桌面/TPAMI2022/Dehazing/#dataset/NH_haze/train --tar_dir /home/dell/桌面/2022毕业设计/Datasets/NH-HAZE/train_patches 2.2 训练代码My_train.py python3 ./My_train.py --arch Uformer --nepoch 270 --batch_size 32 --env My_Infor_CR --gpu '1' --train_ps 128 --train_dir /media/dell/fd6f6662-7e3
资源推荐
资源详情
资源评论
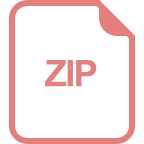
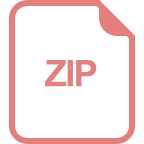
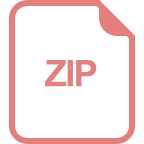
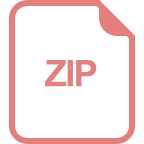
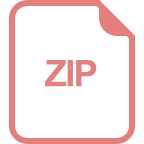
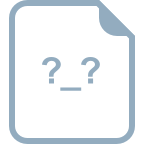
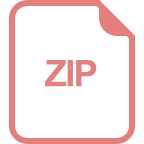
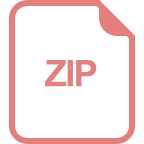
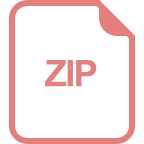
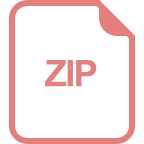
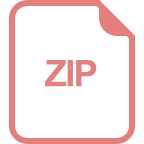
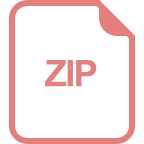
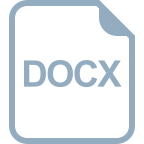
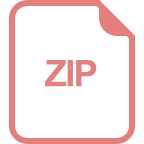
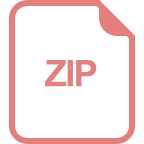
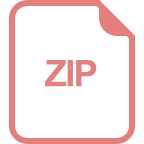
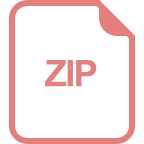
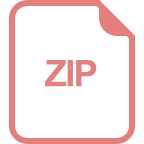
