# KAPAO (Keypoints and Poses as Objects)
[Accepted to ECCV 2022](https://arxiv.org/abs/2111.08557)
KAPAO is an efficient single-stage multi-person human pose estimation method that models
**k**eypoints **a**nd **p**oses **a**s **o**bjects within a dense anchor-based detection framework.
KAPAO simultaneously detects _pose objects_ and _keypoint objects_ and fuses the detections to predict human poses:

When not using test-time augmentation (TTA), KAPAO is much faster and more accurate than
previous single-stage methods like
[DEKR](https://github.com/HRNet/DEKR),
[HigherHRNet](https://github.com/HRNet/HigherHRNet-Human-Pose-Estimation),
[HigherHRNet + SWAHR](https://github.com/greatlog/SWAHR-HumanPose), and
[CenterGroup](https://github.com/dvl-tum/center-group):

This repository contains the official PyTorch implementation for the paper: <br>
Rethinking Keypoint Representations: Modeling Keypoints and Poses as Objects for Multi-Person Human Pose Estimation.
Our code was forked from ultralytics/yolov5 at commit [5487451](https://github.com/ultralytics/yolov5/tree/5487451).
### Setup
1. If you haven't already, [install Anaconda or Miniconda](https://docs.conda.io/projects/conda/en/latest/user-guide/install/index.html).
2. Create a new conda environment with Python 3.6: `$ conda create -n kapao python=3.6`.
3. Activate the environment: `$ conda activate kapao`
4. Clone this repo: `$ git clone https://github.com/wmcnally/kapao.git`
5. Install the dependencies: `$ cd kapao && pip install -r requirements.txt`
6. Download the trained models: `$ python data/scripts/download_models.py`
## Inference Demos
**Note:** FPS calculations include **all processing** (i.e., including image loading, resizing, inference, plotting / tracking, etc.).
See script arguments for inference options.
---
#### Static Image
To generate the four images in the GIF above:
1. `$ python demos/image.py --bbox`
2. `$ python demos/image.py --bbox --pose --face --no-kp-dets`
3. `$ python demos/image.py --bbox --pose --face --no-kp-dets --kp-bbox`
4. `$ python demos/image.py --pose --face`
#### Shuffling Video
KAPAO runs fastest on low resolution video with few people in the frame. This demo runs KAPAO-S on a single-person 480p dance video using an input size of 1024.
The inference speed is **~9.5 FPS** on our CPU, and **~60 FPS** on our TITAN Xp.
**CPU inference:**<br>
<br>
To display the results in real-time: <br>
`$ python demos/video.py --face --display`
To create the GIF above:<br>
`$ python demos/video.py --face --device cpu --gif`
**CPU specs:**<br>
Intel Core i7-8700K<br>
16GB DDR4 3000MHz<br>
Samsung 970 Pro M.2 NVMe SSD<br>
---
#### Flash Mob Video
This demo runs KAPAO-S on a 720p flash mob video using an input size of 1280.
**GPU inference:**<br>
<br>
To display the results in real-time: <br>
`$ python demos/video.py --yt-id 2DiQUX11YaY --tag 136 --imgsz 1280 --color 255 0 255 --start 188 --end 196 --display`
To create the GIF above:<br>
`$ python demos/video.py --yt-id 2DiQUX11YaY --tag 136 --imgsz 1280 --color 255 0 255 --start 188 --end 196 --gif`
---
#### Red Light Green Light
This demo runs KAPAO-L on a 480p clip from the TV show _Squid Game_ using an input size of 1024.
The plotted poses constitute keypoint objects only.
