#define _CRT_SECURE_NO_WARNINGS
#include <iostream>
#include <fstream>
#include <string>
#include <opencv2/imgproc.hpp>
#include <opencv2/highgui.hpp>
//#include <cuda_provider_factory.h>
#include <onnxruntime_cxx_api.h>
using namespace cv;
using namespace std;
using namespace Ort;
class LSTR
{
public:
LSTR();
Mat detect(Mat& cv_image);
~LSTR(); // 析构函数, 释放内存
private:
void normalize_(Mat img);
int inpWidth;
int inpHeight;
vector<float> input_image_;
vector<float> mask_tensor;
float mean[3] = { 0.485, 0.456, 0.406 };
float std[3] = { 0.229, 0.224, 0.225 };
const int len_log_space = 50;
float* log_space;
const Scalar lane_colors[8] = { Scalar(68,65,249), Scalar(44,114,243),Scalar(30,150,248),Scalar(74,132,249),Scalar(79,199,249),Scalar(109,190,144),Scalar(142, 144, 77),Scalar(161, 125, 39) };
Env env = Env(ORT_LOGGING_LEVEL_ERROR, "LSTR");
Ort::Session *ort_session = nullptr;
SessionOptions sessionOptions = SessionOptions();
vector<char*> input_names;
vector<char*> output_names;
vector<vector<int64_t>> input_node_dims; // >=1 outputs
vector<vector<int64_t>> output_node_dims; // >=1 outputs
};
LSTR::LSTR()
{
string model_path = "lstr_360x640.onnx";
std::wstring widestr = std::wstring(model_path.begin(), model_path.end());
//OrtStatus* status = OrtSessionOptionsAppendExecutionProvider_CUDA(sessionOptions, 0);
sessionOptions.SetGraphOptimizationLevel(ORT_ENABLE_BASIC);
ort_session = new Session(env, widestr.c_str(), sessionOptions);
size_t numInputNodes = ort_session->GetInputCount();
size_t numOutputNodes = ort_session->GetOutputCount();
AllocatorWithDefaultOptions allocator;
for (int i = 0; i < numInputNodes; i++)
{
input_names.push_back(ort_session->GetInputName(i, allocator));
Ort::TypeInfo input_type_info = ort_session->GetInputTypeInfo(i);
auto input_tensor_info = input_type_info.GetTensorTypeAndShapeInfo();
auto input_dims = input_tensor_info.GetShape();
input_node_dims.push_back(input_dims);
}
for (int i = 0; i < numOutputNodes; i++)
{
output_names.push_back(ort_session->GetOutputName(i, allocator));
Ort::TypeInfo output_type_info = ort_session->GetOutputTypeInfo(i);
auto output_tensor_info = output_type_info.GetTensorTypeAndShapeInfo();
auto output_dims = output_tensor_info.GetShape();
output_node_dims.push_back(output_dims);
}
this->inpHeight = input_node_dims[0][2];
this->inpWidth = input_node_dims[0][3];
this->mask_tensor.resize(this->inpHeight * this->inpWidth, 0.0);
log_space = new float[len_log_space];
FILE* fp = fopen("log_space.bin", "rb");
fread(log_space, sizeof(float), len_log_space, fp);//导入数据
fclose(fp);//关闭文件。
}
LSTR::~LSTR()
{
delete[] log_space;
log_space = NULL;
}
void LSTR::normalize_(Mat img)
{
//img.convertTo(img, CV_32F);
int row = img.rows;
int col = img.cols;
this->input_image_.resize(row * col * img.channels());
for (int c = 0; c < 3; c++)
{
for (int i = 0; i < row; i++)
{
for (int j = 0; j < col; j++)
{
float pix = img.ptr<uchar>(i)[j * 3 + c];
this->input_image_[c * row * col + i * col + j] = (pix / 255.0 - mean[c]) / std[c];
}
}
}
}
Mat LSTR::detect(Mat& srcimg)
{
const int img_height = srcimg.rows;
const int img_width = srcimg.cols;
Mat dstimg;
resize(srcimg, dstimg, Size(this->inpWidth, this->inpHeight), INTER_LINEAR);
this->normalize_(dstimg);
array<int64_t, 4> input_shape_{ 1, 3, this->inpHeight, this->inpWidth };
array<int64_t, 4> mask_shape_{ 1, 1, this->inpHeight, this->inpWidth };
auto allocator_info = MemoryInfo::CreateCpu(OrtDeviceAllocator, OrtMemTypeCPU);
vector<Value> ort_inputs;
ort_inputs.push_back(Value::CreateTensor<float>(allocator_info, input_image_.data(), input_image_.size(), input_shape_.data(), input_shape_.size()));
ort_inputs.push_back(Value::CreateTensor<float>(allocator_info, mask_tensor.data(), mask_tensor.size(), mask_shape_.data(), mask_shape_.size()));
// ¿ªÊ¼ÍÆÀí
vector<Value> ort_outputs = ort_session->Run(RunOptions{ nullptr }, input_names.data(), ort_inputs.data(), 2, output_names.data(), output_names.size());
const float* pred_logits = ort_outputs[0].GetTensorMutableData<float>();
const float* pred_curves = ort_outputs[1].GetTensorMutableData<float>();
const int logits_h = output_node_dims[0][1];
const int logits_w = output_node_dims[0][2];
const int curves_w = output_node_dims[1][2];
vector<int> good_detections;
vector< vector<Point>> lanes;
for (int i = 0; i < logits_h; i++)
{
float max_logits = -10000;
int max_id = -1;
for (int j = 0; j < logits_w; j++)
{
const float data = pred_logits[i*logits_w + j];
if (data > max_logits)
{
max_logits = data;
max_id = j;
}
}
if (max_id == 1)
{
good_detections.push_back(i);
const float *p_lane_data = pred_curves + i * curves_w;
vector<Point> lane_points(len_log_space);
for (int k = 0; k < len_log_space; k++)
{
const float y = p_lane_data[0] + log_space[k] * (p_lane_data[1] - p_lane_data[0]);
const float x = p_lane_data[2] / powf(y - p_lane_data[3], 2.0) + p_lane_data[4] / (y - p_lane_data[3]) + p_lane_data[5] + p_lane_data[6] * y - p_lane_data[7];
lane_points[k] = Point(int(x*img_width), int(y*img_height));
}
lanes.push_back(lane_points);
}
}
/// draw lines
vector<int> right_lane;
vector<int> left_lane;
for (int i = 0; i < good_detections.size(); i++)
{
if (good_detections[i] == 0)
{
right_lane.push_back(i);
}
if (good_detections[i] == 5)
{
left_lane.push_back(i);
}
}
Mat visualization_img = srcimg.clone();
if (right_lane.size() == left_lane.size())
{
Mat lane_segment_img = visualization_img.clone();
vector<Point> points = lanes[right_lane[0]];
reverse(points.begin(), points.end());
points.insert(points.begin(), lanes[left_lane[0]].begin(), lanes[left_lane[0]].end());
fillConvexPoly(lane_segment_img, points, Scalar(0, 191, 255));
addWeighted(visualization_img, 0.7, lane_segment_img, 0.3, 0, visualization_img);
}
for (int i = 0; i < lanes.size(); i++)
{
for (int j = 0; j < lanes[i].size(); j++)
{
circle(visualization_img, lanes[i][j], 3, lane_colors[good_detections[i]], -1);
}
}
return visualization_img;
}
int main()
{
LSTR mynet;
string imgpath = "images/0.jpg";
Mat srcimg = imread(imgpath);
Mat dstimg = mynet.detect(srcimg);
static const string kWinName = "Deep learning lane detection in ONNXRuntime";
namedWindow(kWinName, WINDOW_NORMAL);
imshow(kWinName, dstimg);
waitKey(0);
destroyAllWindows();
}
没有合适的资源?快使用搜索试试~ 我知道了~
人工智能-Transformer-使用ONNXRuntime部署LSTR基于Transformer的端到端实时车道线检测
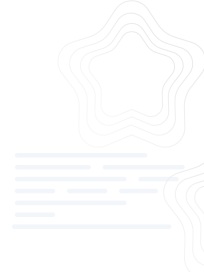
共8个文件
jpg:4个
py:1个
bin:1个

