# Multi-Object Tracking with Ultralytics YOLO
<img width="1024" src="https://user-images.githubusercontent.com/26833433/243418637-1d6250fd-1515-4c10-a844-a32818ae6d46.png" alt="YOLOv8 trackers visualization">
Object tracking in the realm of video analytics is a critical task that not only identifies the location and class of objects within the frame but also maintains a unique ID for each detected object as the video progresses. The applications are limitless—ranging from surveillance and security to real-time sports analytics.
## Why Choose Ultralytics YOLO for Object Tracking?
The output from Ultralytics trackers is consistent with standard object detection but has the added value of object IDs. This makes it easy to track objects in video streams and perform subsequent analytics. Here's why you should consider using Ultralytics YOLO for your object tracking needs:
- **Efficiency:** Process video streams in real-time without compromising accuracy.
- **Flexibility:** Supports multiple tracking algorithms and configurations.
- **Ease of Use:** Simple Python API and CLI options for quick integration and deployment.
- **Customizability:** Easy to use with custom trained YOLO models, allowing integration into domain-specific applications.
**Video Tutorial:** [Object Detection and Tracking with Ultralytics YOLOv8](https://www.youtube.com/embed/hHyHmOtmEgs?si=VNZtXmm45Nb9s-N-).
## Features at a Glance
Ultralytics YOLO extends its object detection features to provide robust and versatile object tracking:
- **Real-Time Tracking:** Seamlessly track objects in high-frame-rate videos.
- **Multiple Tracker Support:** Choose from a variety of established tracking algorithms.
- **Customizable Tracker Configurations:** Tailor the tracking algorithm to meet specific requirements by adjusting various parameters.
## Available Trackers
Ultralytics YOLO supports the following tracking algorithms. They can be enabled by passing the relevant YAML configuration file such as `tracker=tracker_type.yaml`:
- [BoT-SORT](https://github.com/NirAharon/BoT-SORT) - Use `botsort.yaml` to enable this tracker.
- [ByteTrack](https://github.com/ifzhang/ByteTrack) - Use `bytetrack.yaml` to enable this tracker.
The default tracker is BoT-SORT.
## Tracking
To run the tracker on video streams, use a trained Detect, Segment or Pose model such as YOLOv8n, YOLOv8n-seg and YOLOv8n-pose.
#### Python
```python
from ultralytics import YOLO
# Load an official or custom model
model = YOLO("yolov8n.pt") # Load an official Detect model
model = YOLO("yolov8n-seg.pt") # Load an official Segment model
model = YOLO("yolov8n-pose.pt") # Load an official Pose model
model = YOLO("path/to/best.pt") # Load a custom trained model
# Perform tracking with the model
results = model.track(
source="https://youtu.be/LNwODJXcvt4", show=True
) # Tracking with default tracker
results = model.track(
source="https://youtu.be/LNwODJXcvt4", show=True, tracker="bytetrack.yaml"
) # Tracking with ByteTrack tracker
```
#### CLI
```bash
# Perform tracking with various models using the command line interface
yolo track model=yolov8n.pt source="https://youtu.be/LNwODJXcvt4" # Official Detect model
yolo track model=yolov8n-seg.pt source="https://youtu.be/LNwODJXcvt4" # Official Segment model
yolo track model=yolov8n-pose.pt source="https://youtu.be/LNwODJXcvt4" # Official Pose model
yolo track model=path/to/best.pt source="https://youtu.be/LNwODJXcvt4" # Custom trained model
# Track using ByteTrack tracker
yolo track model=path/to/best.pt tracker="bytetrack.yaml"
```
As can be seen in the above usage, tracking is available for all Detect, Segment and Pose models run on videos or streaming sources.
## Configuration
### Tracking Arguments
Tracking configuration shares properties with Predict mode, such as `conf`, `iou`, and `show`. For further configurations, refer to the [Predict](https://docs.ultralytics.com/modes/predict/) model page.
