# TensorFlow Recommenders


[](https://pypi.python.org/pypi/tensorflow-recommenders/)
TensorFlow Recommenders is a library for building recommender system models
using [TensorFlow](https://www.tensorflow.org).
It helps with the full workflow of building a recommender system: data
preparation, model formulation, training, evaluation, and deployment.
It's built on Keras and aims to have a gentle learning curve while still giving
you the flexibility to build complex models.
## Installation
Make sure you have TensorFlow 2.x installed, and install from `pip`:
```shell
pip install tensorflow-recommenders
```
## Documentation
Have a look at our
[tutorials](https://tensorflow.org/recommenders/examples/quickstart) and
[API reference](https://www.tensorflow.org/recommenders/api_docs/python/tfrs/).
## Quick start
Building a factorization model for the Movielens 100K dataset is very simple
([Colab](https://tensorflow.org/recommenders/examples/quickstart)):
```python
from typing import Dict, Text
import tensorflow as tf
import tensorflow_datasets as tfds
import tensorflow_recommenders as tfrs
# Ratings data.
ratings = tfds.load('movielens/100k-ratings', split="train")
# Features of all the available movies.
movies = tfds.load('movielens/100k-movies', split="train")
# Select the basic features.
ratings = ratings.map(lambda x: {
"movie_id": tf.strings.to_number(x["movie_id"]),
"user_id": tf.strings.to_number(x["user_id"])
})
movies = movies.map(lambda x: tf.strings.to_number(x["movie_id"]))
# Build a model.
class Model(tfrs.Model):
def __init__(self):
super().__init__()
# Set up user representation.
self.user_model = tf.keras.layers.Embedding(
input_dim=2000, output_dim=64)
# Set up movie representation.
self.item_model = tf.keras.layers.Embedding(
input_dim=2000, output_dim=64)
# Set up a retrieval task and evaluation metrics over the
# entire dataset of candidates.
self.task = tfrs.tasks.Retrieval(
metrics=tfrs.metrics.FactorizedTopK(
candidates=movies.batch(128).map(self.item_model)
)
)
def compute_loss(self, features: Dict[Text, tf.Tensor], training=False) -> tf.Tensor:
user_embeddings = self.user_model(features["user_id"])
movie_embeddings = self.item_model(features["movie_id"])
return self.task(user_embeddings, movie_embeddings)
model = Model()
model.compile(optimizer=tf.keras.optimizers.Adagrad(0.5))
# Randomly shuffle data and split between train and test.
tf.random.set_seed(42)
shuffled = ratings.shuffle(100_000, seed=42, reshuffle_each_iteration=False)
train = shuffled.take(80_000)
test = shuffled.skip(80_000).take(20_000)
# Train.
model.fit(train.batch(4096), epochs=5)
# Evaluate.
model.evaluate(test.batch(4096), return_dict=True)
```
没有合适的资源?快使用搜索试试~ 我知道了~
tensorflow-recommenders-0.3.2.tar.gz
0 下载量 12 浏览量
2024-03-21
12:49:42
上传
评论
收藏 24KB GZ 举报
温馨提示
Python库是一组预先编写的代码模块,旨在帮助开发者实现特定的编程任务,无需从零开始编写代码。这些库可以包括各种功能,如数学运算、文件操作、数据分析和网络编程等。Python社区提供了大量的第三方库,如NumPy、Pandas和Requests,极大地丰富了Python的应用领域,从数据科学到Web开发。Python库的丰富性是Python成为最受欢迎的编程语言之一的关键原因之一。这些库不仅为初学者提供了快速入门的途径,而且为经验丰富的开发者提供了强大的工具,以高效率、高质量地完成复杂任务。例如,Matplotlib和Seaborn库在数据可视化领域内非常受欢迎,它们提供了广泛的工具和技术,可以创建高度定制化的图表和图形,帮助数据科学家和分析师在数据探索和结果展示中更有效地传达信息。
资源推荐
资源详情
资源评论
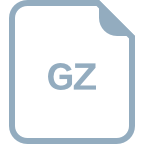
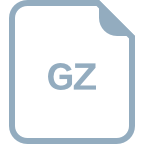
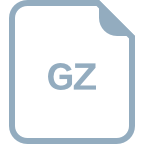
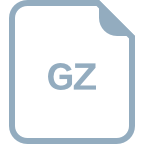
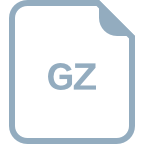
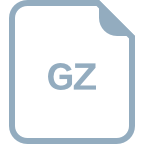
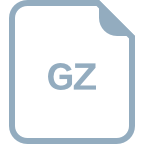
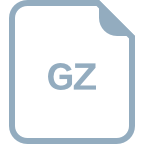
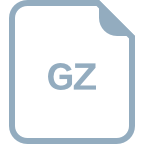
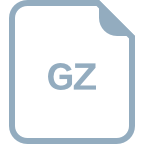
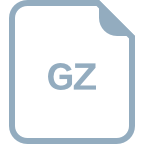
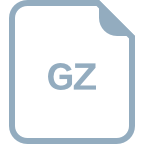
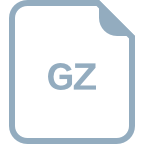
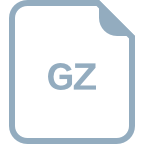
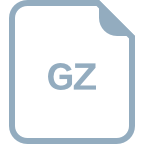
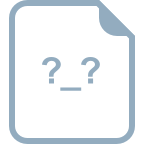
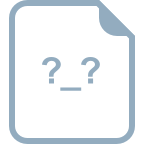
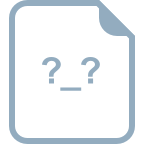
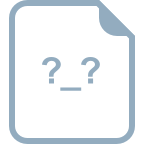
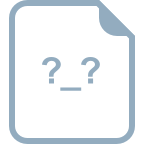
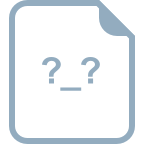
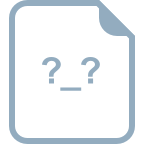
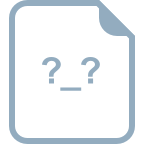
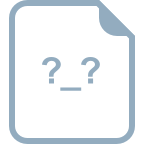
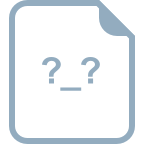
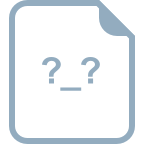
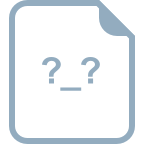
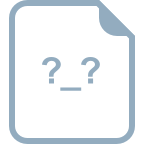
收起资源包目录