1.该资源内容由用户上传,如若侵权请联系客服进行举报
2.虚拟产品一经售出概不退款(资源遇到问题,请及时私信上传者)
2.虚拟产品一经售出概不退款(资源遇到问题,请及时私信上传者)
版权申诉
0 下载量 63 浏览量
2024-06-05
13:43:17
上传
评论
收藏 3.51MB ZIP 举报
温馨提示
使用ONNXRuntime部署LSTR基于Transformer的端到端实时车道线检测,包含C++和Python两个版本的程序。 onnx文件的大小只有2.93M,可以做到实时性轻量化部署。 起初,我想使用opencv做部署的,但是opencv的dnn模块读取onnx文件出错, 无赖只能使用onnxruntime做部署了。
资源推荐
资源详情
资源评论
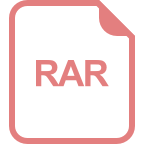
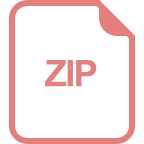
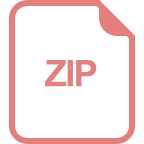
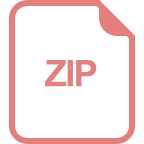
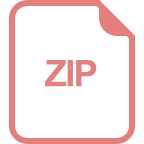
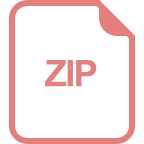
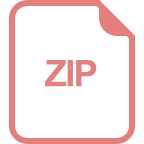
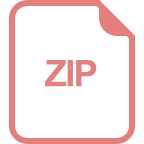
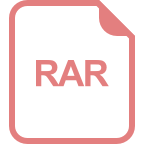
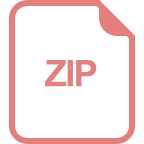
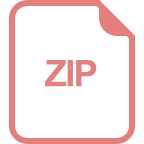
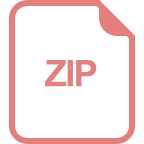
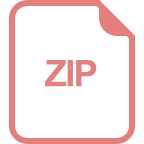
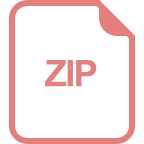
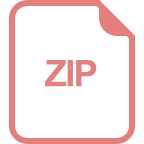
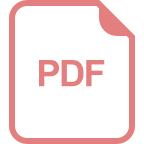
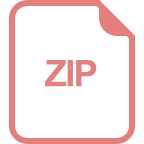
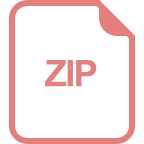
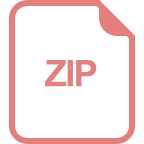
收起资源包目录