#### Python
```python
from ultralytics import YOLO
# Configure the tracking parameters and run the tracker
model = YOLO("yolov8n.pt")
results = model.track(
source="https://youtu.be/LNwODJXcvt4", conf=0.3, iou=0.5, show=True
)
```
#### CLI
```bash
# Configure tracking parameters and run the tracker using the command line interface
yolo track model=yolov8n.pt source="https://youtu.be/LNwODJXcvt4" conf=0.3, iou=0.5 show
```
### Tracker Selection
Ultralytics also allows you to use a modified tracker configuration file. To do this, simply make a copy of a tracker config file (for example, `custom_tracker.yaml`) from [ultralytics/cfg/trackers](https://github.com/ultralytics/ultralytics/tree/main/ultralytics/cfg/trackers) and modify any configurations (except the `tracker_type`) as per your needs.
#### Python
```python
from ultralytics import YOLO
# Load the model and run the tracker with a custom configuration file
model = YOLO("yolov8n.pt")
results = model.track(
source="https://youtu.be/LNwODJXcvt4", tracker="custom_tracker.yaml"
)
```
#### CLI
```bash
# Load the model and run the tracker with a custom configuration file using the command line interface
yolo track model=yolov8n.pt source="https://youtu.be/LNwODJXcvt4" tracker='custom_tracker.yaml'
```
For a comprehensive list of tracking arguments, refer to the [ultralytics/cfg/trackers](https://github.com/ultralytics/ultralytics/tree/main/ultralytics/cfg/trackers) page.
## Python Examples
### Persisting Tracks Loop
Here is a Python script using OpenCV (`cv2`) and YOLOv8 to run object tracking on video frames. This script still assumes you have already installed the necessary packages (`opencv-python` and `ultralytics`). The `persist=True` argument tells the tracker than the current image or frame is the next in a sequence and to expect tracks from the previous image in the current image.
#### Python
```python
import cv2
from ultralytics import YOLO
# Load the YOLOv8 model
model = YOLO("yolov8n.pt")
# Open the video file
video_path = "path/to/video.mp4"
cap = cv2.VideoCapture(video_path)
# Loop through the video frames
while cap.isOpened():
# Read a frame from the video
success, frame = cap.read()
if success:
# Run YOLOv8 tracking on the frame, persisting tracks between frames
results = model.track(frame, persist=True)
# Visualize the results on the frame
annotated_frame = results[0].plot()
# Display the annotated frame
cv2.imshow("YOLOv8 Tracking", annotated_frame)
# Break the loop if 'q' is pressed
if cv2.waitKey(1) & 0xFF == ord("q"):
break
else:
# Break the loop if the end of the video is reached
break
# Release the video capture object and close the display window
cap.release()
cv2.destroyAllWindows()
```
Please note the change from `model(frame)` to `model.track(frame)`, which enables object tracking instead of simple detection. This modified script will run the tracker on each frame of the video, visualize the results, and display them in a window. The loop can be exited by pressing 'q'.
### Plotting Tracks Over Time
Visualizing object tracks over consecutive frames can provide valuable insights into the movement patterns and behavior of detected objects within a video. With Ultralytics YOLOv8, plotting these tracks is a seamless and efficient process.
In the following example, we demonstrate how to utilize YOLOv8's tracking capabilities to plot the movement of detected objects across multiple video frames. This script involves opening a video file, reading it frame by frame, and utilizing the YOLO model to identify and track various objects. By retaining the center points of the detected bounding boxes and connecting them, we can draw lines that represent the paths followed by the tracked objects.
