# `pandas-profiling`

[](https://github.com/ydataai/pandas-profiling/actions/workflows/tests.yml)
[](https://pypi.python.org/pypi/pandas-profiling/)
[](https://pypi.org/project/pandas-profiling/)
[](https://codecov.io/gh/ydataai/pandas-profiling)
[](https://github.com/ydataai/pandas-profiling/releases)
[](https://pypi.org/project/pandas-profiling/)
[](https://github.com/python/black)
<p align="center">
<a href="https://pandas-profiling.ydata.ai/docs/master/">Documentation</a>
|
<a href="https://discord.com/invite/mw7xjJ7b7s">Discord</a>
|
<a href="https://stackoverflow.com/questions/tagged/pandas-profiling">Stack Overflow</a>
|
<a href="https://pandas-profiling.ydata.ai/docs/master/pages/reference/changelog.html#changelog">Latest changelog</a>
</p>
<p align="center">
Do you like this project? Show us your love and <a href="https://engage.ydata.ai">give feedback!</a>
</p>
`pandas-profiling` primary goal is to provide a one-line Exploratory Data Analysis (EDA) experience in a consistent and fast solution. Like pandas `df.describe()` function, that is so handy, pandas-profiling delivers an extended analysis of a DataFrame while alllowing the data analysis to be exported in different formats such as **html** and **json**.
The package outputs a simple and digested analysis of a dataset, including **time-series** and **text**.
### Key features
- **Type inference**: automatic detection of columns' data types (*Categorical*, *Numerical*, *Date*, etc.)
- **Warnings**: A summary of the problems/challenges in the data that you might need to work on (*missing data*, *inaccuracies*, *skewness*, etc.)
- **Univariate analysis**: including descriptive statistics (mean, median, mode, etc) and informative visualizations such as distribution histograms
- **Multivariate analysis**: including correlations, a detailed analysis of missing data, duplicate rows, and visual support for variables pairwise interaction
- **Time-Series**: including different statistical information relative to time dependent data such as auto-correlation and seasonality, along ACF and PACF plots.
- **Text analysis**: most common categories (uppercase, lowercase, separator), scripts (Latin, Cyrillic) and blocks (ASCII, Cyrilic)
- **File and Image analysis**: file sizes, creation dates, dimensions, indication of truncated images and existence of EXIF metadata
- **Compare datasets**: one-line solution to enable a fast and complete report on the comparison of datasets
- **Flexible output formats**: all analysis can be exported to an HTML report that can be easily shared with different parties, as JSON for an easy integration in automated systems and as a widget in a Jupyter Notebook.
The report contains three additional sections:
- **Overview**: mostly global details about the dataset (number of records, number of variables, overall missigness and duplicates, memory footprint)
- **Alerts**: a comprehensive and automatic list of potential data quality issues (high correlation, skewness, uniformity, zeros, missing values, constant values, between others)
- **Reproduction**: technical details about the analysis (time, version and configuration)
> ### ð Latest features
> - Looking for how you can do an EDA for Time-Series ð ? Check [this blogpost](https://towardsdatascience.com/how-to-do-an-eda-for-time-series-cbb92b3b1913).
> - You want to compare 2 datasets and get a report? Check [this blogpost](https://medium.com/towards-artificial-intelligence/how-to-compare-2-dataset-with-pandas-profiling-2ae3a9d7695e)
## ð Use cases
Pandas-profiling can be used to deliver a variety of different use-case. The documentation includes guides, tips and tricks for tackling them:
| Use case | Description |
|----------|----------------------------------------------------------------------------------------------|
| [Comparing datasets](https://pandas-profiling.ydata.ai/docs/master/pages/use_cases/comparing_datasets.html ) | Comparing multiple version of the same dataset |
| [Profiling a Time-Series dataset](https://pandas-profiling.ydata.ai/docs/master/pages/use_cases/time_series_datasets.html) | Generating a report for a time-series dataset with a single line of code |
|[Profiling large datasets](https://pandas-profiling.ydata.ai/docs/master/pages/use_cases/big_data.html ) | Tips on how to prepare data and configure `pandas-profiling` for working with large datasets |
| [Handling sensitive data](https://pandas-profiling.ydata.ai/docs/master/pages/use_cases/sensitive_data.html ) | Generating reports which are mindful about sensitive data in the input dataset |
| [Dataset metadata and data dictionaries](https://pandas-profiling.ydata.ai/docs/master/pages/use_cases/metadata.html) | Complementing the report with dataset details and column-specific data dictionaries |
| [Customizing the report's appearance](https://pandas-profiling.ydata.ai/docs/master/pages/use_cases/custom_report_appearance.html ) | Changing the appearance of the report's page and of the contained visualizations |
> â¡ Looking for a Spark backend to profile large datasets? It's [work in progress](https://github.com/ydataai/pandas-profiling/projects/3).
