clc
clear
close all
%%房产
load maydata.mat
n = randperm(length(numg));
m=500;
input_train =numg(n(1:m),1:pan1 )';%训练数据输出数
output_train = numg(n(1:m),pan1+1)';%训练数据输入数据
input_test = numg((m+1:end),1:pan1)';%测试数据输出数据
output_test = numg((m+1:end),pan1+1)';%测试数据输入数据
[inputn,inputps]=mapminmax(input_train,-1,1);%训练数据的输入数据的归一化
[outputn,outputps]=mapminmax(output_train,-1,1);%训练数据的输出数据的归一化de
inputn_test=mapminmax('apply',input_test,inputps);
%% Define Network Architecture
% Define the network architecture.
numFeatures = pan1;%输入层维度
numResponses = 1;%输出维度
% 200 hidden units
numHiddenUnits = 50;%第一层维度
% a fully connected layer of size 50 & a dropout layer with dropout probability 0.5
layers = [ ...
sequenceInputLayer(numFeatures)%输入层
lstmLayer(numHiddenUnits,'OutputMode','sequence')%第一层
fullyConnectedLayer(100)%链接层
dropoutLayer(0.01)%遗忘层
fullyConnectedLayer(numResponses)%链接层
regressionLayer];%回归层
% Specify the training options.
% Train for 60 epochs with mini-batches of size 20 using the solver 'adam'
maxEpochs = 60;%最大迭代次数
miniBatchSize =2;%最小批量
% the learning rate == 0.01
% set the gradient threshold to 1
% set 'Shuffle' to 'never'
options = trainingOptions('adam', ... %解算器
'MaxEpochs',maxEpochs, ... %最大迭代次数
'MiniBatchSize',miniBatchSize, ... %最小批次
'InitialLearnRate',0.01, ... %初始学习率
'GradientThreshold',0.01, ... %梯度阈值
'Shuffle','every-epoch', ... %打乱顺序
'Plots','training-progress',... %画图
'Verbose',0); %不输出训练过程
%% Train the Network
net = trainNetwork(inputn,outputn,layers,options);%开始训练
% save maydata.mat
%% Test the Network
y_pred = predict(net,inputn_test,'MiniBatchSize',miniBatchSize)';%测试仿真输出
[y_pred]=(mapminmax('reverse',y_pred',outputps));
% y_pred0 = predict(net,inputn,'MiniBatchSize',1)';%训练拟合值
% y_pred0=(mapminmax('reverse',y_pred0',outputps))';
y_pred=double(y_pred);
% zz=(mapminmax('reverse',zz,inputps));
figure%打开一个图像窗口
plot(output_test,'r-')%红色实线,点的形状为o
hold on
plot(y_pred,'b--')%黑色实线,点的形状为*
hold on%继续画图
hold off%停止画图测试图')%Y轴名称
legend('实际值','预测输出')%标签
ylabel('工业电力负荷')
set(gca,'fontsize',12)
error1 = y_pred-output_test;%误差
figure
plot(error1,'k-*')
title('测试误差图')
R_2 = R_2(output_test,y_pred)
[MSE,RMSE,MBE,MAE ] =MSE_RMSE_MBE_MAE(output_test,y_pred);
result_table = table;
result_table.simd = y_pred';
result_table.trued = output_test';
writetable(result_table,'工业结果2.csv')
没有合适的资源?快使用搜索试试~ 我知道了~
温馨提示
基于MATLAB编程,用长短期神经网络LSTM的电力负荷预测,基于长短期神经网络LSTM的电力负荷回归分析,电力负荷是一种时间序列的数据,用LSTM比一般神经网络更适合,代码完整,包含数据,有注释,方便扩展应用 1,如有疑问,不会运行,可以私信, 2,需要创新,或者修改可以扫描二维码联系博主, 3,本科及本科以上可以下载应用或者扩展, 4,内容不完全匹配要求或需求,可以联系博主扩展。
资源推荐
资源详情
资源评论
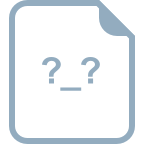
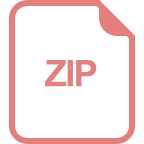
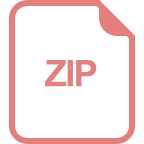
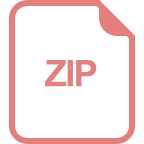
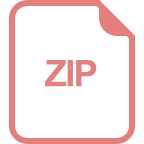
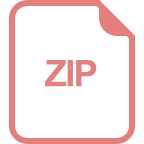
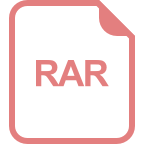
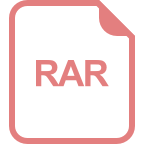
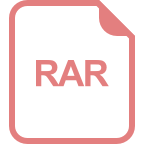
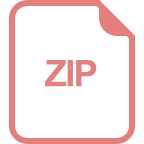
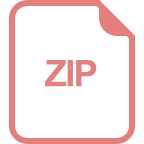
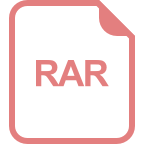
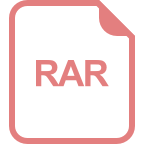
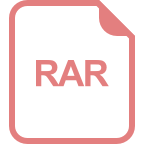
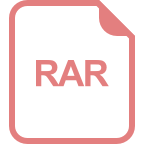
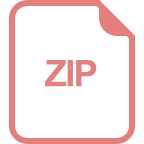
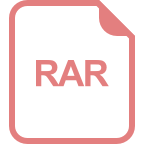
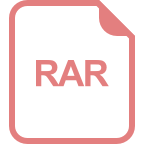
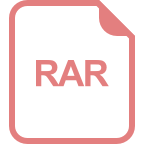
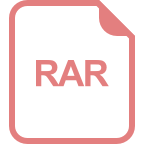
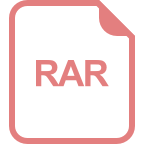
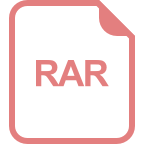
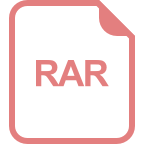
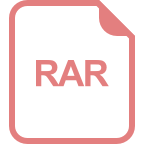
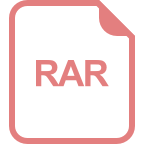
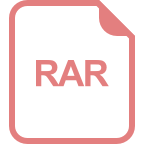
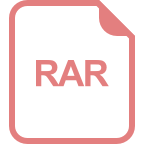
收起资源包目录

