Traditional testing faces significant complexity issues due to the increasing number of data, paths, combinations, permutations, and so on. Various testing methods have been proposed and used to improve the quality and reliability of software. As one branch of software testing, combinatorial testing (CT) is one black-box testing method to identify faults caused by the interaction of a few components. CT is considered a hard problem due to its exponential complexity: A system with 30 choice items may need to explore 230 combinations and this is time- and effort-consuming. Many approaches have been proposed in the last 40 years using various theo- retical models or techniques such as Latin square, orthogonal array, covering array, machine learning. In the past 20 years, the evolutionary solutions of combinatorial testing, such as AETG, IPO, have been proposed to generate a small set of test cases that achieves 100% test coverage. However, in spite of significant progress, it is still difficult to apply CT for a system of moderate sizes such as 100 choice items. These solutions mainly focus on test coverage and not much work on fault identification. This book proposes a computational approach to address the CT problem; instead of general purpose machine learning algorithms to learn from cases, it explores the CT structure to eliminate combinations for consideration, and the exploration can be done in a parallel manner, and the process can be done in a cloud environment where parallel processing is a standard feature. This approach allows performing CT for a system with size of 240, with 2240 combinations to consider. Thus, effectively, CT can run on a large system. This book intends to propose a faulty location analysis solution of CT as well as review existing CT solutions. Chapter 1 reviews existing combinatorial designs and CT solutions of test case generation. Chapter 2 discusses CT practical application in cloud computing and compares existing faulty location analysis solutions in CT. Chapter 3 introduces adaptive reasoning (AR) algorithm in multi-tenancy Software-as-a-Service (SaaS) system. In the next three chapters, it describes the formal definitions of test algebra (TA), discusses the related optimizations of TA, and simulates TA in cloud environment. The last three chapters propose an v