## â¶ï¸ Quickstart
Start by loading your pandas `DataFrame` as you normally would, e.g. by using:
```python
import numpy as np
import pandas as pd
from pandas_profiling import ProfileReport
df = pd.DataFrame(np.random.rand(100, 5), columns=["a", "b", "c", "d", "e"])
```
To generate the standard profiling report, merely run:
```python
profile = ProfileReport(df, title="Pandas Profiling Report")
```
### Using inside Jupyter Notebooks
There are two interfaces to consume the report inside a Jupyter notebook: through widgets and through an embedded HTML report.
<img alt="Notebook Widgets" src="https://pandas-profiling.ydata.ai/docs/master/assets/widgets.gif" width="800" />
The above is achieved by simply displaying the report as a set of widgets. In a Jupyter Notebook, run:
```python
profile.to_widgets()
```
The HTML report can be directly embedded in a cell in a similar fashion:
```python
profile.to_notebook_iframe()
```
<img alt="HTML" src="https://pandas-profiling.ydata.ai/docs/master/assets/iframe.gif" width="800" />
### Exporting the report to a file
To generate a HTML report file, save the `ProfileReport` to an object and use the `to_file()` function:
```python
profile.to_file("your_report.html")
```
Alternatively, the report's data can be obtained as a JSON file:
```python
# As a JSON string
json_data = profile.to_json()
# As a file
profile.to_file("your_report.json")
```
### Using in the command line
For standard formatted CSV files (which can be read directly by pandas without additional settings), the `pandas_profiling` executable can be used in the command line. The example below generates a report named _Example Profiling Report_, using a configuration file called `default.yaml`, in the file `report.html` by processing a `data.csv` dataset.
```sh
pandas_profiling --title "Exampl
没有合适的资源?快使用搜索试试~ 我知道了~
pandas-profiling-3.6.3.tar.gz
需积分: 1 0 下载量 179 浏览量
2024-03-07
12:45:55
上传
评论
收藏 257KB GZ 举报
温馨提示
Python库是一组预先编写的代码模块,旨在帮助开发者实现特定的编程任务,无需从零开始编写代码。这些库可以包括各种功能,如数学运算、文件操作、数据分析和网络编程等。Python社区提供了大量的第三方库,如NumPy、Pandas和Requests,极大地丰富了Python的应用领域,从数据科学到Web开发。Python库的丰富性是Python成为最受欢迎的编程语言之一的关键原因之一。这些库不仅为初学者提供了快速入门的途径,而且为经验丰富的开发者提供了强大的工具,以高效率、高质量地完成复杂任务。例如,Matplotlib和Seaborn库在数据可视化领域内非常受欢迎,它们提供了广泛的工具和技术,可以创建高度定制化的图表和图形,帮助数据科学家和分析师在数据探索和结果展示中更有效地传达信息。
资源推荐
资源详情
资源评论
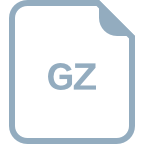
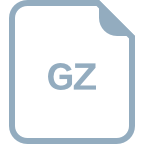
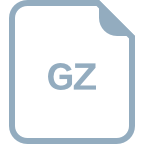
