没有合适的资源?快使用搜索试试~ 我知道了~
资源推荐
资源详情
资源评论
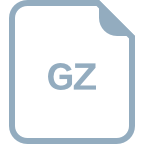
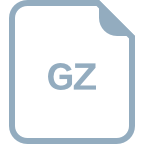
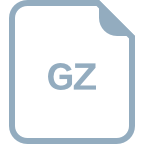
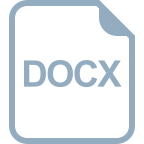
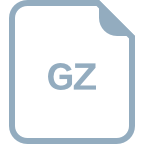
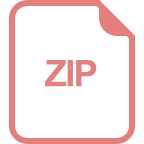
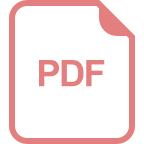
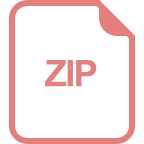
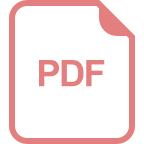
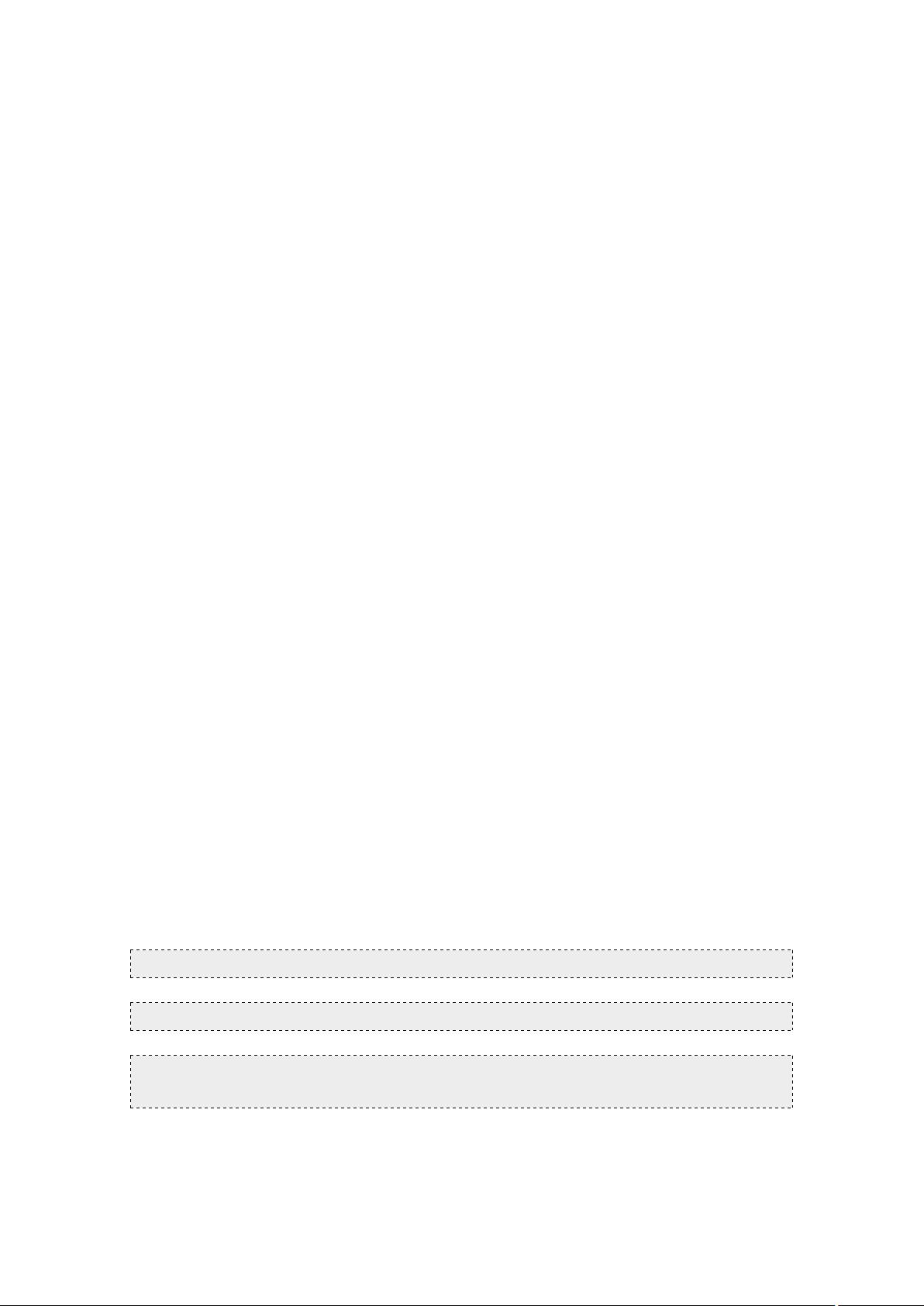
1
Airflow:Airflow 与 Python 集成开发
1 Airflow 简介与安装
1.1 Airflow 的核心概念
Airflow 是一个用于编排工作流的平台,它允许用户定义、监控和执行复杂
的数据管道。在 Airflow 中,工作流被定义为 DAGs(Directed Acyclic Graphs,有
向无环图),每个 DAG 代表一个工作流,由一系列的任务(Tasks)组成,这些
