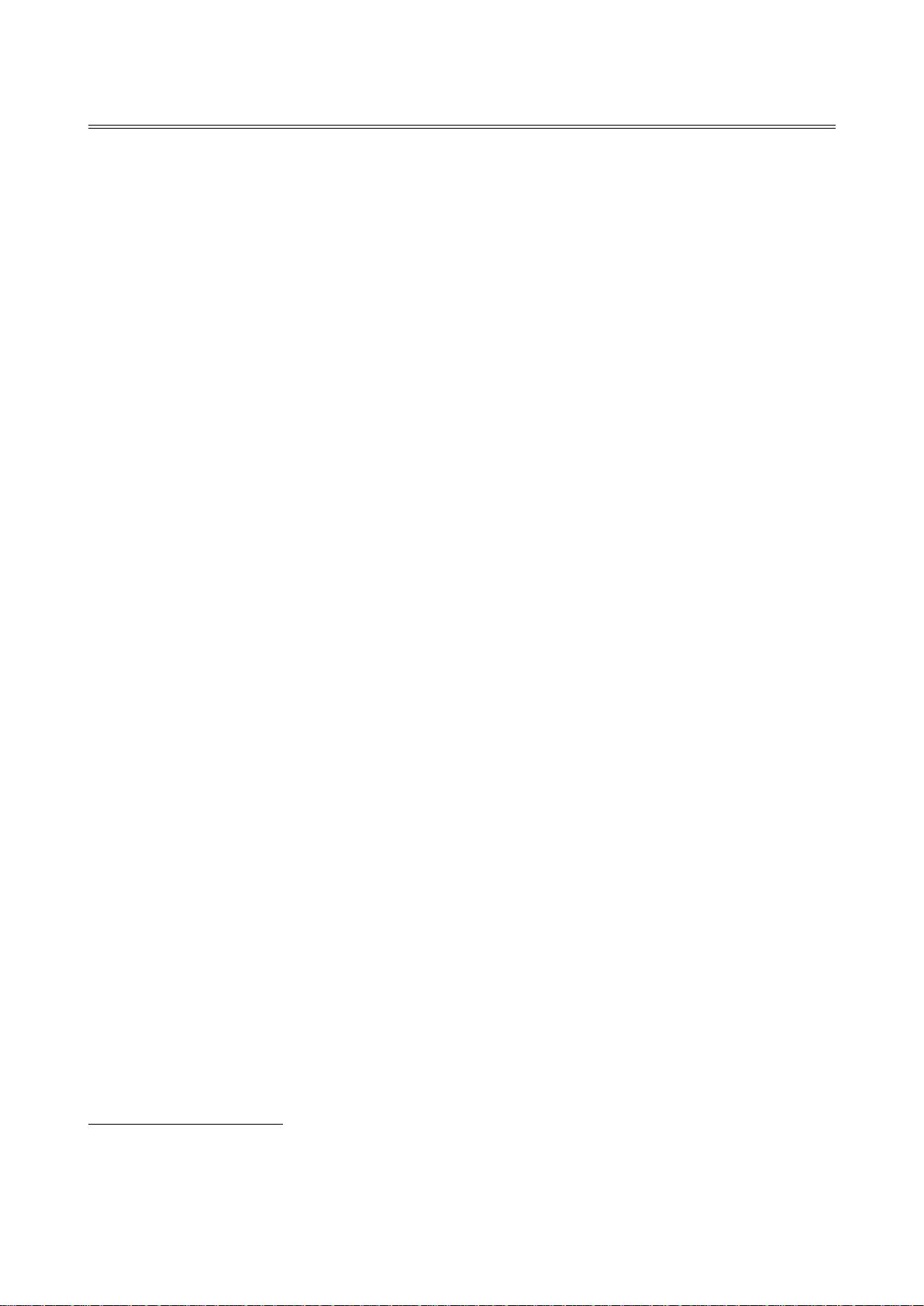
水
利水电科技进展
,2020,40( 6) Tel: 025
-
83786335 E-mail: jz@ hhu.edu.cn http: / /jour.hhu.edu.cn
第
40
卷
第
6
期
Vol.40 No.6
水利水电科技进展
Advances in Science and Technology of Water Resources
2020
年
11
月
Nov. 2020
基
金项目
:
国家重点研发计划
( 2017YFC1502603)
;
江
苏水利厅项目
( 2018058)
作者简介
:
王亦斌
( 1970—)
,
女
,
高
级工程师
,
主要从事水利工程建设与管理研究
。E-mail: 821983828@ qq.com
通信作者
:
梁雪春
( 1969—)
,
女
,
教
授
,
博士
,
主要从事复杂系统建模与决策分析研究
。E-mail: liangxuechun@ njtech.edu.cn
DOI: 10.3880/j.issn.1006
-
7647.2020.06.008
基于
EMD-LSTM
模型的河流水量水位预测
王
亦斌
1
,
孙 涛
1
,
梁
雪春
2
,
谢
海洋
1
( 1.