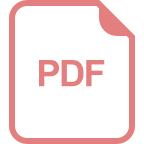
收起资源包目录

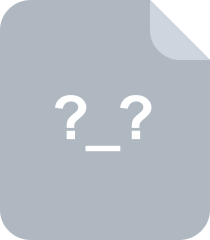
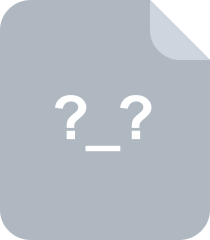
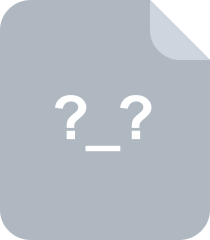
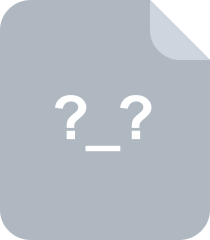
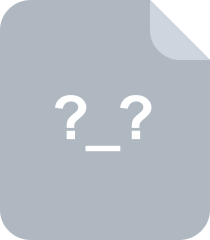
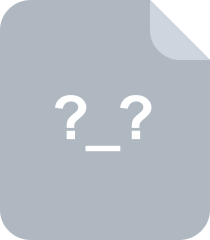
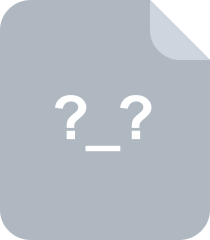
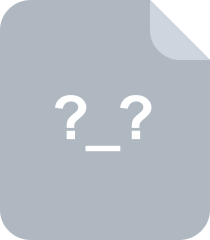
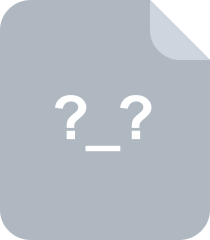
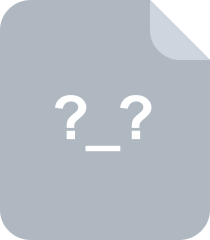
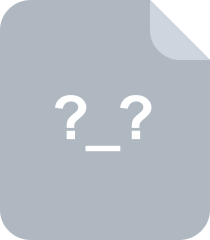
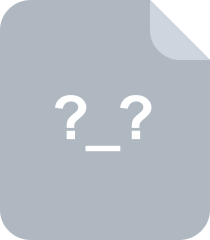
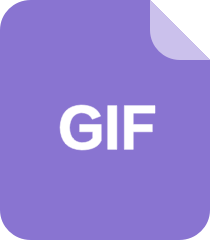
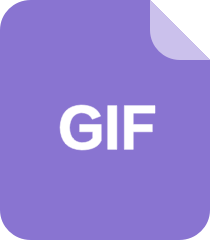
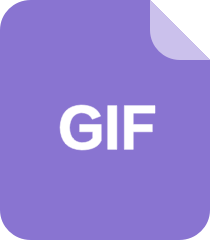
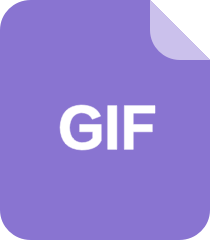
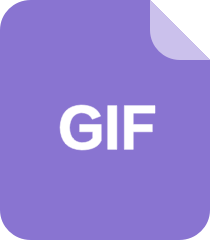
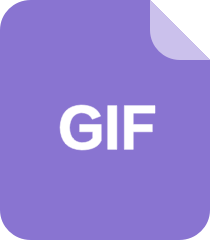
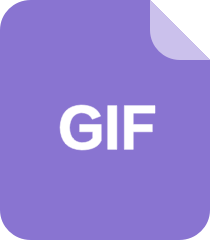
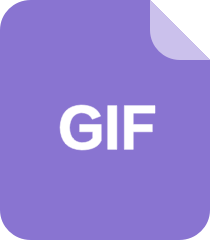
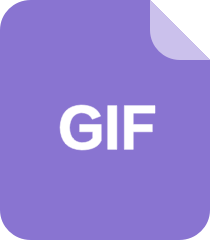
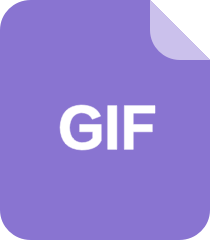
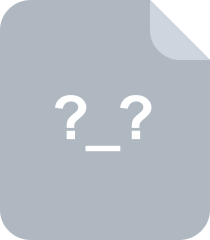