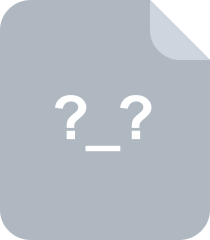
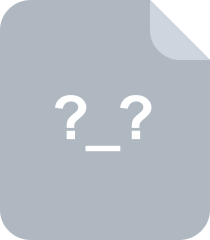

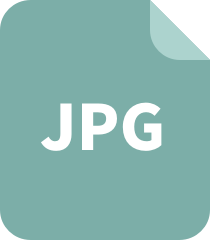
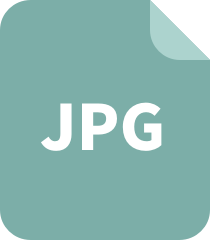
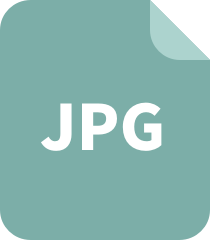
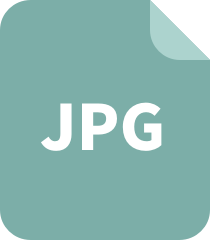
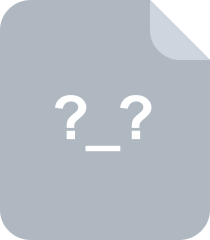
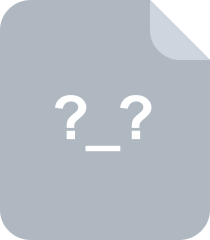
共 8 条
- 1
资源评论
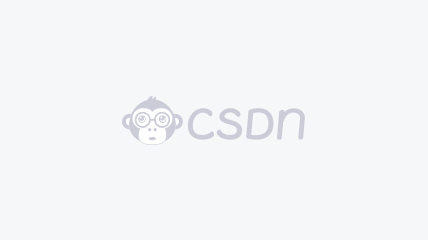

博士僧小星
- 粉丝: 2262
- 资源: 5991
上传资源 快速赚钱
我的内容管理 展开
我的资源 快来上传第一个资源
我的收益
登录查看自己的收益我的积分 登录查看自己的积分
我的C币 登录后查看C币余额
我的收藏
我的下载
下载帮助

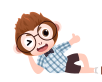
安全验证
文档复制为VIP权益,开通VIP直接复制