#### Python
```python
from collections import defaultdict
import cv2
import numpy as np
from ultralytics import YOLO
# Load the YOLOv8 model
model = YOLO("y
没有合适的资源?快使用搜索试试~ 我知道了~
YOLOv8桥梁道路裂缝检测+训练好的模型+标注好的数据集+QT界面
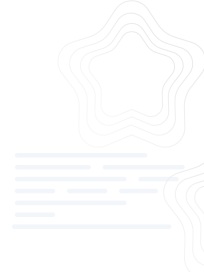
共2000个文件
txt:1610个
py:171个
jpg:139个

1.该资源内容由用户上传,如若侵权请联系客服进行举报
2.虚拟产品一经售出概不退款(资源遇到问题,请及时私信上传者)
2.虚拟产品一经售出概不退款(资源遇到问题,请及时私信上传者)
版权申诉
1 下载量 158 浏览量
2024-04-21
17:22:31
上传
评论
收藏 374.31MB ZIP 举报
温馨提示
1、YOLOv8训练好的道路裂缝检测模型,并包含1000多张标注好的数据集,标签格式为xml和txt两种,配置好YOLOv5环境后可以直接使用,含QT界面 2、数据集和检测结果参考:https://blog.csdn.net/zhiqingAI/article/details/124230743 3、采用pytrch框架,代码是python的
资源推荐
资源详情
资源评论
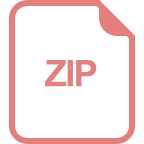
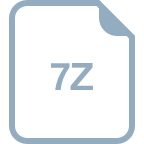
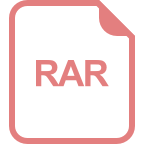
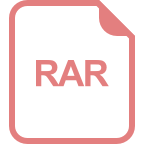
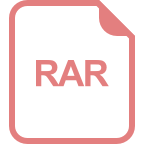
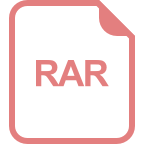
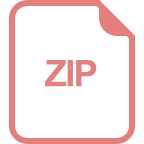
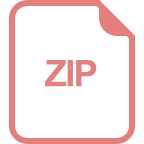
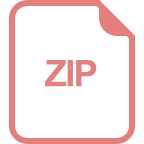