南水北调东线江苏水源有限责任公司
,
江
苏 南京
210019;
2.
南京工业大学电气工程与控制科学学院
,
江苏 南京
211816)
摘
要
:
基于经验模式分解方法和长短期记忆网络
( empirical model decomposition and long short-term
memory network,EMD-LSTM)
模型对水位数据进行预测
。
先采用中值滤波对数据序列进行预处
理
,
然后对数据序列进行
EMD
分解
,
并对
EMD
分解的每个特征序列使用
LSTM
模型进行预测
,
最
后叠加各个序列预测值
,
得到最终的预测结果
。
以南水北调工程某河流每隔
1 h
的瞬时流量
、
流速
和水深监测数据为研究对象
,
采用
EMD-LSTM
模型进行建模
,
试验结果表明
,
该模型能够实现水
位
、
水速和瞬时流量连续
12 h
和
6 h
的准确预测
,
且比
LSTM
模型具有更高的预测精度
,
可为水位预
判和水资源的实时调度提供决策依据
。
关键词
:
水量预测
;
水位预测
;
中值滤波
;
经验模式分解方法
;
长短期记忆神经网络
中图分类号
: TV125
文献标志码
: A
文章编号
: 1006
-
7647( 2020) 06
-
0040
-
08
Prediction of river water flow and water level based on EMD-LSTM model / /WANG Yibin
1
,SUN Tao
1
,LIANG
Xuechun
2
,XIE Haiyang
1
( 1. Eastern Route of South-to-North Water Diversion Project Jiangsu Water Resources Co.,Ltd.,
Nanjing 210019,China; 2. College of Electrical Engineering and Control Science,Nanjing Tech University,Nanjing
211816,China)
Abstract: The combination of empirical model decomposition and long short-term memory network ( EMD-LSTM) model
was used to forecast the hydrological time series data. The median filter for data preprocessing was used first and then EMD
was applied to decompose the time sequence. The LSTM model was used to prediction for each characteristic series from
EMD,and each prediction sequence was superposed to obtain the final prediction result. Based on the data of the
instantaneous flow,water velocity and water level per hour of a certain river in the South-to-North Water Diversion Project,
the EMD-LSTM was used for modeling. The experimental results show that this method can realize accurate prediction of
water level,water velocity and instantaneous flow for 12 h and 6 h continuously,has higher accuracy compared with the
LSTM model and provides decision basis for the forecast of hydrological time series and the real-time dispatching of water
resources.
Key words: water flow prediction; water level prediction; median filter; empirical mode decomposition; long short-term
memory network
准确的水位和水量等水文时间序列预测是水资
源管理的重要依据
,
对于调水监测有着重要的作用
。
传统的水文时
间序列预测方法有回归分析
、
时间序
列分析等
,
还有模糊识别法
、
灰色模型
、
小波神经网
络分析
[1-5]
。
随着机器学习方法的兴起
,
很
多学者使
用机器学习方法进行建模
,
相对于传统的统计预测
模型
,
机器学习方法具有更好的适应性
。
面对数据
量化不确定性问题
,Porter
等
[6]
提出一种人工神经
网
络
( artificial neural networks,ANN)
方法来预测地
下水水位
;
针对在台风期间受潮汐影响水位波动较
大问题
,Wei
[7]
提
出了小波
-
支持向量机算法来预测
每小时的水位数据
,
为台风袭击期间的水位预测问
题提供了实用的解决方案
; Moosavi
等
[8]
提
出了小波
-
自适应神经模糊推理系统
( wavelet-adaptive neuro-
fuzzy inference system,wavelet -ANIS)
方法
,
比较 了
ANN、Wavelet-ANN、ANIS
和
Wavelet-ANIS
在地下水
位预报上的表现
; Zhong
等
[9]
分析了长江潮汐水位
预
测的复杂性
,
在获取了长江下游
(
安庆
、
芜湖
、
南
·04·