任务之间通过依赖关系(Dependencies)连接,形成一个有向无环图。
1.1.1 DAG
DAG 是 Airflow 的核心,它定义了任务的执行顺序。每个 DAG 由一个或多
个任务组成,这些任务可以是 Python 函数的执行、数据的导入、数据的处理等。
1.1.2 Task
Task 是 DAG 中的一个节点,代表一个具体的执行单元。在 Airflow 中,
Task 可以是 Python 函数、SQL 查询、Shell 命令等。
1.1.3 Operator
Operator 是 Airflow 中用于创建 Task 的类,它封装了特定类型的 Task,如
PythonOperator 用于执行 Python 函数,BashOperator 用于执行 Shell 命令。
1.1.4 Executor
Executor 负责调度和执行 Task。Airflow 支持多种 Executor,如
LocalExecutor、CeleryExecutor 等,可以根据实际需求选择合适的 Executor。
1.2 Airflow 的安装与配置
Airflow 的安装可以通过 pip 进行,首先确保 Python 和 pip 已经安装在你的
系统上,然后在命令行中运行以下命令:
pip install apache-airflow
安装完成后,需要初始化 Airflow 的数据库:
airflow db init
接下来,启动 Airflow 的 Web 服务器和 Scheduler:
airflow webserver -D