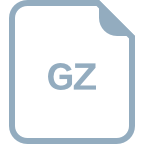
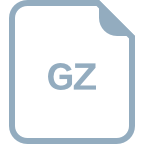
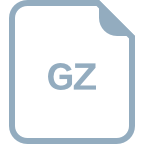
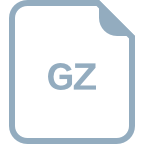
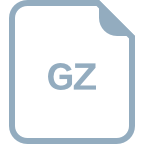
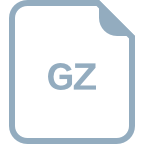
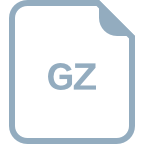
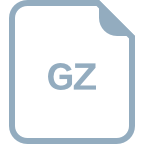
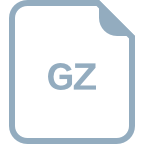
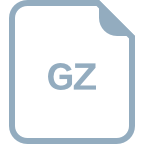
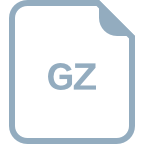
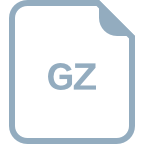
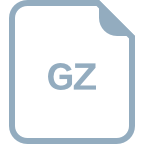
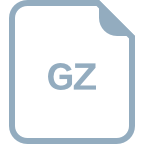
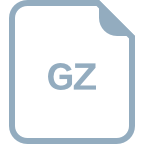
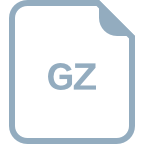
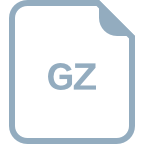
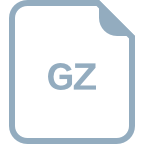
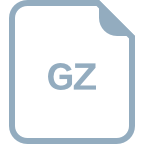
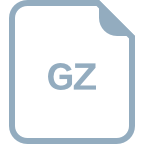
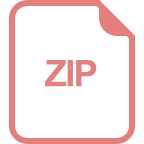
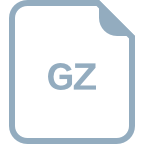
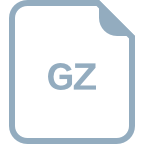
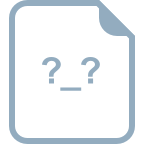
收起资源包目录

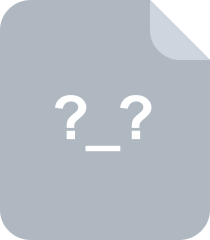
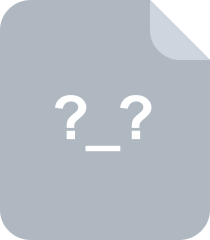
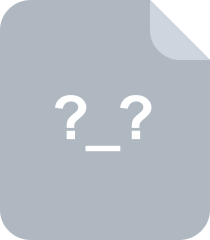
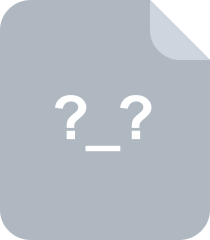
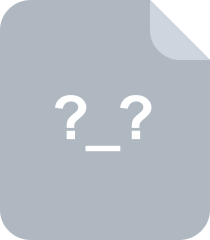
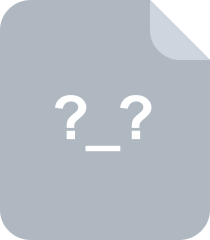