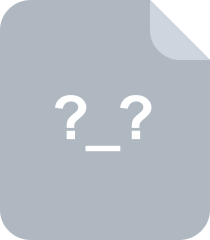
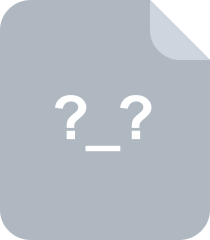
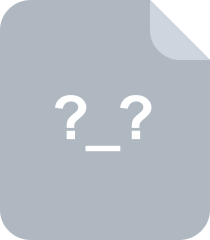
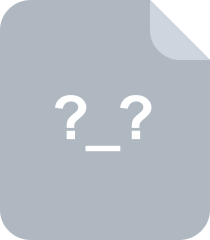
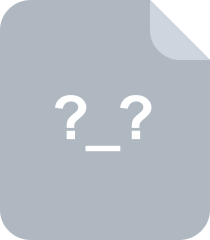
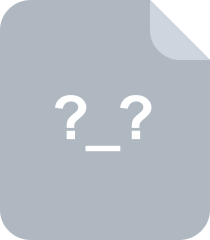
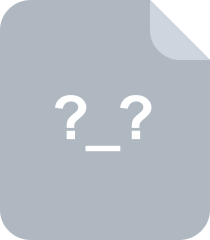
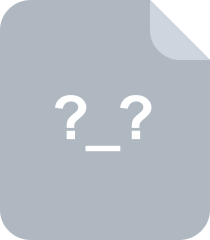
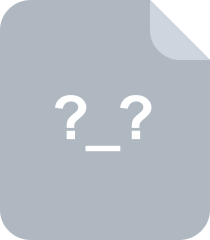
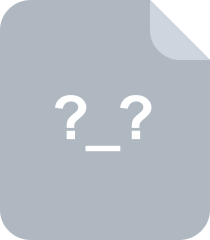
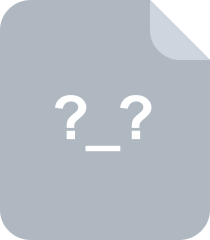
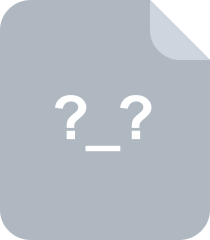
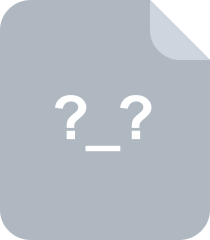
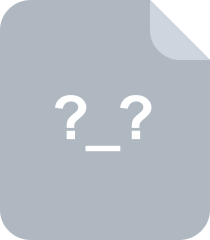
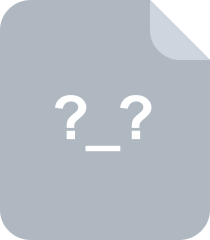
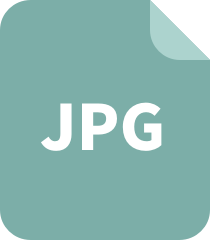
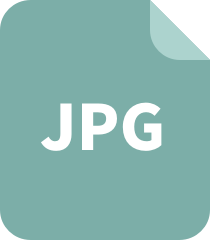
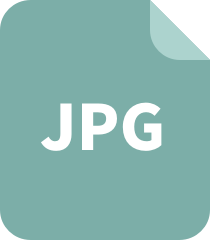
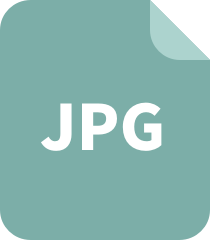
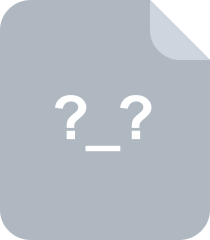
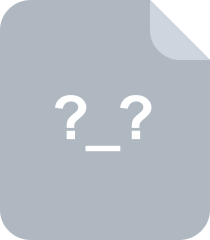
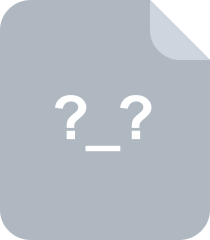
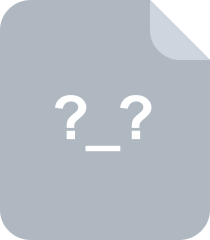
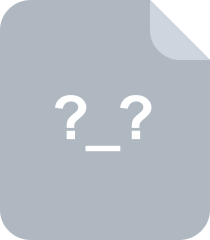
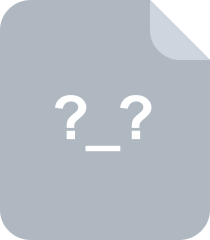
