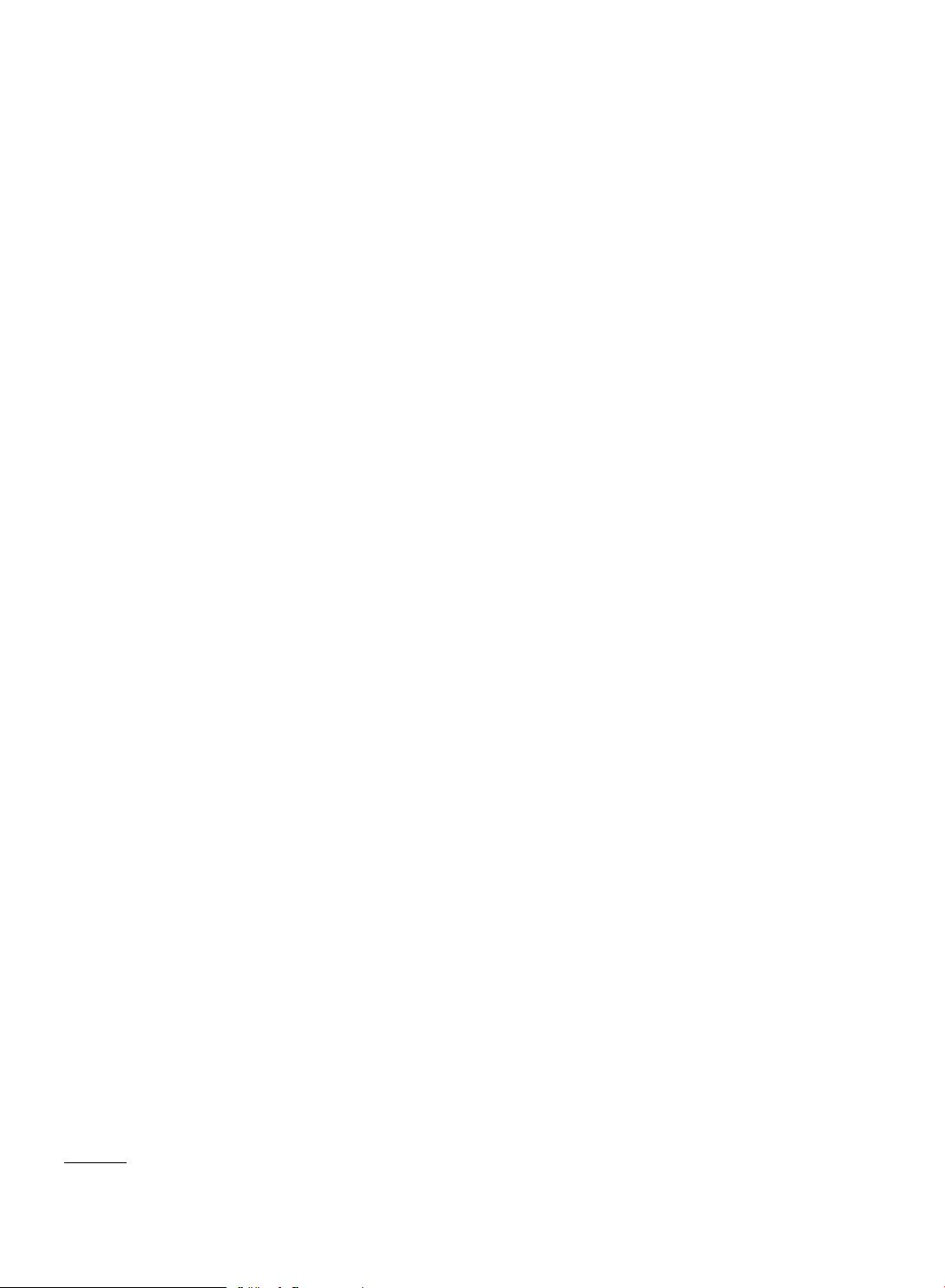
L. Lü et al. / Physics Reports 519 (2012) 1–49 5
For example, Baifendian developed a technique that could track individual users’ activities in several e-commerce sites,
so that for a cold-start user in site A, we could make recommendation according to her records in sites B, C , D, etc [34].