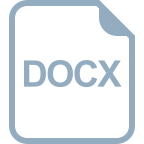
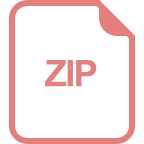
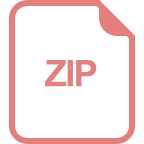
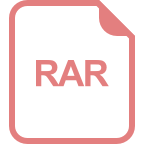
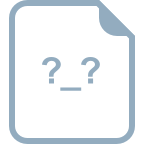
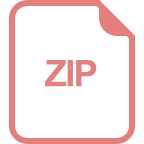
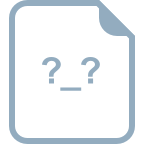
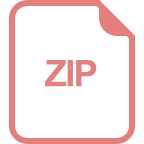
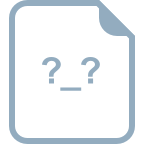
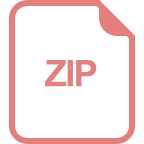
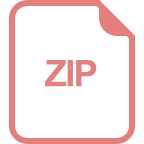
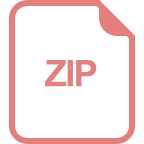
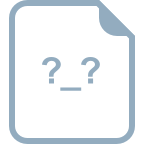
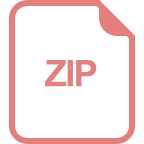
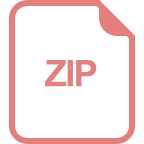
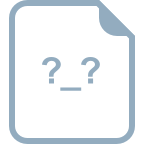
收起资源包目录

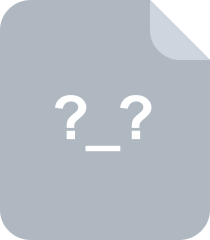
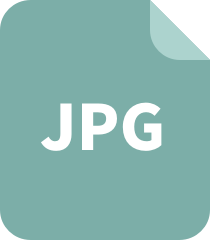
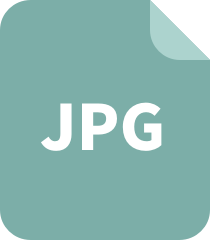
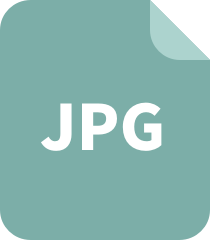
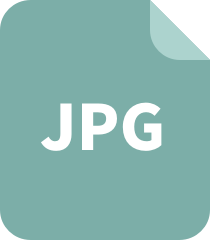
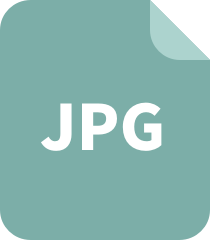
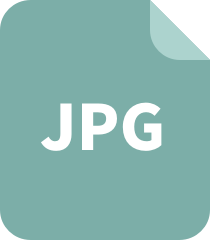
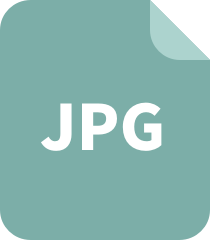
