import datetime
import json
import pickle
import random
import numpy
import matplotlib
import numpy as np
import scipy
from sklearn import metrics, ensemble
from sklearn.metrics import mean_absolute_error
from sklearn.neighbors import RadiusNeighborsRegressor
from sklearn.neural_network import MLPRegressor
from sklearn.preprocessing import OneHotEncoder, MinMaxScaler
import pymysql
from django.core import serializers
from django.http import JsonResponse
from django.shortcuts import render
from sklearn.svm import SVR
from sklearn import linear_model
from django.views.decorators.csrf import csrf_exempt
# Create your views here.
from django.views.decorators.csrf import csrf_exempt
from django.views.decorators.http import require_http_methods
from sklearn.tree import DecisionTreeRegressor
from RailTransitCrowdForecasting.models import Lineflow, Stationflow, Station, Linedayflow, Stationdayflow
# 获取站点信息
@require_http_methods(["GET"])
def get_line(request, ):
response = {}
idlist = []
lines = Lineflow.objects.values()
mset = set()
for line in lines:
if line["lineid"] not in mset:
mdic = dict()
mset.add(line["lineid"])
mdic["lineid"] = line["lineid"]
idlist.append(mdic)
response["linelist"] = idlist
return JsonResponse(response)
@require_http_methods(["GET"])
def initial(request, ):
response = {}
stations = Station.objects.values()
line_set = set()
res = []
for station in stations:
if station["lineid_id"] not in line_set:
line_set.add(station["lineid_id"])
line = {"value": station["lineid_id"], "label": str(station["lineid_id"]) + "号线", "children": []}
children = {"value": station["stationid"], "label": station["stationname"]}
