The professional programmer’s Deitel guide to Python with introductory artificial intelligence case studies Written for programmers with a background in another high-level language, this book uses hands-on instruction to teach today’s most compelling, leading-edge computing technologies and programming in Python–one of the world’s most popular and fastest-growing languages. Please read the Table of Contents diagram inside the front cover and the Preface for more details. In the context of 500+, real-world examples ranging from individual snippets to 40 large scripts and full implementation case studies, you’ll use the interactive IPython interpreter with code in Jupyter Notebooks to quickly master the latest Python coding idioms. After covering Python Chapters 1—5 and a few key parts of Chapters 6—7, you’ll be able to handle significant portions of the hands-on introductory AI case studies in Chapters 11—16, which are loaded with cool, powerful, contemporary examples. These include natural language processing, data mining Twitter for sentiment analysis, cognitive computing with IBM Watson™, supervised machine learning with classification and regression, unsupervised machine learning with clustering, computer vision through deep learning and convolutional neural networks, deep learning with recurrent neural networks, big data with Hadoop, Spark™ and NoSQL databases, the Internet of Things and more. You’ll also work directly or indirectly with cloud-based services, including Twitter, Google Translate™, IBM Watson, Microsoft Azure, OpenMapQuest, PubNub and more. Features 500+ hands-on, real-world, live-code examples from snippets to case studies IPython + code in Jupyter Notebooks Library-focused: Uses Python Standard Library and data science libraries to accomplish significant tasks with minimal code Rich Python coverage: Control statements, functions, strings, files, JSON serialization, CSV, exceptions Procedural, functional-style