<br>
<div>
<a href="https://github.com/Qihoo360/XLearning">
<img width="400" heigth="400" src="./doc/img/logo.jpg">
</a>
</div>
[](./LICENSE)
[]()
[]()
**XLearning** is a convenient and efficient scheduling platform combined with the big data and artificial intelligence, support for a variety of machine learning, deep learning frameworks. XLearning is running on the Hadoop Yarn and has integrated deep learning frameworks such as TensorFlow, MXNet, Caffe, Theano, PyTorch, Keras, XGBoost. XLearning has the satisfactory scalability and compatibility.
[**中文文档**](./README_CN.md)
## Architecture

There are three essential components in XLearning:
- **Client**: start and get the state of the application.
- **ApplicationMaster(AM)**: the role for the internal schedule and lifecycle manager, including the input data distribution and containers management.
- **Container**: the actual executor of the application to start the progress of Worker or PS(Parameter Server), monitor and report the status of the progress to AM, and save the output, especially start the TensorBoard service for TensorFlow application.
## Functions
### 1 Support Multiple Deep Learning Frameworks
Besides the distributed mode of TensorFlow and MXNet frameworks, XLearning supports the standalone mode of all deep learning frameworks such as Caffe, Theano, PyTorch. Moreover, XLearning allows the custom versions and multi-version of frameworks flexibly.
### 2 Unified Data Management Based On HDFS
XLearning is enable to specify the input strategy for the input data `--input` by setting the `--input-strategy` parameter or `xlearning.input.strategy` configuration. XLearning support three ways to read the HDFS input data:
- **Download**: AM traverses all files under the specified HDFS path and distributes data to workers in files. Each worker download files from the remote to local.
- **Placeholder**: The difference with Download mode is that AM send the related HDFS file list to workers. The process in worker read the data from HDFS directly.
- **InputFormat**: Integrated the InputFormat function of MapReduce, XLearning allows the user to specify any of the implementation of InputFormat for the input data. AM splits the input data and assigns fragments to the different workers. Each worker passes the assigned fragments through the pipeline to the execution progress.
Similar with the read strategy, XLearning allows to specify the output strategy for the output data `--output` by setting the `--output-strategy` parameter or `xlearning.output.strategy` configuration. There are two kinds of result output modes:
- **Upload**: After the program finished, each worker upload the local directory of the output to specified HDFS path directly. The button, "Saved Model", on the web interface allows user to upload the intermediate result to remote during the execution.
- **OutputFormat**: Integrated the OutputFormat function of MapReduce, XLearning allows the user to specify any of the implementation of OutputFormat for saving the result to HDFS.
More detail see [**data management**](./doc/datamanage_cn.md)
### 3 Visualization Display
The application interface can be divided into four parts:
- **All Containers**:display the container list and corresponding information, including the container host, container role, current state of container, start time, finish time, current progress.
- **View TensorBoard**:If set to start the service of TensorBoard when the type of application is TensorFlow, provide the link to enter the TensorBoard for real-time view.
- **Save Model**:If the application has the output, user can upload the intermediate output to specified HDFS path during the execution of the application through the button of "Save Model". After the upload finished, display the list of the intermediate saved path.
- **Worker Metrix**:display the resource usage information metrics of each worker.
As shown below:

### 4 Compatible With The Code At Native Frameworks
Except the automatic construction of the ClusterSpec at the distributed mode TensorFlow framework, the program at standalone mode TensorFlow and other deep learning frameworks can be executed at XLearning directly.
## Compilation & Deployment Instructions
### 1 Compilation Environment Requirements
- jdk >= 1.7
- Maven >= 3.3
### 2 Compilation Method
Run the following command in the root directory of the source code:
`mvn package`
After compiling, a distribution package named `xlearning-1.1-dist.tar.gz` will be generated under `target` in the root directory.
