public class GA {
private int ChrNum = 10; //染色体数量
private String[] ipop = new String[ChrNum]; //一个种群中染色体总数
private int generation = 0; //染色体代号
public static final int GENE = 46; //基因数
private double bestfitness = Double.MAX_VALUE; //函数最优解
private int bestgenerations; //所有子代与父代中最好的染色体
private String beststr; //最优解的染色体的二进制码
/**
* 初始化一条染色体(用二进制字符串表示)
*/
private String initChr() {
String res = "";
for (int i = 0; i < GENE; i++) {
if (Math.random() > 0.5) {
res += "0";
} else {
res += "1";
}
}
return res;
}
/**
* 初始化一个种群(10条染色体)
*/
private String[] initPop() {
String[] ipop = new String[ChrNum];
for (int i = 0; i < ChrNum; i++) {
ipop[i] = initChr();
}
return ipop;
}
/**
* 将染色体转换成x,y变量的值
*/
private double[] calculatefitnessvalue(String str) {
//二进制数前23位为x的二进制字符串,后23位为y的二进制字符串
int a = Integer.parseInt(str.substring(0, 23), 2);
int b = Integer.parseInt(str.substring(23, 46), 2);
double x = a * (6.0 - 0) / (Math.pow(2, 23) - 1); //x的基因
double y = b * (6.0 - 0) / (Math.pow(2, 23) - 1); //y的基因
//需优化的函数
double fitness = 3 - Math.sin(2 * x) * Math.sin(2 * x)
- Math.sin(2 * y) * Math.sin(2 * y);
double[] returns = { x, y, fitness };
return returns;
}
/**
* 轮盘选择
* 计算群体上每个个体的适应度值;
* 按由个体适应度值所决定的某个规则选择将进入下一代的个体;
*/
private void select() {
double evals[] = new double[ChrNum]; // 所有染色体适应值
double p[] = new double[ChrNum]; // 各染色体选择概率
double q[] = new double[ChrNum]; // 累计概率
double F = 0; // 累计适应值总和
for (int i = 0; i < ChrNum; i++) {
evals[i] = calculatefitnessvalue(ipop[i])[2];
if (evals[i] < bestfitness){ // 记录下种群中的最小值,即最优解
bestfitness = evals[i];
bestgenerations = generation;
beststr = ipop[i];
}
F = F + evals[i]; // 所有染色体适应值总和
}
for (int i = 0; i < ChrNum; i++) {
p[i] = evals[i] / F;
if (i == 0)
q[i] = p[i];
else {
q[i] = q[i - 1] + p[i];
}
}
for (int i = 0; i < ChrNum; i++) {
double r = Math.random();
if (r <= q[0]) {
ipop[i] = ipop[0];
} else {
for (int j = 1; j < ChrNum; j++) {
if (r < q[j]) {
ipop[i] = ipop[j];
}
}
}
}
}
/**
* 交叉操作 交叉率为60%,平均为60%的染色体进行交叉
*/
private void cross() {
String temp1, temp2;
for (int i = 0; i < ChrNum; i++) {
if (Math.random() < 0.60) {
int pos = (int)(Math.random()*GENE)+1; //pos位点前后二进制串交叉
temp1 = ipop[i].substring(0, pos) + ipop[(i + 1) % ChrNum].substring(pos);
temp2 = ipop[(i + 1) % ChrNum].substring(0, pos) + ipop[i].substring(pos);
ipop[i] = temp1;
ipop[(i + 1) / ChrNum] = temp2;
}
}
}
/**
* 基因突变操作 1%基因变异
*/
private void mutation() {
for (int i = 0; i < 4; i++) {
int num = (int) (Math.random() * GENE * ChrNum + 1);
int chromosomeNum = (int) (num / GENE) + 1; // 染色体号
int mutationNum = num - (chromosomeNum - 1) * GENE; // 基因号
if (mutationNum == 0)
mutationNum = 1;
chromosomeNum = chromosomeNum - 1;
if (chromosomeNum >= ChrNum)
chromosomeNum = 9;
String temp;
String a; //记录变异位点变异后的编码
if (ipop[chromosomeNum].charAt(mutationNum - 1) == '0') { //当变异位点为0时
a = "1";
} else {
a = "0";
}
//当变异位点在首、中段和尾时的突变情况
if (mutationNum == 1) {
temp = a + ipop[chromosomeNum].substring(mutationNum);
} else {
if (mutationNum != GENE) {
temp = ipop[chromosomeNum].substring(0, mutationNum -1) + a
+ ipop[chromosomeNum].substring(mutationNum);
} else {
temp = ipop[chromosomeNum].substring(0, mutationNum - 1) + a;
}
}
//记录下变异后的染色体
ipop[chromosomeNum] = temp;
}
}
public static void main(String args[]) {
GA Tryer = new GA();
Tryer.ipop = Tryer.initPop(); //产生初始种群
String str = "";
//迭代次数
for (int i = 0; i < 100000; i++) {
Tryer.select();
Tryer.cross();
Tryer.mutation();
Tryer.generation = i;
}
double[] x = Tryer.calculatefitnessvalue(Tryer.beststr);
str = "最小值" + Tryer.bestfitness + '\n' + "第"
+ Tryer.bestgenerations + "个染色体:<" + Tryer.beststr + ">" + '\n'
+ "x=" + x[0] + '\n' + "y=" + x[1];
System.out.println(str);
}
}

若明天不见
- 粉丝: 1w+
- 资源: 272
最新资源
- 基于flink的实时数仓详细文档+全部资料.zip
- 基于Flink的数据同步工具详细文档+全部资料.zip
- 基于Flink的数据流业务处理平台详细文档+全部资料.zip
- 基于flink的物流业务数据实时数仓建设详细文档+全部资料.zip
- 外卖时间数据,食品配送时间数据集,外卖影响因素数据集(千条数据)
- 基于flink的异构数据源同步详细文档+全部资料.zip
- 基于flink的营销系统详细文档+全部资料.zip
- 基于Flink对用户行为数据的实时分析详细文档+全部资料.zip
- 基于Flink分析用户行为详细文档+全部资料.zip
- 基于flink可以创建物理表的catalog详细文档+全部资料.zip
- 基于Flink流批一体数据处理快速集成开发框架、快速构建基于Java的Flink流批一体应用程序,实现异构数据库实时同步和ETL,还可以让Flink SQL变得
- 太和-圣德西实施—部门负责人以上宣贯培训大纲.doc
- 太和-圣德西实施—部门负责人非HR的HRM培训.pptx
- 太和-圣德西实施—宣贯培训大纲.docx
- 基于Flink流处理的动态实时亿级全端用户画像系统可视化界面详细文档+全部资料.zip
- 基于Flink全端用户画像商品推荐系统详细文档+全部资料.zip
资源上传下载、课程学习等过程中有任何疑问或建议,欢迎提出宝贵意见哦~我们会及时处理!
点击此处反馈


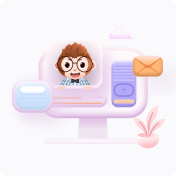