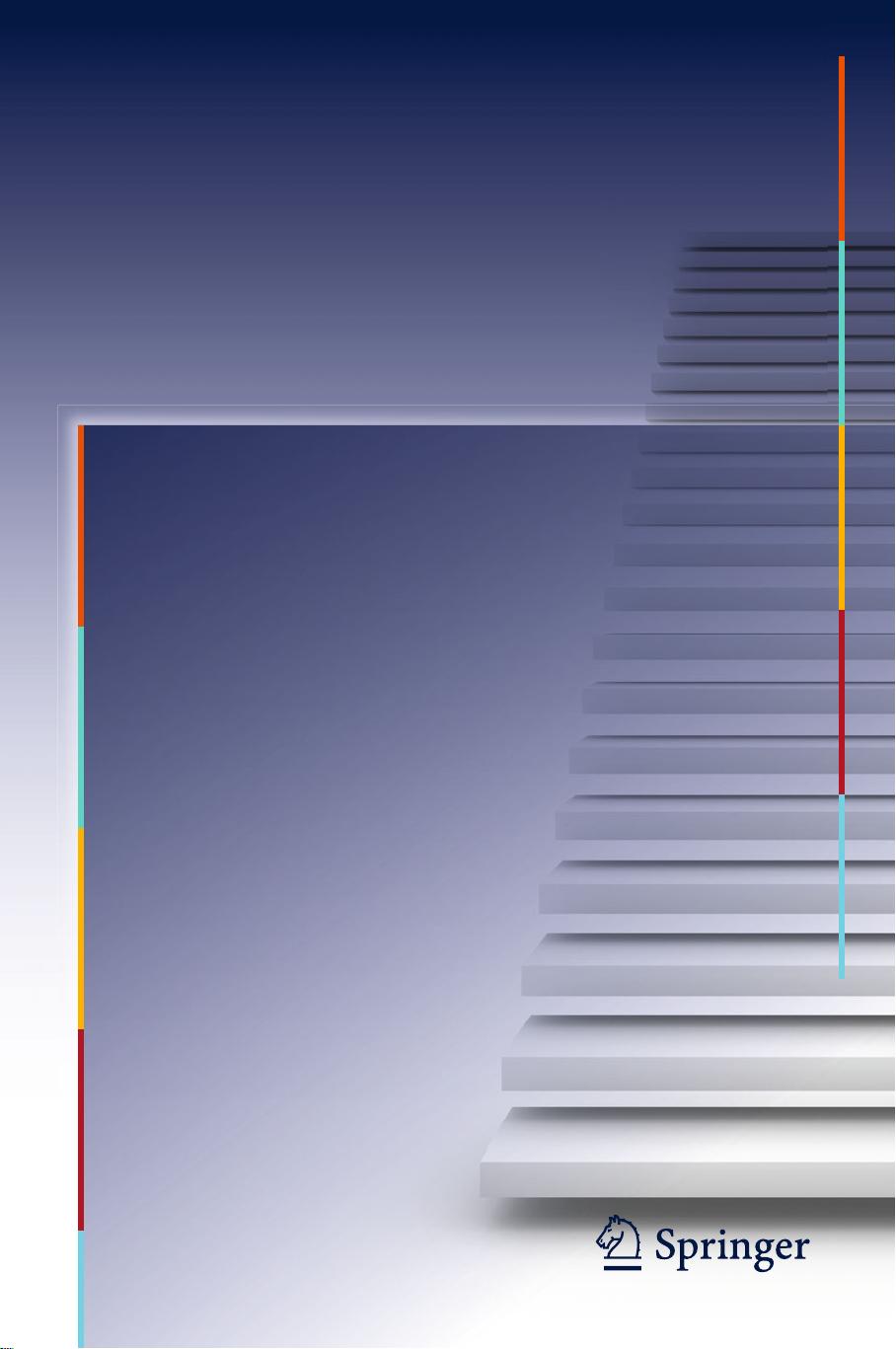
Springer Theses
Recognizing Outstanding Ph.D. Research
Machine Learning
Methods for
Behaviour Analysis
and Anomaly
Detection in Video
Olga Isupova
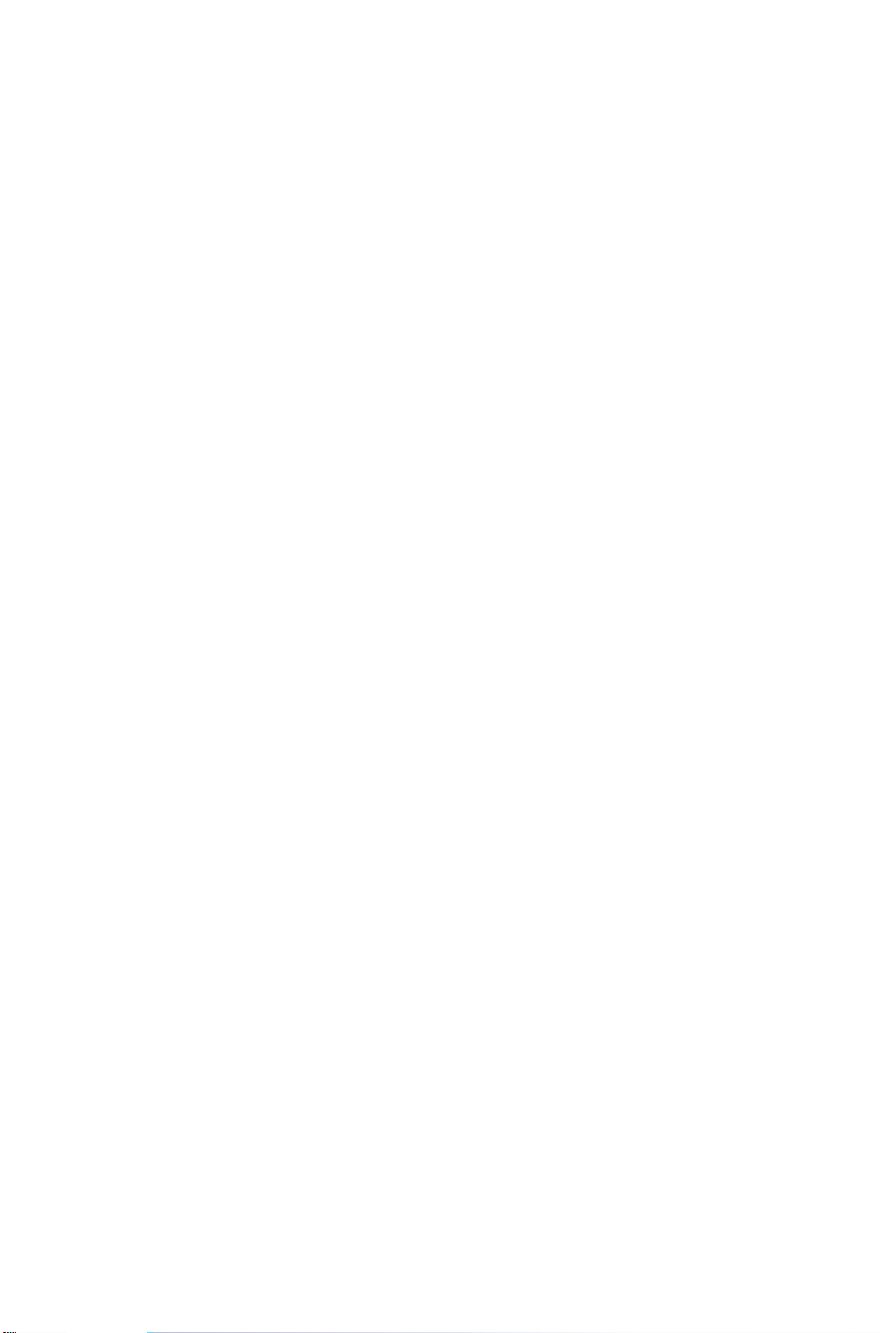
Springer Theses
Recognizing Outstanding Ph.D. Research
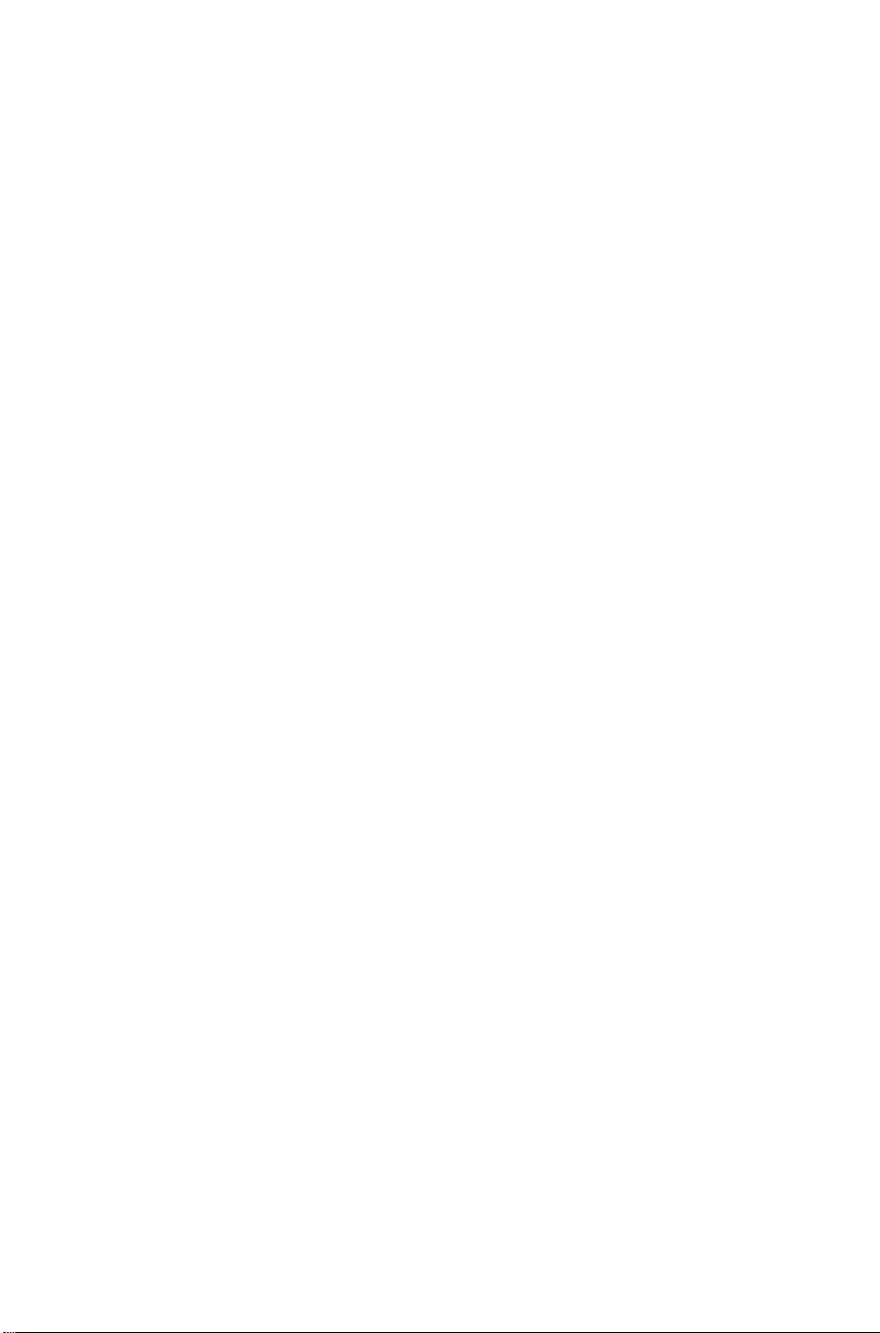
Aims and Scope
The series “Springer Theses” brings together a selec tion of the very best Ph.D.
theses from around the world and across the physical sciences. Nominated and
endorsed by two recognized specialists, each published volume has been selected
for its scientific excellence and the high impact of its contents for the pertinent field
of research. For greater accessibility to non-specialists, the published versions
include an extended introduction, as well as a foreword by the student’s supervisor
explaining the special relevance of the work for the field. As a whole, the series will
provide a valuable resource both for newcomers to the research fields described,
and for other scientists seeking detailed background information on special
questions. Finally, it provides an accredited documentation of the valuable
contributions made by today’s younger generation of scientists.
Theses are accepted into the series by invited nomination only
and must fulfill all of the following criteria
• They must be written in good English.
• The topic should fall within the confines of Chemistry, Physics, Earth Sciences,
Engineering and related interdisciplinary fields such as Materials, Nanoscien ce,
Chemical Engineering, Complex Systems and Biophysics.
• The work reported in the thesis must represent a significant scientific advance.
• If the thesis includes previously published material, permission to reproduce this
must be gained from the respective copyright holder.
• They must have been examined and passed during the 12 months prior to
nomination.
• Each thesis should include a foreword by the supervisor outlining the signifi-
cance of its content.
• The theses should have a clearly defined structure incl uding an introduction
accessible to scientists not expert in that particular field.
