#include <stdio.h>
#include <stdlib.h>
#include <string.h>
#include <ctype.h>
#include "svm.h"
#include "mex.h"
#include "svm_model_matlab.h"
#ifdef MX_API_VER
#if MX_API_VER < 0x07030000
typedef int mwIndex;
#endif
#endif
#define CMD_LEN 2048
#define Malloc(type,n) (type *)malloc((n)*sizeof(type))
void print_null(const char *s) {}
void print_string_matlab(const char *s) {mexPrintf(s);}
void exit_with_help()
{
mexPrintf(
"Usage: model = svmtrain(training_label_vector, training_instance_matrix, 'libsvm_options');\n"
"libsvm_options:\n"
"-s svm_type : set type of SVM (default 0)\n"
" 0 -- C-SVC (multi-class classification)\n"
" 1 -- nu-SVC (multi-class classification)\n"
" 2 -- one-class SVM\n"
" 3 -- epsilon-SVR (regression)\n"
" 4 -- nu-SVR (regression)\n"
"-t kernel_type : set type of kernel function (default 2)\n"
" 0 -- linear: u'*v\n"
" 1 -- polynomial: (gamma*u'*v + coef0)^degree\n"
" 2 -- radial basis function: exp(-gamma*|u-v|^2)\n"
" 3 -- sigmoid: tanh(gamma*u'*v + coef0)\n"
" 4 -- precomputed kernel (kernel values in training_instance_matrix)\n"
"-d degree : set degree in kernel function (default 3)\n"
"-g gamma : set gamma in kernel function (default 1/num_features)\n"
"-r coef0 : set coef0 in kernel function (default 0)\n"
"-c cost : set the parameter C of C-SVC, epsilon-SVR, and nu-SVR (default 1)\n"
"-n nu : set the parameter nu of nu-SVC, one-class SVM, and nu-SVR (default 0.5)\n"
"-p epsilon : set the epsilon in loss function of epsilon-SVR (default 0.1)\n"
"-m cachesize : set cache memory size in MB (default 100)\n"
"-e epsilon : set tolerance of termination criterion (default 0.001)\n"
"-h shrinking : whether to use the shrinking heuristics, 0 or 1 (default 1)\n"
"-b probability_estimates : whether to train a SVC or SVR model for probability estimates, 0 or 1 (default 0)\n"
"-wi weight : set the parameter C of class i to weight*C, for C-SVC (default 1)\n"
"-v n : n-fold cross validation mode\n"
"-q : quiet mode (no outputs)\n"
);
}
// svm arguments
struct svm_parameter param; // set by parse_command_line
struct svm_problem prob; // set by read_problem
struct svm_model *model;
struct svm_node *x_space;
int cross_validation;
int nr_fold;
double do_cross_validation()
{
int i;
int total_correct = 0;
double total_error = 0;
double sumv = 0, sumy = 0, sumvv = 0, sumyy = 0, sumvy = 0;
double *target = Malloc(double,prob.l);
double retval = 0.0;
svm_cross_validation(&prob,¶m,nr_fold,target);
if(param.svm_type == EPSILON_SVR ||
param.svm_type == NU_SVR)
{
for(i=0;i<prob.l;i++)
{
double y = prob.y[i];
double v = target[i];
total_error += (v-y)*(v-y);
sumv += v;
sumy += y;
sumvv += v*v;
sumyy += y*y;
sumvy += v*y;
}
mexPrintf("Cross Validation Mean squared error = %g\n",total_error/prob.l);
mexPrintf("Cross Validation Squared correlation coefficient = %g\n",