airflow scheduler -D
配置 Airflow 主要通过修改 airflow.cfg 文件,这个文件位于 Airflow 的安装
目录下。你可以在这里设置 Airflow 的运行参数,如 DAGs 的存储位置、日志的
存储位置、使用的 Executor 等。
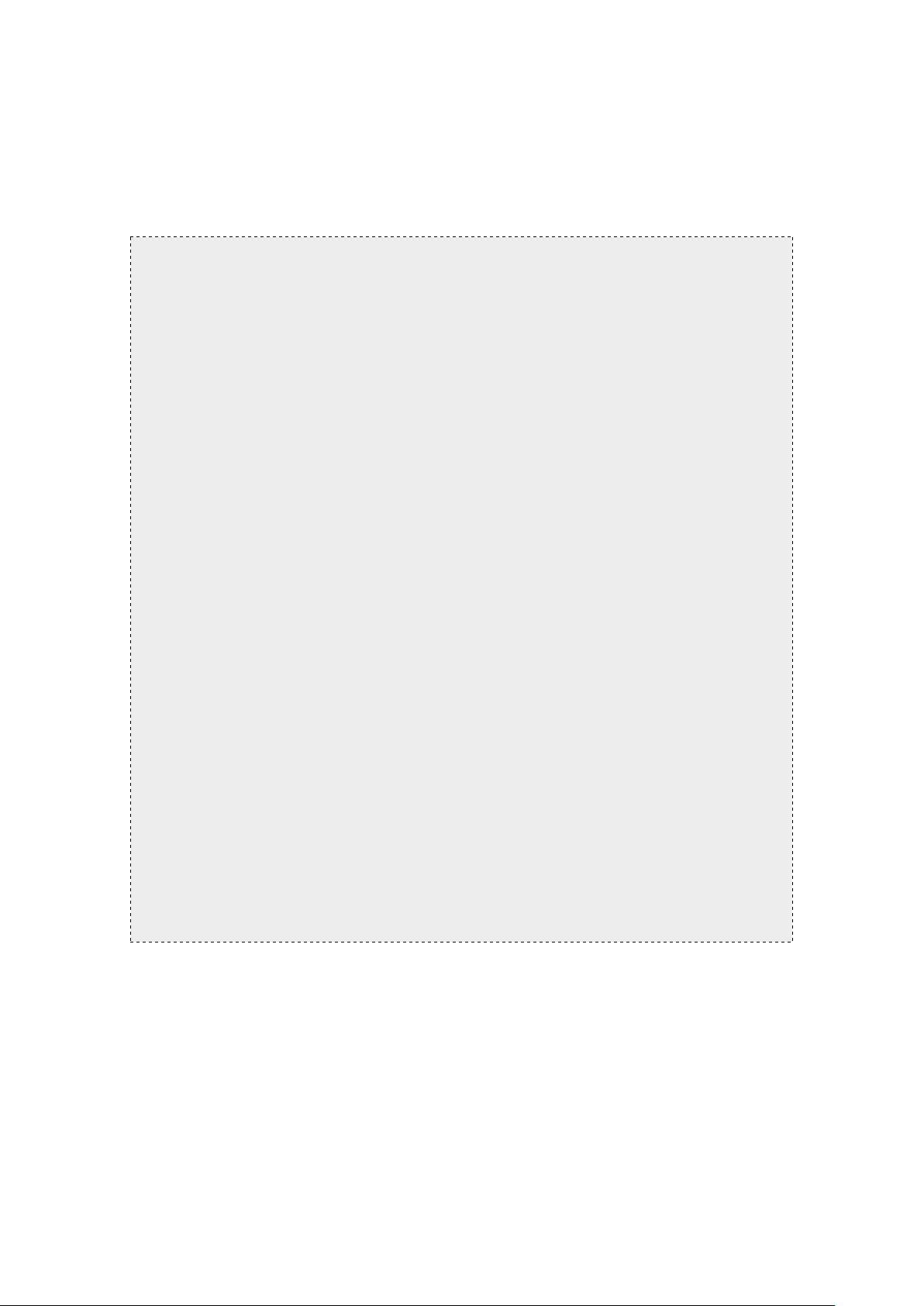
2
1.3 第一个 DAG:HelloWorld 示例
下面是一个简单的 DAG 示例,它定义了一个只有一个任务的 DAG,这个任
务就是打印“Hello World”。
import datetime as dt
import airflow
from airflow import DAG
from airflow.operators.python_operator import PythonOperator
#
定义
DAG
的默认参数
default_args = {
'owner': 'airflow',
'start_date': airflow.utils.dates.days_ago(2),
}
#
定义
DAG
dag = DAG(
dag_id='hello_world',
default_args=default_args,
schedule_interval='@daily',
catchup=False,
)
#
定义任务
def print_hello():
print('Hello World!')