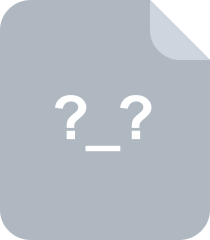
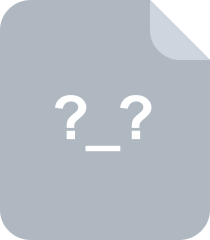
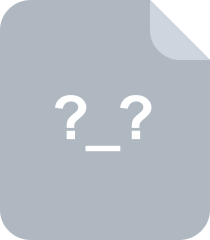
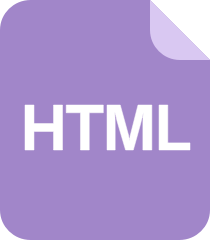
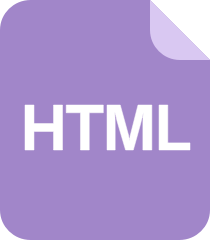
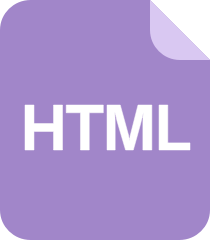
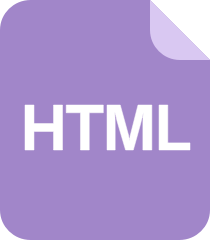
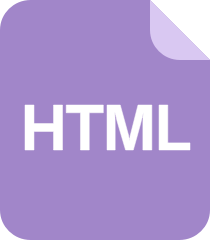
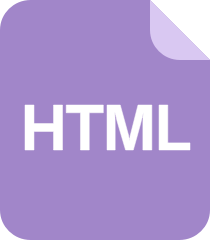
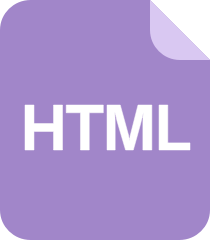
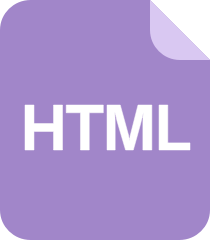
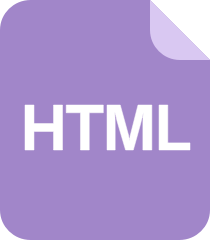
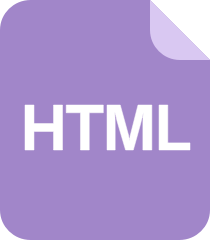
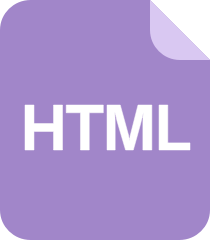
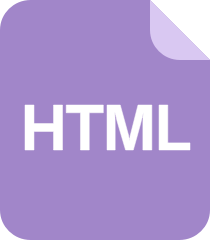
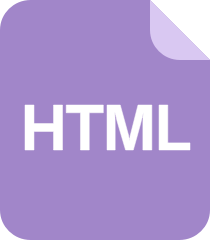
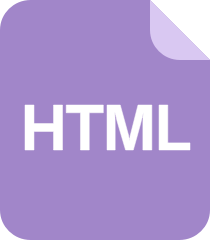
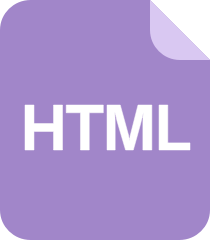
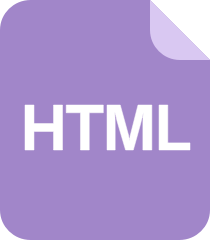
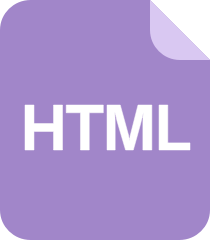
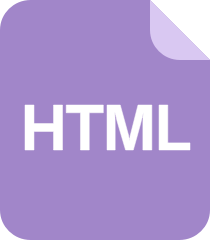
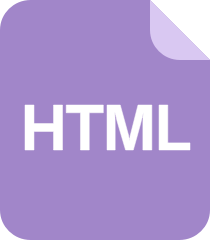
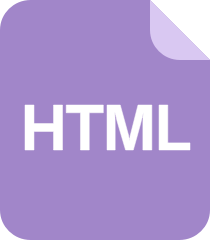
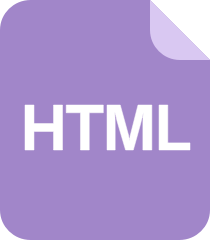
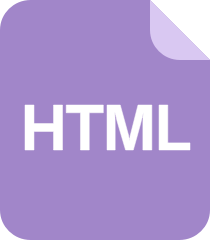
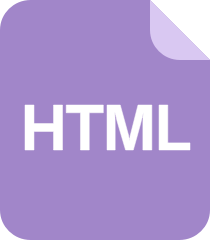
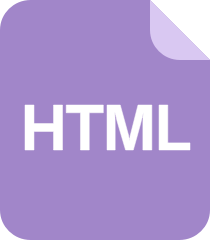
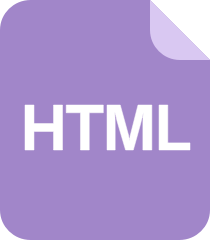
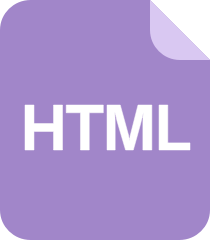
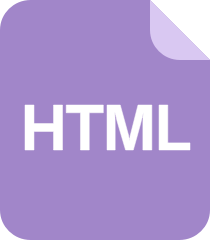
