
Leo青山
- 粉丝: 1
- 资源: 1
最新资源
- 【SEC-2025行业研究报告】Form 10-K Blue Owl Credit Income Corp. .pdf
- 【SEC-2025行业研究报告】Form 10-K CEDAR REALTY TRUST, INC. .pdf
- 【SEC-2025行业研究报告】Form 10-K ChromaDex Corp. .pdf
- 【SEC-2025行业研究报告】Form 10-K BRIGHTHOUSE LIFE INSURANCE Co .pdf
- 【SEC-2025行业研究报告】Form 10-K CPI Card Group Inc. .pdf
- 【SEC-2025行业研究报告】Form 10-K Custom Truck One Source, Inc. .pdf
- 【SEC-2025行业研究报告】Form 10-K Crexendo, Inc. .pdf
- 【SEC-2025行业研究报告】Form 10-K Cyclerion Therapeutics, Inc. .pdf
- 【SEC-2025行业研究报告】Form 10-K Dave Inc. DE .pdf
- 【SEC-2025行业研究报告】Form 10-K Emergent BioSolutions Inc. .pdf
- 【SEC-2025行业研究报告】Form 10-K Eos Energy Enterprises, Inc. .pdf
- 【SEC-2025行业研究报告】Form 10-K Evolus, Inc. .pdf
- 【SEC-2025行业研究报告】Form 10-K FLAGSTAR FINANCIAL, INC. .pdf
- 【SEC-2025行业研究报告】Form 10-Q NKGen Biotech, Inc. .pdf
- 【SEC-2025行业研究报告】Form 10-Q DONALDSON Co INC .pdf
- 【SEC-2025行业研究报告】Form 10-K Flutter Entertainment plc .pdf
资源上传下载、课程学习等过程中有任何疑问或建议,欢迎提出宝贵意见哦~我们会及时处理!
点击此处反馈


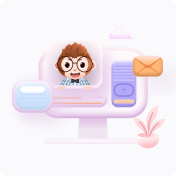