#
创建
PythonOperator
hello_world = PythonOperator(
task_id='hello_world',
python_callable=print_hello,
dag=dag,
)
在这个示例中,我们首先导入了必要的模块,然后定义了 DAG 的默认参数,
包括 DAG 的所有者和开始日期。接着,我们定义了一个 DAG,指定了 DAG 的
ID、默认参数、调度间隔和是否回溯执行。最后,我们定义了一个任务,这个
任务就是打印“Hello World”,然后创建了一个 PythonOperator,指定了任务的
ID、要执行的 Python 函数和所属的 DAG。
这个 DAG 每天都会运行一次,每次运行都会执行 print_hello 函数,打印
“Hello World”。
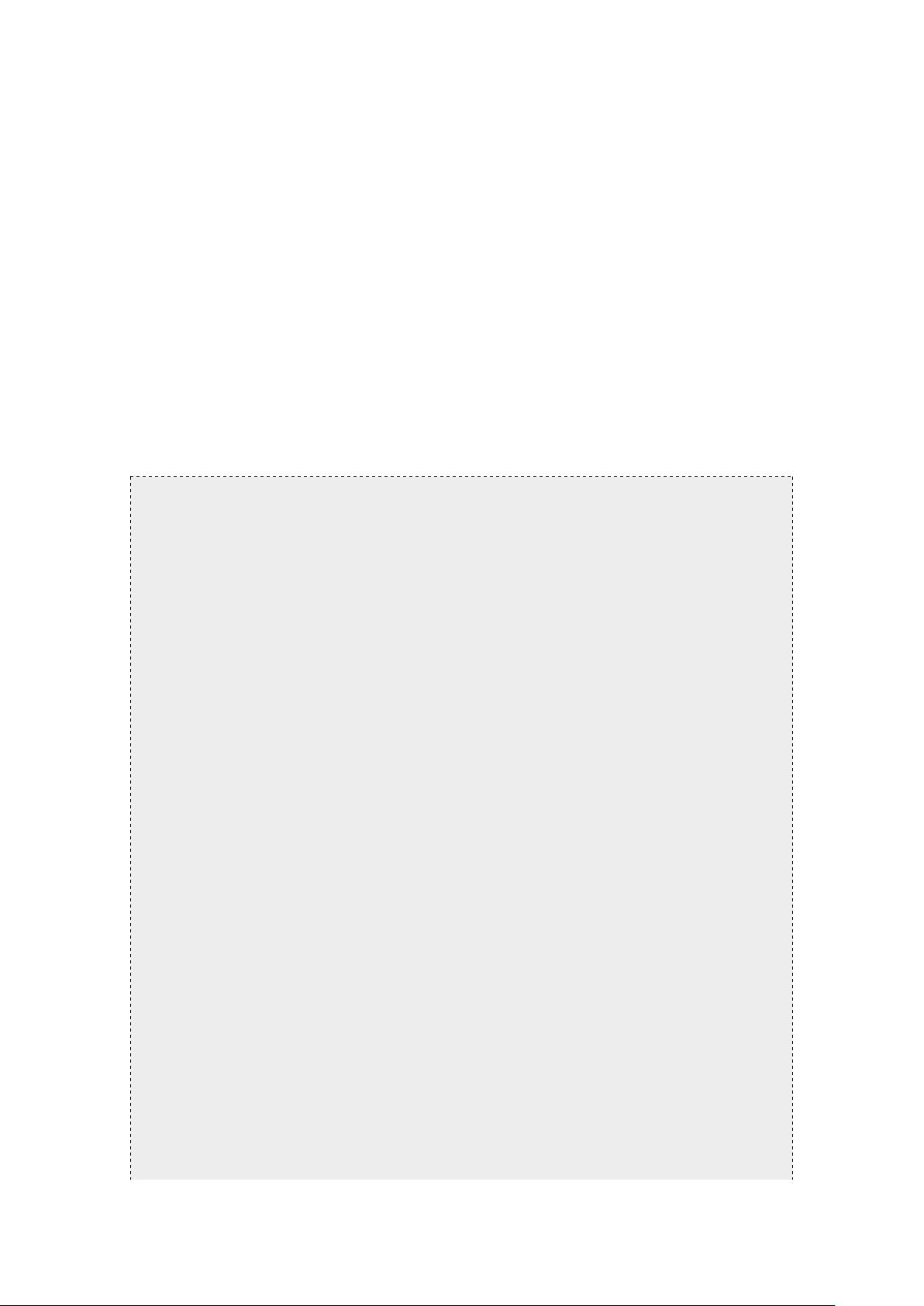
3
2 Airflow: PythonOperator 与 Python 集成开发
2.1 PythonOperator 的使用
在 Apache Airflow 中,PythonOperator 是一个强大的工具,允许你直接在
Airflow 的 DAG 中执行 Python 代码。这为数据工程师和数据科学家提供了一个
灵活的框架,可以利用 Python 的丰富库和功能来构建复杂的 ETL 流程和数据管
道。
2.1.1 创建 PythonOperator
要创建一个 PythonOperator,你需要定义一个 Python 函数,这个函数将在
任务执行时运行。然后,使用 PythonOperator 类创建一个任务,将你的 Python
函数作为参数传递。
from datetime import datetime, timedelta
from airflow import DAG
from airflow.operators.python_operator import PythonOperator
#
定义默认参数
default_args = {
'owner': 'airflow',
'depends_on_past': False,
'start_date': datetime(2023, 1, 1),
'email_on_failure': False,
'email_on_retry': False,
'retries': 1,
'retry_delay': timedelta(minutes=5),
}
#
初始化
DAG
dag = DAG(
'tutorial_dag',
default_args=default_args,
description='A simple tutorial DAG',
schedule_interval=timedelta(days=1),
)
#
定义
Python
函数
def print_hello():
"""
打印问候信息的函数
"""
print("Hello world!")