line["children"].append(children)
res.append(line)
else:
for line in res:
if line["value"] == station["lineid_id"]:
children = {"value": station["stationid"], "label": station["stationname"]}
line["children"].append(children)
response["list"] = res
return JsonResponse(response)
# 获取历史客流量
@require_http_methods(["GET"])
def getFlow(request):
lineID = request.GET["lineID"]
stationID = request.GET["stationID"]
date = request.GET["date"]
# lineID = '3'
# stationID = '303'
# date = '2018-06-05'
dateFormat = datetime.datetime.strptime(date, "%Y-%m-%d")
lineRes = Linedayflow.objects.filter(lineid=lineID, date=dateFormat).values()
stationRes = Stationdayflow.objects.filter(stationid=stationID, date=dateFormat).values()
stationName = Station.objects.filter(stationid=stationID).values()[0]["stationname"]
lineFlowList = []
stationFlowList = []
for line in lineRes:
lineFlowList.append(line["flow"])
for station in stationRes:
stationFlowList.append(station["flow"])
response = {"lineCheck": {"lineID": lineID, "lineName": str(lineID) + "号线", "date": date, "flow": lineFlowList},
"stationCheck": {"stationID": stationID, "stationName": stationName, "date": date,
"flow": stationFlowList}
}
return JsonResponse(response)
# 预测客流量
@csrf_exempt
@require_http_methods(["POST"])
def predict(request):
params = json.loads(request.body)["params"]
lineID = params["lineID"]
stationID = params["stationID"]
date = params["date"]
methodList = params["methods"][1:-1].replace('"', '').split(',')
response = {}
response["station"] = {}
response["line"] = {}