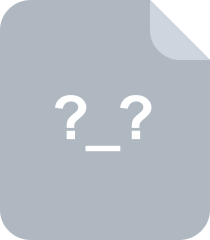
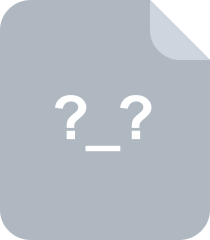
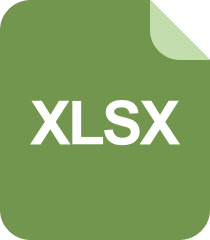
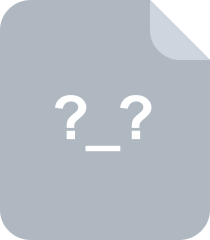
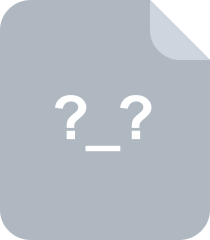
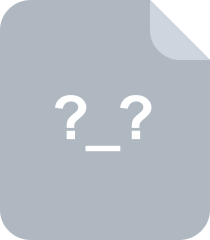
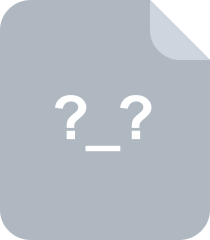
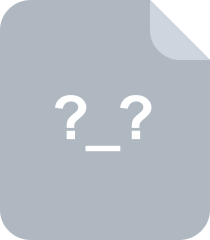
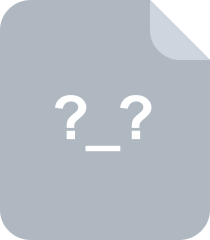
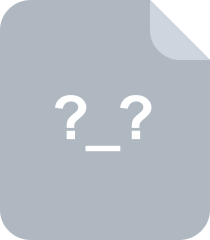
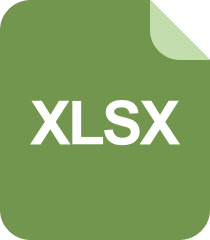
共 11 条
- 1
资源评论
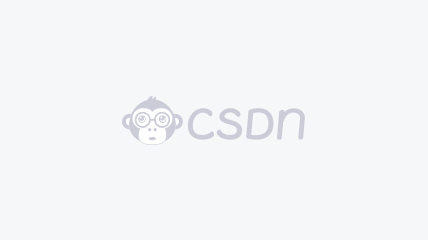
- m0_751088582024-04-17超赞的资源,感谢资源主分享,大家一起进步!
- Astronaut_XXY2024-04-23资源有一定的参考价值,与资源描述一致,很实用,能够借鉴的部分挺多的,值得下载。


神经网络机器学习智能算法画图绘图
- 粉丝: 2481
- 资源: 603
上传资源 快速赚钱
我的内容管理 展开
我的资源 快来上传第一个资源
我的收益
登录查看自己的收益我的积分 登录查看自己的积分
我的C币 登录后查看C币余额
我的收藏
我的下载
下载帮助

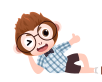
安全验证
文档复制为VIP权益,开通VIP直接复制
