
机器未来
- 粉丝: 1w+
- 资源: 2
最新资源
- CAD安装插件程序和全部左手键命令表文档
- 《机顶盒刷机固件大全》.xlsx
- Labiew噪音与振动检测模块源码,改功能模块已运用到实际项目,原理是利用傅里叶变和倍频程实现的,产品一旦发概不 需要的可以联系哟
- MATLAB代码实现凝固相场模拟-凝固模型,各向异性枝晶生长 纯物质凝固模型,激光增材制造,选择性激光熔融,SLM,凝固,铸造,焊接等等多种耦合场景 好评提供讲解video~提供讲解video~提
- ESP8266+SG90+点灯科技+小爱同学实现关灯
- 自动驾驶,carsim simulink联合仿真,基于mpc算法的acc自适应巡航控制,根据acc求解出期望车速后,用mpc算法计算加速度 carsim2019,matlab2018
- 电脑屏幕屏蔽监控,可以避免其他人监视电脑屏幕
- 01 戳泡泡 小游戏 源码可运行.zip
- 模拟量滤波程序 西门子200smart程序,能实现电流电压和热电阻模拟量信号的采集,有滤波,有高位和低位报警,采用for循环指令和间接寻址,让程序简单好用,并且针对程序,录制了视频讲解,详细的介绍了程
- 手势捕捉leapmotion driver驱动4.1.0 for windows
- 汇川H5U PLC程序框架 包含34轴程序样例 共3套,编程手册等 主伺服控制是ETHERCAT总线 程序写的条理分明,清晰易懂,注释清楚,对于初次使用汇川的总线控制有很好的参考价值 气缸的
- 01 大力射手微信小游戏源码可运行.zip
- 01 岛国么么哒小游戏源码.zip
- PLC ethercat总线伺服资料 信捷PLC EtherCat总线9轴凸轮伺服,包括PLC和触摸屏程序,伺服参数设置文件,信捷PLC XDH-60T4,函数功能块没有密码,Q232
- 01 疯狂打企鹅 小游戏 源码.zip
- 模块化多电平变器MMC在三相不平衡工况下的仿真模型,三种控制目标(抑制交流测负序电流、抑制有功功率二次脉动、抑制无功功率二次脉动)的分别通过pi 无源控制 滑模控制策略实现(交流7kV-直流20kV整
资源上传下载、课程学习等过程中有任何疑问或建议,欢迎提出宝贵意见哦~我们会及时处理!
点击此处反馈


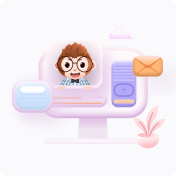
- 1
- 2
前往页