More information about this series at http://www.springer.com/series/8790
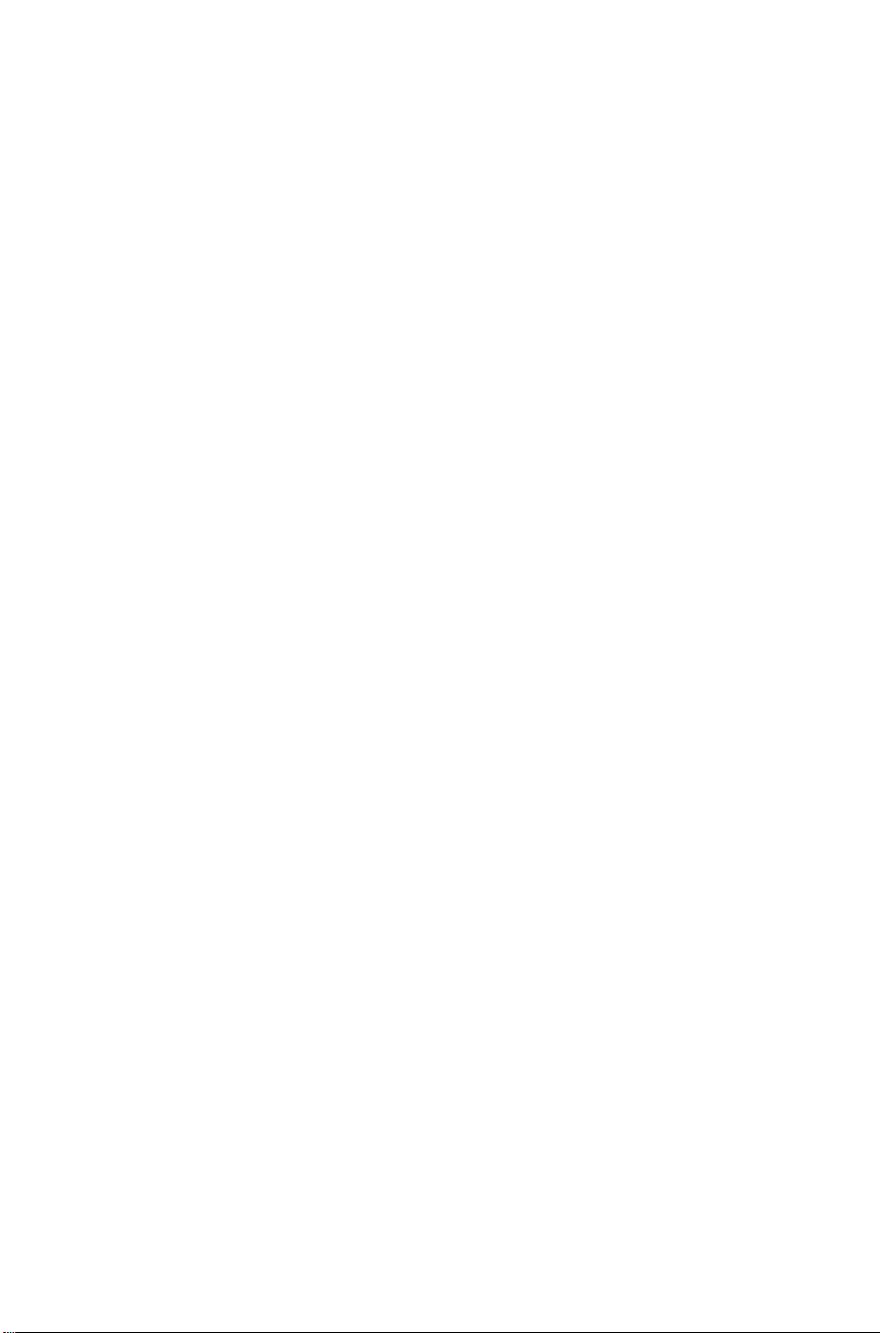
Olga Isupova
Machine Learning Methods
for Behaviour Analysis
and Anomaly Detection
in Video
Doctoral Thesis accepted by
the University of Sheffield, Shef field, UK
123
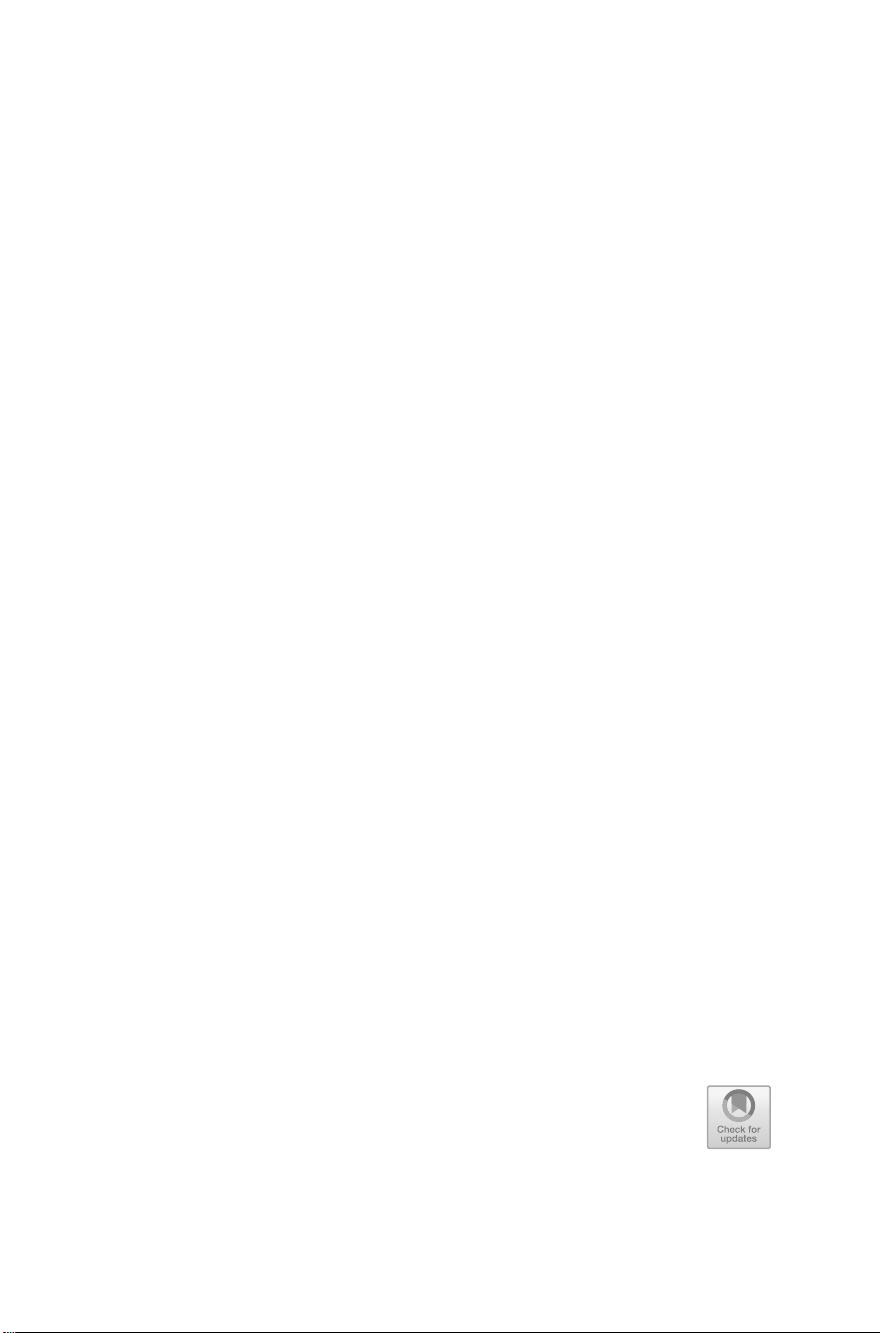
Author
Dr. Olga Isupova
Department of Engineering Science
University of Oxford
Oxford
UK
Supervisor
Prof. Lyudmila Mihaylova
University of Sheffield
Sheffield
UK
ISSN 2190-5053 ISSN 2190-5061 (electronic)
Springer Theses
ISBN 978-3-319-75507-6 ISBN 978-3-319-75508-3 (eBook)
https://doi.org/10.1007/978-3-319-75508-3
Library of Congress Control Number: 2018931499
© Springer International Publishing AG, part of Springer Nature 2018
This work is subject to copyright. All rights are reserved by the Publisher, whether the whole or part
of the material is concerned, specifically the rights of translation, reprinting, reuse of illustrations,
recitation, broadcasting, reproduction on microfilms or in any other physical way, and transmission
or information storage and retrieval, electronic adaptation, computer software, or by similar or dissimilar
methodology now known or hereafter developed.
The use of general descriptive names, registered names, trademarks, service marks, etc. in this
publication does not imply, even in the absence of a specific statement, that such names are exempt from
the relevant protective laws and regulations and therefore free for general use.
The publisher, the authors and the editors are safe to assume that the advice and information in this
book are believed to be true and accurate at the date of publication. Neither the publisher nor the
authors or the editors give a warranty, express or implied, with respect to the material contained herein or
for any errors or omissions that may have been made. The publisher remains neutral with regard to
jurisdictional claims in published maps and institutional affiliations.
Printed on acid-free paper
This Springer imprint is published by the registered company Springer
International Publishing AG part of Springer Nature
The registered company address is: Gewerbestrasse 11, 6330 Cham, Switzerland