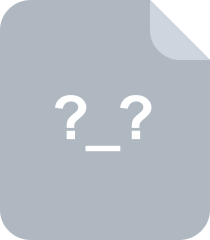
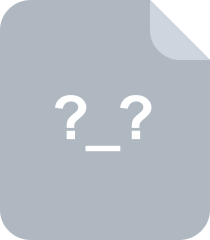
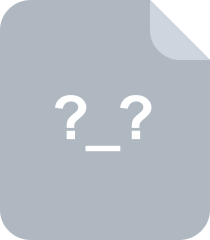
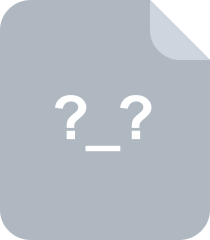
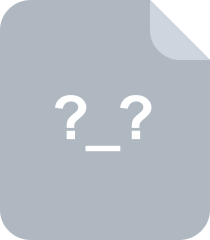
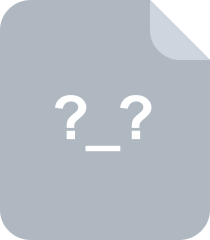
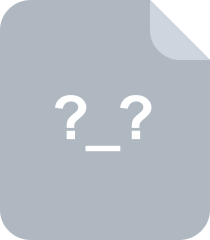
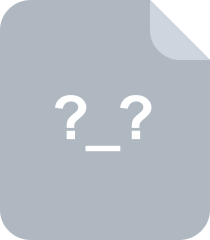
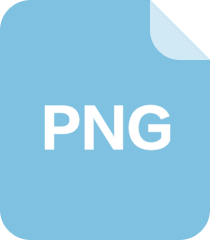
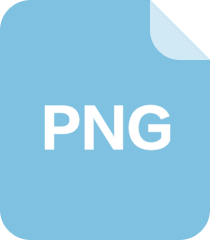
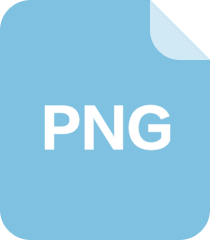
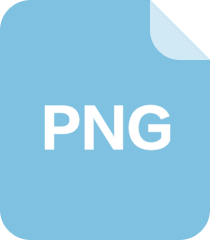
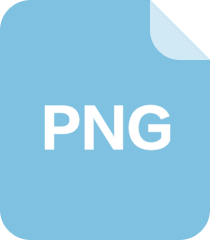
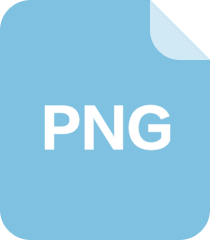
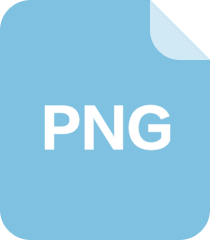
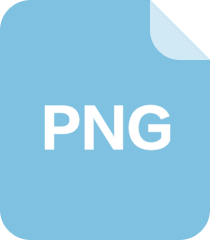
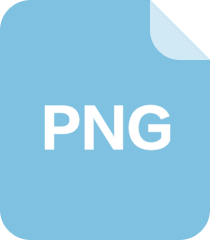
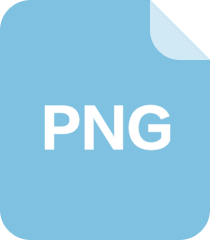
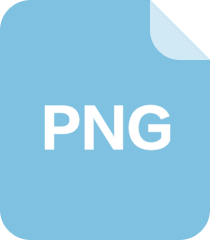
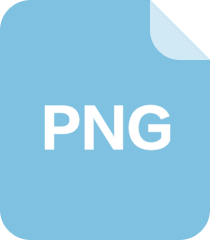
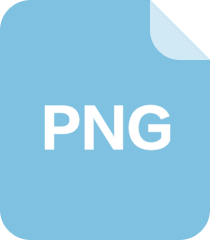
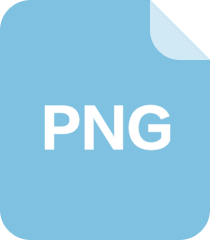
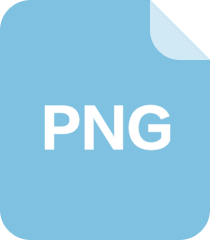
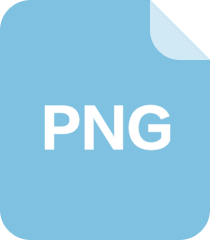
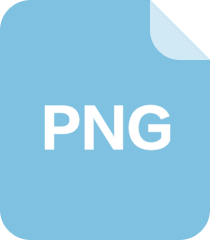