# lineID = '3'
# stationID = '303'
# date = '2018-06-03'
# methodList= "BPNN,BPNN"
dayType = datetime.datetime.strptime(date, "%Y-%m-%d").weekday() + 1
stationName = Station.objects.filter(stationid=stationID).values()[0]["stationname"]
for method in methodList:
print(method)
m = datetime.timedelta(hours=7)
station_pre_flow = list()
line_pre_flow = list()
station_test_flow = list()
line_test_flow = list()
while m <= datetime.timedelta(hours=23):
station_data = get_station_data(m, stationID, date)
line_data = get_line_data(m, lineID, date)
station_res = train(station_data, method)
line_res = train(line_data, method)
station_test_flow.append(station_res[0])
station_pre_flow.append(station_res[1])
line_test_flow.append(line_res[0])
line_pre_flow.append(line_res[1])
m = m + datetime.timedelta(minutes=5)
station_mse = metrics.mean_squared_error(station_test_flow, station_pre_flow)
station_rmse = np.sqrt(station_mse)
station_mae = metrics.mean_absolute_error(station_test_flow, station_pre_flow)
line_mse = metrics.mean_squared_error(line_test_flow, line_pre_flow)
line_rmse = np.sqrt(line_mse)
line_mae = metrics.mean_absolute_error(line_test_flow, line_pre_flow)
response["station"][method] = {
"preflow": station_pre_flow, "mse": int(station_mse), "rmse": int(station_rmse), "mae": int(station_mae)
}
response["line"][method] = {
"preflow": line_pre_flow, "mse": int(line_mse), "rmse": int(line_rmse), "mae": int(line_mae)
}
response["station"]["stationName"] = stationName
response["station"]["stationID"] = stationID
response["station"]["date"] = date
response["station"]["testflow"] = station_test_flow
response["line"]["lineName"] = str(lineID) + "号线"
response["line"]["lineID"] = lineID