Unpacking the distribution package, the following subdirectories will be generated under the root directory:
- bin: scripts for application commit
- lib: jars for XLearning and dependencies
- conf: configuration files
- sbin: scripts for history service
- data: data and files for examples
- examples: XLearning examples
### 3 Deployment Environment Requirements
- CentOS 7.2
- Java >= 1.7
- Hadoop = 2.6, 2.7, 2.8
- [optional] Dependent environment for deep learning frameworks at the cluster nodes, such as TensorFlow, numpy, Caffe.
### 4 XLearning Client Deployment Guide
Under the "conf" directory of the unpacking distribution package "$XLEARNING_HOME", configure the related files:
- xlearning-env.sh: set the environment variables, such as:
+ JAVA\_HOME
+ HADOOP\_CONF\_DIR
- xlearning-site.xml: configure related properties. Note that the properties associated with the history service needs to be consistent with what has configured when the history service started.For more details, please see the [**Configuration**](./doc/configure.md) part。
- log4j.properties:configure the log level
### 5 Start Method of XLearning History Service [Optional]
- run `$XLEARNING_HOME/sbin/start-history-server.sh`.
## Quick Start
Use `$XLEARNING_HOME/bin/xl-submit` to submit the application to cluster in the XLearning client.
Here are the submit example for the TensorFlow application.
### 1 upload data to hdfs
upload the "data" directory under the root of unpacking distribution package to HDFS
cd $XLEARNING_HOME
hadoop fs -put data /tmp/
### 2 submit
cd $XLEARNING_HOME/examples/tensorflow
$XLEARNING_HOME/bin/xl-submit \
--app-type "tensorflow" \
--app-name "tf-demo" \
--input /tmp/data/tensorflow#data \
--output /tmp/tensorflow_model#model \
--files demo.py,dataDeal.py \
--launch-cmd "python demo.py --data_path=./data --save_path=./model --log_dir=./eventLog --training_epochs=10" \
--worker-memory 10G \
--worker-num 2 \
--worker-cores 3 \
--ps-memory 1G \
--ps-num 1 \
--ps-cores 2 \
--queue default \
The meaning of the parameters are as follows:
Property Name | Meaning
---------------- | ---------------
app-name | application name as "tf-demo"
app-type | application type as "tensorflow"
input | input file, HDFS path is "/tmp/data/tensorflow" related to local dir "./data"
output | output file,HDFS path is "/tmp/tensorflow_model" related to local dir "./model"
files | application program and required local files, including demo.py, dataDeal.py
launch-cmd | execute command
worker-memory | amount of memory to use for the worker process is 10GB
worker-num | number of worker containers to use for the application is 2
