### Tensorflow-ChebNet-Tutorial
***
当GCN如日中天的时候,大部分人并不知道GCN其实是对ChebyNet的进一步简化与近似,ChebyNet与GCN都属于谱域上定义的图卷积网络。本教程将教你如何用Tensorflow构建ChebyNet模型进行节点分类任务。完整的代码可在Github中下载:https://github.com/CrawlScript/tf_geometric/blob/master/demo/demo_chebynet.py
### ChebyNet简介
***
由图上的傅里叶变换公式我们可以得到卷积核h在图f上的卷积公式:
* 第一代GCN中简单把中的对角线元素替换为参数,此时的GCN就变成了这个样子:
但是这样问题很多,如:
1. 图卷积核参数量大,参数量与图中的节点的数量相同。
2. 卷积核是全局的。
3. 运算过程设计到特征分解,复杂度高。
* 为了克服以上问题,第二代GCN进行了针对性的改进:用k阶多项式来近似卷积核: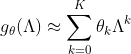,将其代入到可以得到
所以第二代GCN的卷积公式是: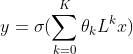
第二代GCN直接对拉普拉斯矩阵进行变换,不再需要特征分解这一耗时大户。
* ChebNet在第二代GCN的基础上用ChebyShev多项式展开对卷积核进行近似,即令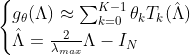
切比雪夫多项式的递归定义: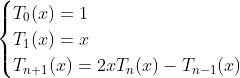
这样有两个好处:
1. 卷积核的参数从原先一代GCN中的n个减少到k个,从原先的全局卷积变为现在的局部卷积,即将距离中心节点k-hop的节点作为邻居节点。
2. 通过切比雪夫多项式的迭代定义降低了计算复杂度。
因此切比雪夫图卷积公式变为: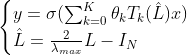
**对上述推导过程不清楚的人可以参考我的博客**:https://www.jianshu.com/p/35212baf6671
教程完整代码链接:https://github.com/CrawlScript/tf_geometric/blob/master/demo/demo_chebynet.py
论文地址:https://arxiv.org/pdf/1606.09375.pdf
### 教程目录
***
* 开发环境
* ChebyNet的实现
* 模型构建
* ChebyNet训练
* ChebyNet评估
### 开发环境
***
* 操作系统: Windows / Linux / Mac OS
* Python 版本: >= 3.5
* 依赖包:
- tf_geometric(一个基于Tensorflow的GNN库)
根据你的环境(是否已安装TensorFlow、是否需要GPU)从下面选择一条安装命令即可一键安装所有Python依赖:
```python
pip install -U tf_geometric # 这会使用你自带的TensorFlow,注意你需要tensorflow/tensorflow-gpu >= 1.14.0 or >= 2.0.0b1
pip install -U tf_geometric[tf1-cpu] # 这会自动安装TensorFlow 1.x CPU版
pip install -U tf_geometric[tf1-gpu] # 这会自动安装TensorFlow 1.x GPU版
pip install -U tf_geometric[tf2-cpu] # 这会自动安装TensorFlow 2.x CPU版
pip install -U tf_geometric[tf2-gpu] # 这会自动安装TensorFlow 2.x GPU版
```
教程使用的核心库是tf_geometric,一个基于TensorFlow的GNN库。tf_geometric的详细教程可以在其Github主页上查询:
* https://github.com/CrawlScript/tf_geometric
### ChebyNet的实现
***
对图的邻接矩阵进行归一化处理得到拉普拉斯矩阵(归一化的方式有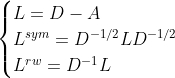),以及根据得到的归一化的拉普拉斯矩阵计算![This is the rendered form of the equation. You can not edit this directly. Right click will give you the option to save the image, and in most browsers you can drag the image onto