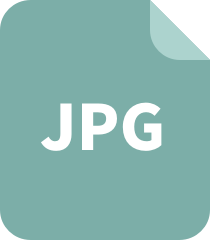
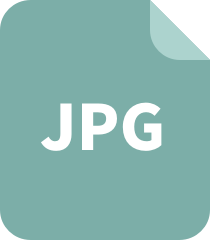
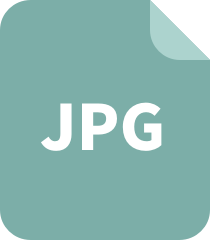
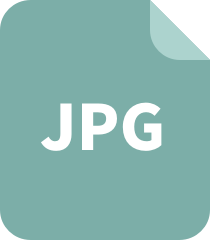
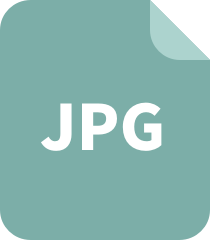
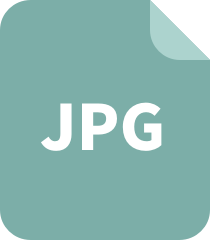
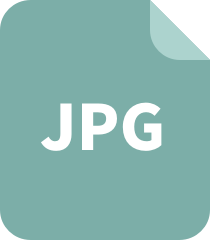
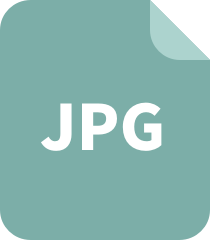
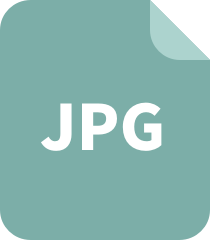
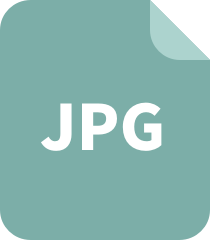
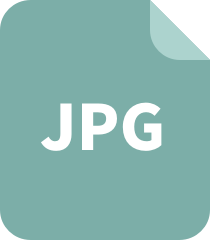
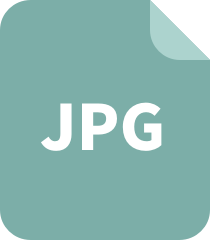
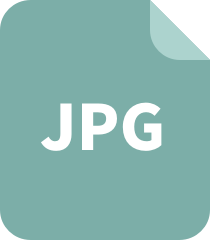
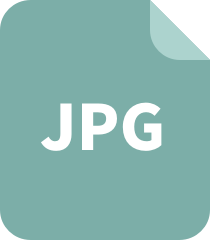
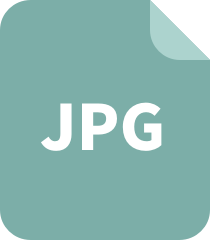
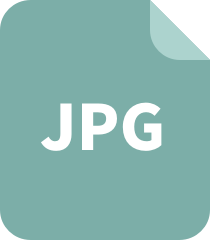
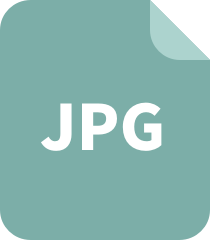
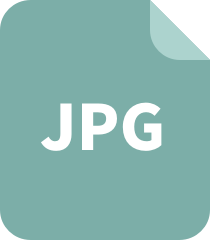
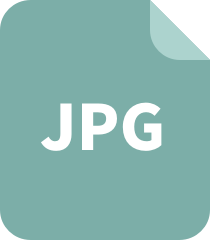
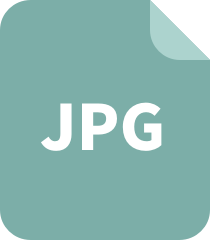
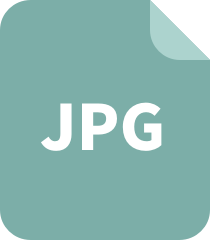
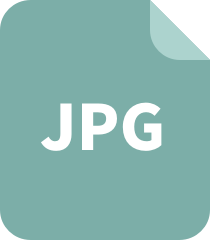
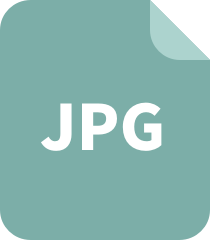
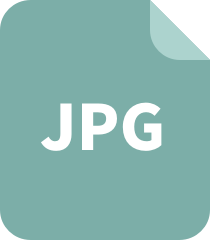
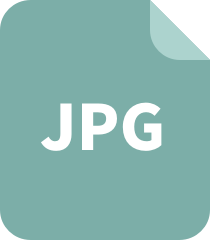