worker-cores | number of cores to use for the worker process is 3
ps-memory | amount of memory to use for the ps process is 1GB
ps-num | number of ps containers to use for the application is 1
ps-co
没有合适的资源?快使用搜索试试~ 我知道了~
温馨提示
机器学习 (1)模拟人脑的机器学习 符号学习:模拟人脑的宏现心理级学习过程,以认知心理学原理为基础,以符号数据为输入,以符号运算为方法,用推理过程在图或状态空间中搜索,学习的目标为概念或规则等。符号学习的典型方法有记忆学习、示例学习、演绎学习.类比学习、解释学习等。 神经网络学习(或连接学习):模拟人脑的微观生理级学习过程,以脑和神经科学原理为基础,以人工神经网络为函数结构模型,以数值数据为输入,以数值运算为方法,用迭代过程在系数向量空间中搜索,学习的目标为函数。典型的连接学习有权值修正学习、拓扑结构学习。 (2)直接采用数学方法的机器学习 主要有统计机器学习。 [2] 统计机器学习是基于对数据的初步认识以及学习目的的分析,选择合适的数学模型,拟定超参数,并输入样本数据,依据一定的策略,运用合适的学习算法对模型进行训练,最后运用训练好的模型对数据进行分析预测。 统计机器学习三个要素: 模型(model):模型在未进行训练前,其可能的参数是多个甚至无穷的,故可能的模型也是多个甚至无穷的,这些模型构成的集合就是假设空间。 策略(strategy):即从假设空间中挑选出参数最优的模型的准则。
资源推荐
资源详情
资源评论
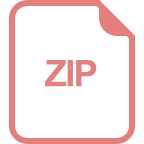
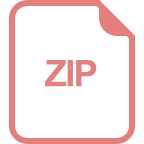
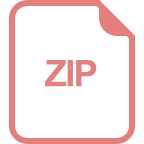
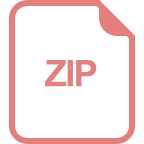
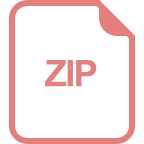
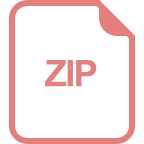
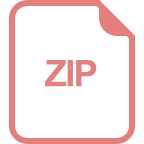
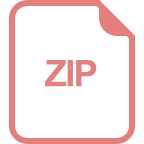
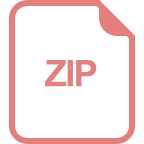
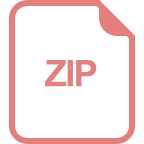
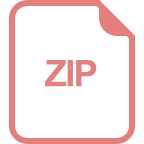
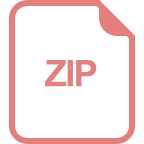
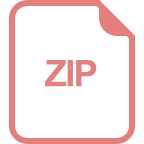
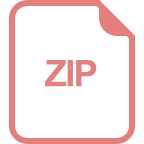
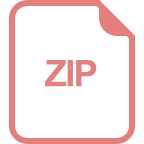