response["line"]["date"] = date
response["line"]["testflow"] = line_test_flow
return JsonResponse(response)
#
# # bpnn预测客流量
# @require_http_methods(["GET"])
# def bpnnpredict(request):
# # print(request)
# # print("12345")
# lineID = request.GET["lineID"]
# stationID = request.GET["stationID"]
# date = request.GET["date"]
# # lineID = '3'
# # stationID = '303'
# # date = '2018-06-03'
# dayType = datetime.datetime.strptime(date, "%Y-%m-%d").weekday() + 1
# stationName = Station.objects.filter(stationid=stationID).values()[0]["stationname"]
# m = datetime.timedelta(hours=7)
# station_test_flow = list()
# station_pre_flow = list()
# line_test_flow = list()
# line_pre_flow = list()
# while m <= datetime.timedelta(hours=23):
# station_data = get_station_data(m, stationID, date)
# line_data = get_line_data(m, lineID, date)
# station_res = bpnn_train(station_data)
# line_res = bpnn_train(line_data)
# station_test_flow.append(station_res[0])
# station_pre_flow.append(station_res[1])
# line_test_flow.append(line_res[0])
# line_pre_flow.append(line_res[1])
# m = m + datetime.timedelta(minutes=5)
#
# station_mse = metrics.mean_squared_error(station_test_flow, station_pre_flow)
# station_rmse = np.sqrt(station_mse)
# station_mae = metrics.mean_absolute_error(station_test_flow, station_pre_flow)
# line_mse = metrics.mean_squared_error(line_test_flow, line_pre_flow)
# line_rmse = np.sqrt(line_mse)
# line_mae = metrics.mean_absolute_error(line_test_flow, line_pre_flow)
# response = {"line": {"lineID": lineID, "lineName": str(lineID) + "号线", "date": date,
# "testflow": line_test_flow, "preflow": line_pre_flow,