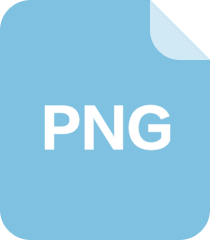
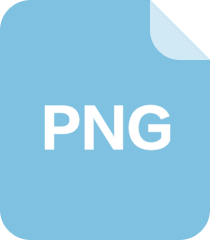
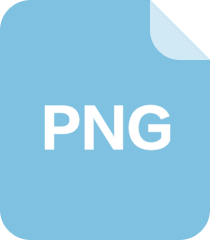
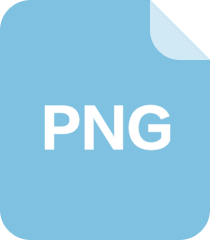
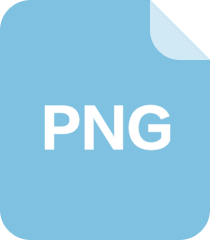
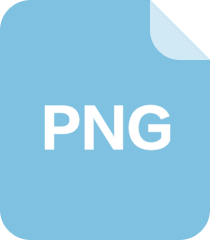
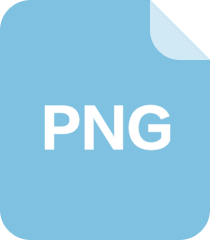
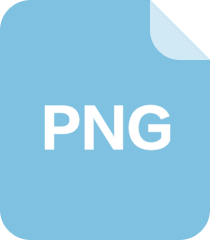
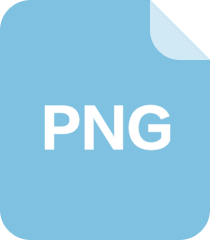
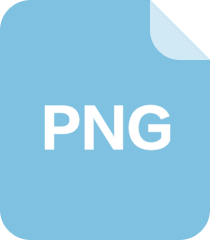
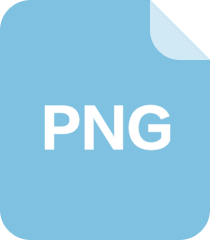
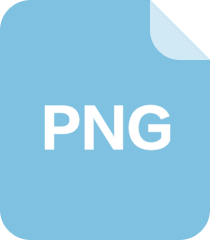
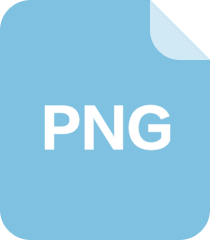
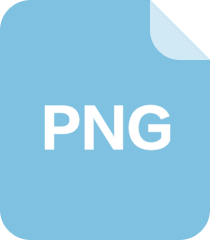
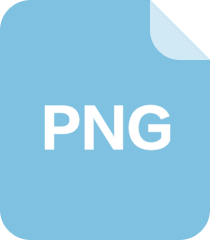
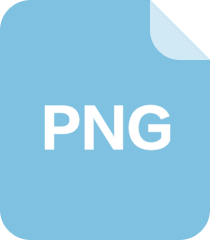
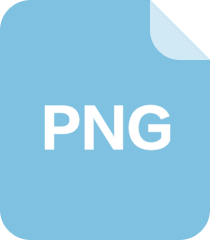
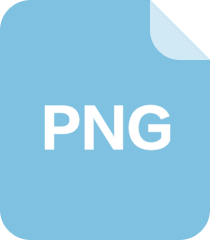
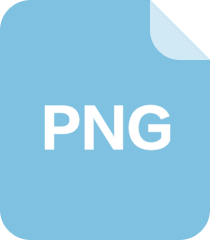
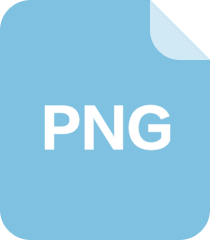
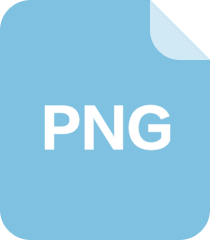
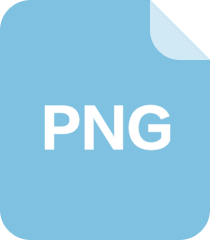
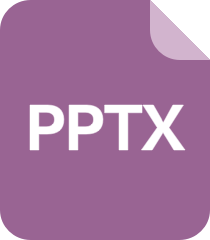
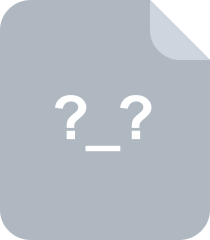
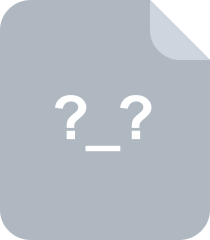