and object-oriented programming Collections: Lists, tuples, dictionaries, sets, NumPy arrays, pandas Series & DataFrames Static, dynamic and interactive visualizations Data experiences with real-world datasets and data sources Intro to Data Science sections: AI, basic stats, simulation, animation, random variables, data wrangling, regression AI, big data and cloud data science case studies: NLP, data mining Twitter, IBM Watson™, machine learning, deep learning, computer vision, Hadoop, Spark™, NoSQL, IoT Open-source libraries: NumPy, pandas, Matplotlib, Seaborn, Folium, SciPy, NLTK, TextBlob, spaCy, Textatistic, Tweepy, scikit-learn, Keras and more.
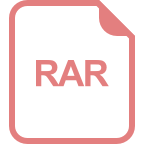
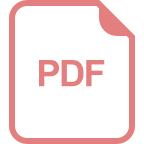
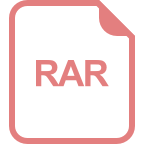
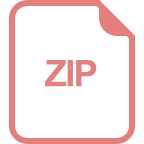
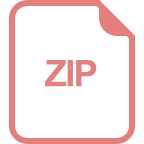
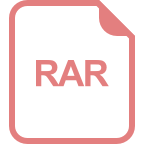
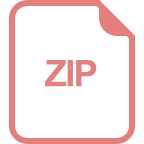
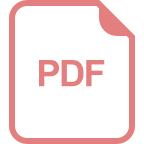
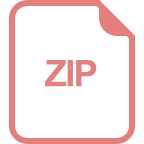
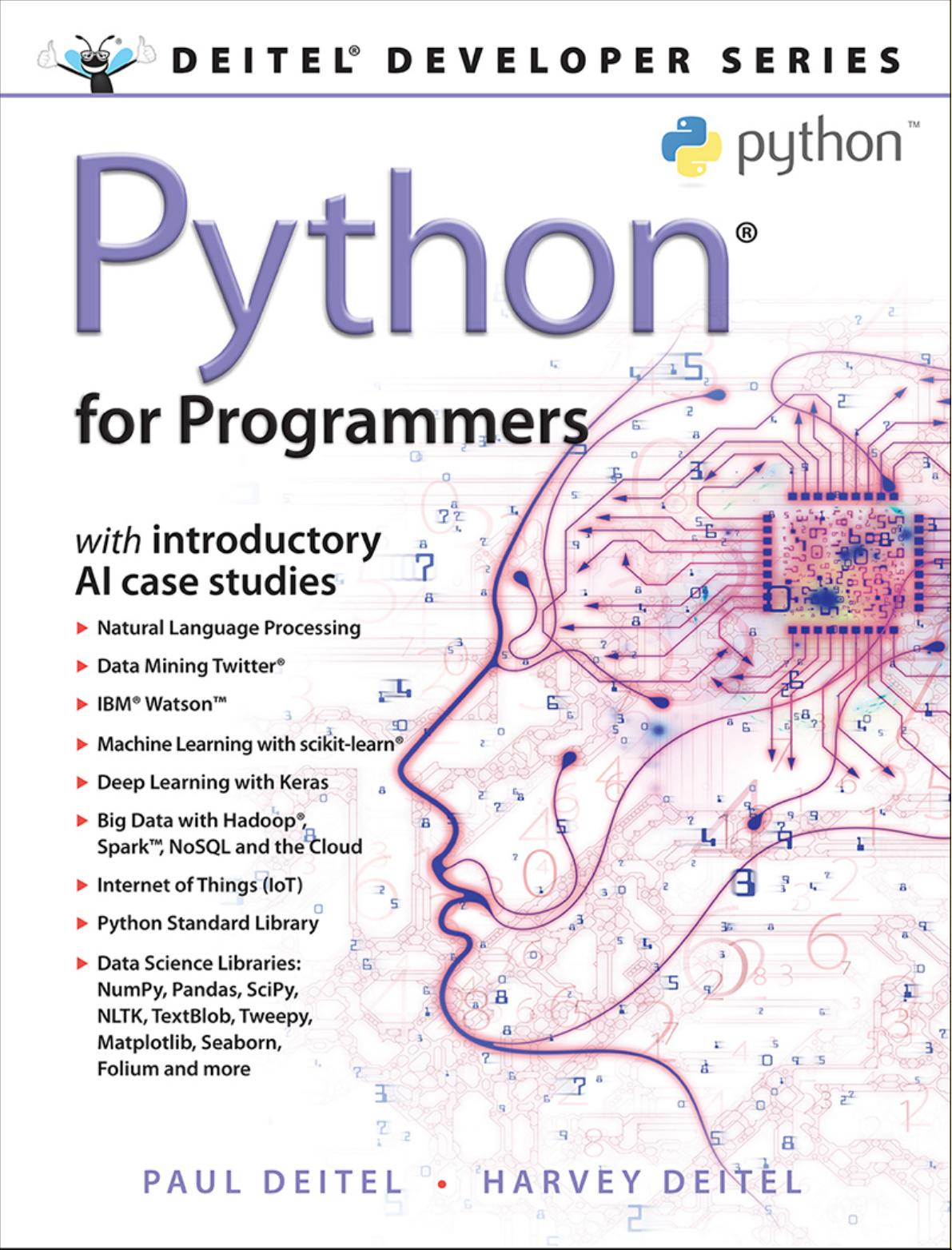
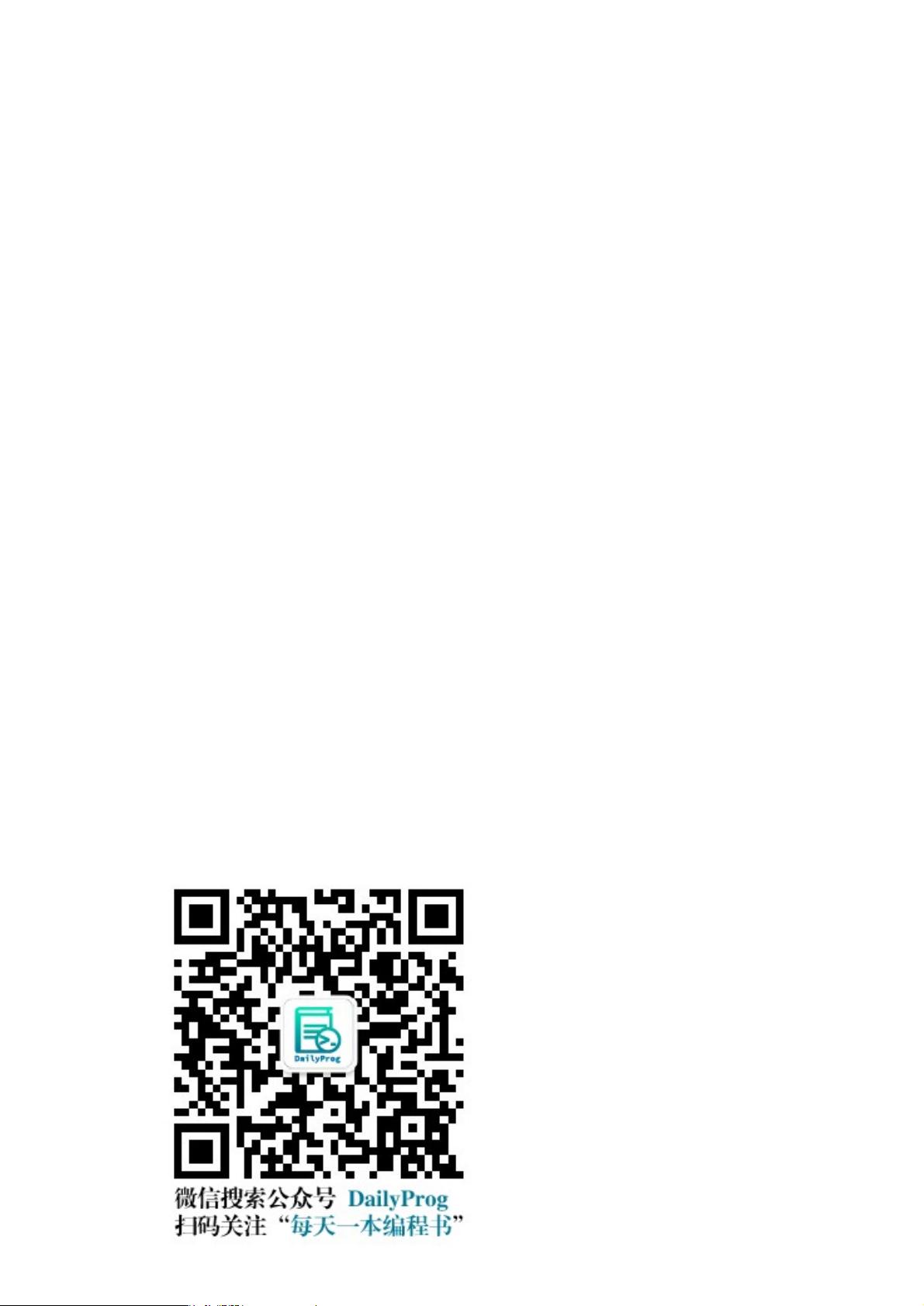
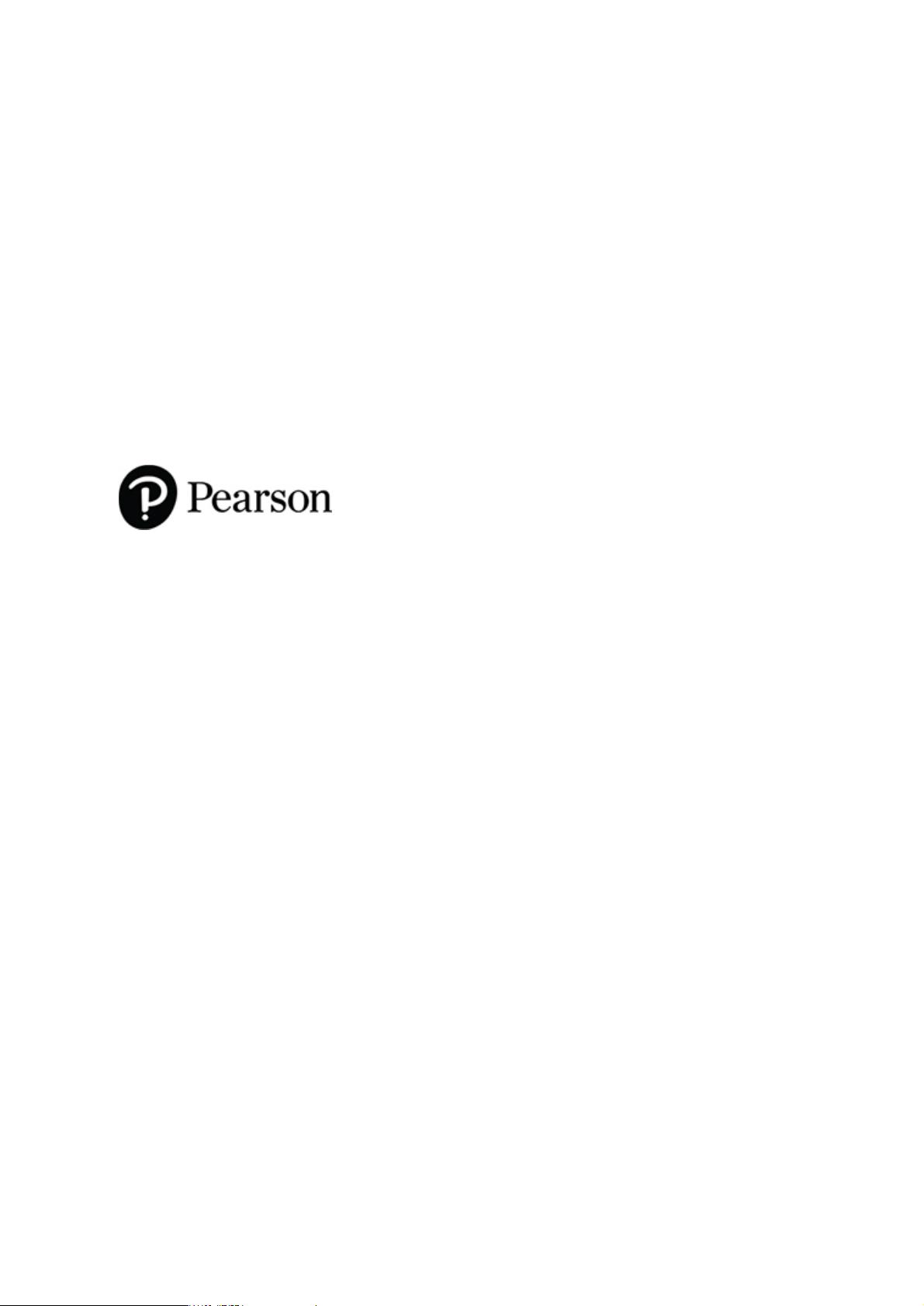
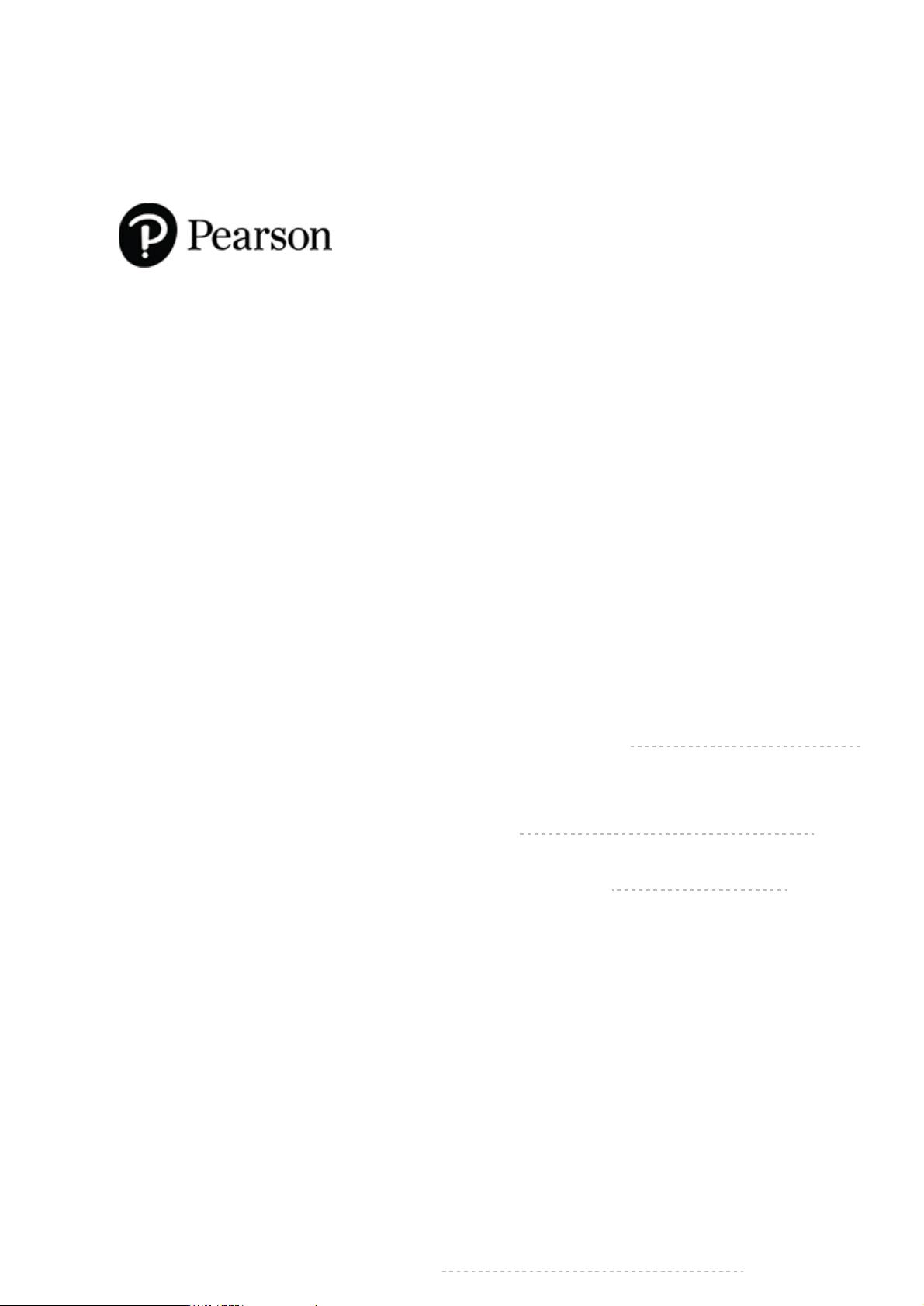
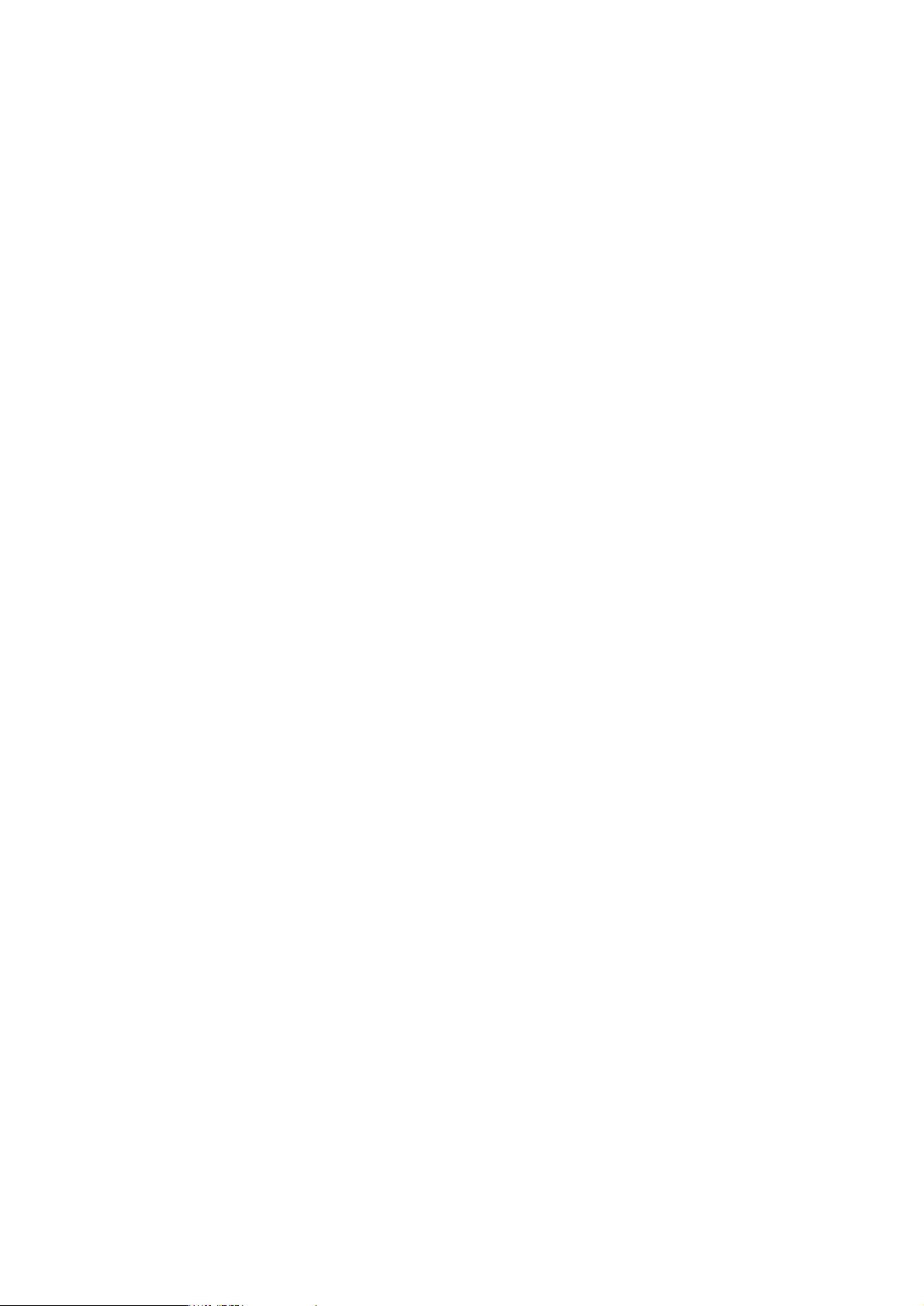
剩余809页未读,继续阅读
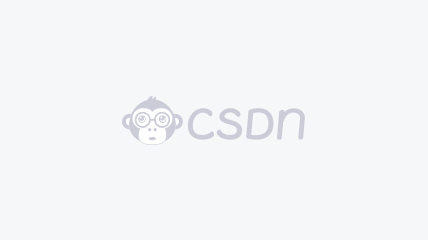