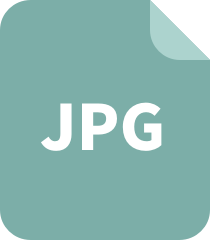
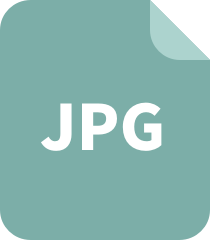
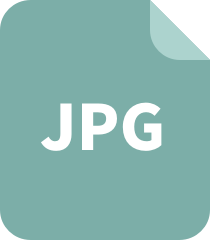
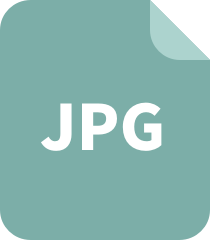
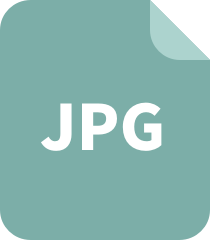
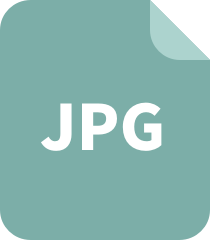
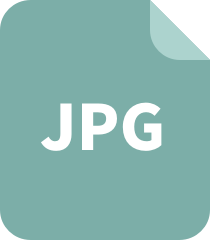
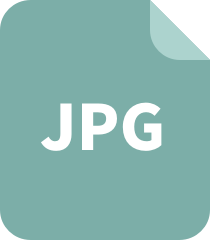
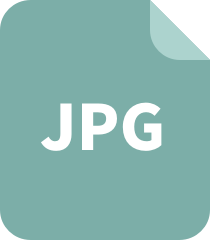
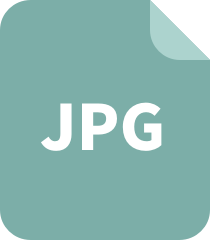
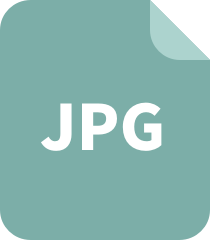
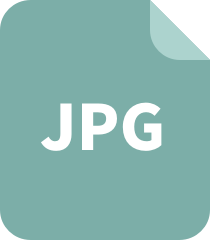
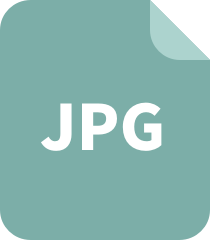
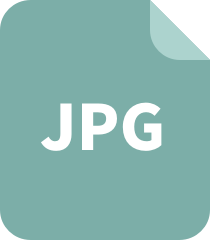
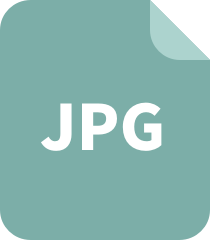
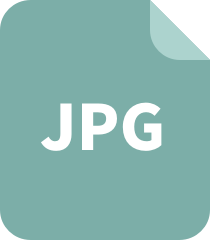
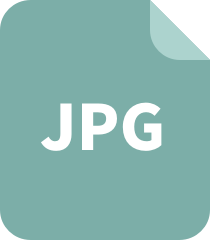
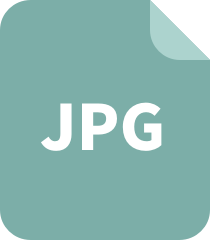
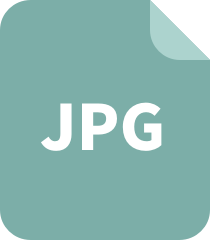
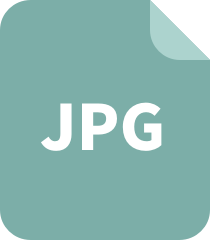
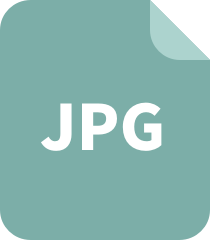
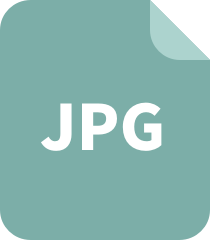
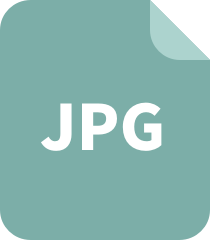
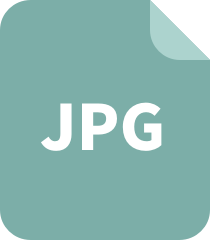
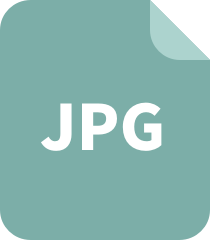