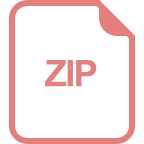
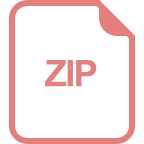
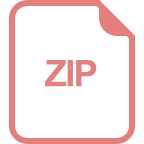
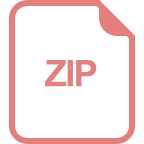
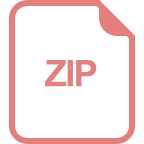
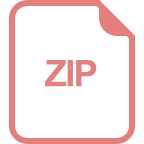
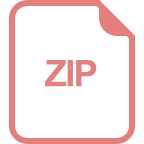
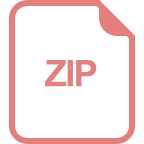
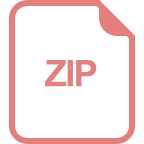
收起资源包目录

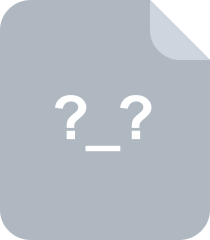
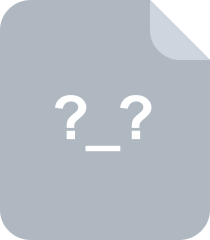
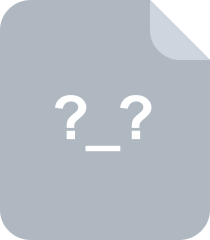
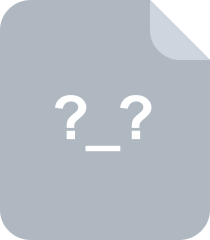
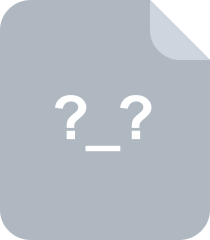
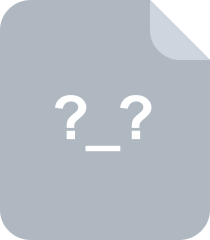
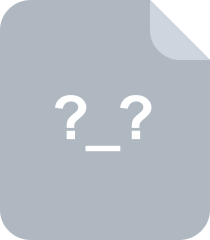
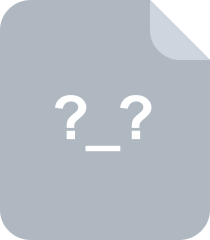
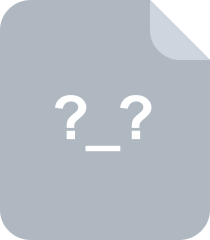
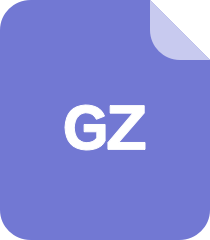
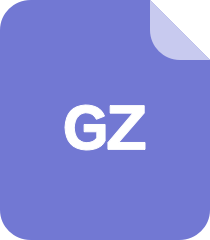
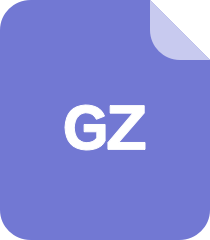
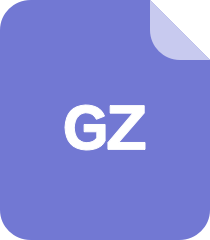
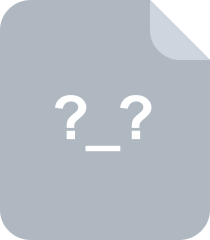
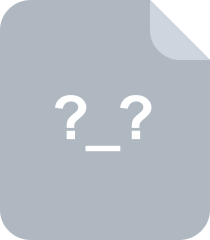
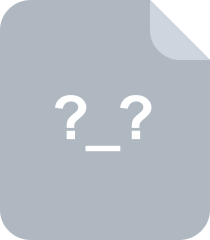
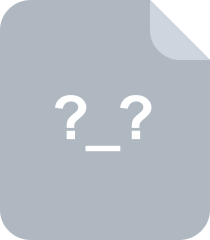
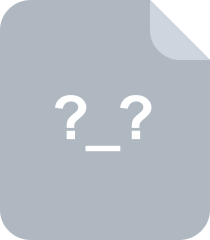
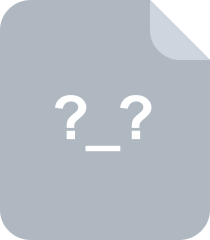
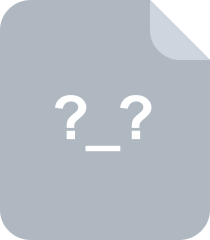
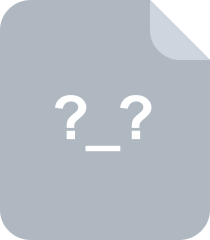
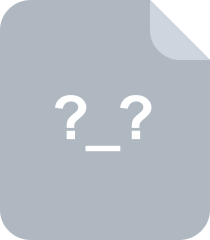
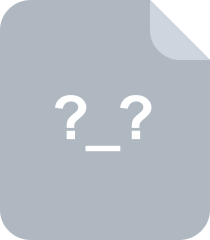