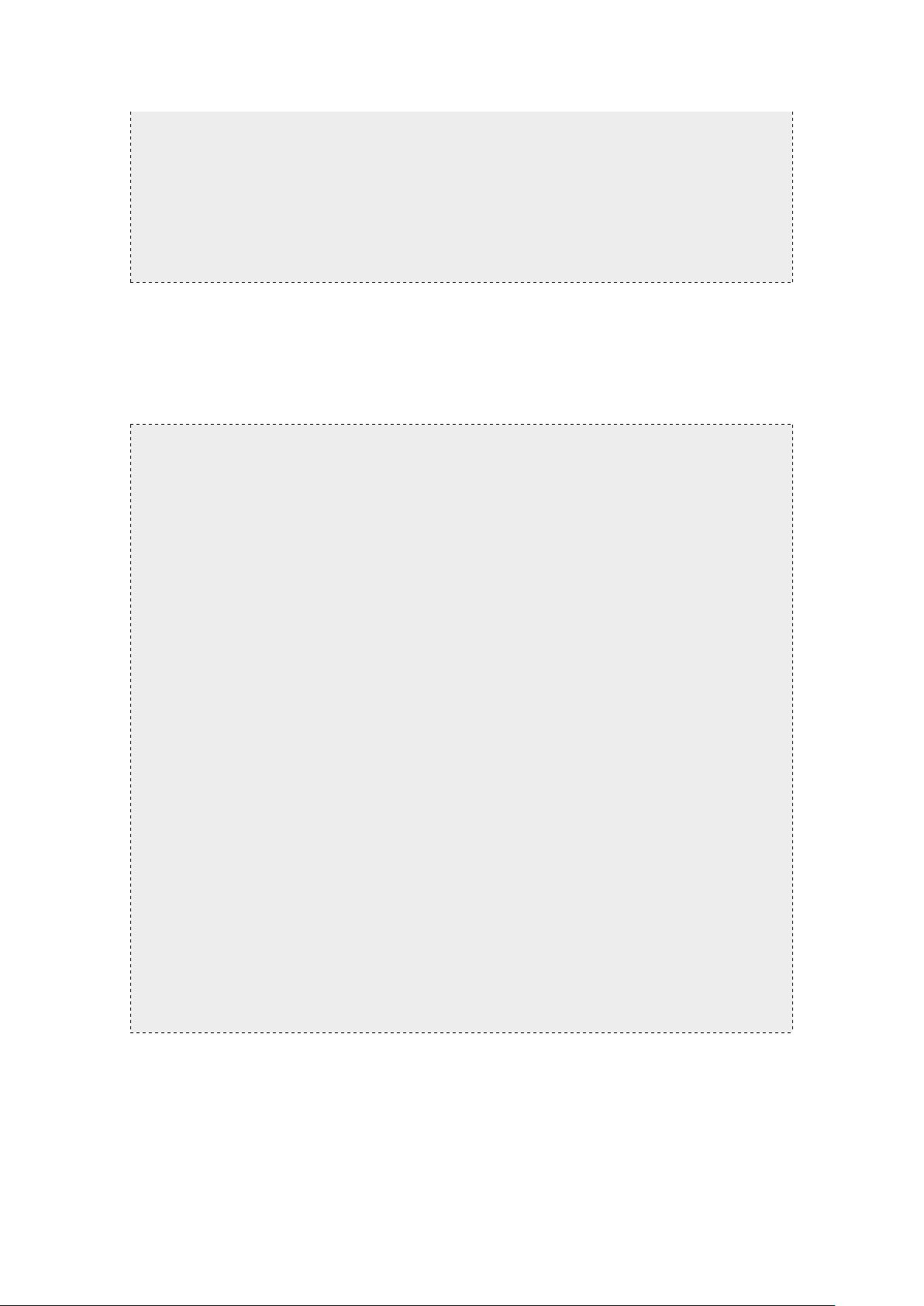
4
#
创建
PythonOperator
hello_task = PythonOperator(
task_id='hello_task',
python_callable=print_hello,
dag=dag,
)
2.1.2 PythonOperator 的灵活性
PythonOperator 的灵活性在于,你可以执行任何 Python 代码,从简单的打
印语句到复杂的机器学习模型训练。例如,下面的代码展示了如何使用
PythonOperator 来处理数据并将其写入数据库。
import pandas as pd
from sqlalchemy import create_engine
def process_data_and_store():
"""
读取数据,处理并存储到数据库的函数
"""
#
读取数据
data = pd.read_csv('/path/to/data.csv')
#
数据处理
processed_data = data[data['value'] > 0]
#
连接数据库
engine = create_engine('postgresql://user:password@host:port/dbname')
#
存储数据
processed_data.to_sql('processed_data', engine, if_exists='replace', index=False)
#
创建
PythonOperator
data_task = PythonOperator(
task_id='data_task',
python_callable=process_data_and_store,
dag=dag,
)
2.2 在 DAG 中执行 Python 代码
