### You Only Look Once ( YOLO v1 )
Implementation of YOLO object detection pipeline using tensorflow. YOLO is a real time object detection method. It treats both object detection and localisation as regression problems. This is in contrast to previous object detection pipelines such as R-CNN, which had seperate entities for detection and localisation and were far more complicated to fine tune/train. More on YOLO [here](https://arxiv.org/pdf/1506.02640.pdf).
As of now, YOLO v2 is out. Check it out [here](https://arxiv.org/pdf/1612.08242.pdf).
### How to use ?
Make sure that weight file is present in weights directory. Currently there are three modes, all pertaining to test the pre-trained model.
- 'testDB' - Tests the code on a database. ( PASCAL VOC 2007, 2012, MS-COCO ). Keep in mind the model has been trained on PASCAL VOC 2007+2012. So any other dataset would require training. As this has not been implemented yet, [darkflow](https://github.com/thtrieu/darkflow) may help.
- 'testLive' - Tests from a live Webcam feed.
- 'testFile' - Tests on a single image.
By default, it runs on 'testLive' mode.
```
python yolo.py
```
### Results (PACAL VOC 2007)
Class Name | Ground Truth | Predicted | True Positive | False Positive | Avg. Precision
---------- | ------------ | --------- | ------------- | -------------- | --------------
aeroplane| 311| 213| 141| 72| 0.55494075262829001
bicycle| 389| 237| 157| 80| 0.56105639251746608
bird| 576| 359| 184| 175| 0.42433899865958929
boat| 393| 213| 77| 136| 0.24679748475368918
bottle| 657| 128| 33| 95| 0.17272727272727273
bus| 254| 168| 117| 51| 0.54621080695222668
car| 1541| 925| 436| 489| 0.34186953795331443
cat| 370| 322| 250| 72| 0.67658801636799115
chair| 1374| 420| 102| 318| 0.12245608573113981
cow| 329| 204| 66| 138| 0.17318304265255621
diningtable| 299| 160| 114| 46| 0.6494860956834515
dog| 530| 422| 299| 123| 0.63538308205967287
horse| 395| 279| 209| 70| 0.63316214093397671
motorbike| 369| 228| 140| 88| 0.48458388143892261
person| 5227| 3319| 1166| 2153| 0.23070649513423477
pottedplant| 592| 200| 53| 147| 0.17236723672367235
sheep| 311| 172| 44| 128| 0.16292819499341238
sofa| 396| 141| 105| 36| 0.6494177280693908
train| 302| 255| 191| 64| 0.66130285346624584
tvmonitor| 361| 209| 133| 76| 0.54199124564843304
### Requirements
- Tenseflow 1.0
- OpenCV 2
- Python 2
- Pre-trained [weights](https://drive.google.com/file/d/0B2JbaJSrWLpza08yS2FSUnV2dlE/view)
#### TODO List
- [x] Complete the mean-Average Precision
- [ ] Document the code
- [x] Add PASCAL VOC 2007 results to the readme
- [ ] Complete network training function
### References
- Author's [Website](https://pjreddie.com/darknet/yolo/)
- [This](https://github.com/hizhangp/yolo_tensorflow) implementation

赵闪闪168
- 粉丝: 1728
- 资源: 6939
最新资源
- 围栏破损检测数据集,954张,带有txt和xml格式的标注
- WPF基于FFmpeg做的媒体转换器 软件支持音频转换、视频转换、视频压缩、视频编辑、合并转换等多种功能.zip
- STM32H750驱动的四轴飞行器实验源码:基于IMU模块的通用四旋翼功能实现,涵盖滤波、控制与通信技术,STM32H750驱动的四轴飞行器实验源码:基于imu模块的滤波、控制与通信技术实现四旋翼通用
- 基于PSO-CNN算法的优化与分类预测:多特征输入单输出模型的迭代混淆矩阵分析图解,PSO-CNN算法优化卷积神经网络分类预测模型:多特征输入迭代优化与混淆矩阵分析的二分类与多分类模型图解,PSO-C
- MicroPython ESP32 ,SSD1306 OLED 显示中文汉字
- 一种基于无监督学习的全局水平辐照度估算方法在光伏功率测量中的应用,全球视角下的无监督光伏功率测量方法:精准估算全局水平辐照度,一种估算光伏功率测量全局水平辐照度的无监督方法 ,核心关键词:光伏功率测量
- 基于PLC的立体车库设计:西门子S7-1200PLC程序与组态仿真技术,电路图及IO表详解,博途软件应用指南,基于PLC的立体车库设计与西门子S7-1200PLC程序开发 通过组态仿真及电路设计,包括
- 基于深度学习的GCNN神经网络模型在EEG数据诊断神经疾病中的应用研究,**GCNN在脑电图EEG神经疾病诊断中的应用:领域引导图卷积神经网络的增强源码数据集**,DL00507-使用领域引导图卷积神
- 智链触屏mqtt通讯协议v2.0(1).pdf
- TortoiseSVN-1.9.7.27907-x64-svn-1.9.7.msi
- 基于Matlab Simulink的电气工程仿真教学:涵盖控制算法与仿真模型制作,基于Matlab Simulink的自动控制教学辅导系统:涵盖控制算法与仿真模型制作实践,代Matlab Simuli
- .NET 面试宝典-2025
- 异步电机与感应电机仿真模型组合推荐:涵盖数学基础模型、无位置速度辨识等十多个模型,适用多种版本,总价特惠 ,基于Matlab/Simulink平台的异步电机与感应电机仿真模型打包,涵盖多种控制策略模型
- Maven Home Path配置Use Maven wrapper时的.mvn文件
- 自动睡眠阶段评分:基于序列到序列深度学习方法的单通道脑电图分析,基于DL00338的序列到序列深度学习自动睡眠阶段评分系统研究与实现,DL00338-使用序列到序列深度学习方法自动睡眠阶段评分 深度学
- 随机孔隙模型与小球生成仿真在光学与微波颗粒加热中的应用:COMSOL与MATLAB联合仿真探究,基于随机孔隙模型与小球生成仿真的光学及微波颗粒加热COMSOL与MATLAB联合仿真研究,随机孔隙模型与
资源上传下载、课程学习等过程中有任何疑问或建议,欢迎提出宝贵意见哦~我们会及时处理!
点击此处反馈


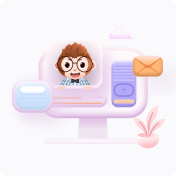