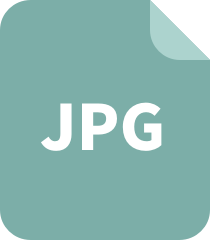
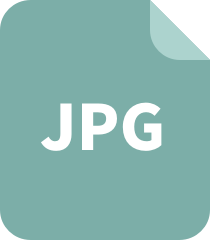
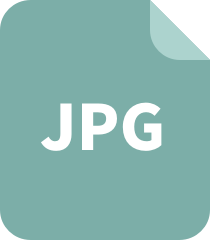
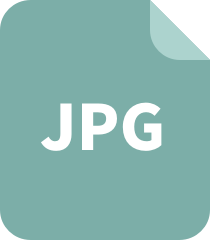
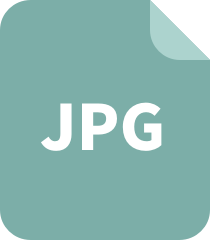
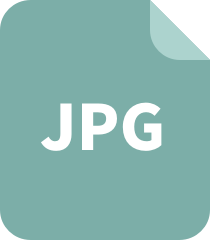
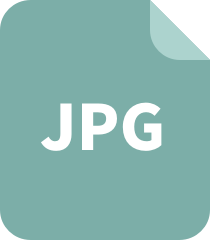
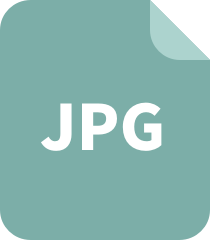
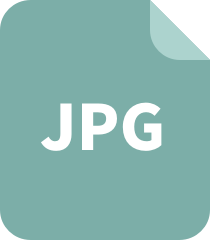
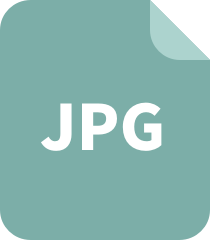
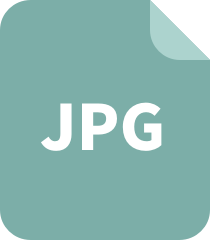
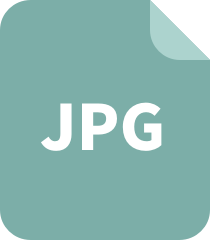
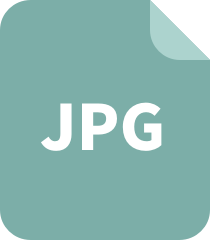
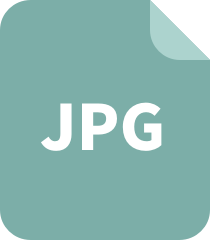
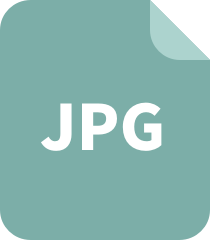
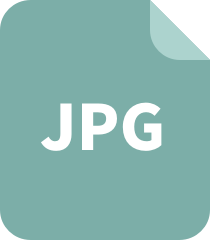
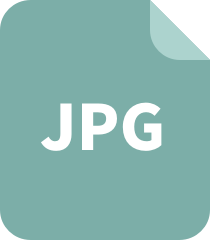
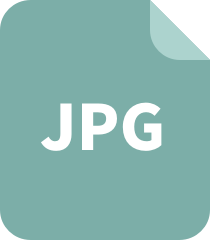
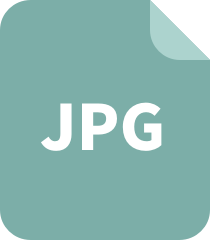
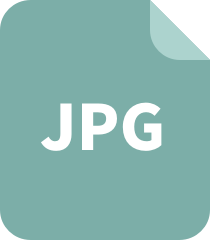
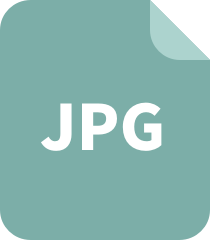
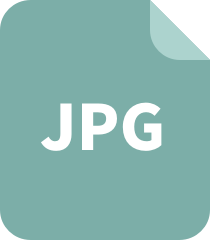
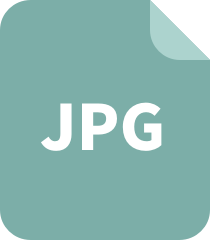
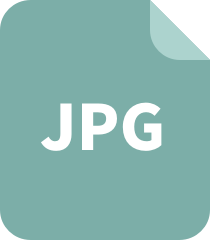