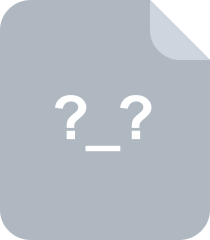
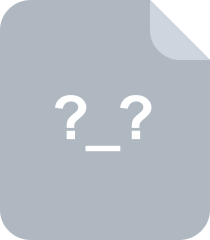
共 338 条
- 1
- 2
- 3
- 4
资源评论
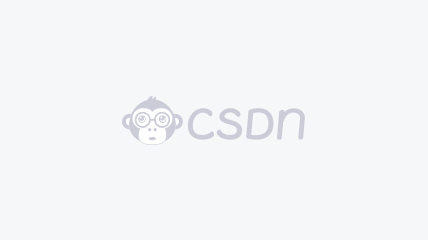
- m0_508421962024-04-22这个资源对我启发很大,受益匪浅,学到了很多,谢谢分享~

猰貐的新时代
- 粉丝: 1w+
- 资源: 2886
上传资源 快速赚钱
我的内容管理 展开
我的资源 快来上传第一个资源
我的收益
登录查看自己的收益我的积分 登录查看自己的积分
我的C币 登录后查看C币余额
我的收藏
我的下载
下载帮助

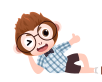
最新资源
- java项目,毕业设计-家具商城系统
- sparse-occ-cpu.onnx
- c2532703d1b4e83f570f28ff6cf94aef_语法.pdf
- C# 将不限数量的Excel表格进行合并,支持多文件多表合并.zip
- java项目,毕业设计-体育场馆运营
- 阿里云联合中国信通院安全所发布-大模型安全研究报告2024
- 低空经济政策与产业生态研究报告(2024年)
- 基于微信小程序的手机商城的设计与实现ssm.zip
- 基于springboot汽车维修管理系统微信小程序springboot.zip
- 非常好用 的一款,网卡流量监控工具,可长时间 监控,有图标展示流量趋势,要记录一段时间 内的平均 流量,可单独记录每个网卡的流量, 绿色好用, 无功能 限制
- 基于微信小程序的医院挂号预约系统ssm.zip
- 基于机器学习的商品评论分析系统源代码+文档说明+GUI界面(高分项目)
- 基于微信小程序的校园二手交易平台ssm.zip
- 基于微信小程序的校园综合服务平台ssm.zip
- 基于微信小程序高校订餐系统的设计与开发ssm.zip
- 线性回归实现股票预测源代码
资源上传下载、课程学习等过程中有任何疑问或建议,欢迎提出宝贵意见哦~我们会及时处理!
点击此处反馈


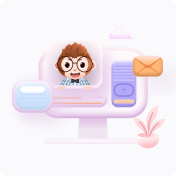
安全验证
文档复制为VIP权益,开通VIP直接复制