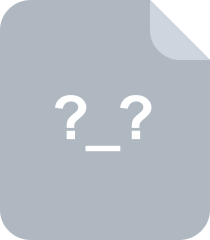

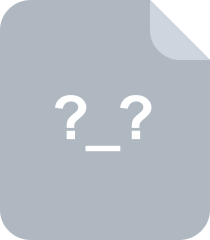
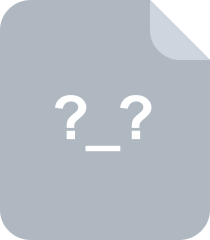
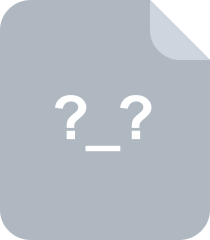
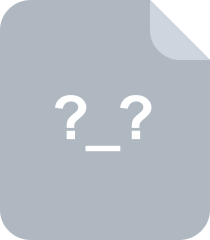
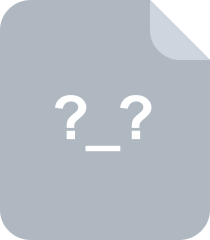
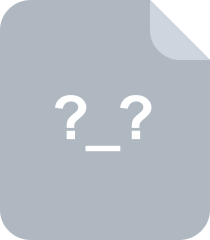
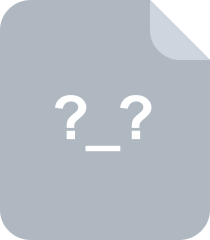

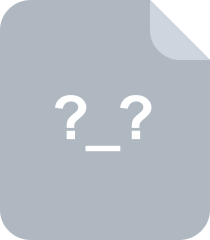
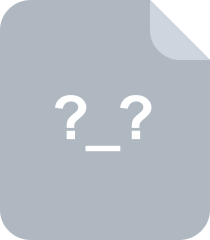
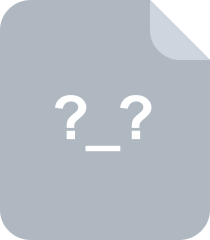
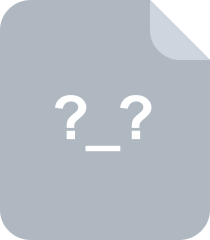
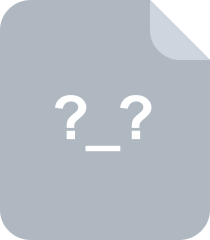
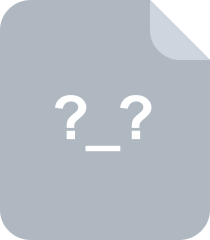