- MichaelHolmes2024-01-29书中文字不全,由的单词缺字母

- 粉丝: 5
- 资源: 111
我的内容管理 展开
我的资源 快来上传第一个资源
我的收益
登录查看自己的收益我的积分 登录查看自己的积分
我的C币 登录后查看C币余额
我的收藏
我的下载
下载帮助

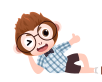
最新资源
- 基于Pygame库实现新年烟花效果的Python代码
- 浪漫节日代码 - 爱心代码、圣诞树代码
- 睡眠健康与生活方式数据集,睡眠和生活习惯关联分析()
- 国际象棋检测10-YOLO(v5至v9)、COCO、CreateML、Paligemma数据集合集.rar
- 100个情侣头像,唯美手绘情侣头像
- 自动驾驶不同工况避障模型(perscan、simulink、carsim联仿),能够避开预设的(静态)障碍物
- 使用Python和Pygame实现圣诞节动画效果
- 数据分析-49-客户细分-K-Means聚类分析
- 车辆轨迹自适应预瞄跟踪控制和自适应p反馈联合控制,自适应预苗模型和基于模糊p控制均在simulink中搭建 个人觉得跟踪效果相比模糊pid效果好很多,轨迹跟踪过程,转角控制平滑自然,车速在36到72
- 企业可持续发展性数据集,ESG数据集,公司可持续发展性数据(可用于多种企业可持续性研究场景)

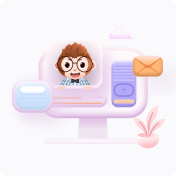