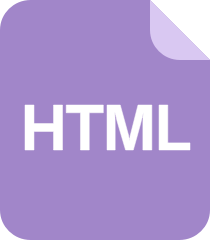
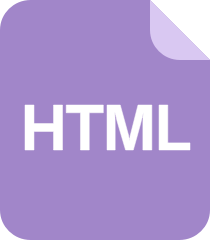
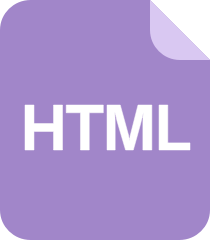
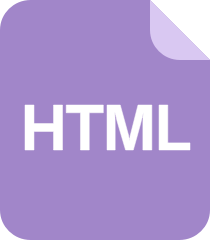
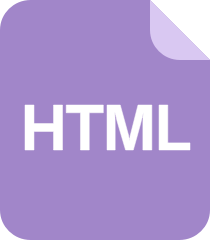
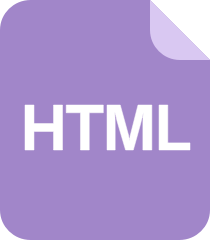
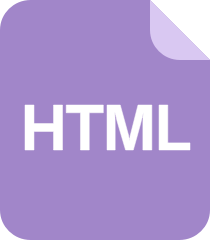
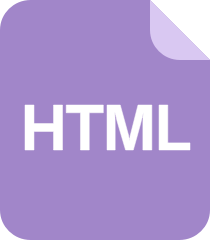
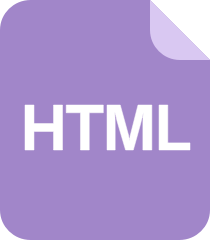
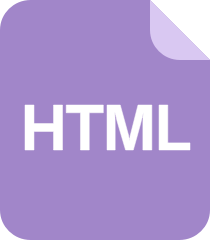
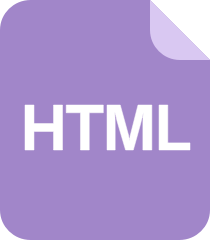
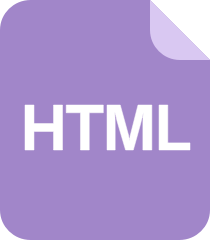
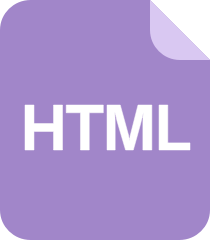
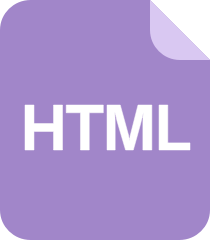
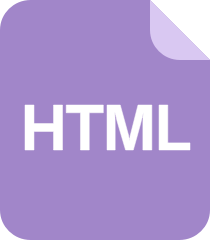
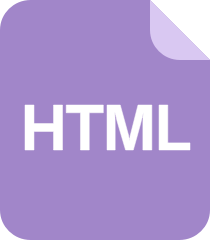
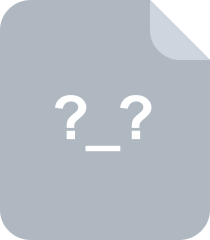
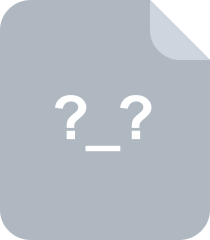
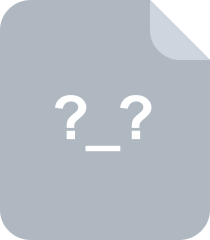
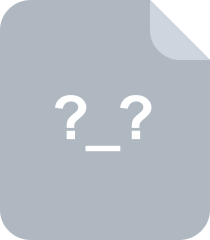
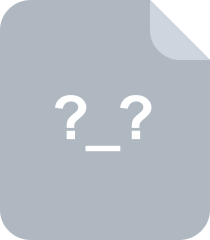
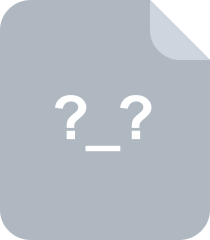
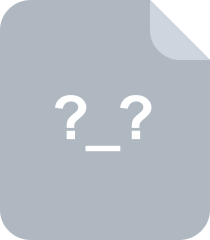
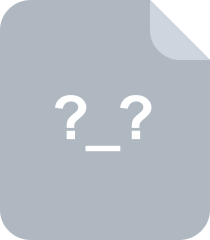
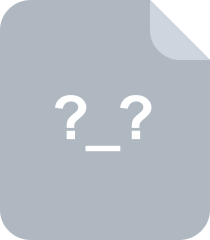
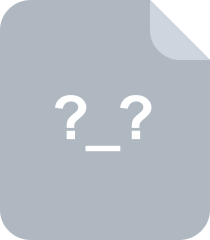
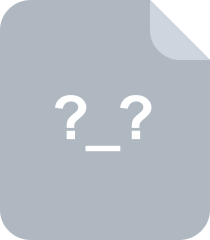
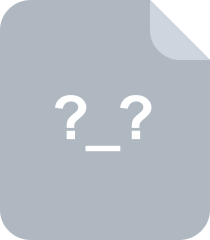
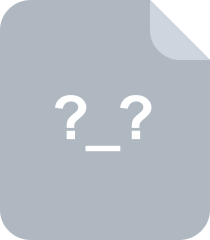