((prob.l*sumvy-sumv*sumy)*(prob.l*sumvy-sumv*sumy))/
((prob.l*sumvv-sumv*sumv)*(prob.l*sumyy-sumy*sumy))
);
retval = total_error/prob.l;
}
else
{
for(i=0;i<prob.l;i++)
if(target[i] == prob.y[i])
++total_correct;
mexPrintf("Cross Validation Accuracy = %g%%\n",100.0*total_correct/prob.l);
retval = 100.0*total_correct/prob.l;
}
free(target);
return retval;
}
// nrhs should be 3
int parse_command_line(int nrhs, const mxArray *prhs[], char *model_file_name)
{
int i, argc = 1;
char cmd[CMD_LEN];
char *argv[CMD_LEN/2];
void (*print_func)(const char *) = print_string_matlab; // default printing to matlab display
// default values
param.svm_type = C_SVC;
param.kernel_type = RBF;
param.degree = 3;
param.gamma = 0; // 1/num_features
param.coef0 = 0;
param.nu = 0.5;
param.cache_size = 100;
param.C = 1;
param.eps = 1e-3;
param.p = 0.1;
param.shrinking = 1;
param.probability = 0;
param.nr_weight = 0;
param.weight_label = NULL;
param.weight = NULL;
cross_validation = 0;
if(nrhs <= 1)
return 1;
if(nrhs > 2)
{
// put options in argv[]
mxGetString(prhs[2], cmd, mxGetN(prhs[2]) + 1);
if((argv[argc] = strtok(cmd, " ")) != NULL)
while((argv[++argc] = strtok(NULL, " ")) != NULL)
;
}
// parse options
for(i=1;i<argc;i++)
{
if(argv[i][0] != '-') break;
++i;
if(i>=argc && argv[i-1][1] != 'q') // since option -q has no parameter
return 1;
switch(argv[i-1][1])
{
case 's':
param.svm_type = atoi(argv[i]);
break;
case 't':
param.kernel_type = atoi(argv[i]);
break;
case 'd':
param.degree = atoi(argv[i]);
break;
case 'g':
param.gamma = atof(argv[i]);
break;
case 'r':
param.coef0 = atof(argv[i]);
break;
case 'n':
param.nu = atof(argv[i]);
break;
case 'm':
param.cache_size = atof(argv[i]);
break;
case 'c':
param.C = atof(argv[i]);
break;
case 'e':
param.eps = atof(argv[i]);
break;
case 'p':
param.p = atof(argv[i]);
break;
case 'h':
param.shrinking = atoi(argv[i]);
break;
case 'b':
param.probability = atoi(argv[i]);
break;
case 'q':
print_func = &print_null;
i--;
break;
case 'v':
cross_validation = 1;
nr_fold = atoi(argv[i]);
if(nr_fold < 2)
{
mexPrintf("n-fold cross validation: n must >= 2\n");
return 1;
}
break;
case 'w':
++param.nr_weight;
param.weight_label = (int *)realloc(param.weight_label,sizeof(int)*param.nr_weight);
param.weight = (double *)realloc(param.weight,sizeof(double)*param.nr_weight);
param.weight_label[param.nr_weight-1] = atoi(&argv[i-1][2]);
param.weight[param.nr_weight-1] = atof(argv[i]);
break;
default:
mexPrintf("Unknown option -%c\n", argv[i-1][1]);
return 1;
}
}
svm_set_print_string_function(print_func);
return 0;
}
// read in a problem (in svmlight format)
int read_problem_dense(const mxArray *label_vec, const mxArray *instance_mat)
{
// using size_t due to the output type of matlab functions
size_t i, j, k, l;
size_t elements, max_index, sc, label_vector_row_num;