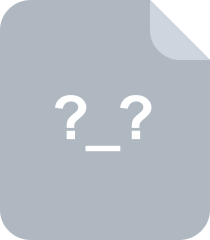
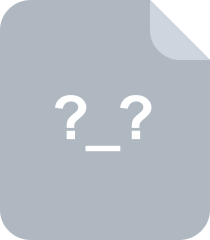
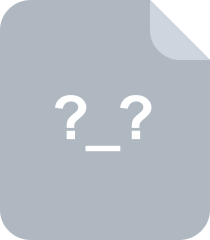
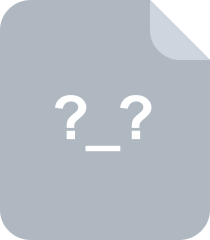
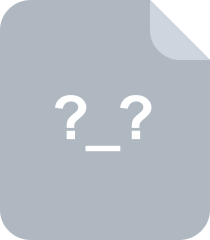
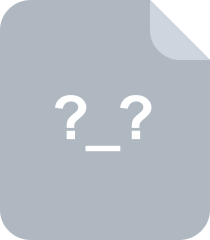
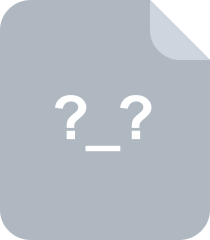
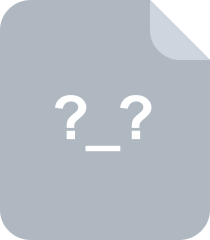
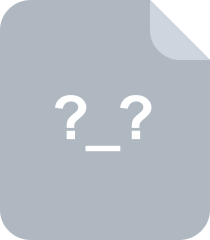
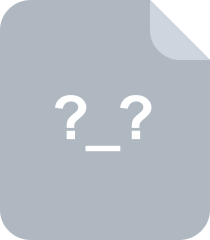
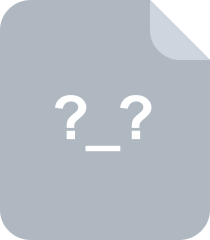
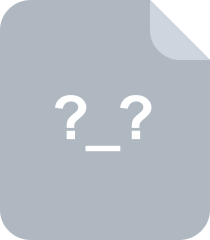
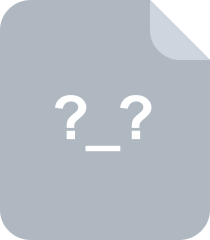
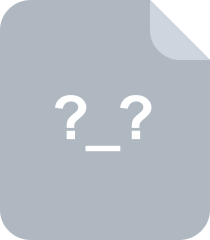
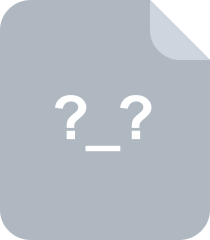
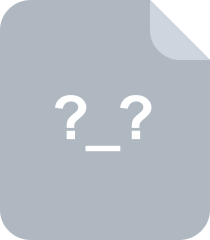
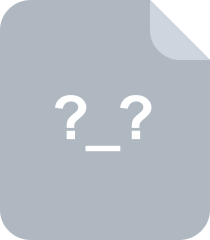
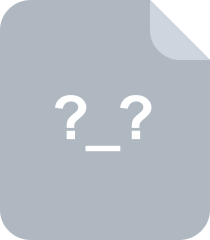
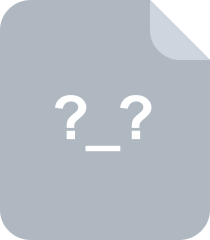
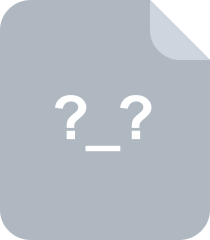
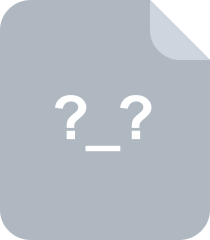
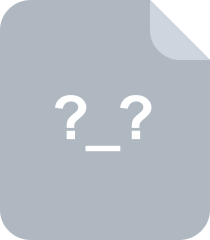
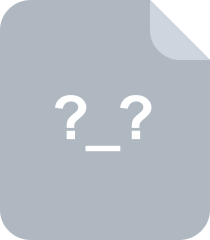
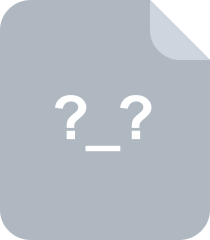
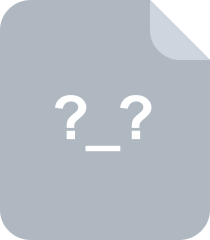
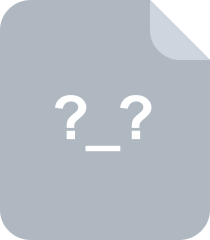
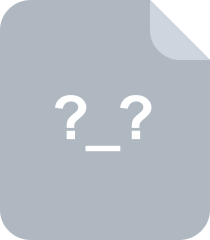
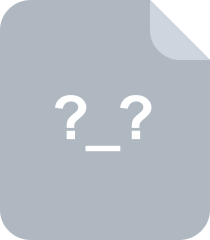
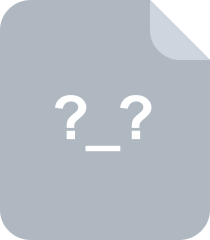
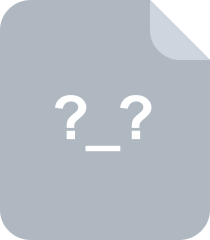
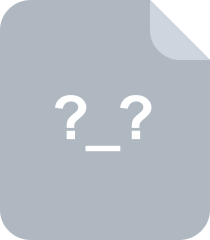
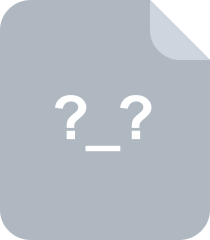