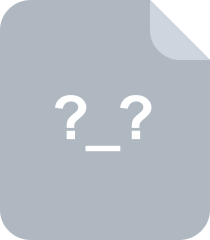
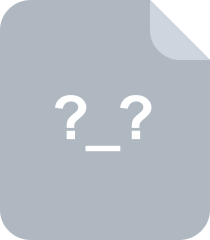
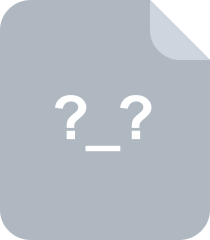
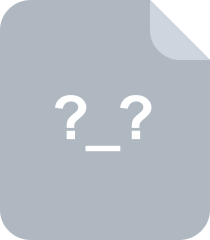
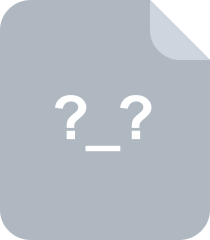
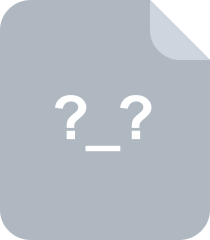
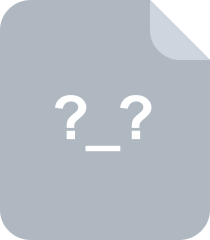
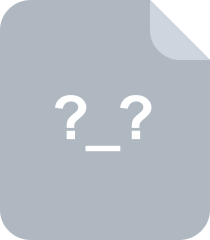
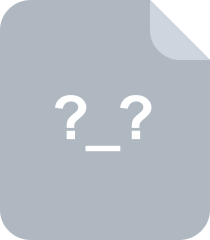
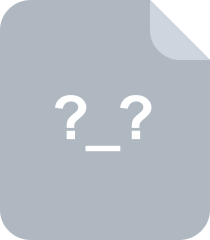
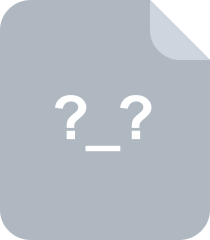
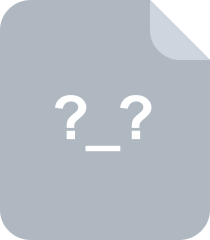
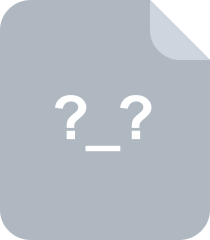
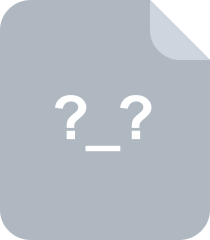
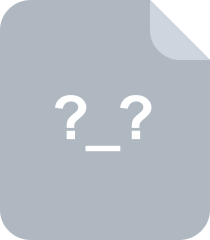
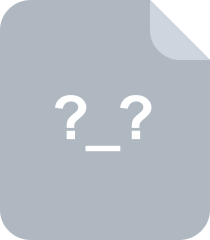
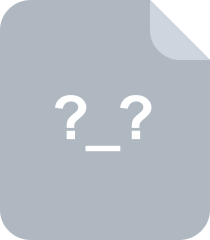
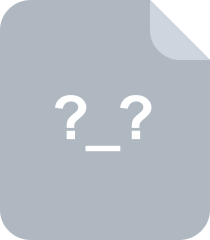
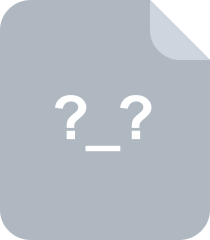
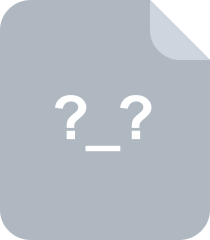
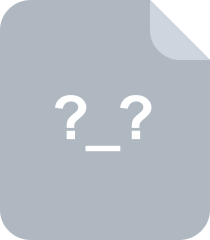
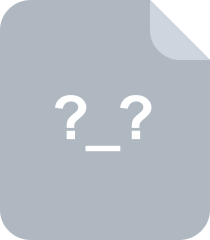
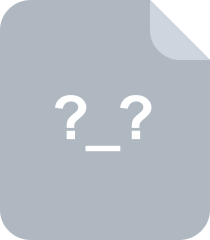
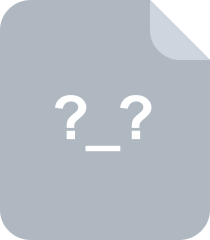
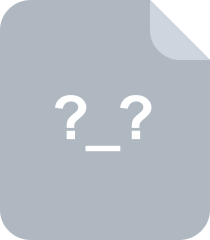
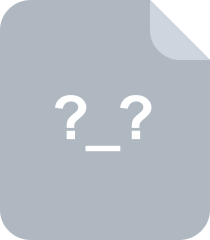
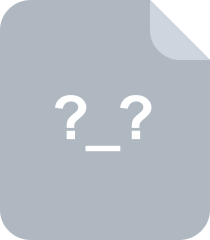
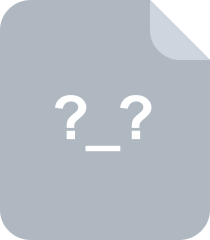
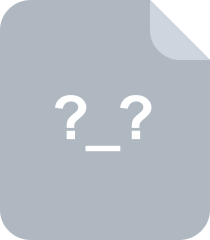
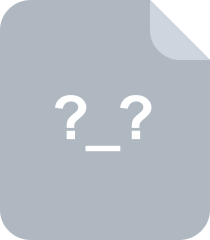