在 Airflow 中,你可以通过定义 DAG 并使用 PythonOperator 来执行 Python
代码。DAG(Directed Acyclic Graph)是 Airflow 的核心概念,它定义了任务的执
行顺序和依赖关系。
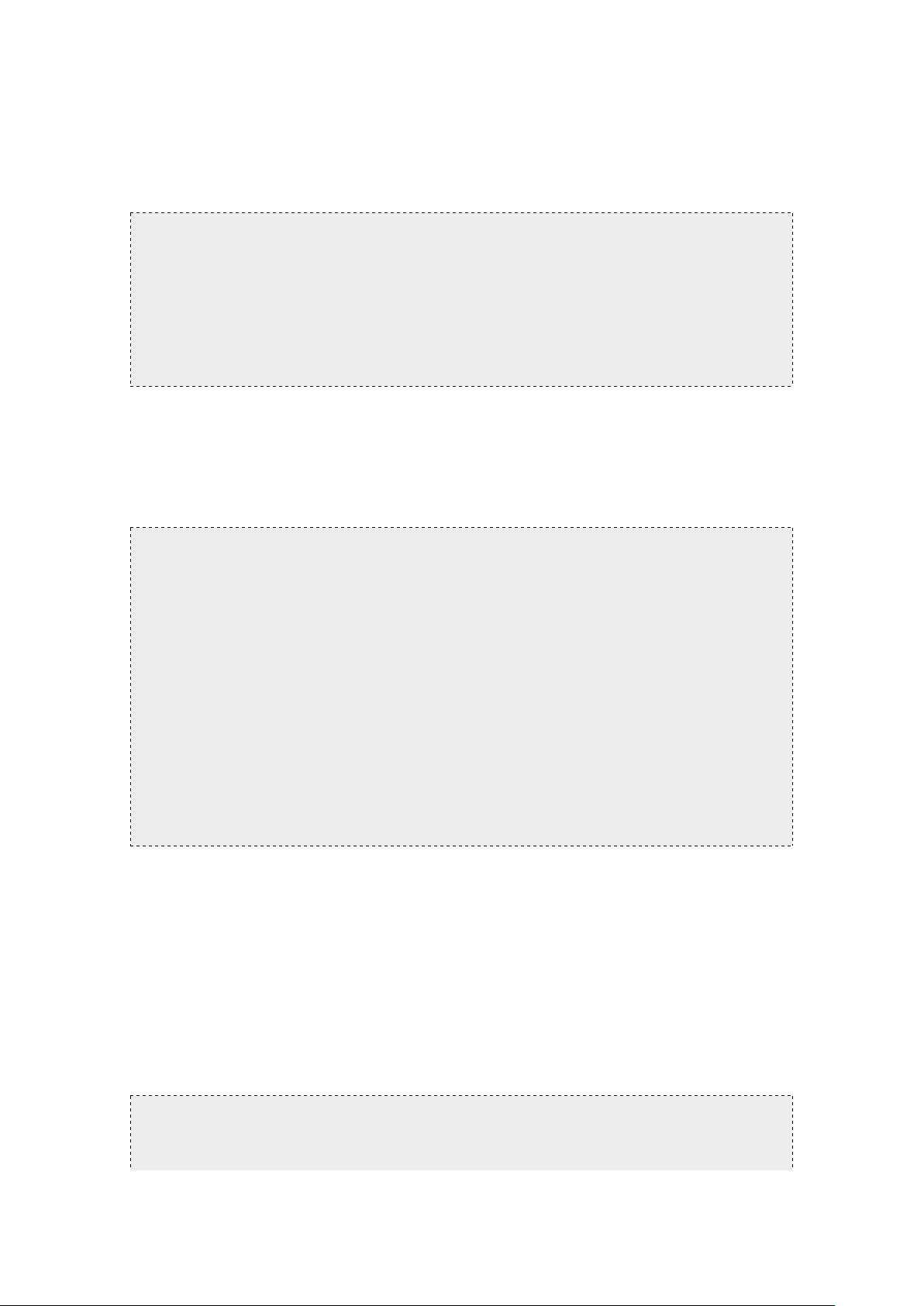
5
2.2.1 定义 DAG
DAG 的定义包括设置默认参数、描述、调度间隔等。一旦定义了 DAG,你
就可以在其中添加任务。
#
初始化
DAG
dag = DAG(
'data_pipeline',
default_args=default_args,
description='A data pipeline using PythonOperator',
schedule_interval=timedelta(hours=1),
)
2.2.2 执行 Python 代码
在 DAG 中,你可以通过 PythonOperator 来执行 Python 代码。例如,下面
的代码展示了如何在 DAG 中定义一个任务,该任务将执行一个 Python 函数来
处理数据。
def process_data():
"""
处理数据的函数
"""
#
你的数据处理代码
pass
#
创建
PythonOperator
process_data_task = PythonOperator(
task_id='process_data_task',
python_callable=process_data,
dag=dag,
)
2.3 参数传递与环境变量
在 Airflow 中,你可以在 PythonOperator 中传递参数,这些参数可以在你的
Python 函数中使用。此外,你还可以设置环境变量,这对于需要特定环境配置
的 Python 代码特别有用。
2.3.1 传递参数