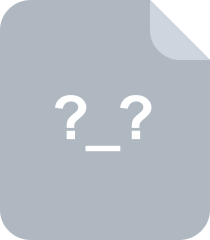
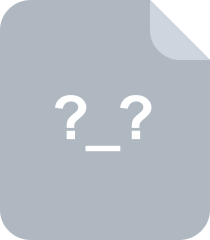
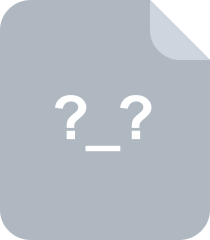
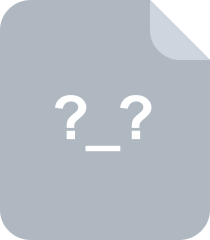
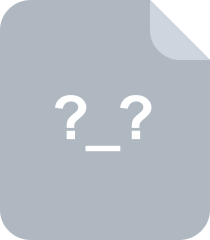
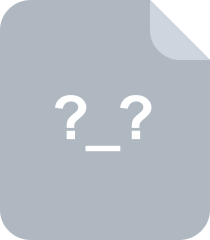
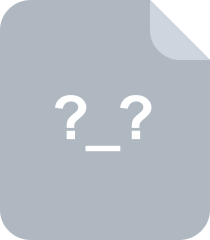
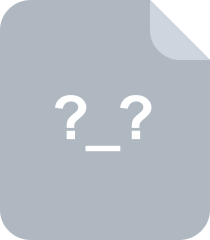
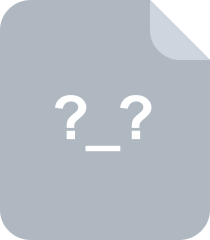
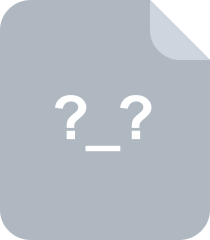
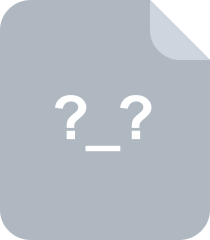
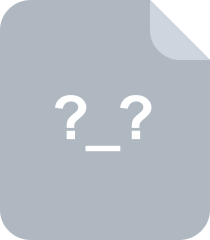
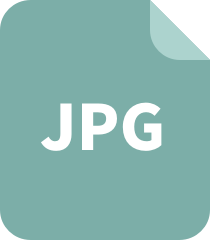
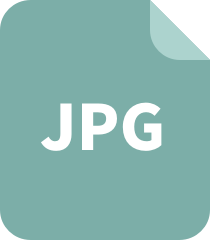
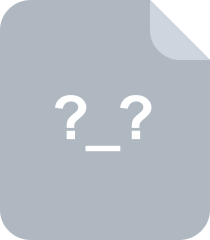
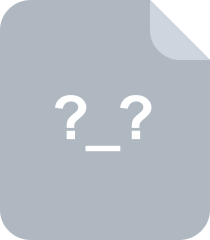
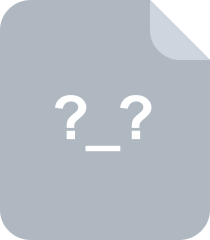
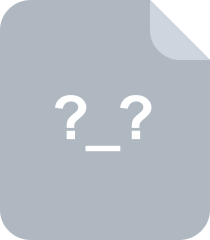
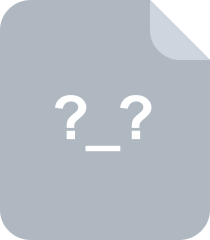
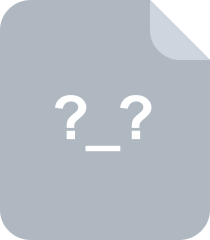
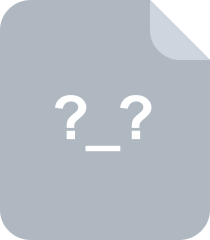
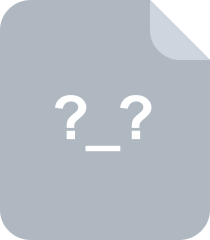
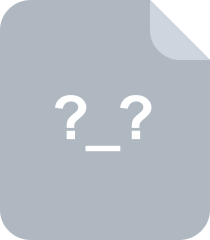
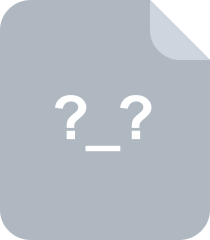
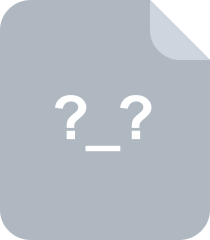
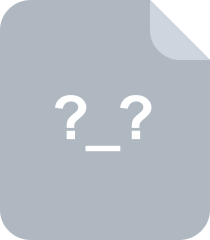
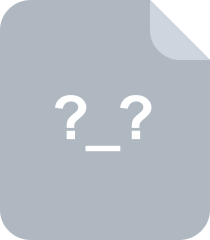
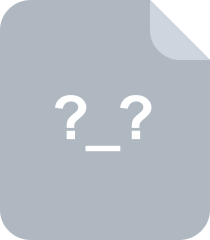
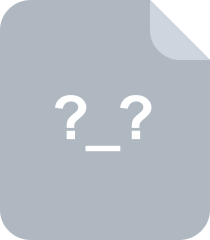
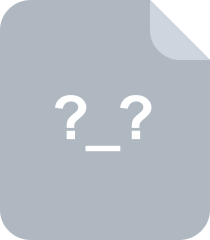
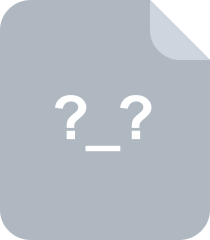
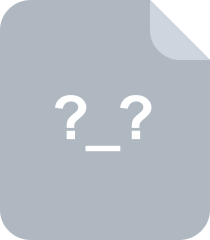
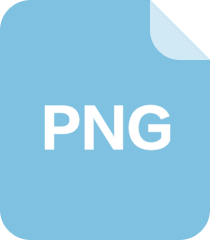