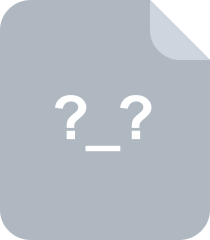
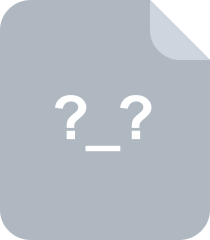
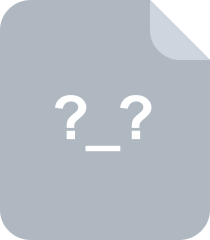
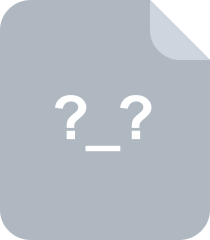
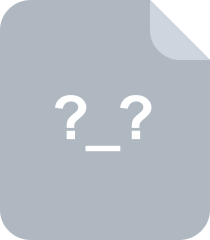
共 216 条
- 1
- 2
- 3
资源评论
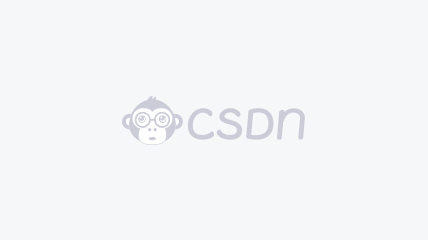

程序员Chino的日记
- 粉丝: 3717
- 资源: 5万+
上传资源 快速赚钱
我的内容管理 展开
我的资源 快来上传第一个资源
我的收益
登录查看自己的收益我的积分 登录查看自己的积分
我的C币 登录后查看C币余额
我的收藏
我的下载
下载帮助

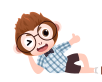
最新资源
- 基于Qt的上海地铁换乘系统详细文档+全部资料+高分项目.zip
- 发那科机器人二次开发 C#读取和写入数据,可以获取点位信息
- 基于QT的人脸识别,定位导航,脑电心率测算,用GPRS传到服务端的疲劳驾驶检测系统详细文档+全部资料+高分项目.zip
- 基于Qt的图书管理系统普通用户操作界面详细文档+全部资料+高分项目.zip
- 基于Qt的文件共享系统,类似百度网盘详细文档+全部资料+高分项目.zip
- 基于QT的网络视频监控系统详细文档+全部资料+高分项目.zip
- 基于QT的图书管理系统详细文档+全部资料+高分项目.zip
- 基于QT的学生成绩管理系统,QSS界面设计,SQL数据库的使用详细文档+全部资料+高分项目.zip
- 基于Qt的物业管理系统详细文档+全部资料+高分项目.zip
- 基于QT的直播管理系统详细文档+全部资料+高分项目.zip
- 基于Qt的学生信息管理系统、教师端:支持增删查改,班级成绩分析。学生端:查看成绩详细文档+全部资料+高分项目.zip
- 基于Qt的智能病房系统详细文档+全部资料+高分项目.zip
- 基于Qt构建的目标检测系统。基于dlib_rear_end_vehicles数据集详细文档+全部资料+高分项目.zip
- 基于QT的智能家居系统详细文档+全部资料+高分项目.zip
- 基于Qt和Mysql的教务管理系统详细文档+全部资料+高分项目.zip
- 基于Qt和mysql的大学生二手管理系统详细文档+全部资料+高分项目.zip
资源上传下载、课程学习等过程中有任何疑问或建议,欢迎提出宝贵意见哦~我们会及时处理!
点击此处反馈


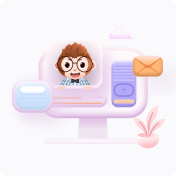
安全验证
文档复制为VIP权益,开通VIP直接复制