你可以通过 op_kwargs 参数来传递关键字参数给 PythonOperator。
def process_data_with_params(param1, param2):
"""
使用参数处理数据的函数
剩余21页未读,继续阅读
资源评论
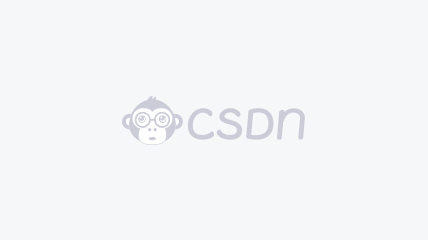


zhubeibei168
- 粉丝: 7508
- 资源: 452
上传资源 快速赚钱
我的内容管理 展开
我的资源 快来上传第一个资源
我的收益
登录查看自己的收益我的积分 登录查看自己的积分
我的C币 登录后查看C币余额
我的收藏
我的下载
下载帮助

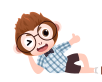
最新资源
- 基于javaweb的网上拍卖系统,采用Spring + SpringMvc+Mysql + Hibernate+ JSP技术
- polygon-mumbai
- Chrome代理 switchyOmega
- GVC-全球价值链参与地位指数,基于ICIO表,(Wang等 2017a)计算方法
- 易语言ADS指纹浏览器管理工具
- 易语言奇易模块5.3.6
- cad定制家具平面图工具-(FG)门板覆盖柜体
- asp.net 原生js代码及HTML实现多文件分片上传功能(自定义上传文件大小、文件上传类型)
- whl@pip install pyaudio ERROR: Failed building wheel for pyaudio
- Constantsfd密钥和权限集合.kt
资源上传下载、课程学习等过程中有任何疑问或建议,欢迎提出宝贵意见哦~我们会及时处理!
点击此处反馈


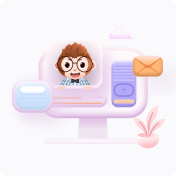
安全验证
文档复制为VIP权益,开通VIP直接复制