double *samples, *labels;
prob.x = NULL;
prob.y = NULL;
x_space = NULL;
labels = mxGetPr(label_vec);
samples = mxGetPr(instance_mat);
sc = mxGetN(instance_mat);
elements = 0;
// number of instances
l = mxGetM(instance_mat);
label_vector_row_num = mxGetM(label_vec);
prob.l = (int)l;
if(label_vector_row_num!=l)
{
mexPrintf("Length of label vector does not match # of instances.\n");
return -1;
}
if(param.kernel_type == PRECOMPUTED)
elements = l * (sc + 1);
else
{
for(i = 0; i < l; i++)
{
for(k = 0; k < sc; k++)
if(samples[k * l + i] != 0)
elements++;
// count the '-1' element
elements++;
}
}
prob.y = Malloc(double,l);
prob.x = Malloc(struct svm_node *,l);
x_space = Malloc(struct svm_node, elements);
max_index = sc;
j = 0;
for(i = 0; i < l; i++)
{
prob.x[i] = &x_space[j];
prob.y[i] = labels[i];
for(k = 0; k < sc; k++)
{
if(param.kernel_type == PRECOMPUTED || samples[k * l + i] != 0)
{
x_space[j].index = (int)k + 1;
x_space[j].value = samples[k * l + i];
j++;
}
}
x_space[j++].index = -1;
}
if(param.gamma == 0 && max_index > 0)
param.gamma = (double)(1.0/max_index);
if(param.kernel_type == PRECOMPUTED)
for(i=0;i<l;i++)
{
if((int)prob.x[i][0].value <= 0 || (int)prob.x[i][0].value > (int)max_index)
{
mexPrintf("Wrong input format: sample_serial_number out of range\n");
return -1;
}
}
return 0;
}
int read_problem_sparse(const mxArray *label_vec, const mxArray *instance_mat)
{
mwIndex *ir, *jc, low, high, k;
// using size_t due to the output type of matlab functions
size_t i, j, l, elements, max_index, label_vector_row_num;
mwSize num_samples;
double *samples, *labels;
mxArray *instance_mat_col; // transposed instance sparse matrix
prob.x = NULL;
prob.y = NULL;
x_space = NULL;
// transpose instance matrix
{
mxArray *prhs[1], *plhs[1];
prhs[0] = mxDuplicateArray(instance_mat);
if(mexCallMATLA

wjs2024
- 粉丝: 2737
- 资源: 6127
最新资源
- Cocos2d-x 2.2.1 版本资源
- 关于DeepSeek的几点思考.pdf
- 基于ISODATA算法优化的负荷场景曲线聚类方法(包含K-means、L-ISODATA及K-L-ISODATA算法,聚类效果评价与风光场景应用),基于ISODATA改进算法的负荷场景曲线聚类:多方法
- qpress的二进制命令
- 基于Matlab Simulink的风光储并网双闭环控制策略及单极调制协同运行模型研究,基于Matlab Simulink的风光储并网双闭环控制策略及其单极调制协同运行模型研究,风光储并网协同运行模型
- deepseek详细对话
- 遗传算法与动态窗口法DWA融合优化:全局路径规划与动态环境适应的算法创新,遗传算法优化与动态窗口法融合:全局最优路径规划与动态环境适应策略,遗传算法(GA)优化与动态窗口法dwa融合,效果极佳,算法新
- COMSOL仿真揭示变压器磁致伸缩现象:电路磁场分布、振动噪声及受力和噪声分布研究,COMSOL仿真揭示变压器磁致伸缩机理:电路磁场分布、振动数据与噪声分布综合分析,COMSOL仿真,变压器磁致伸缩
- 数据科学相关人员的岗位及薪资数据.zip
- 零基础入门转录组下游分析-机器学习算法之xgboost(筛选特征基因)教程配套资源
- 基于相量模型的UPFC在500kV与230kV输电系统中的功率流控仿真研究,基于相量模型的UPFC在500kV与230kV输电系统中的功率流控仿真研究,UPFC(相量模型)控制500 kV 230
- 中秋节静态网站网页设计(HTML+CSS+JS)期末大作业.zip
- C#开发TCP通信demo
- 基于Electron+HTML+CSS+Node.js开发的的中国象棋游戏,支持人机对大模型AI对战 (源码)
- 基于粒子群算法的地表水源热泵系统建模与最佳制冷制热量求解研究,水源热泵系统建模与粒子群优化:求解热泵机组最佳制冷制热量算法研究,matlab代码 从水源热泵机组角度对地表水源热泵系统建模,并采用粒子群
- COCOS2DX 2.2.2 引擎下载
资源上传下载、课程学习等过程中有任何疑问或建议,欢迎提出宝贵意见哦~我们会及时处理!
点击此处反馈


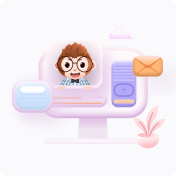