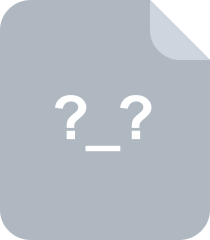
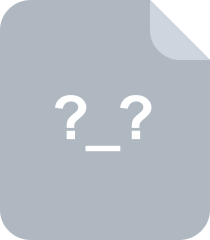
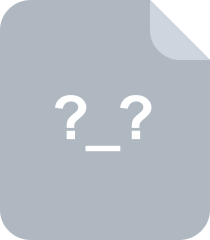

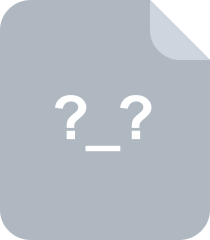
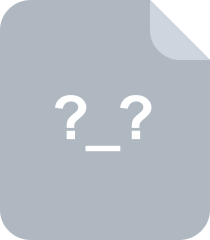
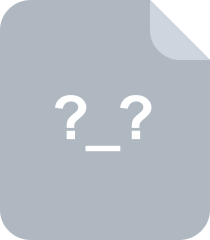

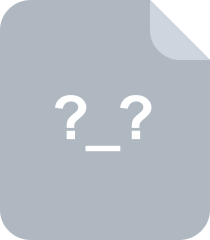
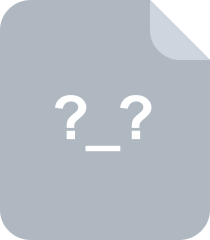
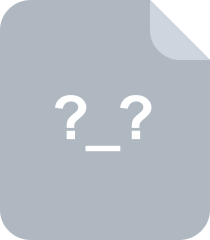
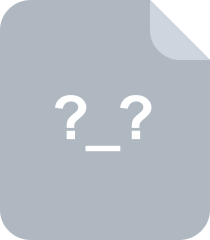

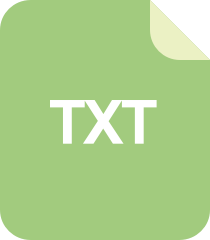
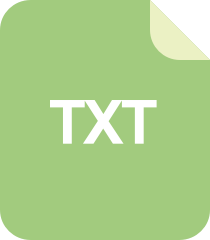
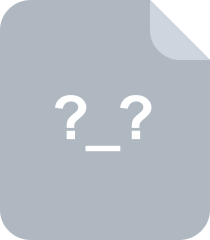
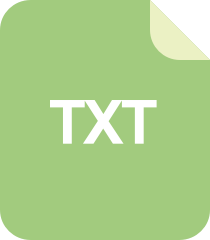
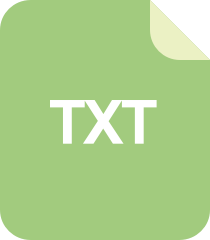
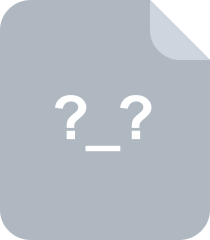
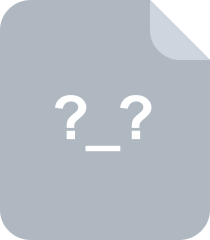
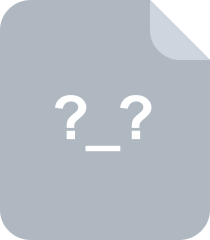
共 32 条
- 1
资源评论
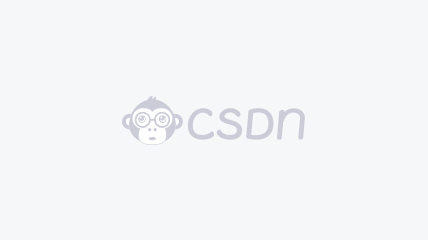

程序员Chino的日记
- 粉丝: 3671
- 资源: 5万+
上传资源 快速赚钱
我的内容管理 展开
我的资源 快来上传第一个资源
我的收益
登录查看自己的收益我的积分 登录查看自己的积分
我的C币 登录后查看C币余额
我的收藏
我的下载
下载帮助

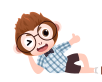
最新资源
资源上传下载、课程学习等过程中有任何疑问或建议,欢迎提出宝贵意见哦~我们会及时处理!
点击此处反馈


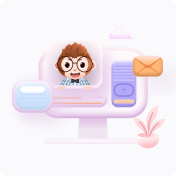
安全验证
文档复制为VIP权益,开通VIP直接复制