**GPU inference:**<br>
<br>
To display the results in real-time:<br>
`$ python demos/video.py --yt-id nrchfeybHmw --imgsz 1024 --weights kapao_l_coco.pt --conf-thres-kp 0.01 --kp-obj --face --start 56 --end 72 --display`
To create the GIF above:<br>
`$ python demos/video.py --yt-id nrchfeybHmw --imgsz 1024 --weights kapao_l_coco.pt --conf-thres-kp 0.01 --kp-obj --face --start 56 --end 72 --gif`
---
#### Squash Video
This demo runs KAPAO-S on a 1080p slow motion squash video. It uses a simple player tracking algorithm based on the frame-to-frame pose differences.
**GPU inference:**<br>
<br>
To display the inference results in real-time: <br>
`$ python demos/squash.py --display --fps`
To create the GIF above:<br>
`$ python demos/squash.py --start 42 --end 50 --gif --fps`
---
#### Depth Video
Pose objects generalize well and can even be detected in depth video.
Here KAPAO-S was run on a depth video from a [fencing action recognition dataset](https://ieeexplore.ieee.org/abstract/document/8076041?casa_token=Zvm7dLIr1rYAAAAA:KrqtVl3NXrJZn05Eb4KGMio-18VPHc3uyDJZSiNJyI7f7oHQ5V2iwB7bK4mCJCmN83NrRl4P).
<br>
The depth video above can be downloaded directly from [here](https://drive.google.com/file/d/1n4so5WN6snyCYxeUk4xX1glADqQuitXP/view?usp=sharing).
To create the GIF above:<br>
`$ python demos/video.py -p 2016-01-04_21-33-35_Depth.avi --face --start 0 --end -1 --gif --gif-size 480 360`
---
#### Web Demo
A web demo was integrated to [Huggingface Spaces](https://huggingface.co/spaces) with [Gradio](https://github.com/gradio-app/gradio) (credit to [@AK391](https://github.com/AK391)).
It uses KAPAO-S to run CPU inference on short video clips.
## COCO Experiments
Download the COCO dataset: `$ sh data/scripts/get_coco_kp.sh`
### Validation (without TTA)
- KAPAO-S (63.0 AP): `$ python val.py --rect`
- KAPAO-M (68.5 AP): `$ python val.py --rect --weights kapao_m_coco.pt`
- KAPAO-L (70.6 AP): `$ python val.py --rect --weights kapao_l_coco.pt`
### Validation (with TTA)
- KAPAO-S (64.3 AP): `$ python val.py --scales 0.8 1 1.2 --flips -1 3 -1`
- KAPAO-M (69.6 AP): `$ python val.py --weights kapao_m_coco.pt \ `<br>
`--scales 0.8 1 1.2 --flips -1 3 -1`
- KAPAO-L (71.6 AP): `$ python val.py --weights kapao_l_coco.pt \ `<br>
`--scales 0.8 1 1.2 --flips -1 3 -1`
### Testing
- KAPAO-S (63.8 AP): `$ python val.py --scales 0.8 1 1.2 --flips -1 3 -1 --task test`
- KAPAO-M (68.8 AP): `$ python val.py --weights kapao_m_coco.pt \ `<br>
`--scales 0.8 1 1.2 --flips -1 3 -1 --task test`
- KAPAO-L (70.3 AP): `$ python val.py --weights kapao_l_coco.pt \ `<br>
`--scales 0.8 1 1.2 --flips -1 3 -1 --task test`
### Training
The following commands were used to train the KAPAO models on 4 V100s with 32GB memory each.