vi Preface integrated Testing-as-a-Service (TaaS) design with AR and TA, discuss the related testing strategies, and simulate the proposed TaaS design to solve a large-scale CT problem in cloud environment. This book can serve as reference text for graduate students and researchers who work in combinatorial testing area. It should also be interesting to those who work in fault identification, fault location, and other related fields in testing. Practitioners may be inspired by this book in testing plan design. Due to the limitation of our knowledge, we could not provide more details in relationship between existing faults and potential faults now. But our research moves forward in fault location analysis step-by-step. You are welcome to contact us, if you have any comments and suggestions. This work is built on many outstanding CT work in the past, and their contri- butions are greatly appreciated. Prof. Charles Colbourn of Arizona State University, a leading expert on CT, was a co-author of some of research papers published. Prof. Wenjun Wu of Beihang University provided the initial computing environment for us to perform computational experiments, and our great friend Tony provided 40 large servers for us to perform CT experiments on a system of 250 choice items. By the way, the data generated by the 250 is so large that it will take months just to transfer data. The editors at Springer, Celine Chang and Jane Li, are always helpful. We are grateful to them for their constructive suggestions and patience. Finally, we are also indebted to our family members who suffered through the writing of this work that seems to last forever.
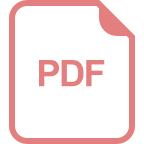
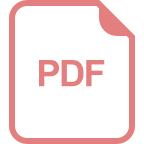
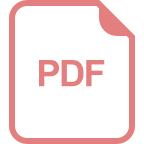
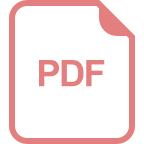
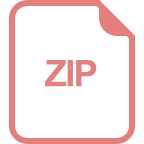
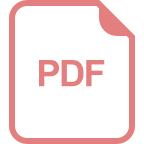
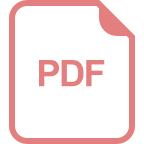
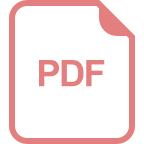
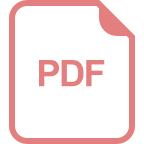
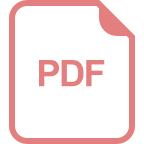
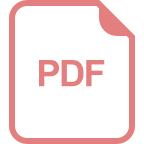
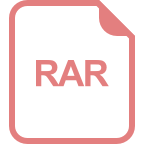
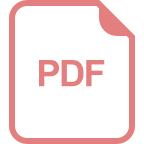
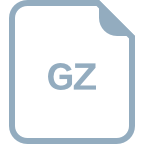
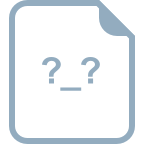
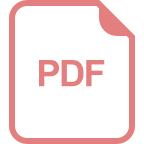
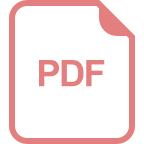