4. Diversity vs. accuracy. When the task is to recommend items which are likely to be appreciated by a particular user, it
is usually most effective to recommend popular and highly rated items. Such recommendation, however, has very little
value for the users because popular objects are easy to find (often they are even hard to avoid) without a recommender
system. A good list of recommended items hence should contain also less obvious items that are unlikely to be reached
by the users themselves [35]. Approaches to this problem include direct enhancement of the recommendation list’s
diversity [36–38] and the use of hybrid recommendation methods [39].
5. Vulnerability to attacks. Due to their importance in e-commerce applications, recommender systems are likely targets of
malicious attacks trying to unjustly promote or inhibit some items [40]. There is a wide scale of tools preventing this
kind of behavior, ranging from blocking the malicious evaluations from entering the system to sophisticated resistant
recommendation techniques [41]. However, this is not a easy task since the strategies of attackers also get more and more
advanced as the developing of preventing tools. As an example, Burke et al. [42] introduced eight attacking strategies,
which are further divided into four classes: basic attack, low-acknowledge attack, nuke attack and informed attack.
6. The value of time. While real users have interests with widely diverse time scales (for example, short term interests related
to a planned trip and long term interests related to the place of living or political preferences), most recommendation
algorithms neglect the time stamps of evaluations. It is an ongoing line of research whether and how value of old opinions
should decay with time and what are the typical temporary patterns in user evaluations and item relevance [43,44].
7. Evaluation of recommendations. While we have plenty of distinct metrics (see Section 3.4), how to choose the ones best
corresponding to the given situation and task is still an open question. Comparisons of different recommender algorithms
are also problematic because different algorithms may simply solve different tasks. Finally, the overall user experience
with a given recommendation system – which includes user’s satisfaction with the recommendations and user’s trust in
the system – is difficult to measure in ‘‘offline’’ evaluation. Empirical user studies thus still represent a welcome source
of feedback on recommender systems.
8. User interface. It has been shown that to facilitate users’ acceptance of recommendations, the recommendations need to
be transparent [45,46]: users appreciate when it is clear why a particular item has been recommended to them. Another
issue is that since the list of potentially interesting items may be very long, it needs to be presented in a simple way
and it should be easy to navigate through it to browse different recommendations which are often obtained by distinct
approaches.
Besides the above long-standing challenges, many novel issues appear recently. Thanks to the development of
methodology in related branches of science, especially the new tools in network analysis, scientists started to consider
the effects of network structure on recommendation and how to make use of known structural features to improve
recommendation. For example, Huang et al. [47] analyzed the consumer–product networks and proposed an improved
recommendation algorithm preferring edges that enhance the local clustering property, and Sahebi et al. [48] designed an
improved algorithm making use of the community structure. Progress and propagation of new techniques also bring new
challenges. For example, the GPS equipped mobile phones have become mainstream and the Internet access is ubiquitous,
hence the location-based recommendation is now feasible and increasingly significant.
3
Accurate recommendation asks for
both the high predictability of human movements [49,50] and quantitative way to define similarities between locations
and people [51,52]. Lastly, intelligent recommender systems should take into account the different behavioral patterns
of different people. For example, new users tend to visit very popular items and select similar items, while old users
usually have more specific interests [53,54], and users behave much differently between low-risk (e.g., collecting bookmarks,
downloading music, etc.) and high-risk (e.g., buying a computer, renting a house, etc.) activities [55,56].
3. Definitions of subjects and problems
We briefly review in this chapter basic concepts that are useful in the study of recommender systems.
3.1. Networks
Network analysis is a versatile tool in uncovering the organization principles of many complex systems [5–9]. A network
is a set of elements (called nodes or vertices) with connections (called edges or links) between them. Many social, biological,
technological and information systems can be described as networks with nodes representing individuals or organizations
and edges capturing their interactions. The study of networks, referred to as graph theory in mathematical literature, has a
long history that begins with the classical Königsberg bridge problem solved by Euler in 18th century [57]. Mathematically
speaking, a network G is an ordered pair of disjoint sets (V , E) where V is the set of nodes and the set of edges, E, is a subset
of V × V [58]. In an undirected network, an edge joining nodes x and y is denoted by x ↔ y, and x ↔ y and y ↔ x mean
exactly the same edge. In a directed network, edges are ordered pairs of nodes and an edge from x to y is denoted by x → y.
3
Websites like Foursquare, Gowalla, Google Latitude, Facebook, Jiapang, and others already provide location-based services and show that many people
want to share their location information and get location-based recommendations.