# "mse": int(line_mse), "rmse": int(line_rmse), "mae": int(line_mae)},
# "station": {"stationID": stationID, "stationName": stationName, "date": date,
# "testflow": station_test_flow, "preflow": station_pre_flow,
# "mse": int(station_mse), "rmse": int(station_rmse), "mae": int(station_mae)}
# }
#
# return JsonResponse(response)
#
#
#
没有合适的资源?快使用搜索试试~ 我知道了~
温馨提示
毕业设计基于机器学习python实现的重庆轨道交通客流量时空分析预测项目源码.zip 本科毕业设计,基于机器学习的重庆轨道交通客流量时空分析预测,客流量统计计算,对于站点:将每个站点抽象为一个图,利用弗洛伊德算法解决多源最短路径路径,求出乘客从站点A 进入,在站点D离开中途经过的所有站点,为途径的每个站点的人流量数加1,这样可以获取每个站点的日均人流量。 对于线路(1号线,2号线的客流量):同用洛伊德算法,求出乘客从站点A 进入,在站点D离开中途经过的所有涉及到的线路,每个线路人流量加1。 利用BP神经网络进行预测 对于每条线路的客流量分析 day,工作日,周六,周天 han_shu_jia,三种值,不是寒暑假,寒假,暑假 week_of_mouth,该周是一个月的中第几周 season_of_year,一年中的哪个季节 只对日均人流量最大的10个站点进行人流预测, type,所属线路 day,工作日,周六,周天 han_shu_jia,三种值,寒假,暑假, 不是假期 week_of_mouth,该周是一个月的中第几周 season_of_year,一年中的哪个季节
资源推荐
资源详情
资源评论
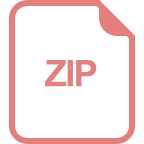
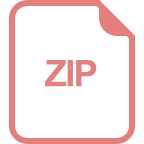
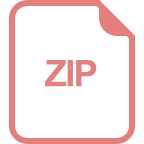
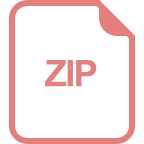
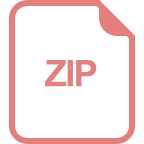
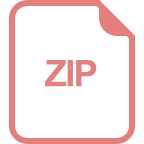
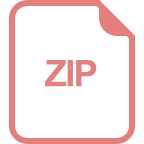
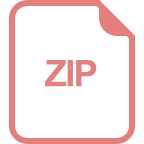
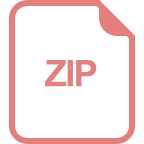
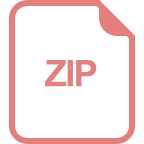
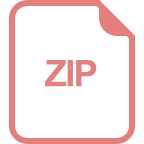
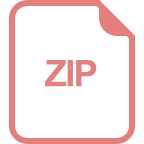
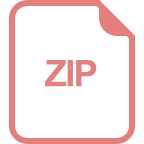
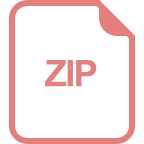
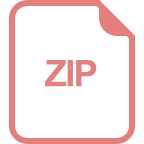
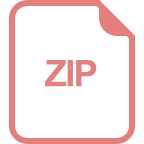
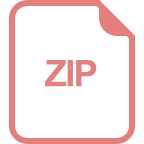
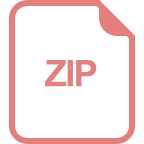