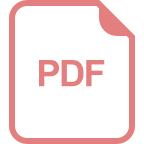
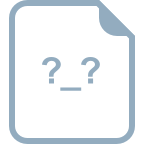
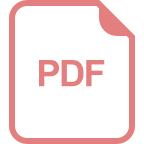
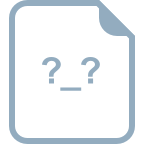
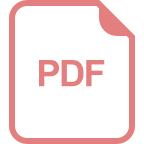
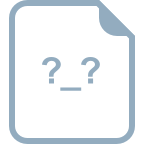
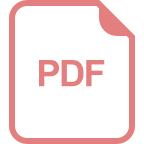
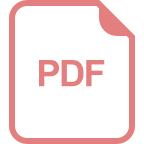
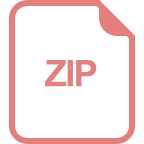
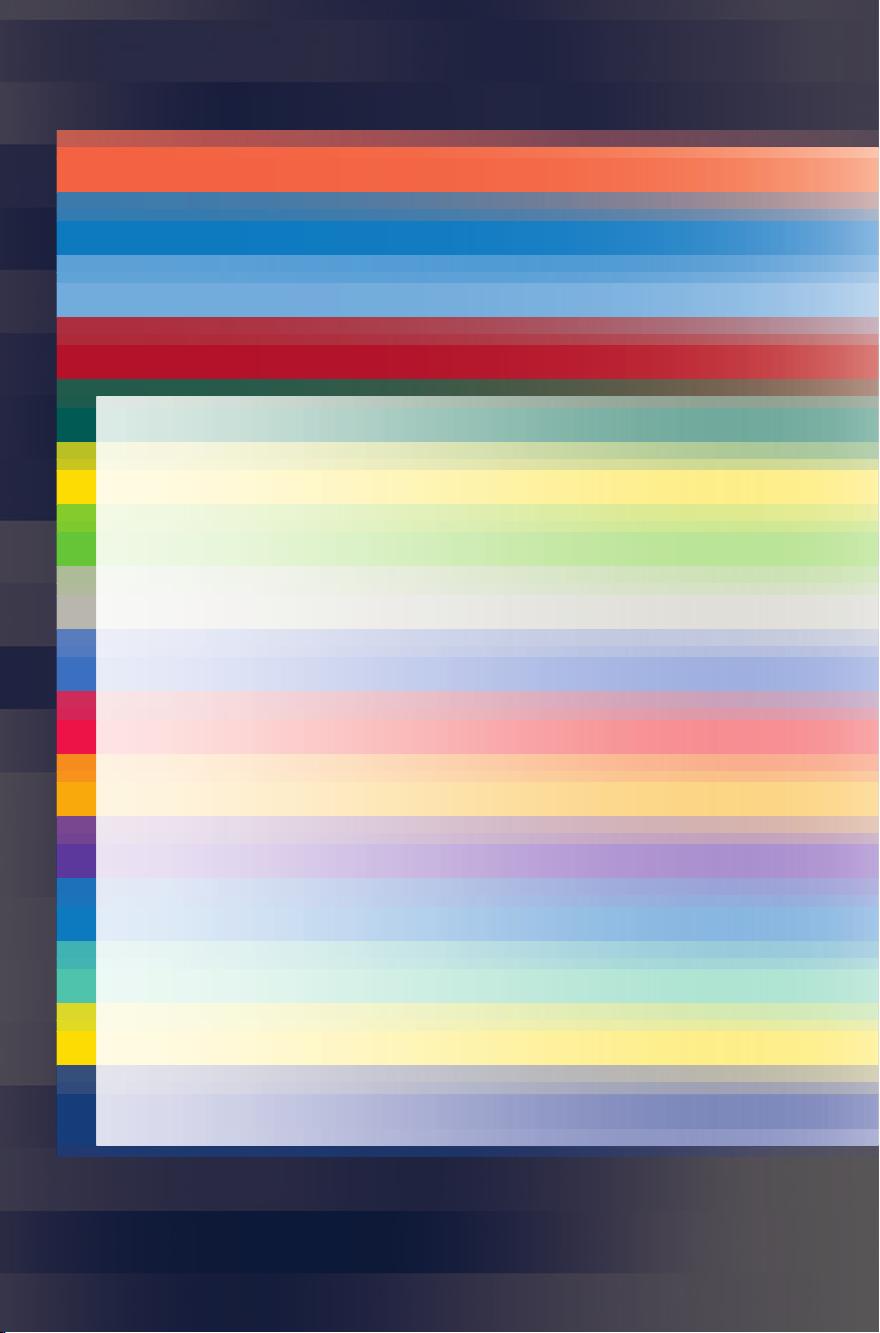
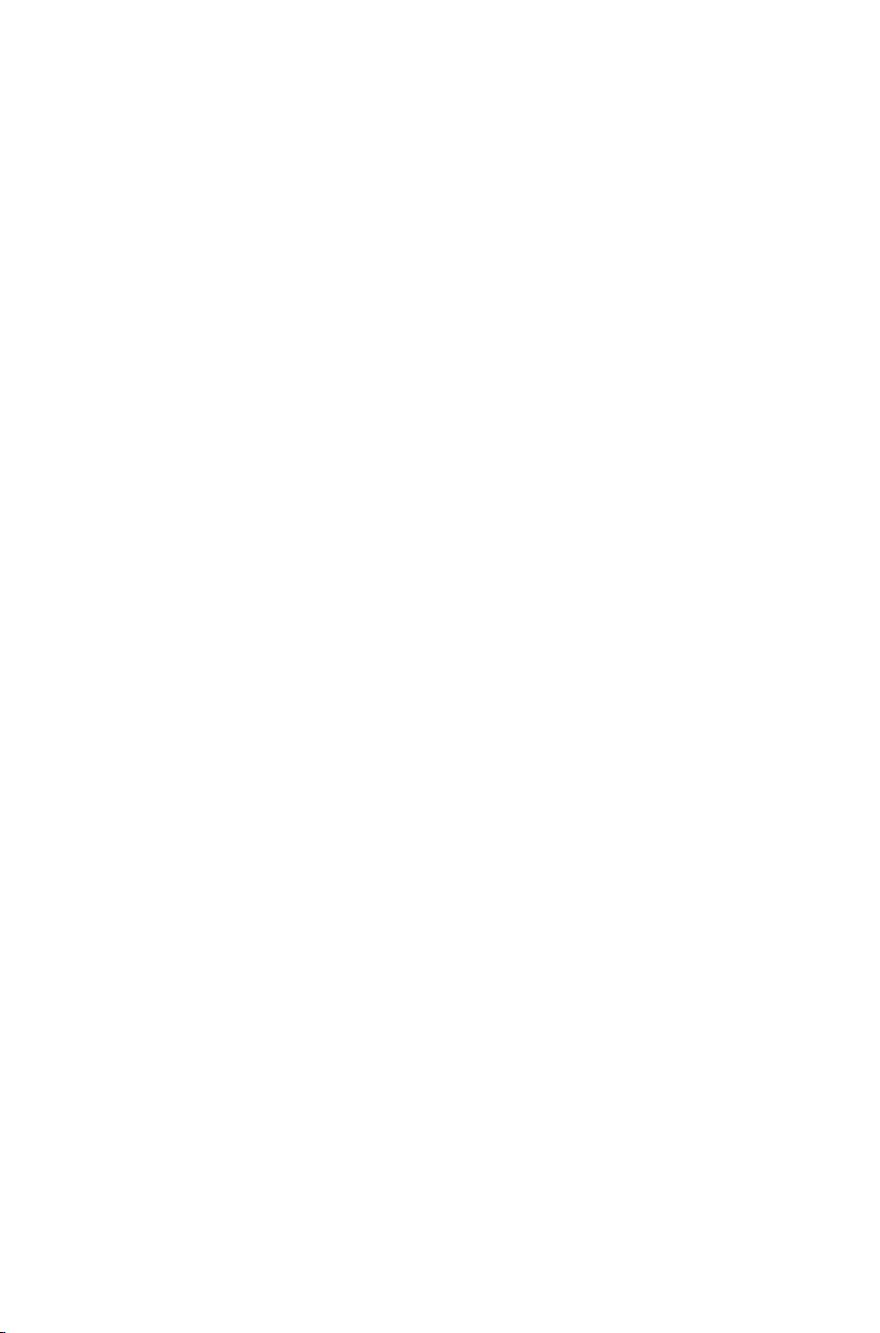
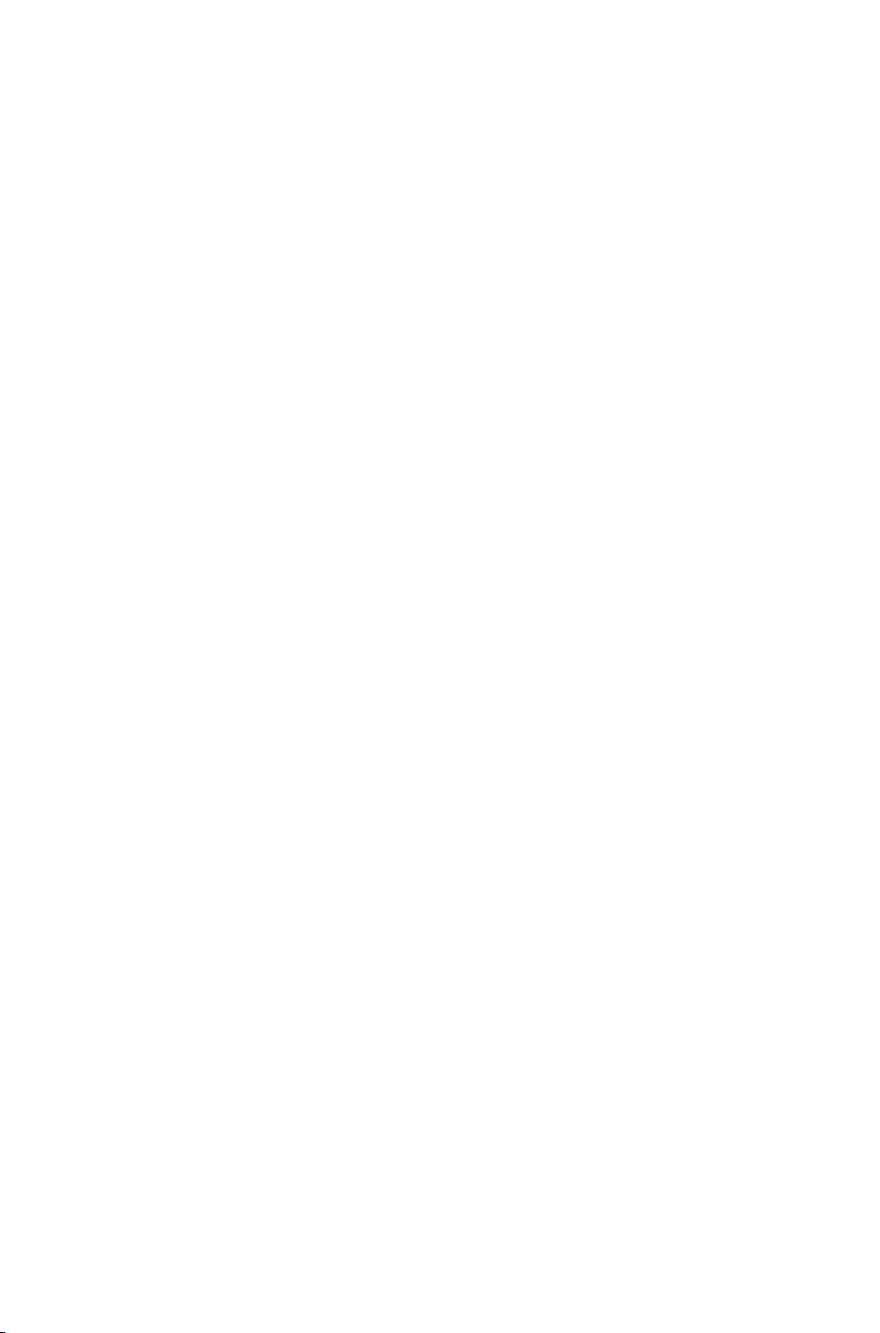
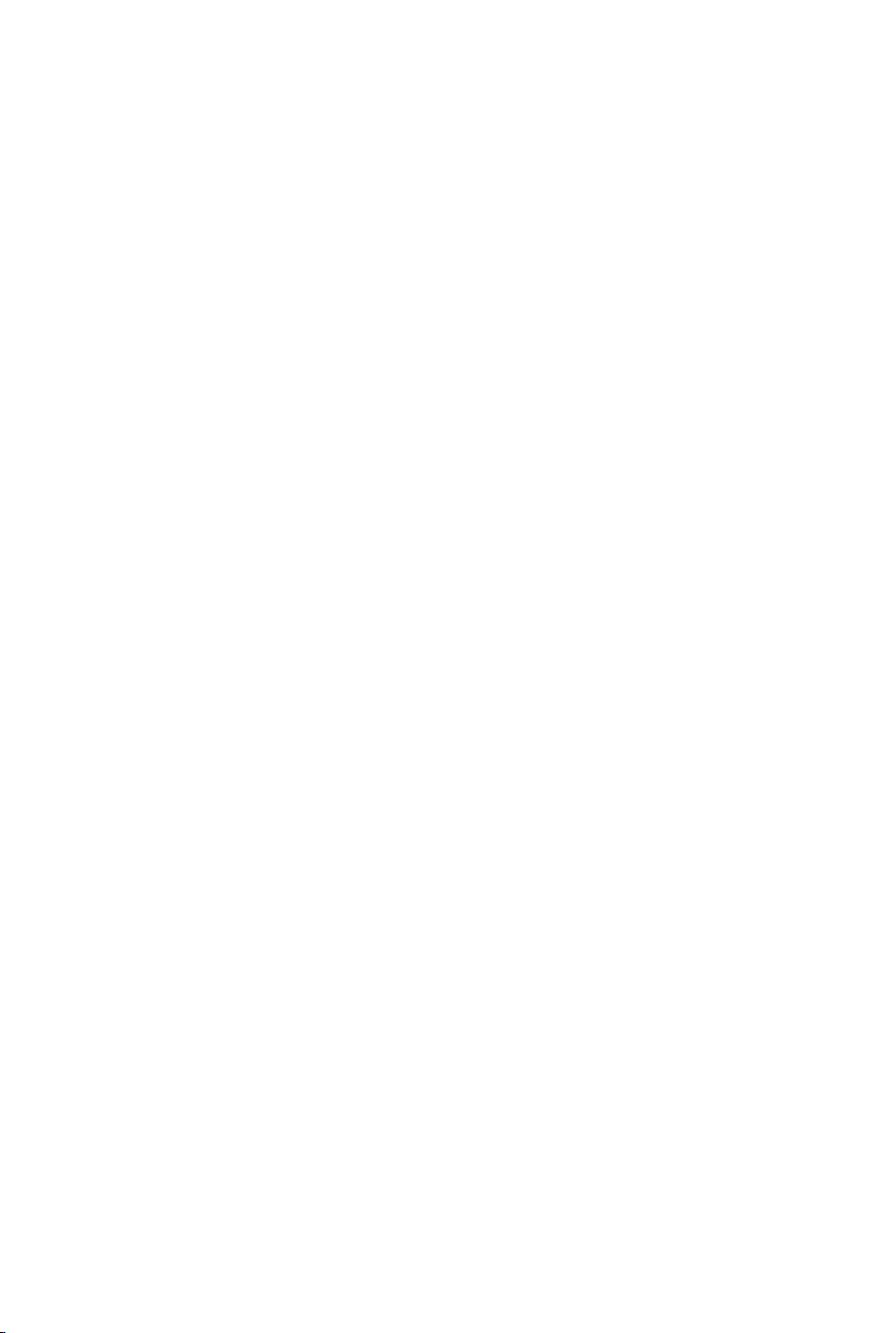
剩余133页未读,继续阅读
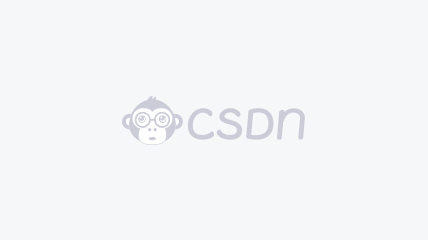

- 粉丝: 35
- 资源: 367
我的内容管理 展开
我的资源 快来上传第一个资源
我的收益
登录查看自己的收益我的积分 登录查看自己的积分
我的C币 登录后查看C币余额
我的收藏
我的下载
下载帮助

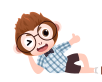
最新资源
- Mini-Imagenet数据集文件
- Matlab实现SSA-CNN-BiLSTM麻雀算法优化卷积双向长短期记忆神经网络时间序列预测(含完整的程序,GUI设计和代码详解)
- PxCook.rar 自动生成HTML、CSS代码
- 52036280467357957001734701299977.jpg
- 52292422648120956871733523098304.jpg
- 你的第一个博客-全套运行代码
- Node.JS网盘系统
- 车辆、飞机、船检测22-YOLO(v5至v11)、COCO、CreateML、Paligemma、TFRecord、VOC数据集合集.rar
- 时间转换工具(BD时、GPS时、UTC时、北京时间相互转换,可计算日期)
- 坐标转换工具(LBH、XYZ、NEH互相转换,可批量)

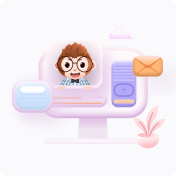