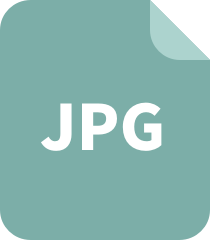
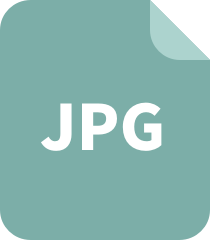
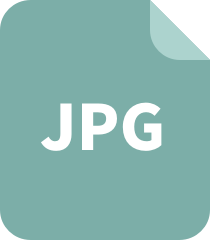
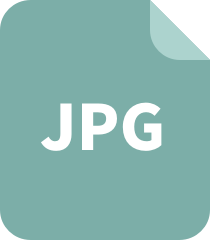
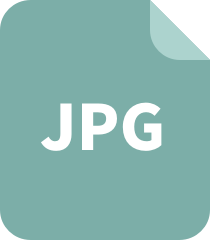
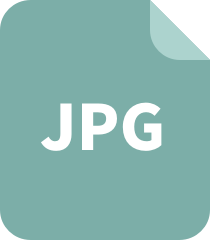
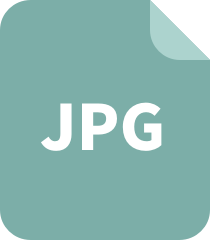
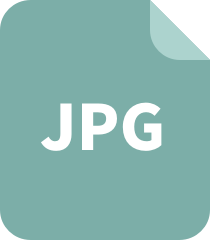
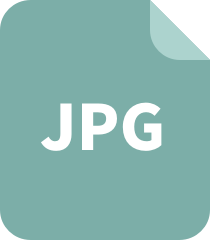
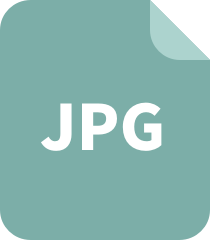
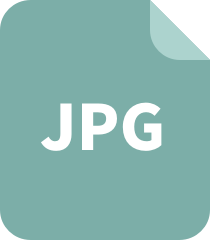
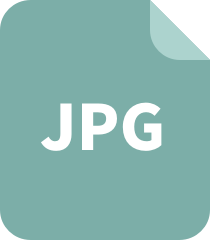
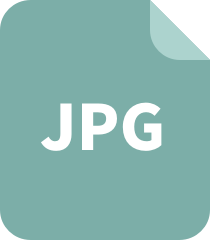
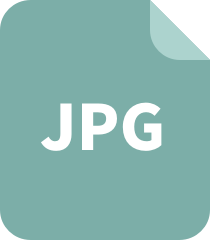
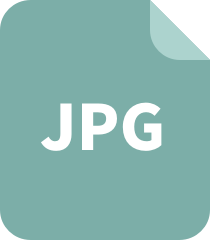
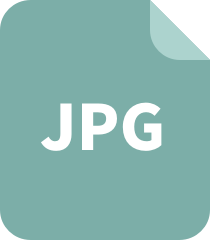
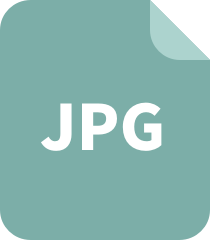
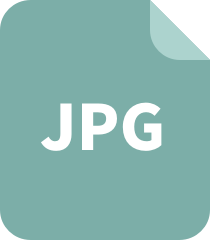
共 2000 条
- 1
- 2
- 3
- 4
- 5
- 6
- 20
资源评论
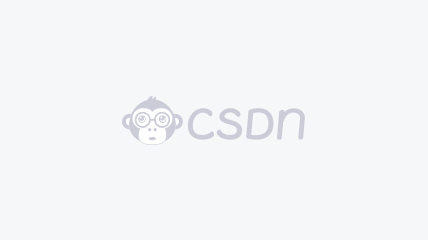

stsdddd
- 粉丝: 2w+
- 资源: 710
上传资源 快速赚钱
我的内容管理 展开
我的资源 快来上传第一个资源
我的收益
登录查看自己的收益我的积分 登录查看自己的积分
我的C币 登录后查看C币余额
我的收藏
我的下载
下载帮助

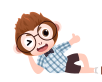
安全验证
文档复制为VIP权益,开通VIP直接复制