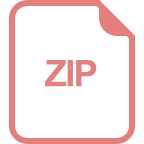
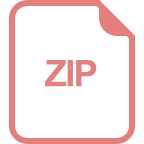
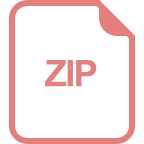
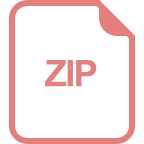
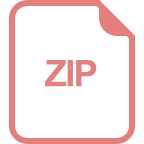
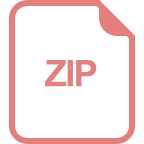
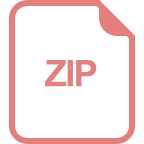
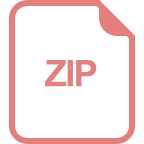
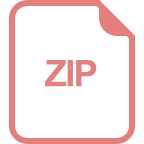
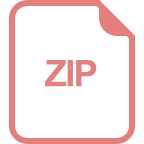
收起资源包目录

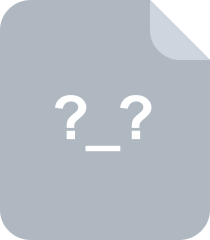
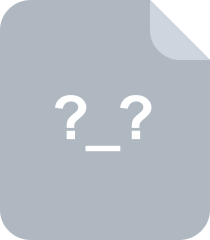
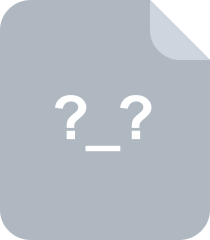
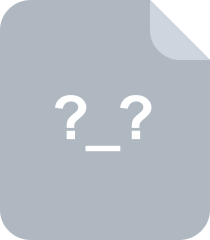
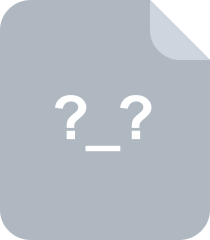
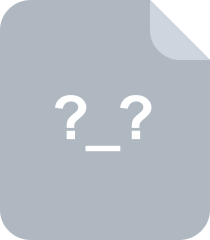
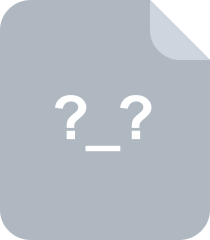
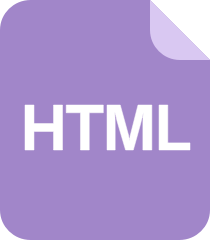
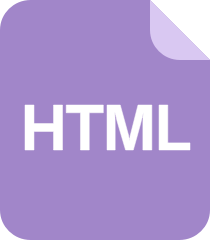
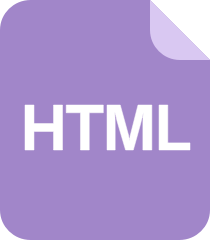
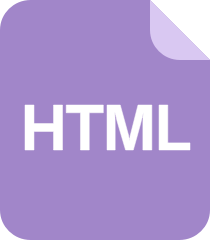
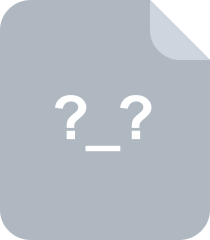
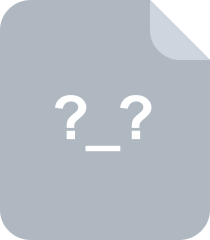
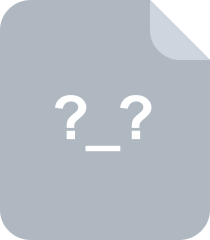
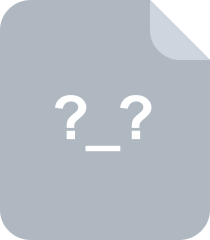
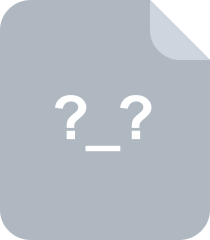
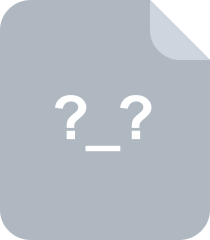
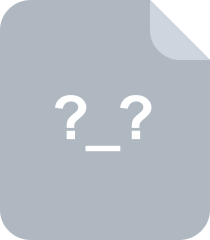
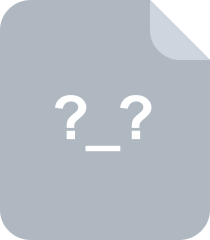
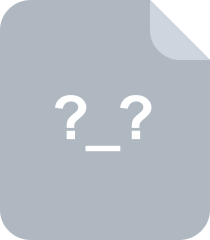
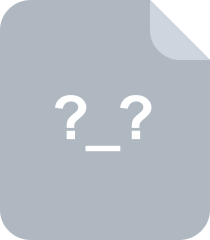
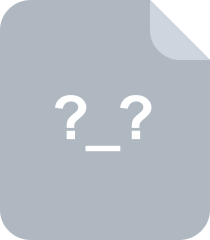
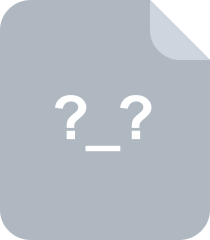
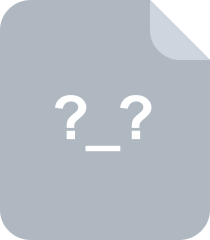
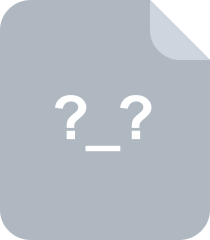
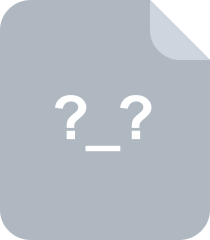
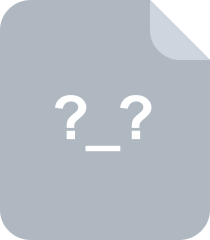
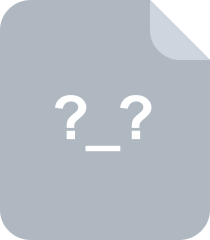
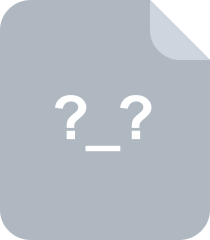
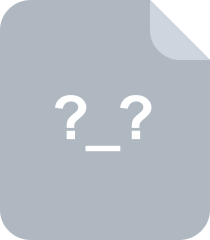