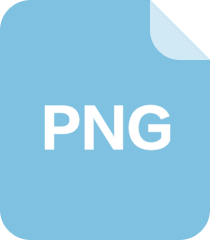
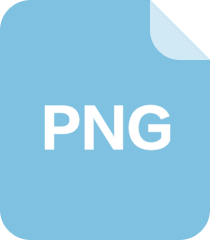
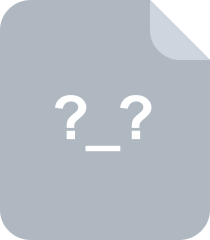
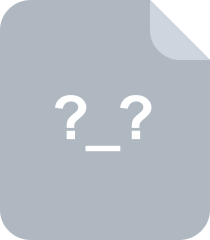
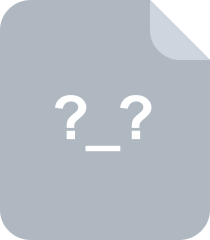
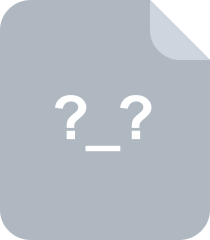
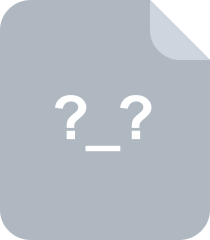
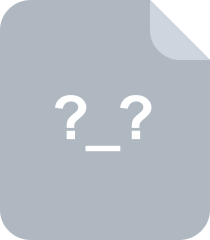
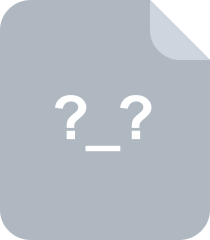
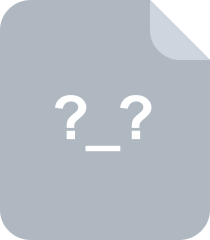
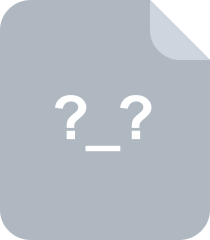
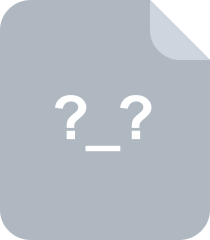
共 136 条
- 1
- 2
资源评论
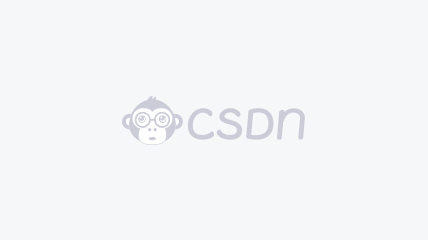

野生的狒狒
- 粉丝: 3394
- 资源: 2436

下载权益

C知道特权

VIP文章

课程特权

开通VIP
上传资源 快速赚钱
我的内容管理 展开
我的资源 快来上传第一个资源
我的收益
登录查看自己的收益我的积分 登录查看自己的积分
我的C币 登录后查看C币余额
我的收藏
我的下载
下载帮助

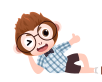
最新资源
- HengCe-18900-2024-2030全球与中国二手半导体设备市场现状及未来发展趋势-样本.docx
- Infinite Scroll View 2.0
- 杂物检测63-YOLO(v5至v8)、COCO、CreateML、VOC数据集合集.rar
- HengCe-18900-2024-2030全球与中国氢燃料电池气体扩散层市场现状及未来发展趋势-样本.docx
- 机器狗的发展历史,介绍
- 木材钢管检测17-YOLO(v5至v9)、COCO、CreateML、Darknet、Paligemma、TFRecord、VOC数据集合集.rar
- 回文判断(C语言)(不必理会)
- 无人机检测24-YOLO(v5至v9)、COCO、CreateML、Darknet、Paligemma、TFRecord、VOC数据集合集.rar
- mybatisplus自定义xml文件
- 严蔚敏《数据结构》(C语言版):核心知识点梳理与考研真题详解(自用)(不必理会)
资源上传下载、课程学习等过程中有任何疑问或建议,欢迎提出宝贵意见哦~我们会及时处理!
点击此处反馈


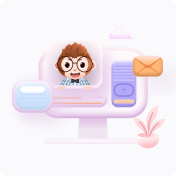
安全验证
文档复制为VIP权益,开通VIP直接复制