KAPAO-S:
```
python -m torch.distributed.launch --nproc_per_node 4 train.py \
--img 1280 \
--batch 128 \
--epochs 500 \
--data data/coco-kp.yaml \
--hyp data/hyps/hyp.kp-p6.yaml \
--val-scales 1 \
--val-flips -1 \
--weights yolov5s6.pt \
--project runs/s_e500 \
--name train \
--workers 128
```
KAPAO-M:
```
python train.py \
--img 1280 \
--batch 72 \
--epochs 500 \
--data data/coco-kp.yaml \
--hyp data/hyps/hyp.kp-p6.yaml \
--val-scales 1 \
--val-flips -1 \
--weights yolov5m6.pt \
--project runs/m_e500 \
--name train \
--workers 128
```
KAPAO-L:
```
python train.py \
--img 1280 \
--batch 48 \
--epochs 500 \
--data data/coco-kp.yaml \
--hyp data/hyps/hyp.kp-p6.yaml \
--val-scales 1 \
--val-flips -1 \
--weights yolov5l6.pt \
--project runs/l_e500 \
--name train \
--workers 128
```
**Note:** [DDP](https://pytorch.org/tutorials/intermediate/ddp_tutorial.html) is usually recommended but we found training was less stable for KAPAO-M/L using DDP. We are investigating this issue.
## CrowdPose Experiments
- Install the [CrowdPose API](https://github.com/Jeff-sjtu/CrowdPose/tree/master/crowdpose-api) to your conda environment: <br>
`$ cd .. && git clone https://github.com/Jeff-sjtu/CrowdPose.git` <br>
`$ cd CrowdPose/crowdpose-api/PythonAPI && sh install.sh && cd ../../../kapao`
- Download the CrowdPose dataset: `$ sh data/scripts/get_crowdpose.sh`
### Testing
- KAPAO-S (63.8 AP): `$ python val.py --data crowdpose.yaml \ `<br>
`--weights kapao_s_crowdpose.pt --scales 0.8 1 1.2 --flips -1 3 -1`
- KAPAO-M (67.1 AP): `$ python val.py --data crowdpose.yaml \ `<br>
`--weights kapa

赵闪闪168
- 粉丝: 1726
- 资源: 6943
最新资源
- 【流体】基于matlab纳维-斯托克斯方程模拟平板上的超音速流动【含Matlab源码 10954期】.zip
- 【化工】基于matlab端点模型预测控制器MPC控制青霉素和CHO细胞生产多变量【含Matlab源码 10953期】.zip
- 【光学】基于matlab计算石墨烯非线性光带和自激类克尔效应【含Matlab源码 10952期】.zip
- 【流体学】基于matlab求解粘性流体通过矩形管道流动的速度扩散【含Matlab源码 10955期】.zip
- 【数据驱动】基于matlab化学势场制定稳态和瞬态质量扩散问题【含Matlab源码 10946期】.zip
- 【数据驱动】基于matlab Koopman算子理论对恒化器模型数据驱动【含Matlab源码 10937期】.zip
- 【数据驱动】基于matlab数据驱动GGM-ET-PHD滤波器【含Matlab源码 10941期】.zip
- 【数据驱动】基于matlab数据驱动的最大轮胎道路摩擦系数预测【含Matlab源码 10945期】.zip
- 【数据驱动】基于matlab数据驱动BORM仿真【含Matlab源码 10940期】.zip
- 【数据驱动】基于matlab数据驱动模型预测控制MPC(闭环保证)【含Matlab源码 10944期】.zip
- 【数据驱动】基于matlab数据驱动动态系统分析的流形学习【含Matlab源码 10943期】.zip
- 【数据驱动】基于matlab四维随机射弹系统的数据驱动建模【含Matlab源码 10947期】.zip
- 【数据驱动】基于matlab系统识别工具箱实时数据驱动控制【含Matlab源码 10938期】.zip
- 【图像分割】基于matlab数字形态学数字视网膜图像血管提取DRIVE数据集分割【含Matlab源码 10948期】.zip
- 【湍流】基于matlab模拟高斯光束通过大气湍流相位屏【含Matlab源码 10956期】.zip
- 毕业设计部署yolov9模型ncnn模型到树莓派4或5嵌入式C++源码.zip
资源上传下载、课程学习等过程中有任何疑问或建议,欢迎提出宝贵意见哦~我们会及时处理!
点击此处反馈


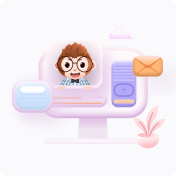