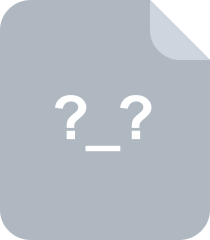
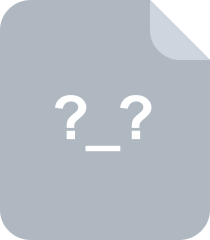
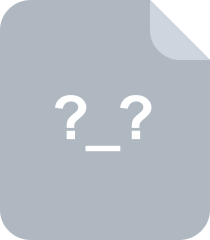
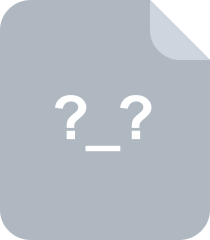
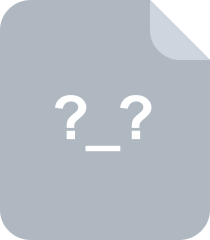
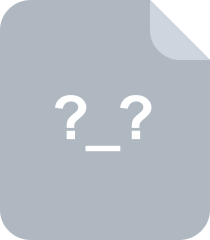
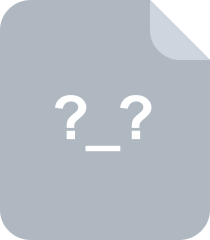
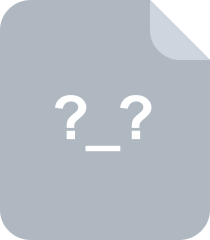
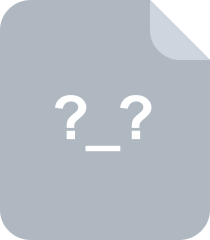
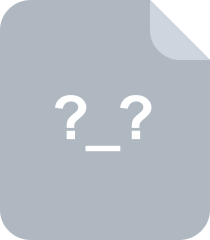
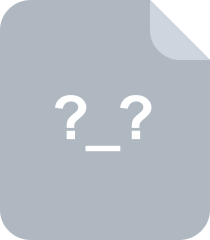
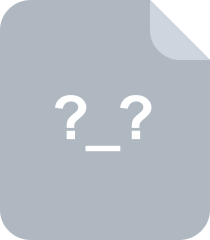
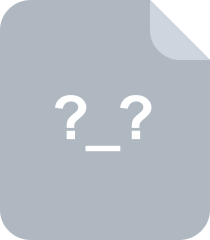
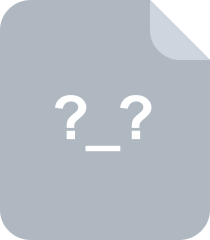
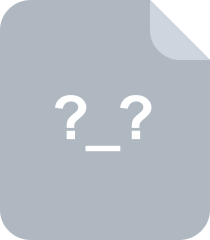
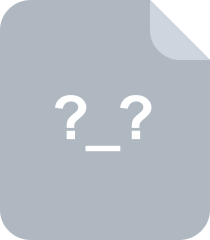
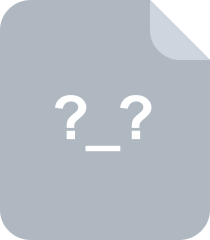
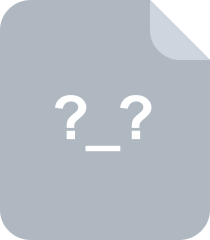
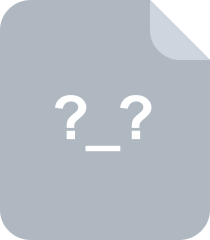
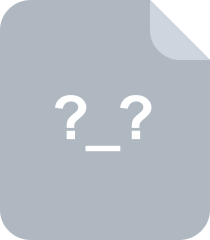
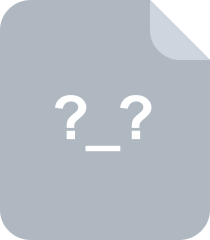
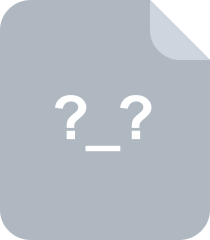
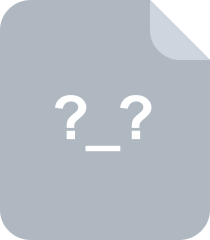
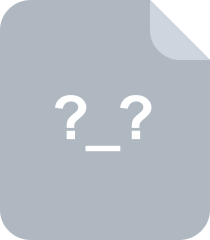
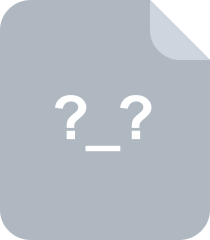
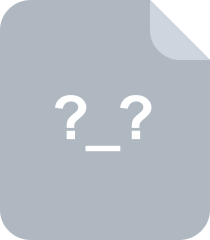
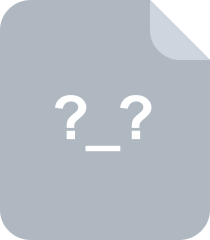
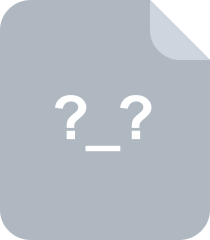
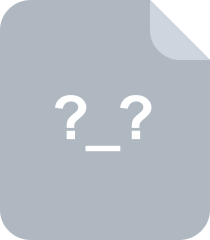
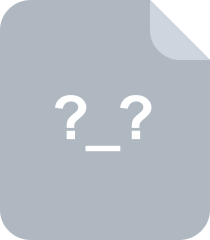
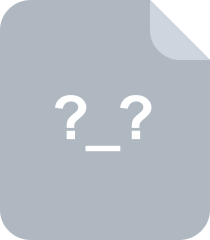
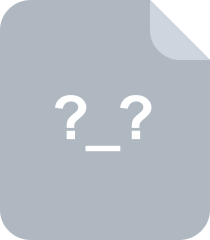
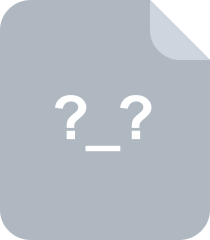
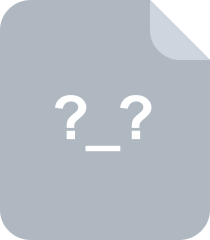
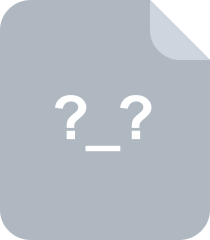
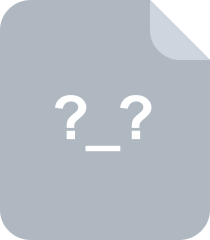
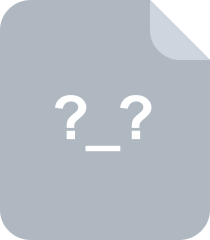
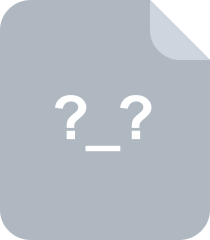
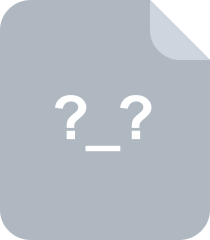
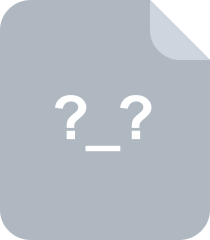
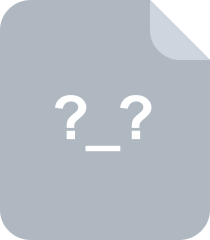
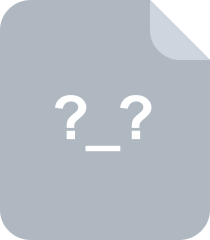
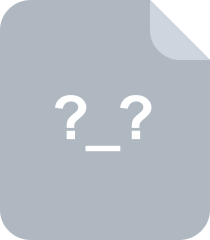
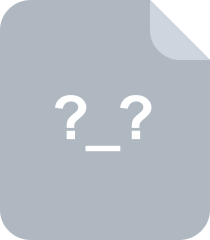
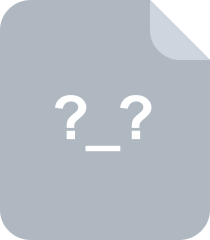
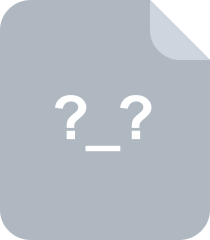
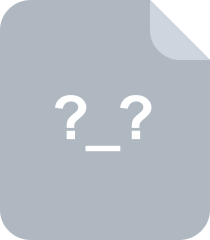
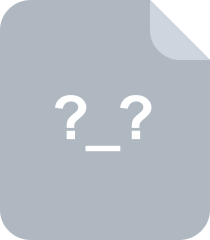
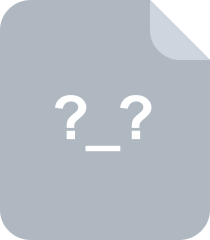
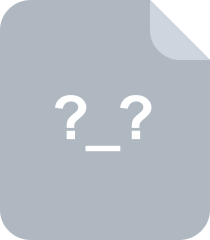
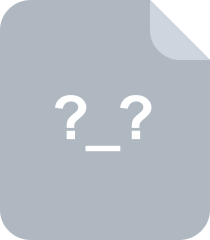
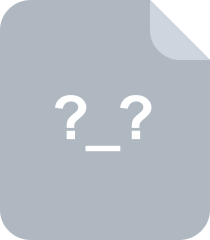
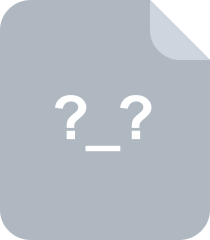
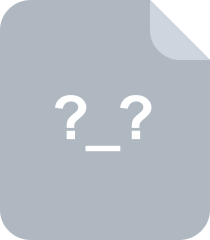
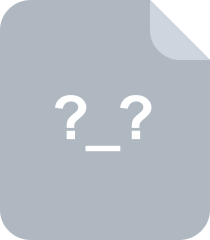
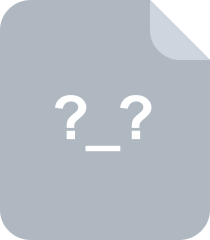
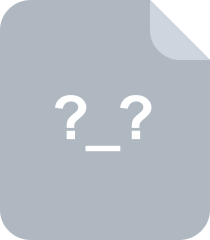
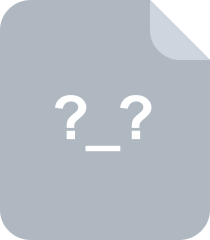
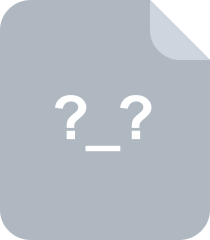
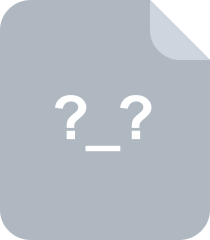
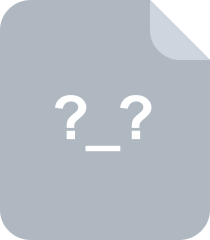
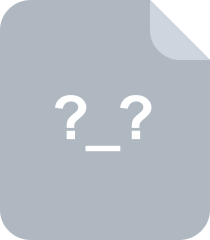
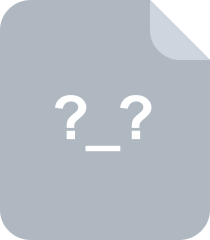
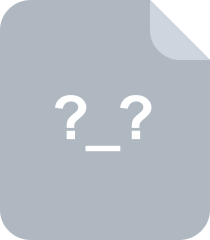
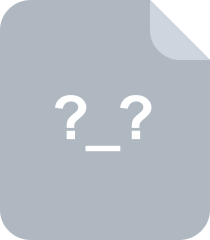
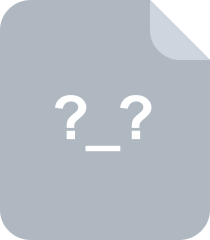
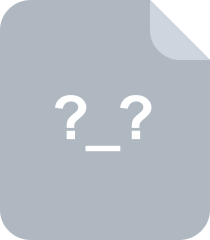
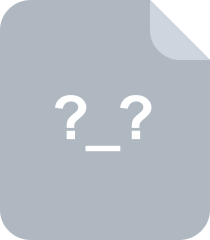
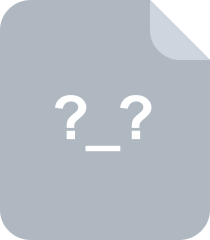
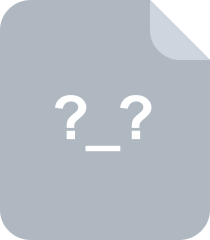
共 1707 条
- 1
- 2
- 3
- 4
- 5
- 6
- 18
资源评论
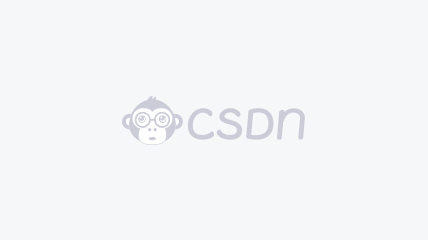

猰貐的新时代
- 粉丝: 1w+
- 资源: 2886
上传资源 快速赚钱
我的内容管理 展开
我的资源 快来上传第一个资源
我的收益
登录查看自己的收益我的积分 登录查看自己的积分
我的C币 登录后查看C币余额
我的收藏
我的下载
下载帮助

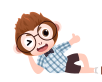
最新资源
资源上传下载、课程学习等过程中有任何疑问或建议,欢迎提出宝贵意见哦~我们会及时处理!
点击此处反馈


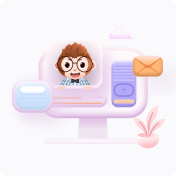
安全验证
文档复制为VIP权益,开通VIP直接复制
